Machine Learning Solutions: Overview and Sources
Table of Contents
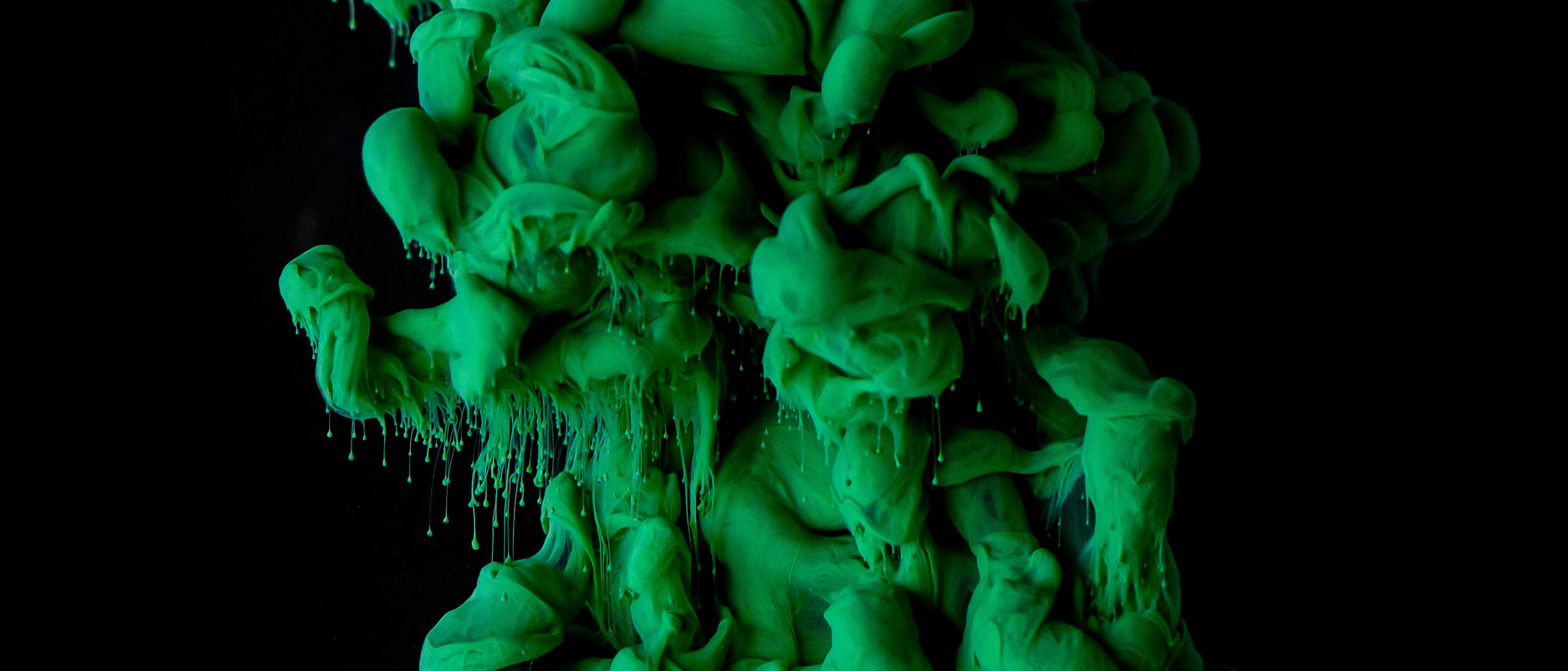
For a while now, we’ve been discussing AI as a service and ML as a service. That’s because AI projects have become more and more common among various businesses. Today, these projects are not just the way to get a competitive advantage over other businesses but a necessity that enables:
- Automation of business workflows,
- Leveraging unstructured data and finding patterns in big data,
- Personalization of customer support,
- Top-tier complex analytics,
- Predictions of multi-factor environmental issues,
- Anomaly and error detection, etc.
that weren’t possible before our information age.
That’s why we’ll be continuing our topic by introducing you to the concept of machine learning solutions, the ready-to-use services that do not require any in-depth knowledge in the spheres of artificial intelligence or data science to use ML for the advantage of your business.
What Are Machine Learning Solutions?
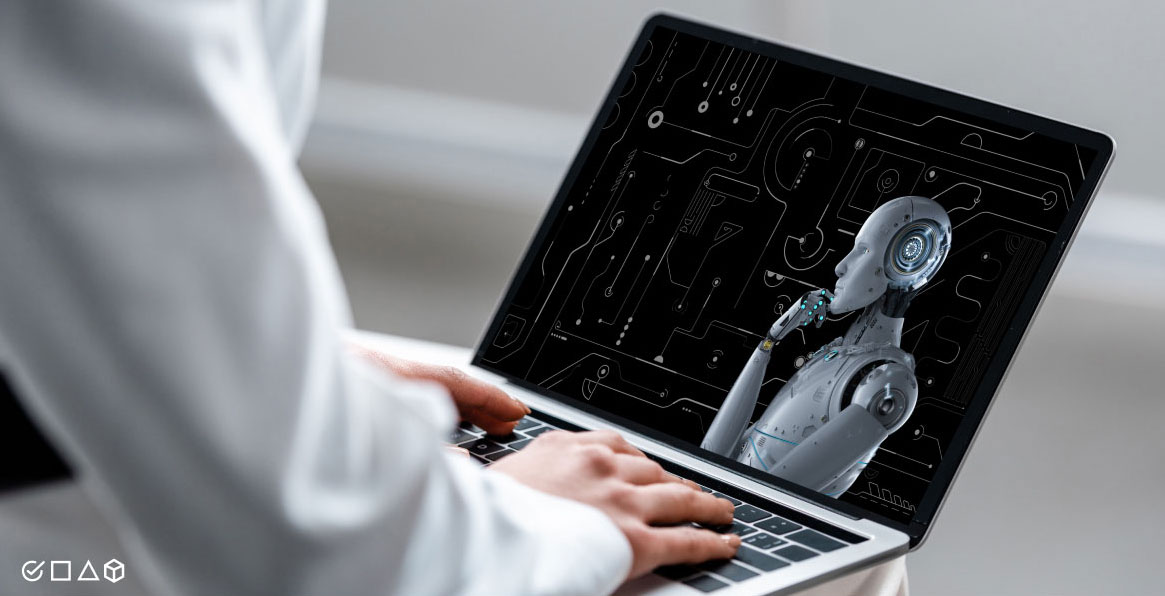
Both AIaaS and MLaaS are quite popular among businesses centered on other products and services rather than being AI-focused. Both of these types of as-a-service solutions significantly facilitate the design and deployment of AI models. Some of these solutions might require a certain understanding of the principles of how algorithms work or offer end-to-end services that allow you to save resources (human, financial, and time) instead.
So what is the meaning of machine learning solutions? Ready ML solutions deliver the complete usable solution to a business. This commonly goes together with the preparation of the AI project agenda, processing of the data, and model deployment and maintenance. Certain services might also include data collection, which makes them optimal for businesses that wish to focus on their core goals.
An important and distinctive advantage of ML solutions is that they commonly cover the whole spectrum of platforms and devices. From low-power edge devices to heavy machinery set within the industrial environment, machine learning solutions ensure that the designed algorithm works everywhere and with no limitations.
Naturally, there are limitations to ready ML solutions, as well. Specifically, end-to-end options may usually lack the finesse and attention to the details of your specific project. While this might not be a dealbreaker for most cases, unique AI projects might require more professional consideration.
Still, ML solutions are widely spread today as they’re convenient, allow saving time and effort, and use the benefits that AI brings for any business.
Where to Get a Good ML Solution?
If you’re our regular reader, you’re probably familiar with the deal by now: there are several IT giants, the Big Four if you will, that offer flexible and comprehensive machine learning solutions for nearly any business. Let’s take a cursory look at each of them to outline their weaker and stronger sides before we break down how to look for the ML solutions that fit your case best.
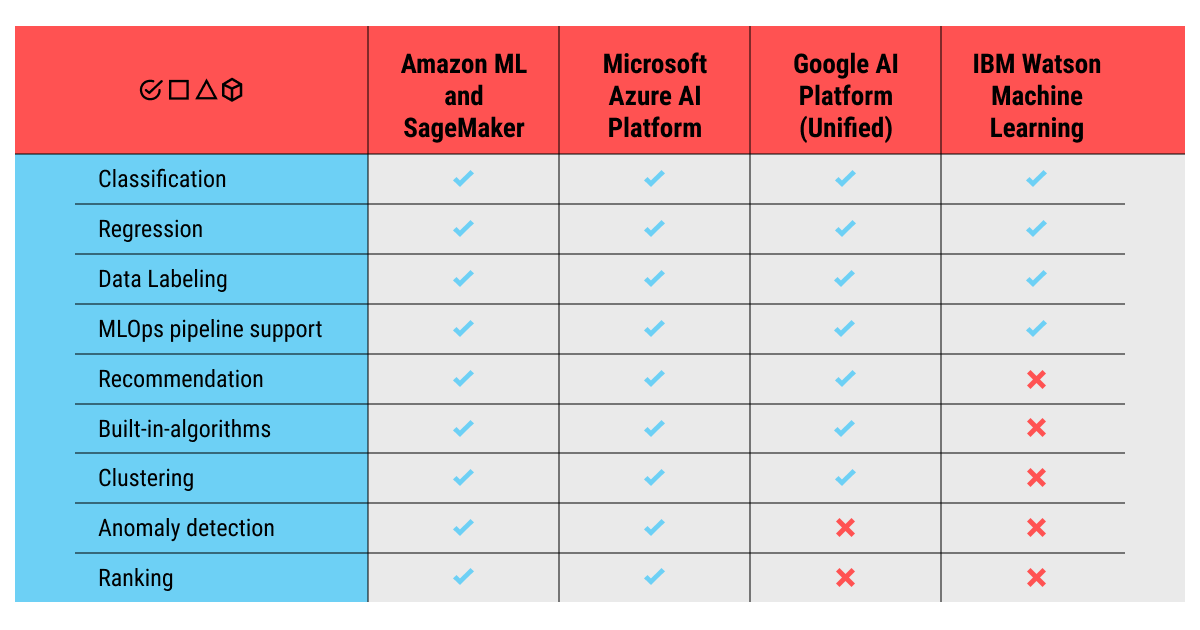
Google Cloud. Offering a comprehensive and wide-reaching set of machine learning solutions from supervised ML to deep learning, Google has a visibly strong NLP focus. Its solutions are also great for image analysis (although there are weaker points in facial and food recognition), although, surprisingly, present a rather lacking video analysis.
AWS Marketplace. Another of the ML giants, Amazon’s AWS shows decent results with NLP solutions. The translation models might be a bit on the weaker side and there’s an evident insufficient range of video analysis solutions (no audio transcription, translation, keywords extraction, annotation, and so on).
Microsoft Azure. Comparably good NLP solutions, reliable image analysis (a bit lacking in the areas of no logo detection and similar-image search), and good video analysis make Microsoft one of the best choices to look for an ML solution.
IBM’s Watson. There’s a lack of focus on image analysis for IBM ML solutions, which is compensated by a fine range of NLP solutions (somewhere in between the variety of Microsoft and Google).
How to Choose a Vendor of Machine Learning Solutions?
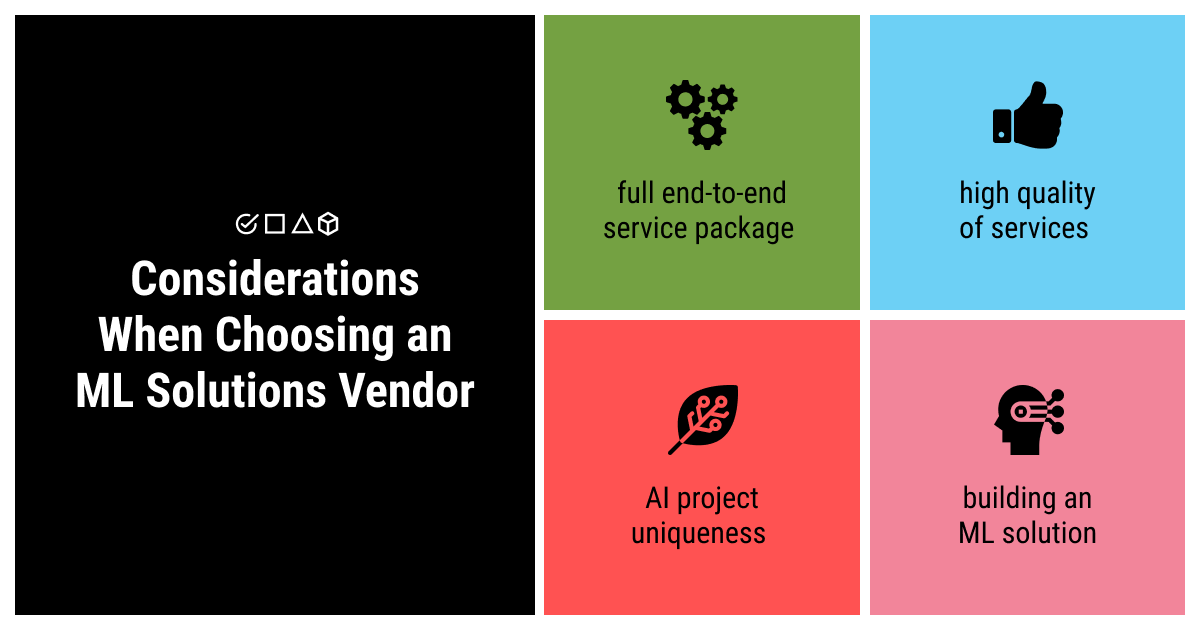
As you can see, the big players offer a comparable range of advanced services for custom modeling and end-to-end ML. But should you choose a big player like Google or Amazon or IBM, or instead opt for a smaller, stand-alone, or more ML-focused vendor?
There are a few differences between the services offered by different platforms. Here are a few tips to keep in mind while you’re choosing:
- Stand-alone vendors are usually more prone to offer full packages of services, end-to-end including the maintenance and support of your model in the long term. However, the set of services is commonly negotiable and depends on how much development and data science work you’re prepared (or willing) to do.
- Big players offer verified high quality and accuracy of services that were perfected by multiple users on a variety of devices and platforms, with comprehensive support all around. At the same time, smaller businesses are more of a gamble: they might have a comparable (or even better) level of quality of their ML solutions but they might also deliver less desirable results.
- Your industry plays a crucial role in choosing a vendor of ML solutions. Bigger players commonly offer machine learning solutions that are applicable for a wider set of cases, whether it’s for banks, manufacturing, transportation networks, or any other type of business. Something unique or unusual, however, might require a more attentive approach of a smaller, more dedicated vendor.
- The process of building an ML solution for your business might be similar among the vendors. Still, keep attention to all the steps that a great solution should have. First, the analysis of the goals of your AI project is required, paired with the understanding of your business and your expectations. Second, data collection may be offered if you still didn’t gather a dataset. This is followed by the processing of the data, from cleaning to anonymization to annotation. Third, feature engineering and model design follow. Finally, the model is trained and deployed. Don’t forget also about the maintenance and regular updates, which are a must in our rapidly changing environment.
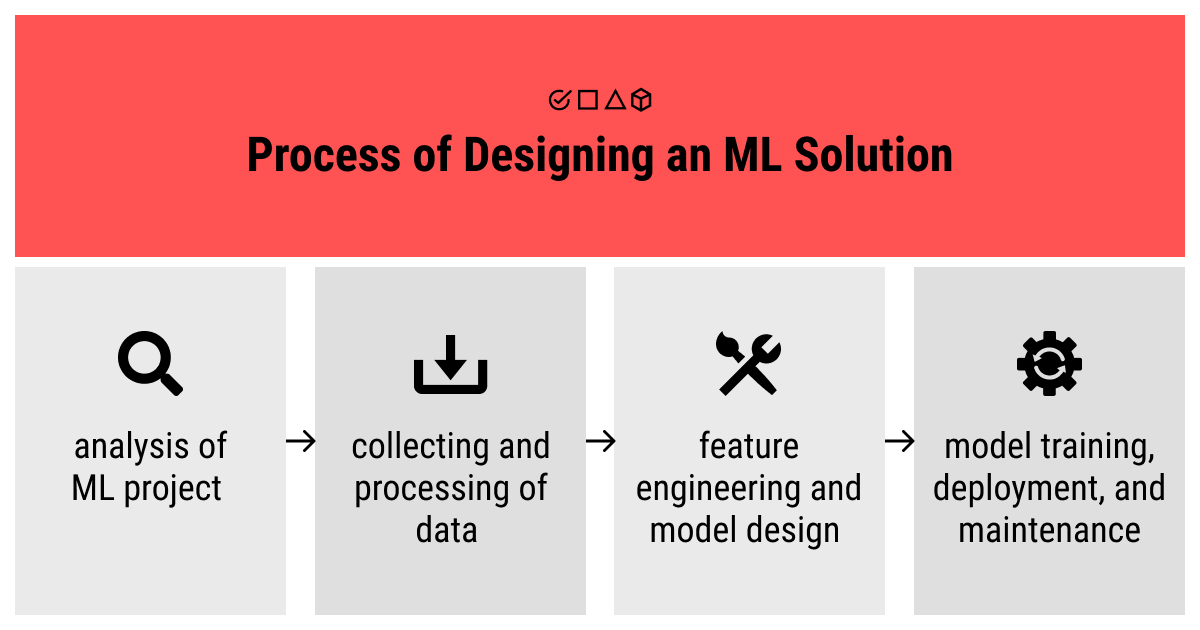
A Few More Options to Search for Great Machine Learning Solutions
To balance out the biggest players, here are a few short reviews of alternative businesses that you might find useful when choosing the best machine learning solution vendor for your AI project:
- Alteryx has a strong focus on rule-based recommendation systems and process automation. This vendor is named among the industry leaders outside of the Big Four as Alteryx strives to build supervised ML solutions that require little to no help from dedicated data scientists and engineers.
- RapidMiner is strongest with the analytics of big data. The vendor offers high-quality end-to-end ML solutions, starting from data processing to model design and training to model deployment.
- SAS Visual Data Mining and Machine Learning is a good choice if you need a multimodal approach and do not care for substantial computational capacity. SAS solutions automate the whole process of creating and training the model, as well as implementing it on different devices.
- SAP Leonardo offers both ready machine learning cloud solutions and a comprehensive machine learning kit for building predictive models. It might not have the software range of the other vendors but it compensates with attention to detail and a thoughtful approach to any type of datasets, from text to image and video.
Naturally, there are far more options for ML solutions that we could possibly cover in this short article. If none of the reviewed vendors fit your needs, keep in mind the tips that we’ve discussed and keep looking for the specific features that’ll satisfy the goals of your machine learning project.
The Essential Takeaways: What a Machine Learning Solution Is and How to Choose the Best Option?
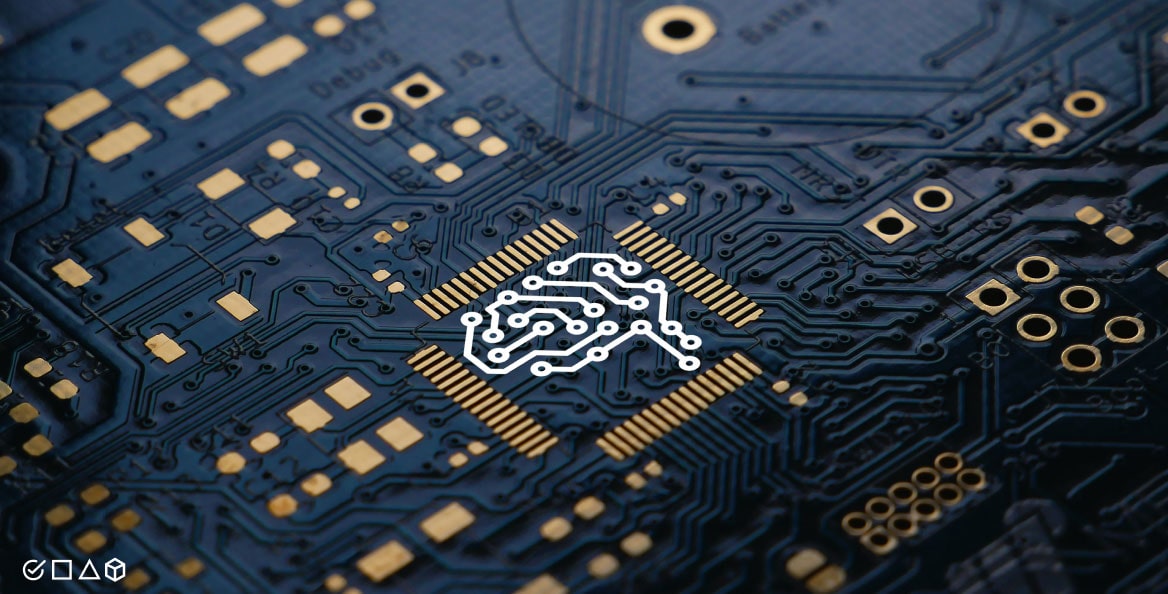
In summary, machine learning solutions can give your business a competitive edge by introducing AI into the processes even if you lack the resource capacity or expertise. With the growing demand for automation and predictive analysis offered by artificial intelligence, ML solutions become very popular and widespread.
The vendors offer a multitude of choices for businesses:
- from the possibility to build an ML model from scratch, which helps if your project is unique and requires special attention to details;
- to end-to-end, ready-to-use solutions, fully equipped with the deployment and maintenance services, which enable your business to focus on your core product.
Choosing a vendor might be a challenge as the number of ML solutions offered grows day by day. Consider both big and small vendors to see if the offered machine learning solutions fit the bill.
If you’re still not sure where to start, pay attention to what features the company of your choice offers. Answer these questions to get the idea of what you’re looking for:
- Is the ML solution fully built or does it require your knowledge and expertise in ML?
- What guarantees of high quality and accuracy does the vendor offer?
- If and how is the uniqueness of your AI project addressed?
- Are the essential steps of building an ML solution present?
Written by
Iryna is one of the dedicated members of the Label Your Data content team who has put all her efforts in developing our knowledge base. Iryna is a seasoned technical writer with wide-ranging experience in artificial intelligence, machine learning, and deep learning. She has been studying the basics of data annotation for many years and is now sharing her expertise on our blog. The technical realm is a true passion of hers, so make sure to check out other articles written by our talented Iryna!