- Key Roles and Skills of Your Data Annotation Team
- How to Hire Data Annotators
- How to Train Data Annotators
While the amount of data continues to explode, finding and retaining skilled annotators can be a challenge. In addition, high turnover due to repetitive tasks can slow progress.
This article dives into the best practices for hiring and training data annotators, ensuring you build a strong team to effectively support your ML projects.
Key Roles and Skills of Your Data Annotation Team
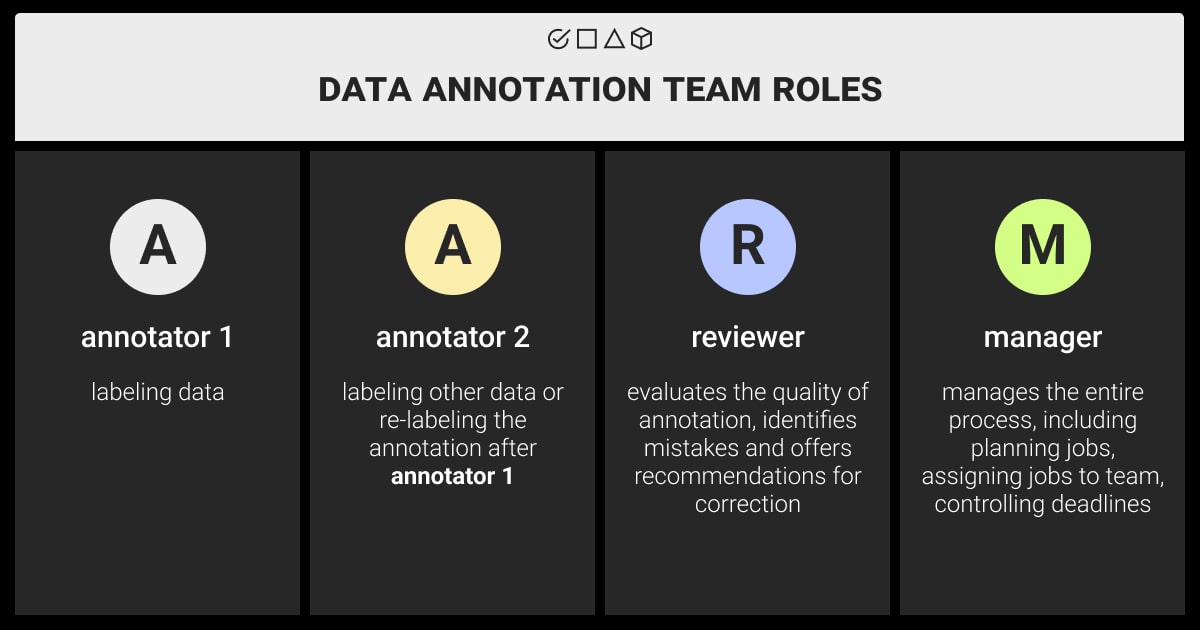
A data annotation team consists of:
Data annotation specialists who prepare high-quality training data for ML models. Their work directly impacts the success of ML projects by guaranteeing the integrity and effectiveness of training data.
Project manager, or a data annotator team manager, who coordinates the annotation process, allocates tasks, and manages timelines to ensure project completion.
QA (Quality Assurance) specialists who verify the accuracy and consistency of annotations, ensuring high-quality labeled datasets.
Subject-matter experts that provide domain-specific knowledge to guide annotators in accurately labeling data relevant to the project.
Data scientists or ML engineers can sometimes be on the team to oversee the annotation process from a technical perspective, ensuring alignment with the requirements of the ML models being developed.
When it comes to the core team members, annotators, their role extends beyond mere data annotation:
Data annotator responsibility | Explanation |
Working with data |
Analyzing data for key features and attributes to guide how it’s labeled. |
Creating labeling rules |
Establishing clear and consistent instructions for data annotators to follow. |
Applying labels to data |
Accurately assigning predefined tags to data to make it machine-readable. |
Checking the quality |
Reviewing and verifying the accuracy and consistency of performed annotations. |
Teaming up with diverse experts |
Collaborating with other team members involved in the project, such as data scientists and engineers. |
Securing the data |
Following strict security protocols to ensure data privacy during annotation. |
Skip the labeling hassle. Run your free pilot with Label Your Data
How to Hire Data Annotators
The repetitive nature of data labeling takes a mental toll. It demands focus and precision, but can lead to burnout and high turnover. This constant churn disrupts project timelines and increases training costs. Furthermore, it impacts the performance and consistency of dedicated annotators, ultimately driving up overall expenses for businesses.
To address these challenges and ensure high-quality data, effective hiring strategies are pivotal. By identifying candidates with the right skills, temperament, and training them effectively, companies can create a more engaged and resilient annotation workforce. This, in turn, leads to lower turnover rates, improved data quality, and reduced project costs.
1. How to Write Job Descriptions for Hiring Data Annotators
Crafting a compelling job description is the first step to attracting qualified data annotators. Here’s how to structure your description for maximum impact:
Grab attention with a catchy opening sentence. Highlight the importance of the role and what the successful candidate will achieve.
Clearly outline the responsibilities. What are the day-to-day tasks of a data annotator in your company? Be specific about the data they will be working with and the tools they will be using.
List essential skills and experience required. Not all data annotation projects require the same skill set. Tailor this section to the specific needs of your project. For example, some projects may require experience with specific labeling tools like CVAT, while others may require Excel knowledge.
Highlight the benefits you offer. Competitive salary and benefits are important, but don’t forget to showcase what makes your company unique. This could include opportunities for growth, project variety, flexible employment types, or a positive work environment like we provide at Label Your Data.
“The most critical point for annotator job description is working on your EVP. It’s an Employee value proposition (EVP) that serves as a magnet for high performers. Write a concise message that tells potential hires exactly what it’s like to work at your company. By highlighting what sets you apart, you’ll attract, engage, and retain the best talent.”
Liudmyla Boichun
HR Director at Label Your Data
In addition, focus on attracting candidates with the following key qualities:
Attention to detail. Even small mistakes can have a big impact on the quality of your ML dataset.
Ability to handle large data volumes. Data labeling often involves processing large datasets. Make sure your candidates can work quickly and accurately without getting overwhelmed.
Willingness to work with monotonous work. Data annotation can involve some repetitive and mundane tasks. While you want candidates who can be meticulous, you also want those who can stay motivated and engaged over time.
Analytical mind. The ability to identify and address inconsistencies or ambiguities in the data is an important skill for a data annotator.
By writing a clear and compelling job description that highlights the key qualities you are looking for, you can attract top data annotator talent.
2. Where to Publish Job Vacancies
Once you have a great job description written, it’s time to get it in front of the right candidates.
Target job postings based on location. For a broader international reach, consider using an Applicant Tracking System (ATS) to post your jobs on platforms like Jooble, Startup Jobs, and LinkedIn. Be sure to tailor your postings to the specific countries you are targeting by highlighting relevant skills and experience.
“Grow your team through a referral program. Your existing data annotators can be a valuable source of new talent. Encourage them to recommend friends and colleagues who might be a good fit for your company. This is a cost-effective way to find qualified candidates who already have some understanding of the role and company culture.”
Liudmyla Boichun
HR Director at Label Your Data
By using a combination of online platforms and a referral program, you can increase your chances of finding the best annotators for your team.
3. How to Interview Data Annotators
The interview process is your chance to assess a candidate’s skills and suitability for your ML project. Here’s a breakdown of the key interview stages to consider:
Start with a brief introduction explaining the interview format. Briefly outline the topics that will be covered and allow the candidate to ask any questions they may have.
Discuss the candidate’s experience. Even if the candidate’s experience is not directly related to data annotation, this can still help you understand their work ethic, transferable skills, and ability to learn new things.
Gauge their understanding of data annotation. Ask questions to assess their knowledge of common data annotation tasks and tools to see if they did any research to study the topic.
Ensure a company culture fit. Discussing your values and work environment to give the candidate a chance to learn about your company culture and see if it aligns with their own values and work style.
Watch out for red flags. This can be anything from negativity towards past employers to odd questions that could signal potential issues.
Use a presentation to showcase your company. Highlight growth, projects, values, and culture in your presentation. This is a chance to impress the candidate and give them a reason to want to work for you. Encourage questions. Let them see the exciting work you’re doing and the positive environment they could be a part of.
Provide a project-specific test task to assess skills. This will give you a firsthand look at the candidate’s ability to perform the actual tasks of the job. See how they handle the data, use the tools, and approach any challenges that arise.
Conducting a well-structured interview will help you gain valuable insights into a candidate’s qualifications and suitability for the data annotator role.
4. How to Choose the Best Data Annotators
Once the interviews are complete, carefully evaluate each candidate and select the best fit for your team. Here are some key strategies:
Evaluate each candidate after the interview. Take time to complete written evaluations that assess their strengths, weaknesses, and overall impression on the team. Consider factors like their technical skills, attention to detail, problem-solving abilities, and cultural fit.
Use Google forms to assess their workplace behavior. Analyze responses from a Google form used during the interview process. This form can provide valuable insights into the candidate’s work style, comfort level with communication, and adaptability. For example, how do they handle hypothetical work scenarios or questions about teamwork?
-
Look for candidates who demonstrate the following:
Strong and timely performance on the test task. This is a clear indicator of their ability to handle the specific demands of the data annotation role.
A genuine interest in data annotation and AI. Passionate candidates are more likely to be engaged and motivated in their work.
The ability to ask thoughtful questions. This shows their curiosity and desire to learn more about the role and your company.
By carefully evaluating each candidate against these criteria, you can identify the top performers.
5. How to Retain Data Annotators
In today’s competitive job market, retaining top talent is crucial. Here are some strategies to keep your data annotators happy and engaged:
Focus on positive employee experiences. Invest in creating a positive work environment that fosters employee well-being and satisfaction. At Label Your Data, we’ve created a dedicated People Experience Team that specifically helps annotators with onboarding, communication, and addressing concerns.
Conduct regular check-ins with annotators throughout their employment. Schedule regular meetings to discuss their work, address any concerns they may have, and provide valuable feedback. This ongoing communication helps them feel valued and supported.
Offer clear career paths within the company. Make sure to provide opportunities for career growth and advancement, such as promotions from Project Annotator (part-time employment) or Dedicated Annotator (full-time employment) to Project Supervisor or even an Account Manager.
Provide flexible work arrangements for a work-life balance. Offering flexible hours, remote work options, or compressed workweeks can help your data annotators achieve a healthy balance between their work and personal lives.
“Offer diversity to keep things interesting. Data annotation can involve repetitive tasks. To maintain annotator engagement and mitigate repetitive work, propose a variety of data types for labeling. Additionally, consider offering part-time arrangements or rotating tasks within projects to prevent annotators’ burnout and ensure long-term focus on high-quality annotations.”
Ivan Lebediev,
Integration Specialist at Label Your Data
This way, you can create a work environment that fosters loyalty and reduces employee turnover, allowing you to retain your top annotation specialists.
6. How to Use the Referral Program
Don’t underestimate the power of your existing data annotators. A referral program can be a goldmine for attracting top talent.
Here’s why:
Proven results: Referral programs accounted for 25-40% of our main candidate traffic within two years at Label Your Data. That’s a significant chunk of qualified applicants coming through the company.
Quality referrals: Candidates referred by current employees already have a certain understanding of the job, expectations, and company culture. They’ve likely heard positive feedback from their friends, which translates to motivated individuals who are ready to hit the ground running.
Cost-effective: Compared to traditional recruitment methods, referral programs are a budget-friendly way to find qualified candidates. You’re leveraging your existing employee network, reducing advertising and agency fees.
There are two main ways to structure your program:
Internal referrals: Encourage employees to recommend friends or colleagues who might be a good fit.
External referrals: Open your program to the broader network, allowing anyone to refer qualified candidates.
No matter which approach you choose, make sure to offer attractive incentives to encourage participation. This could be a cash bonus, additional paid time off, or other perks.
Additional Hiring Tips
Leverage your network: Utilize job boards like Indeed, Glassdoor, and LinkedIn, targeting experienced professionals. Engage with relevant AI communities on social media. Encourage employee referrals for qualified candidates.
Look beyond your network: Consider partnering with third-party annotation companies for leveraging their existing pool of pre-trained professionals. Create targeted online ads to attract qualified individuals, focusing on relevant keywords, such as “data labeling expert” or “AI project annotator.”
Prioritize quality over cost: Assess the annotators’ skills, experience, and portfolio to ensure they meet your ML project’s needs. Remember, good data annotation is an investment in your AI’s accuracy.
-
Choose the right hiring approach:
In-house: Offers control and consistency but can be expensive.
Freelancers: Cost-effective with access to specific skills, but managing quality can be challenging.
Outsourcing: Turnkey solution with minimal management, but expensive and less flexible.
Crowdsourcing: Highly cost-effective, but ensuring consistent quality is difficult.
How to Train Data Annotators
Effective data labeling requires a well-trained team. Taking these steps, you are more likely to build one:
-
Define your data annotation process clearly
Document guidelines: Establish clear instructions for labeling conventions, training procedures, and quality control measures. Make them readily accessible and regularly updated to ensure everyone is on the same page.
Training procedures: Streamline onboarding for new members and ensure existing members stay aligned. Encourage real-time questions and provide written feedback during training.
“A clearly defined process ensures clarity, consistency, and enhanced efficiency. First, it fosters a collaborative environment where each of the annotation team members understands roles, expectations, and quality standards. Second, clear procedures minimize confusion and wasted time, leading to faster completion and consistent high-quality data.”
Ivan Lebediev,
Integration Specialist at Label Your Data
-
Establish effective training procedures
An effective training procedure is crucial for building a data annotation team. Here’s how to achieve one:
Clear communication: Establish clear and consistent guidelines to avoid confusion and maintain data annotation quality.
Onboarding and ongoing support: Defined procedures ensure efficient training for new members and continued reference for experienced members.
Consistency in labeling: Consistent application of annotation standards across the team is crucial for reliable data applicable for machine learning models.
The success of training annotators for a project hinges on several factors, including the project’s specific needs, time constraints, and the capabilities of the managing team. When planning your project timeline, consider the duration of the training phrase. It depends on the experience level of the workforce, the project’s complexity, and the chosen method for ensuring data quality.
-
Additional considerations for a data annotation team building
Beyond the core training, consider these additional factors:
Tagging ontology: Design for consistency by considering potential edge cases and using contrasting examples to clarify labeling.
User experience: Design task guidelines with ergonomics and collaboration in mind.
Language and culture: Consider variations when setting up tag sets and data collection guidelines.
Team diversity: Create a data annotation team that is diverse, with relevant language skills, and different backgrounds to reduce bias in data models and ensure fair outcomes.
Performance monitoring: Implement a plan to address consistently low performers to maintain data quality.
User-friendly tools: Choose intuitive and user-friendly data annotation tools for efficient data processing and higher-quality results.
By following these steps, you can build a strong annotation team that delivers consistent, high-quality training data. But it’s crucial to have a system in place for identifying and addressing consistently underperforming annotators. This could involve either providing additional training for annotators or, if necessary, removing them from the project to safeguard the quality of the ML datasets.
The Role of Subject-Matter Specialists
Subject-matter experts (SMEs) play a crucial role in building a data annotation team for several reasons:
Domain-specific knowledge: SMEs possess deep understanding and expertise in the specific field or domain the data pertains to. This allows them to accurately interpret, categorize, and label data points with the necessary context and nuance.
Quality assurance: Their expertise enables them to identify inconsistencies, ambiguities, and potential errors in data annotation.
Developing guidelines and standards: SMEs are instrumental in establishing clear and consistent annotation guidelines and standards for the team to follow. This minimizes discrepancies in how different annotators perceive and annotate the data.
“SMEs can effectively train and mentor other annotators, especially those new to the domain. They can provide valuable insights into the specific characteristics and nuances of the data, leading to a more skilled and efficient annotation team.”
Viktoriia Yarmolchuk
Account Manager at Label Your Data
While not always feasible to have a team solely of SMEs, their involvement is vital for ensuring high-quality, reliable data annotation.
The Benefits of Building an In-House Data Annotation Team
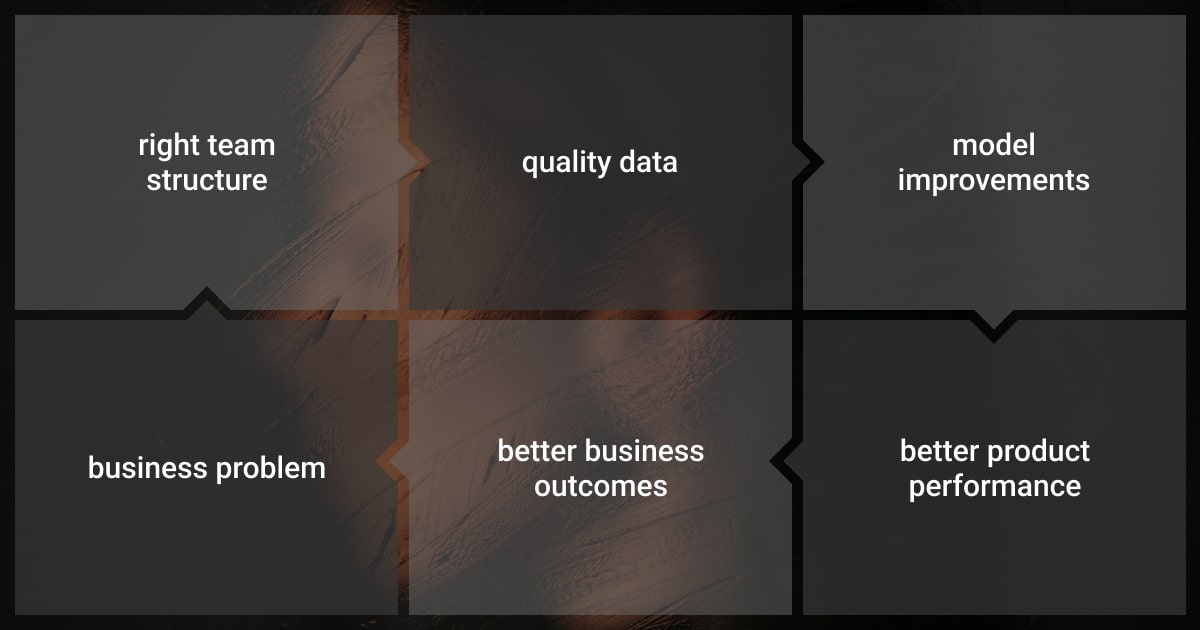
Having a dedicated data annotation team offers several advantages over crowdsourcing or other options. While both approaches involve a large pool of workers, relying on anonymous individuals can lead to inconsistent and lower-quality data compared to a managed team working with the same data.
One of the key benefits of building a data annotation team is annotators’ increasing familiarity with your project. That is, the expertise of the data annotation team in your specific corporate rules, context, and edge cases grows in parallel with their practice. This understanding allows them to ensure high-quality results and efficiently train new members who join the team. Not to mention the fact that in-house teams offer enhanced data security thanks to the implementation of non-disclosure agreements (NDA).
Moreover, a managed annotation team is particularly crucial for ML projects, where data quality and the ability to adapt are critical. Unlike crowdsourced workers, in-house data annotation team members gain a deeper understanding of your model and its context with continued exposure to your data. This ongoing collaboration fosters smoother workflows and ultimately leads to superior training data for your project.
Key Takeaways
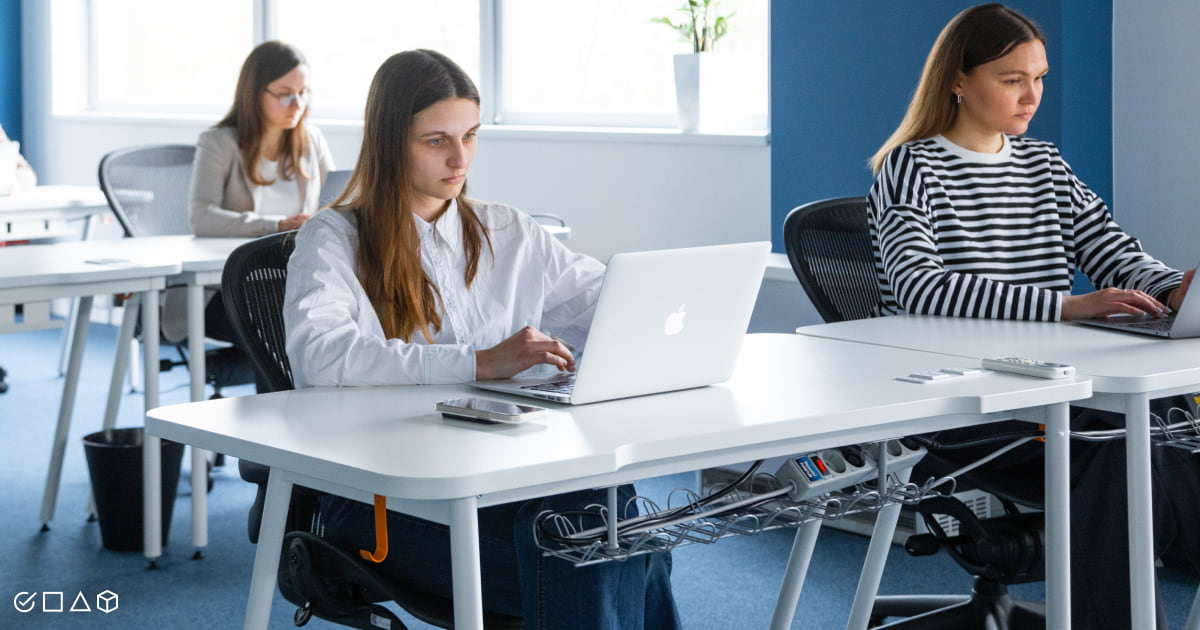
To get high-performing ML models, you need to build a data annotation team capable of handling different project complexity and types of data. While it may seem challenging at first, accurate and well-labeled data translates to efficient and accurate models, ultimately saving you time and resources in the long run.
To reap the benefits of building a data annotation team in-house, be sure to follow the steps we’ve outlined in this guide.
You can always skip the team building process and get reliable data annotation delivered by experts. Contact us here!
FAQ
What is the role of a data annotation job?
The role of a data annotator is to accurately label data to train machine learning models and improve their performance. This involves tasks such as categorizing images, transcribing audio, or tagging text to create labeled datasets used for training ML algorithms across various applications such as computer vision and natural language processing (NLP).
Is data annotation an IT job?
The data annotator job can be considered an IT-adjacent task, as it involves labeling and processing data for ML applications, often performed by IT professionals or specialists within data science teams.
Where should I start with building a data annotation team for my ML project?
When assembling a data annotation team for an ML project, consider these key primary steps:
Identify necessary skill sets, such as data labeling expertise and domain knowledge.
Recruit team members with strong attention to detail and ability to work with large datasets.
Establish clear guidelines and processes for annotation tasks to ensure consistency and accuracy.
Table of Contents
Get Notified ⤵
Receive weekly email each time we publish something new: