AI in Emotion Recognition: Does It Work?
Table of Contents
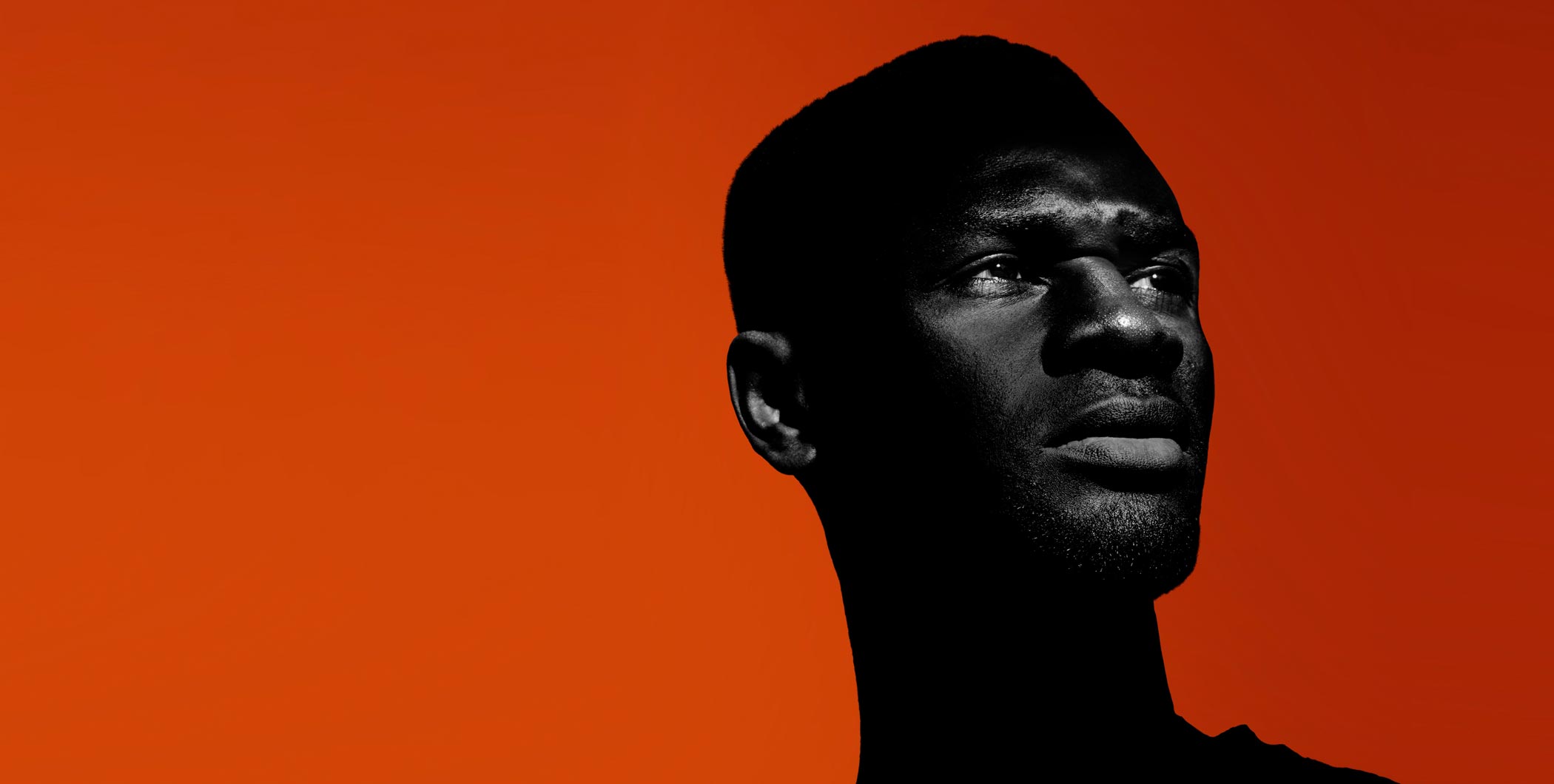
As information technology gains more momentum than ever, the demand for solutions that would help with automation and predictive analytics soars. That’s why AI has become a household name and a source of a competitive edge for any business.
Among the technologies that attract a lot of interest for business owners are facial recognition and emotion recognition algorithms. These two are tightly related as emotion recognition is the natural progression for facial recognition. However, there are problems in this regard that we’ll return to later in the article.
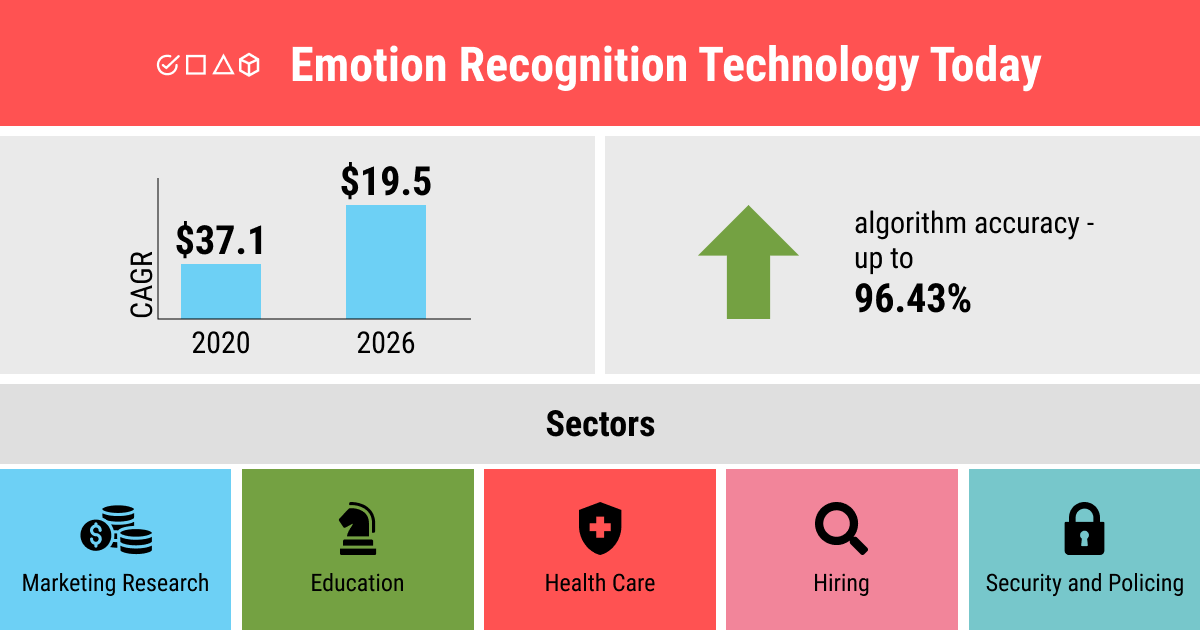
Today, the emotion recognition market is estimated to grow exponentially in the next five years, from $19.5 billion in 2020 to $37.1 billion in 2026. Other sources name an even higher number of $85 billion that could be reached in 2025. These estimations demonstrate the growing business need that exists despite the obvious and hidden flaws of the current emotion recognition technology.
In our new article, we take a look at what emotion recognition is, how it works, how it can be used, and also the problems that surround this technology. Let’s dive in!
What Is Emotion Recognition Artificial Intelligence?
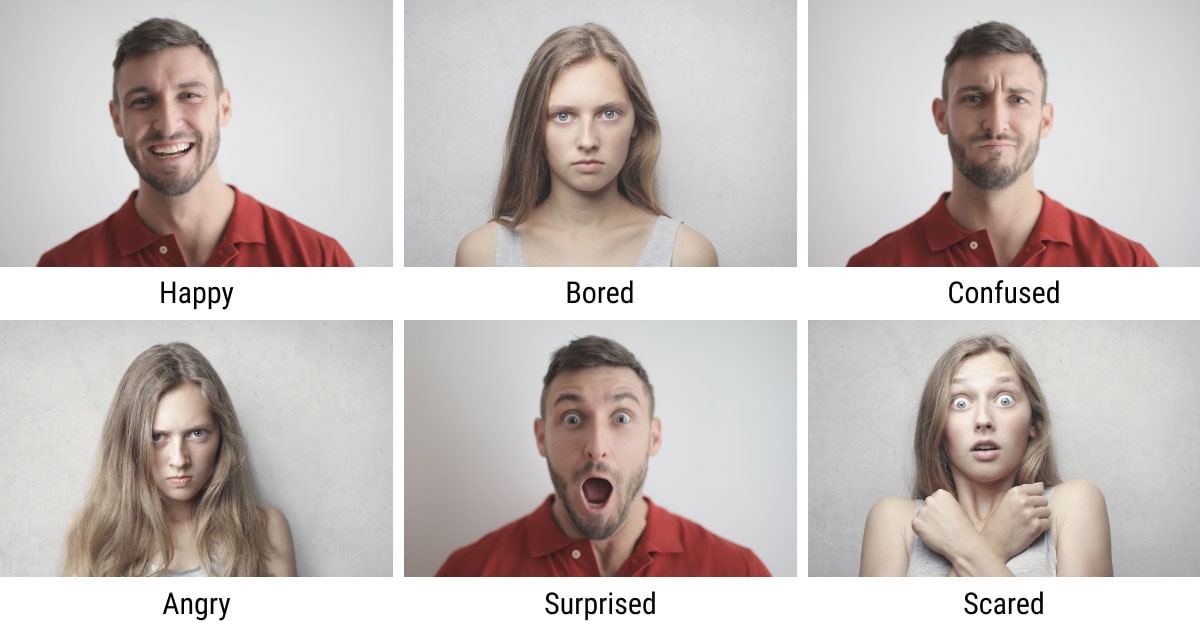
Emotion recognition is the natural progression of facial recognition technology. Currently, emotion detection (or mood detection, as it is also known) is based on the universal emotion theory that has a set of six “basic” emotions: fear, anger, happiness, sadness, disgust, and surprise. This theory was proposed, researched, and defended by Paul Ekman, a well-known American psychologist.
Some algorithms may have a seventh emotion, like Microsoft’s Face API that has contempt added to the algorithm. This traditional approach, however, is sometimes seen as lacking and incomplete by researchers engaged in this area of human psychology.
So basically what emotion recognition algorithms do is predict the emotion of a person based on their facial expression at the moment. This allows estimating the user reaction to certain content, offered products, the engagement in the process, etc. (depending on the area of implementation of the emotion recognition algorithm). Let’s dive a little deeper into the inner workings of such algorithms.
Inside Out: How Do Emotion Recognition AI Algorithms Work?
The process of building an emotion recognition model, like any other AI project, starts with project planning and data collection. You can read more about the stages of an AI project and the collection of datasets in our dedicated articles.
Let’s stop for a while on the data collected for an emotion recognition model. It is an essential (and most laborious) part of the future algorithm as it requires a lot of time and effort to be collected, processed, secured, and annotated. This data is required to train the emotion recognition model, which is basically a process that allows the machine to understand how to interpret the data you show to it.
Naturally, for the proper work of an emotion recognition algorithm, you need to ensure the data you collect is of high quality, devoid of blind spots and biases. When collecting the data, it’s useful to remember the major governing principle for this process: garbage in—garbage out. If you feed low-quality data into your algorithm, you shouldn’t expect any high-quality predictions.
Let’s say you’ve collected 10.000 photos of people in different emotional states. A blind spot would be having no Asian, Middle Eastern, or Latin people in the dataset. A bias would be collecting photos of men only with frowning or smiling faces. Either case would lead to the inability of a computer to understand and predict these corner cases that the algorithm will see in real life.
Then there’s the process of data annotation aka data labeling that helps to translate our vision of the data into a machine-readable format. This is achieved by the addition of meaningful labels to each data piece. What does this mean for an emotion recognition algorithm?
Remember that hypothetical dataset of 10.000 photos you’ve collected for the previous step? Now it’s time to label each of the photos for training (and reserving some part of it for testing and validation, naturally). Usually, a keypoint (or landmark) annotation is used by placing key points on the faces of the people and then adding tags like “happy”, “angry”, “sad”, “surprised”, etc.
Can you spot the problem already? The labelers who annotate the photos usually don’t have the context for the facial expressions of people on these photos. Which means that the annotation tags may be incorrect. On the other hand, the human annotators aren’t devoid of natural bias and may more commonly see certain races, genders, or ethnicities as more hostile, less scared, inherently joyful, etc. That’s why it’s still nearly impossible to get rid of the data noise in the system, which means the emotion detection algorithms will keep reproducing human errors and bias.
How to Use AI for Facial and Emotion Recognition: The Problems and Risks of Mood and Emotion Detection AI
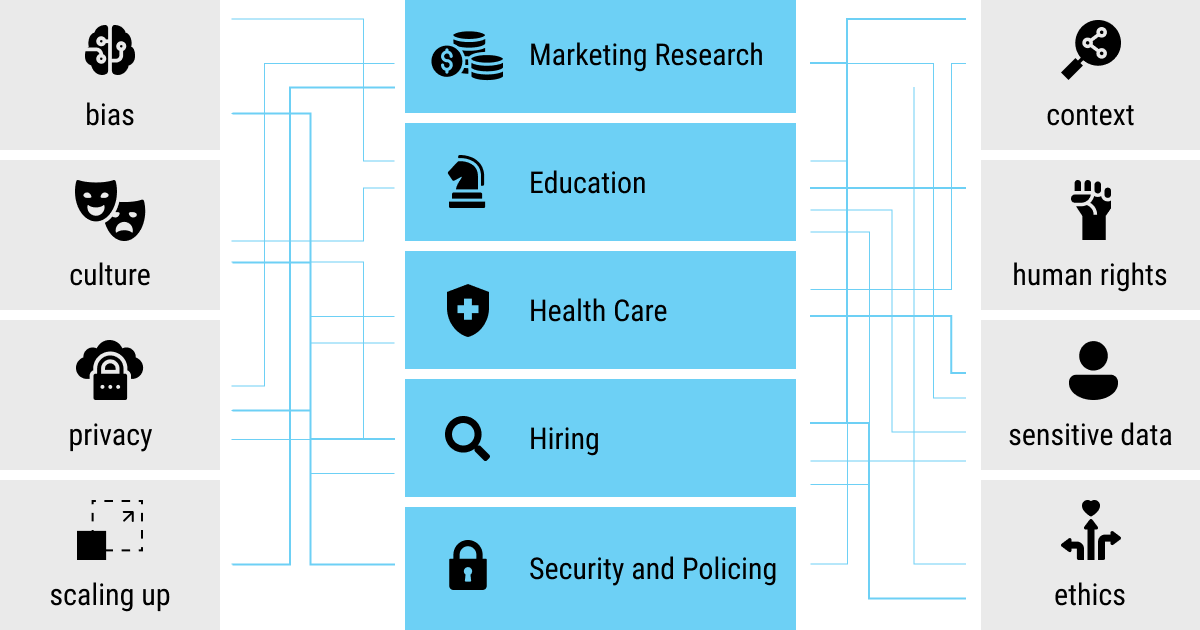
Certain sources claim that the accuracy of emotion recognition models based on facial expressions reaches 96.43%. So what seems to be the problem? There are a few, actually, from the scientific background to biases in data collection and annotation to privacy issues.
So if these problems exist and we know about them, why does emotion recognition technology is in such high demand? No technology is inherently bad or evil, it depends on how it is being used. And there are quite a few industries that are already benefiting from implementing emotion recognition software.
Here’s a rundown of the most interesting ones (coupled with a few problems that deem the application of emotion recognition software untrustworthy at the moment):
Health Care: Biased Decision-Making in Highly Sensitive Areas
Since life and health became the core values in most cultures of the modern world, the HealthTech industry started growing rapidly. Today, emotion recognition offers a lot of opportunities for such complicated areas as psychology, neuroscience, and even biomedical engineering.
Such software if executed correctly can have a great impact on automating the diagnosing of multiple disorders (specifically brain and psychological ones). This should help facilitate the doctors’ work and prioritize patients with severe conditions that require immediate attention.
On the other hand, given the difficulties in annotation and security connected to the sensitive nature of health-related data, the number of errors and biases in emotion recognition software built for HealthTech may be staggering. To avoid such errors, the quality of both data and emotion recognition model design should be on the highest possible level.
Education: What About Human Rights?
As the COVID-19 pandemic put everyone into self-isolation mode and students started attending school from the comfort of their private homes, a demand for a tool to enforce the educators’ job appeared. Emotion recognition gained popularity in the form of software to help teachers observe student reactions and facilitate the learning process.
Today, such software exists in the form of a program monitoring how interested and engaged the students are. However, many believe that the objects such silent observation are often unaware they are being seen. This borders on the violation of human rights and indicates the obvious misuse of emotion recognition technology.
Hiring (HR): Contextual and Cultural Considerations vs. Automation
Automated job interview software has become a notorious case for the utilization of emotion recognition software in the past few years. On the outside, it looks like an automation initiative to help businesses find the most suitable and interested candidates for the job.
On the other hand, however, such software is used to judge a candidate’s dependability, cognitive abilities, engagement, conscientiousness, and emotional intelligence. All of these concepts are much more complicated than a basic emotion (or even a combination of such) can translate. They require the analysis of context and fixing judgment errors that only a human being can perform during a live conversation based on feedback from the person they talk to.
Currently, that’s not how emotion recognition works at all. This is known as emojification, which is the predictions made solely on facial expressions without regard to context or conditioning. Naturally, the experts are worried that current emotion recognition models are insufficiently reliant on scientific data. According to Andrew McStay from Bangor University, Wales, who was researching AI and emotion recognition models, “emotions are a social label applied to physiological states”. Yet It should be the other way around: the models shouldn’t be based on a theory that fits what AI can do but AI should adapt to the scientifically validated emotion theory.
Besides, there are cultural and regional nuances that emotion detection models cannot detect if they’re built on the theory of universal emotions. For this reason, a lot of companies that offer such software start to develop regional solutions that take into account the intricacies and cultural predispositions of candidates.
Surveillance and Security: The Case for Bias
Surveillance systems that enable the automation of policing and border control represent yet another problem of the emotion recognition algorithms. How to identify dangerous people? Even more fundamentally, how to define a dangerous person? Is facial recognition a sufficient basis for such activity?
Facial recognition is the most widely adopted surveillance technology worldwide. The most surveilled cities include London, UK, as well as Taiyuan and Wuxi in China. In the US, however, the trend moves away from facial and emotion recognition tech being adopted for policing. Some US cities (San Francisco, CA, Boston, MA, Springfield, MA, Portland, OR, and Portland, ME) banned the police from using such software altogether.
And for a good reason, too. Spotting a wanted person on the street can be efficient for capturing and apprehending a dangerous criminal. However, adding emotion recognition may be very biased for many cultural, ethnic, and religious minorities.
Marketing Research: Is Scaling Up a Solution or a Problem?
Last but not least, marketing research and behavioral predictions at scale represent the interest of most retail businesses in emotion recognition software. Setting aside the problem of making biased decisions in highly sensitive areas that we discussed above (education, hiring, policing, and border control), retail offers another disputable case when using emotion recognition. Scaling up business decisions based on behavioral predictions is definitely the result that many companies hope to get from an emotion recognition algorithm. However, is it a solution or a problem?
Here’s an ethical perspective to explain this argument: should machines be allowed to decide what humans feel? Should they be able to control what content is shown to people or how the businesses should react to these predicted emotions?
The question of permissions was recently raised in EU regulations, where it was proposed to limit the effect that AI can have on a decision-making process if it comes close to human rights. And it seems this perspective will soon be shared by many countries as it puts a customer and user first, making an AI algorithm a servant rather than a decision-maker.
What’s the Fuss About: Solutions for Emotion Recognition and Mood Detection Software
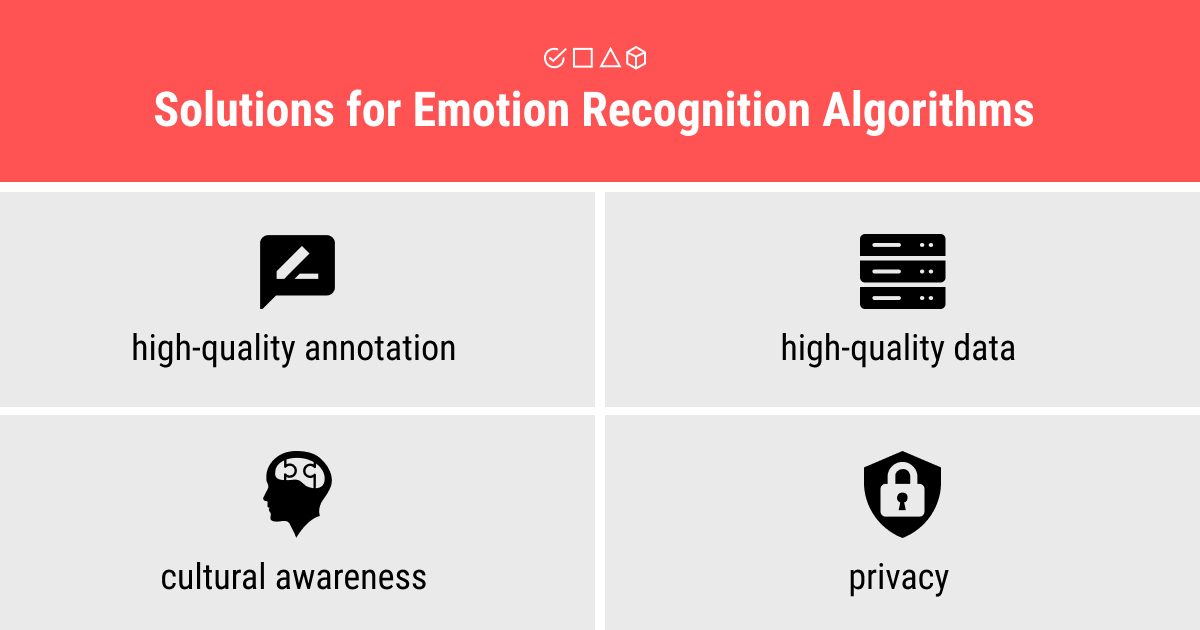
So is emotion recognition any good? Well, yes and no—it depends, really. While emotion detection models definitely lack the polish and scientific basis, it doesn’t mean the technology is bad or cannot be improved. Quite the contrary, actually: as the market demands for emotion recognition grow, these models will become better, more sophisticated, and better suited for real-life scenarios.
Let’s break down a few most significant solutions for the current problems of emotion recognition AI:
- Better data. As the era of big data arrives, it becomes easier to get your hands on better datasets. The better data you collect, the more and cleaner it gets, the better will be the prediction results of your emotion recognition algorithm. The few things you should keep in mind are the relevance, completeness, cleanliness, and security of the data.
- High-quality annotation. The labeling process might seem like a simple process at first but get overwhelming as you scale up. That’s why it’s best to find a trustworthy annotation partner who will ensure your data will be properly labeled and you’ll get a high-quality emotion recognition training set.
- Cultural awareness. Country (or region) specific algorithms and training are already blatantly obvious for many businesses today—not only as a necessity dictated by the public but as a business-savvy decision. It’s only wise to develop culturally determined solutions to avoid losing customers because of inaccurate predictions.
- Privacy regulations. Restrictions to reading, measurement, and interpretation of facial expressions (especially without explicit consent) should be essential for any business. As the world becomes more digitized, it is appropriate to have more restrictions for emotion recognition algorithms to protect the human rights to privacy.
Summary: Step Up Your AI Game with Emotion Recognition
Emotion recognition is one of those technologies that has captured the attention of everyone. From its vast application opportunities in many areas to the controversial effects and problems, it presents an interesting case to study and discuss.
Currently, as the demand for automation and predictive analytics at scale is unprecedentedly high, emotion recognition models can be extremely useful for a variety of industries. In health care, it can help with diagnosing brain and psychological conditions. In education, help teachers make the lessons more engaging for students. In hiring, help HRs find the most suitable candidates. The surveillance possibilities are nearly limitless although this also poses corresponding risks.
For retail businesses, emotion recognition is the treasure trove of market research. If you seek a scaling-up opportunity based on behavioral predictions, there’s probably no better technology than mood and emotion detection AI.
However, it’s worth remembering that there are unresolved problems and risks connected to emotion recognition. The ideological foundation of this technology is shaky at best while the privacy issues made a few major cities in the US limit its use for the police. Yet there’s no need to be disheartened by these shortcomings. Emotion recognition is still rather new and it will become stronger, more accurate, and more protected. With the high-quality data and annotation, proper cultural awareness, and privacy regulations in place, emotion recognition algorithms may prove to be among the most beneficial technologies of our age.
Written by
Iryna is one of the dedicated members of the Label Your Data content team who has put all her efforts in developing our knowledge base. Iryna is a seasoned technical writer with wide-ranging experience in artificial intelligence, machine learning, and deep learning. She has been studying the basics of data annotation for many years and is now sharing her expertise on our blog. The technical realm is a true passion of hers, so make sure to check out other articles written by our talented Iryna!