Data Labelers: The Loyal Wingmen of Machine Learning
Table of Contents
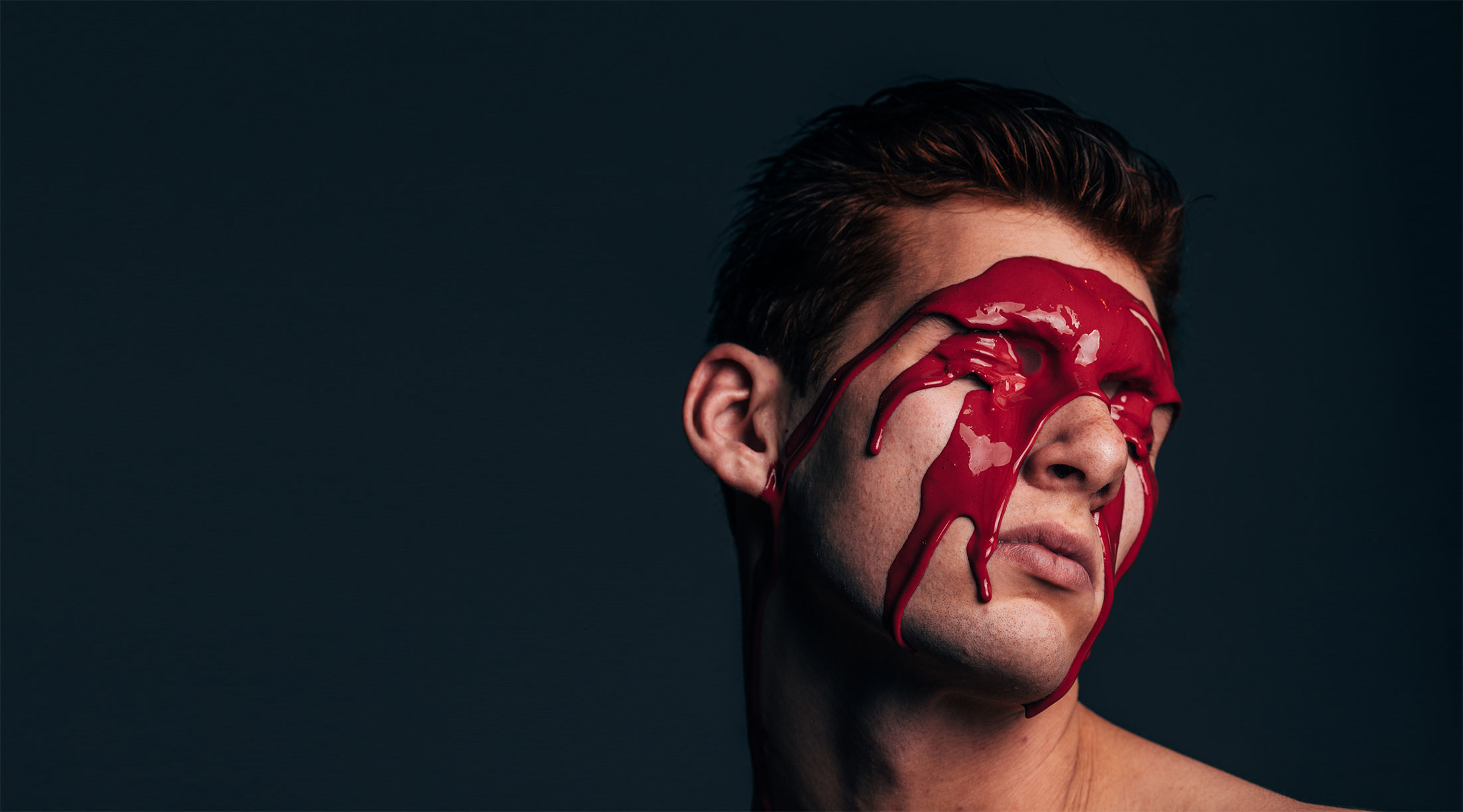
There's not a field that wasn't transformed by artificial intelligence. The variety of tasks that machines can do to entertain, enforce, and help humans do their work are nearly unlimited. As the technology of ML algorithms becomes more advanced, they naturally take on the jobs that were previously done by people. McKinsey reported that, by 2030, significant workplace and job requirement changes will be in place. 5% of the jobs will be completely eliminated by automation. At the same time, a third of the 60% of the jobs will be automated, leading the way of the transformations. The occupations most affected by this trend include physical labor, office support, and customer interaction jobs.
Still, AI and ML don't only "kill" the jobs but also create new opportunities. The experts agree that data scientists, AI engineers, and data labelers will flourish in this era of AI. And there's a good chance that data labelers could become one of the most in-demand jobs on the market given the constant need for data annotation and the time spent on this hidden task essential for nearly any AI project.
What Does a Data Labeler Do?
First, let's briefly talk about data annotation. Let's say you've built a model that uses image recognition to classify photos. How do you proceed? Computers don't see things as humans do. They lack the understanding that would allow explaining to them that a small furry creature with four legs and long whiskers is a cat. In order for a machine to understand that and be able to tell if there's a cat on a new photo you upload, you have to show it thousands of other photos with cats on it. That way, a computer will figure out what a cat is on its own.
However, it's not enough to show the photos to the machine. You need supervision in order for the computer to make correct predictions. That where the term "supervised machine learning" comes from. You need a human, a data labeler to tell the model that this is a cat on the image.
In a nutshell, a data labeler has to manually assign meaningful labels to the separate data pieces that will be later fed into the machine learning model. What we must not forget is that data labelers need to do a lot of tedious, boring work to annotate the photos, in other words, to properly label every photo with the tag that says 'cat'. While it doesn't seem complicated, this is where the majority of the work is done. 80% of the time of an AI project is dedicated to data processing and annotation. There's no magic and no flare to the process of annotation, and data labelers know this best of all.
Challenges of a Data Labeler's Job
The job requirements for a data labeler don't seem like much, with no specific training in development or knowledge of data science needed. It's a comparatively lower-skill job that you can even do online, as a freelancer, which is great both in the wake of the self-isolation mode brought by COVID-19 and the growing trend for workforce independence within the digital industrialism of the Information Age. Still, there are a few crucial challenges of the jobs for data labelers that we'd like to discuss in detail.
Specialization
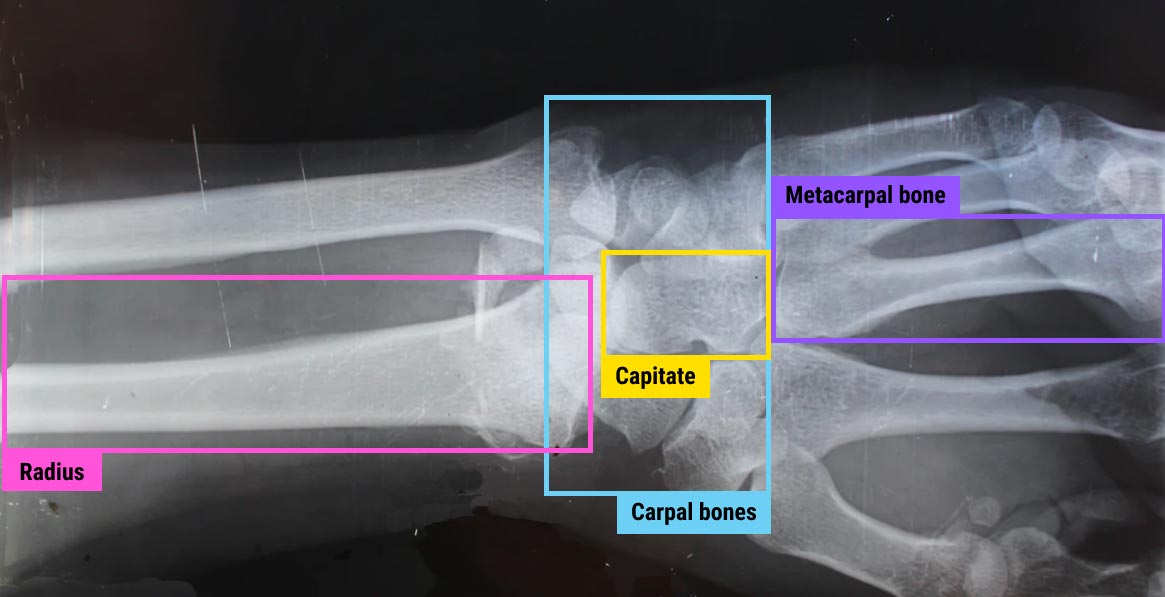
Although most data annotation jobs do not require any in-depth knowledge in other spheres, there are still projects that cannot be done by people without special training. This is usually true for very technical, highly-specialized industries. Healthcare is a great example to demonstrate this challenge of a data labeler's job.
There's a wide array of medical tasks that need AI for automated activities and the facilitation of human work. Reading CT and MR scans and deciphering X-rays are among them. Curiously, the task of recognizing a tumor on an X-ray is, in essence, the same as recognizing a dog on a photo you just uploaded to Facebook. For a machine, both are just a selection of pixels on an image or a scan. But there is an inherent difference between the labeling jobs that go into both of these tasks. Any human being (and even our toddlers) can tell what a dog looks like. But you require a very specific skill set to recognize a tumor on an X-ray.
In order to teach a machine to perform this task correctly, you need to draw the tumor outlines for thousands of X-rays, to pinpoint cancer cells and do it with the highest level of precision. And even then there's still a high chance that different specialists will disagree on the accuracy of certain annotations. This is true for the cases where the staining is faint and makes the interpretation extremely nuanced. Such cases may require not one, not two, but at least three specialists working on the same data set.
Obviously, highly-specialized areas commonly lack the personnel to do the annotation since the professionals are often busy without the additional task of labeling the data. All the while, training the data labeler in a specific field may require a lot of time and resources to make this option unviable. Thus, human resources in knowledge industries are one of the biggest challenges in data annotation.
Security
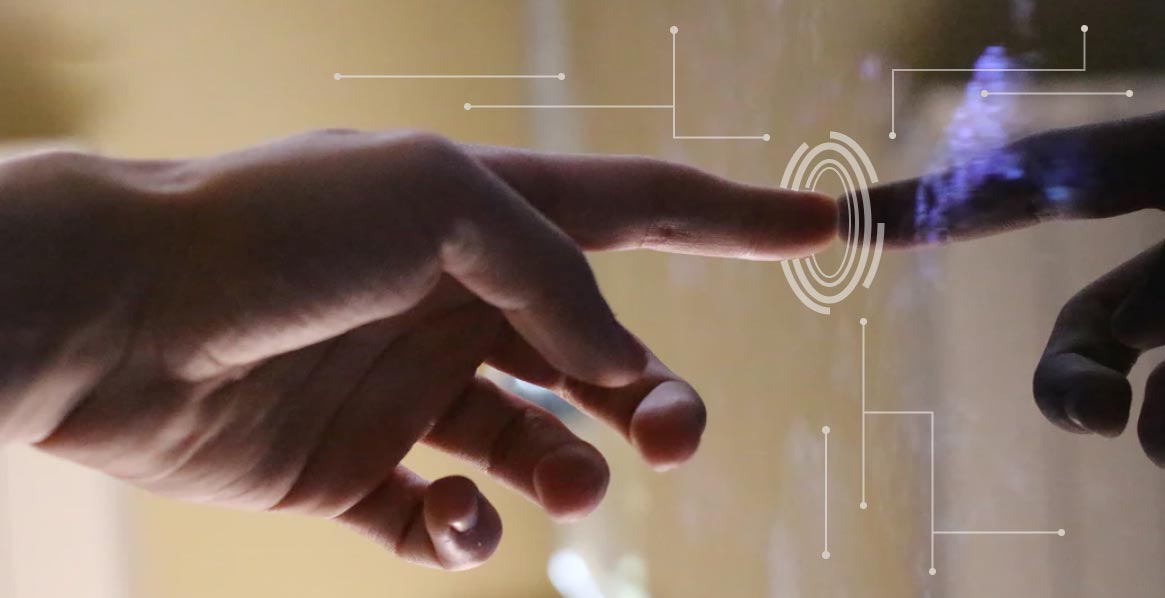
When it comes to data, security is always one of the biggest concerns, especially given the complexity of the subject. Data labelers have to understand the nature of data protection and how to deal with breaches and leakage of data in case it happens. The matter of sensitive data is even more complicated. The PII, as well as other types of data that can be used to identify a person should be treated with the utmost care. For this reason, NDAs are a common practice when hiring data labelers, as well as training in the industry standards compliance (such as GDPR and CCPA).
Still, there's the issue of location. A lot of data labelers work online, which doesn't let them participate in annotation projects that require increased security. Extra security measures are common on the premises of the outsourcing companies that work on annotation projects. For this reason, projects that require work on sensitive data are commonly outsourced to annotation enterprises that can ensure conformity with privacy and data protection protocols.
Languages
This challenge is specific to NLP that teaches machines to make sense of human languages. The thing is, algorithms often require to be trained with multiple languages in mind. GPT-3 (the ultimate case of a content-writing algorithm) is an illustrative example: it's great at speaking English, and not as good at other languages. In order to master them, it requires to be trained on the data annotated in those languages.
For this reason, multilingual teams of data labelers are not uncommon. Such teams are usually working remotely from different locations, and they require proper management to reinforce productive collaboration and adherence to the requirements of the annotation project. Besides, employing data labelers speaking multiple languages allows thinking about localization beforehand, which is never the wrong thing to do, especially with the projects that need analysis of sentiment and interpretation of subjective meanings.
Tools
Given the level of technology development, this challenge will be lessening substantially in the nearest years. However, it is still obvious that the annotation tools that exist today are not sufficient for effective and fast annotation. Probably, it would be easier to draw a bounding box around an object using an iPad than clicking a mouse. We will be talking more about the annotation tools in our next article. Subscribe now to stay tuned!
Data Labeler: Job Description
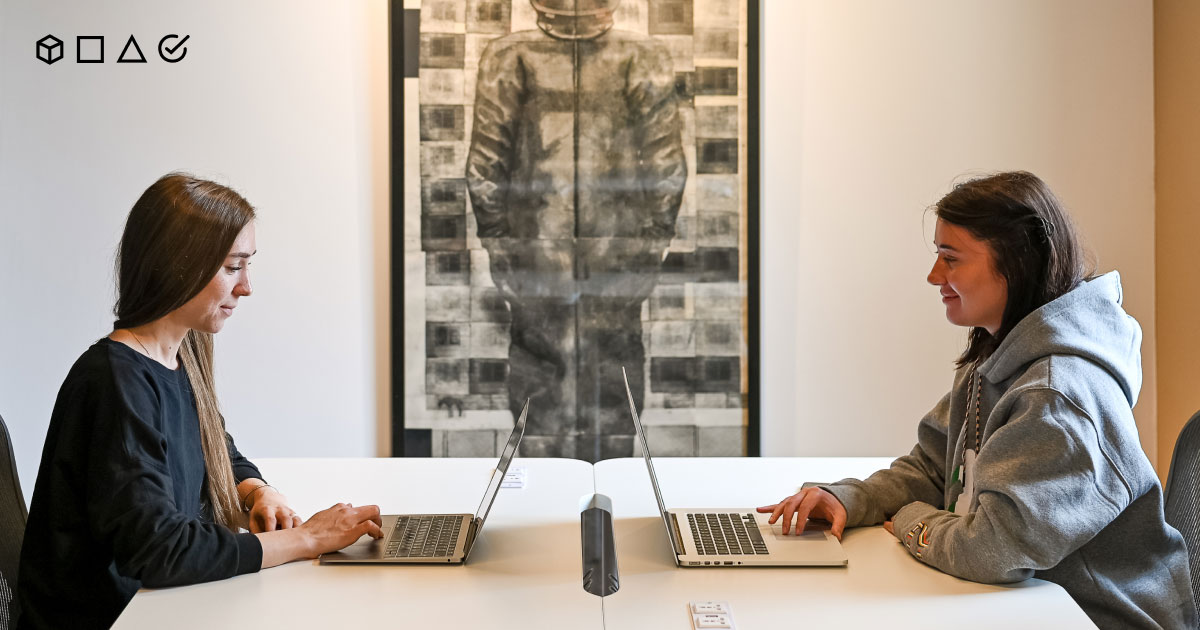
Data labeler is a pretty straightforward job yet it comes in different names. You can see the job title of a data annotator, a data (or label) associate, a data specialist, a machine learning labeler, and so on. You should not confuse this job with a data scientist or an AI engineer since those positions require more understanding of designing and building of an ML algorithm and usually have a big chunk of work dedicated to development.
The skills required for a data labeler include:
- Attention to fine details. Annotating a bit more or a bit less of the object, missing some part of it or incorrect tagging will not impact the whole algorithm much. But make enough of those little mistakes, and the correct training of the model may be jeopardized.
- Focus for long periods of time. Labeling requires perseverance. A data labeler should be able to sit down and pay attention to what's happening on the screen, without being easily distracted and making mistakes as a result.
- Tech-oriented mind. Data annotation requires working with computers rather than people. It may be hard for some people while a great career choice for others. As a trade-off, a data labeler can work online and part-time, which is a perfect option for a freelancer who doesn't possess enough skills or wants to change the type of job they've been doing.
The data labeler will need to show accuracy, dedication, and consistency of results in order to be successful in this line of work.
As we've mentioned before, the jobs for a data labeler usually do not require a lot of training outside of the highly-specialized industries like healthcare. Still, aside from the skillset discussed above, there are subtle differences in the annotation jobs that depend on the field of AI and the type of annotation required for each specific project.
Computer Vision
Machines process data in a different way than humans. In order to "see" how people see, a data labeler must be able to clearly indicate the outline and/or the position of the object of interest on an image (or a video). Accurate outputs for data labeling allows training the ML models faster and better. Too much or too little of the object, inaccurate framing or boxing, skewed key points, and the results will be subpar. For an annotation task in computer vision, a data labeler will have to be attentive to visual details. The ability to draw is not obligatory but will be a plus.
Natural Language Processing
NLP deals with text and audio data. Similar to CV, computers don't understand human (natural) languages, and a data labeler should clearly indicate the significant parts of phrases to explain the sentiment of the text to the machine. Audio-to-text transcription requires listening to the recordings and processing the speech into written text. For NLP, subjective cues and perceptual judgments can present a challenge (e.g., things like humor can be hard to interpret and thus require extra attention and potential collaboration with other data labelers).
Additional Jobs
While data labelers usually only work with annotations, there are a few adjacent tasks that can be merged into the job requirements. At Label Your Data, we also offer data collection and model validation, which are highly useful to take the load off the shoulders of AI engineers who design the ML algorithms.
Data collection is the process of assembling a data set that will be used to train the ML model. There are quite a few requirements for the data to meet if you want the model to be properly trained. It should be relevant to your task, high-quality, consistent, complete, devoid of biases, and preferably big. Read more about it in our article on the data labeling challenge.
Model validation is the step necessary to ensure the data collected and annotated will allow the proper training of your algorithm. This step includes the QA testing of the data for accuracy and quality, making sure the classes were assigned correctly, and there are no missing or inappropriate tags that could mess with your model's interpretation of the data. This process ensures that the model will perform as it should when fed with the testing data set.
How to Get Your Data Labeled: Outsource or Keep It In-House?
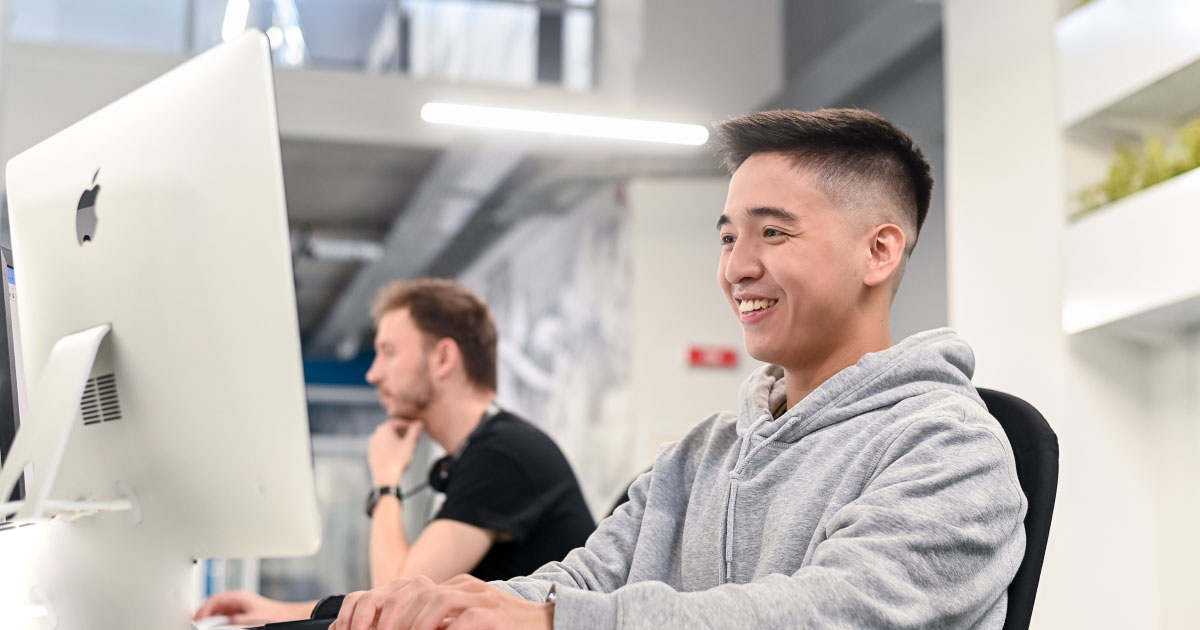
In the highly-competitive circumstances of the modern global market, an organization cannot be great at everything. You need your time focusing on the core business goals that will bring you the most revenue. That's why, for now, outsourcing and crowdsourcing are the most popular options to get the labeling job done.
Naturally, if data annotation is an essential part of your operations, it might be viable to build an in-house team of labelers. However, it is really the case for small and middle-sized businesses and usually is common for large corporations like Google, Amazon, IBM, etc. The reason for this is that an in-house team is expensive in both money and time. It should be justified usually not only by the available resources but also by the goals. Think of it this way: big corporations usually invest not only in the AI projects they develop for a specific practical goal (e.g., a web app) but research in the field of machine learning.
If you want to dive deeper into this topic, read our article on outsourcing vs in-house data labelers that will explain to you the pros, cons, and best options in terms of quality, speed, and price.
Data Annotators at Label Your Data
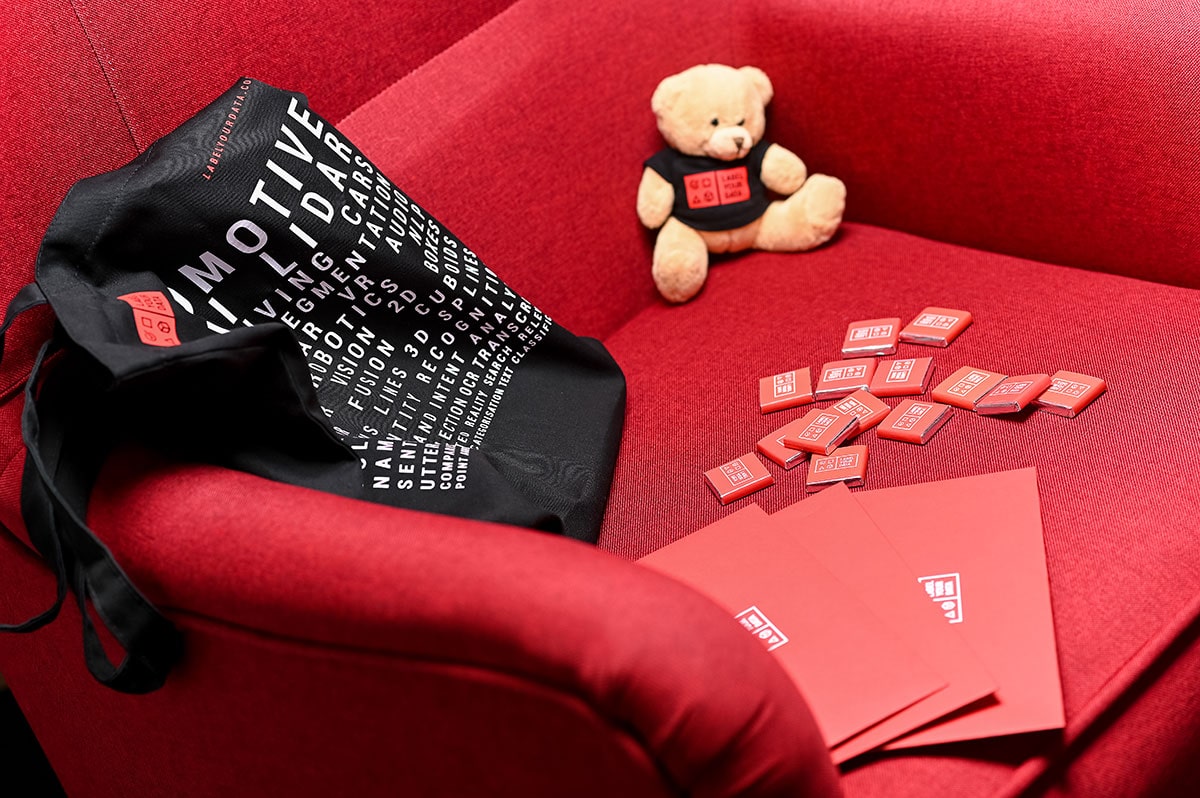
Today, we face the age of transition: from analog to digital, from offline to online, from manual to automated. AI has permeated every industry, and its rapid growth creates the demand for new data-driven jobs.
A data labeler is who knows the ins and outs of annotation as the crucial process of machine learning. They know how to perform the tedious but essential work to let you enjoy the fruits of automation and high-level prediction. We still have a long way to go before computers develop at least a semblance of their own cognition. And before that time comes, data labelers will stay an integral part of every AI project.
At Label Your Data, we know this well. That's why we've paid special attention to hiring qualified specialists and managing both remote and on-location team of data labelers. Our 600+ professionals can do any type of annotation for any project, from standard to the state-of-the-art. Our data labelers work with 31 languages and adhere to the data security standards including GDPR and CCPA. With our data labelers, Label Your Data can guarantee speed, high quality, and security of your annotation. Ask us for a quote now and make sure for yourself!
Written by
Karyna is the CEO of Label Your Data, a company specializing in data labeling solutions for machine learning projects. With a strong background in machine learning, she frequently collaborates with editors to share her expertise through articles, whitepapers, and presentations.