When Definition and Context Matter the Most: Addressing Specific Data Labeling Requests
Table of Contents
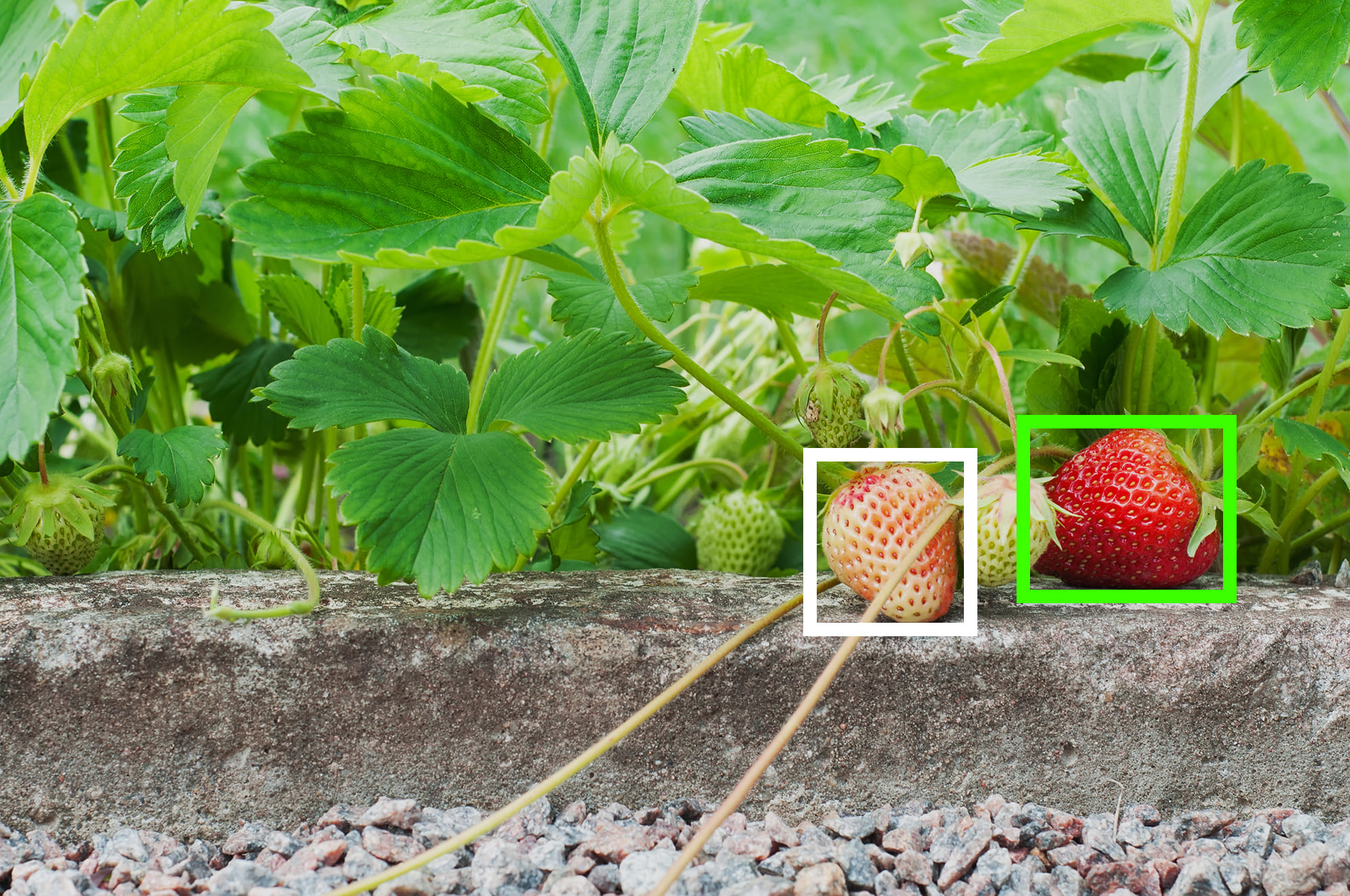
In the past years, we have heard plenty about developments in fields of autonomous driving, hard sciences or text mining using artificial intelligence. It has been all over the news and on the lips of people interested in new technologies around the world. Although fields of artificial intelligence application are widening to include many others, only specialists or niche news sources dig into other areas that may use specific types of AI algorithms and very specific labeled data to make them work. For many companies that provide AI-related or data labeling services, unusual requests make the process quite complicated. Luckily, we had plenty of experience with those! So, in this article, we have decided to give you a synopsis of the most interesting examples of which AI and labeling tools are used in agriculture, insurance business, social media, psychology and games today and tell you how we can help in making your project a success.
Agriculture: having a byte
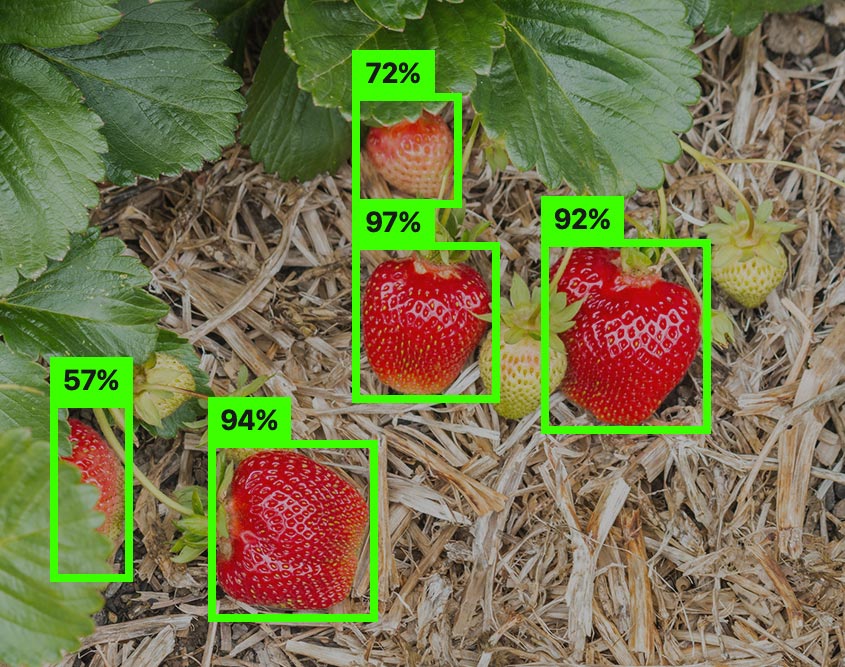
AI and robots redefine the ways farmers grow food. Perhaps, it is not surprising after all that such an essential part of our lives is being automated and enhanced. Very often, robots are used for a labor-intensive task of picking fruit using ripe fruit identification technology. As for the specific algorithms developed to help machines do it, fruits are analyzed based on their stage of readiness. The set of measures may vary based on a specific plant and its characteristics, for instance, the intensity of colors (often, shades from green to primary color), size or softness of harvest. Training sets include comparisons between ripe and unripe produce, that teaches a smart algorithm, which later on gets integrated with the mechanical parts of the robot. As a rule, our clients tend to ask us to mark the objects fully, because even if hidden behind the leaf, developers must make sure the robot doesn't miss a fruit.
Insurance: all about the cache
Among others, insurance businesses have also been expanding their reach and possibilities with artificial intelligence. Apart from cyber insurance, which covers hacker attacks and security breaches, innovative companies start applying AI to real-life insurance claims, such as property, cars or healthcare. At Label Your Data, we have labeled datasets for a company, aiming to fully automate damage severity on machinery for insurance claims. Their algorithm estimated impact and determined repair costs. Though in many cases such algorithms may still need human reassurance or assistance, their usage in the field is rising steadily.
Many researchers and professionals highlight the high risk of using automated insurance disputes, however, this does not seem to stop ML engineers looking for better and better algorithms and solutions in order to modernize the industry.
Social media: staying on the same wavelength
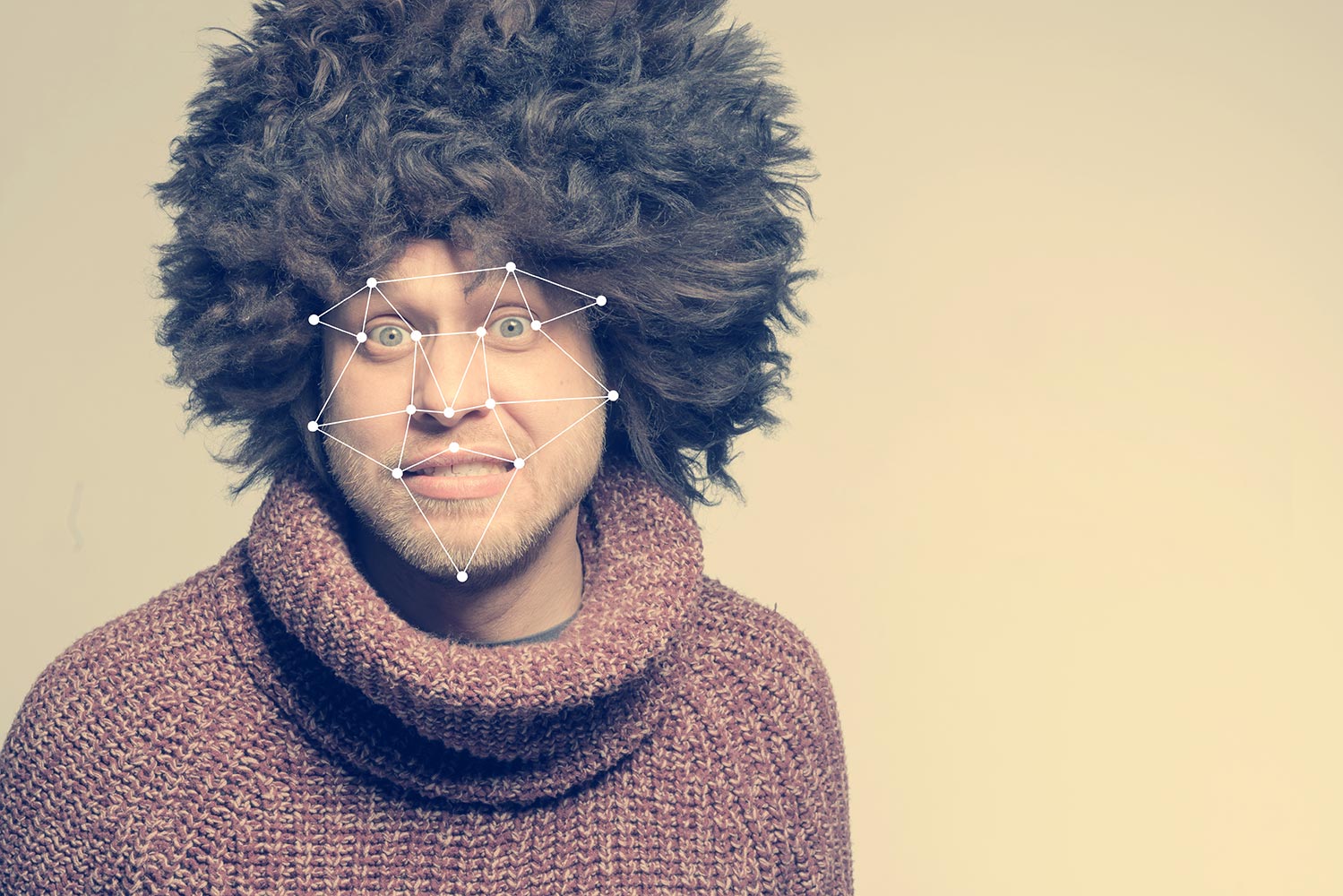
Social media, perhaps, is one of the least unexpected fields of AI usage in our list. To make it more interesting, we have decided to peek into the visual component of social media AI — we are asking a question of how Instagram masks work.
Nearly all of us (at least at some point) have tried quirky faces that make us look like animals, random foods or outright alien creatures from the 70s sci-fi novel. Instagram, among others, has a wide range of AR masks and filters that work based on face mapping. Coordinates (by default, there are 68 of them) describe the face oval, forehead, hair and the placement of brows, eyes, cheekbones, nose and eyes. They produce facial landmarks, which later are transformed into 2D and 3D template masks with an ability to bend and move with the face. Template masks are possible to modify with different filters (such as zoom and multiplication) or objects (for instance, flower crowns or sunglasses).
Psychology: pushing our buttons
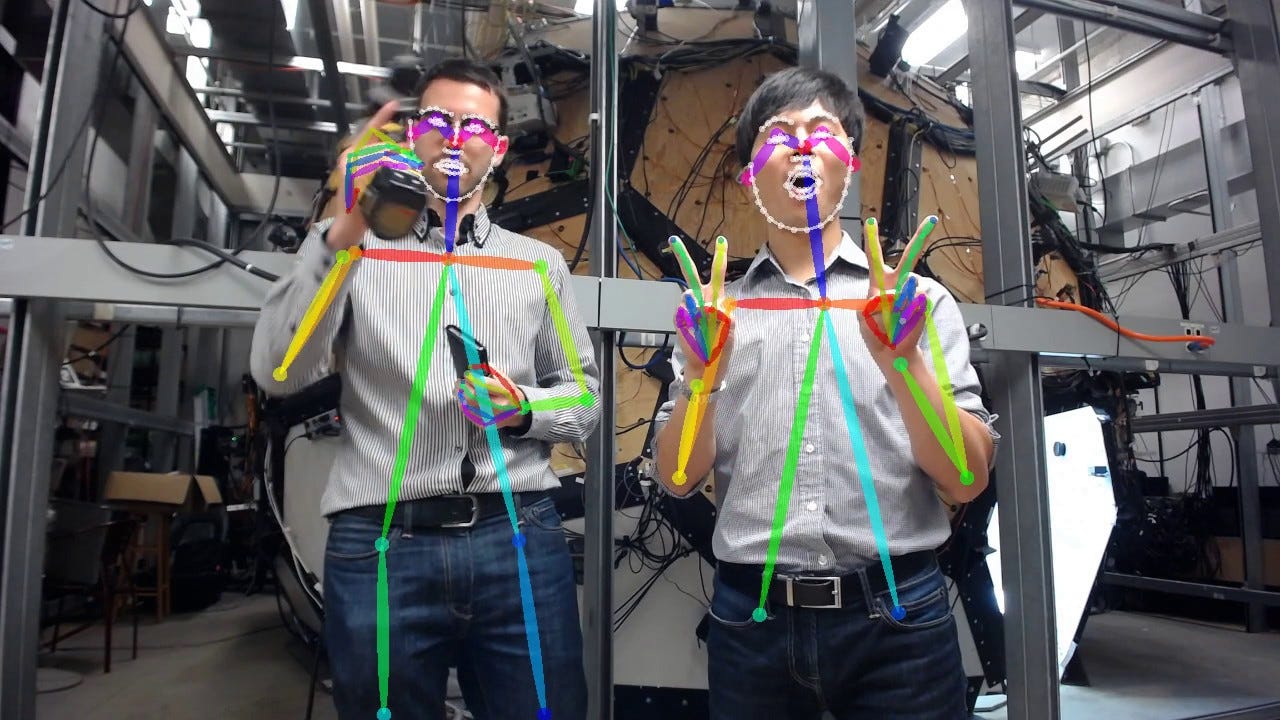
In our previous article, we talked about applications of AI in modifying behaviors and interactions. Here we will expand on that article by describing the kinds of tools applied in the processes of developing and annotating, as well as the overall logic behind human emotion and gesture labeling work.
- Emotions Annotation
- Emotions do not happen instantly, therefore using photos for labeling and detecting them is not sufficient. When working on such a project, AI developers ask us to label image sequences or even full videos of emotion appearing, staying on and fading from a face. Similarly to algorithms of Instagram masks described above, coordinates and face mesh are often used to map predefined labels for emotions. Since understanding emotions is a very subjective process, the same face could be perceived differently. Consequently, a quality assurance process takes place. Each image is checked by several people and the label with the highest recurrence rate gets selected.
- Gestures Labeling
- Ability to perceive motion can be vital for increasing user experience (think sign language) or for using AI in healthcare. Data labeling and programming of gestures is similar to emotions in some ways but very different in others. Like emotions, gestures are continuous movements, so annotating them requires sequences or videos too. Unlike emotions, categorizing and labeling gestures is more complicated. Detection of a gesture consists of three major steps: hand detection, hand tracking and gesture recognition. It is usually accomplished by creating a set of rigging key points and a "skeleton" with predefined labels for each kind of gesture.
Sports: game analysis
It is a common practice for coaches and game analysts to assemble large amounts of data about a certain sport in order to identify the most successful player tactics or even predict future winners. While before these were long processes with a high error rate, nowadays some professionals decide to automate the analytical process. Among all the methods we discussed today, annotating sports data or sports rules is the most complex task for data labelers. Why? It often requires more than one labeling tool and numerous layers of labels. Analogous to emotions and gestures, labeling sports data is often about continuous movements but not only. It also includes full-body key points of players (and referee) with "skeletons" for the detection of pose in an image. In sports that have balls, bats, rackets or other equipment, those are often labeled too, with detection of range, trajectory and velocity of movements.
Outsourcing data annotation of specific objects
Needless to say, data labeling of specific and complex objects or annotation of movements is a complicated process with high error-rate, unless you leave it to professionals. At Label Your Data, we use our long-standing experience in data annotation to help businesses thrive in their big data projects by providing high quality annotation and secure data labeling solutions to all of our clients, even if they come with intricate projects.
- The quality of each dot matters
- Data protection is crucial for AI and ML projects
- Sometimes standard software just isn't flexible enough, so we provide customizable software to fit our clients' needs
- Specific projects need specific sets of instructions, which we can help develop
- Detailed reviews and communication throughout the process are key to success
Written by
A pioneer of the Label Your Data blog, Veronika has helped many of us understand the ins and outs of today's cutting-edge technology and opportunities provided by artificial intelligence. She speaks on the most critical issues of data labeling, machine learning, big data, and more. You should definitely read her other articles to plunge into the world of AI and data annotation!