We rarely think about AI that we carry around with us in our pockets. Despite being truly ubiquitous and increasingly influential, the concept of machine learning has not reached the common public vocabulary yet. However, companies worldwide acknowledge the value that ML might bring to any business, from financial services to healthcare and from manufacturing to commerce.
According to a report by Statista, the global machine learning market is steadily growing, along with greater data access, improved computer power, and industries demanding increased automation and optimization. The larger market of AI will reach nearly $100 billion by 2025. As for ML, Grand View Research names an impressive machine learning market growth reaching the worth of $419.94 billion by 2030, with a CAGR of 43.5%. Its most rapidly growing subset, the deep learning market, will reach $179.96 billion by the same year.
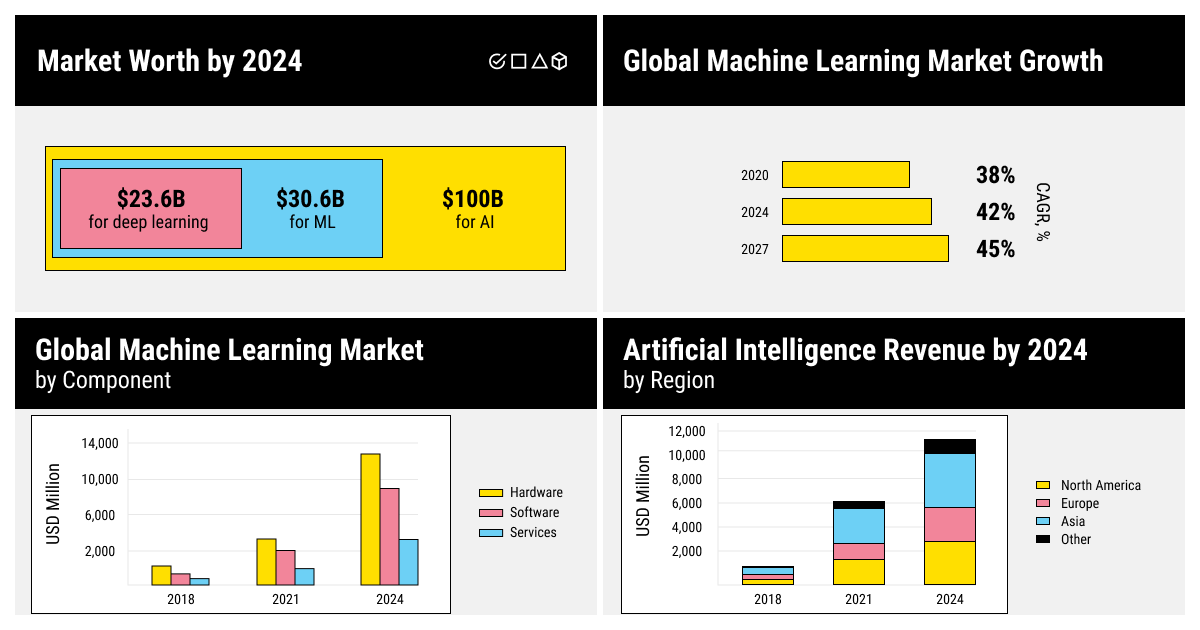
These numbers visualize the growing demand for machine learning as a service. But what is ML as a service, exactly, and how can you use it to benefit your business? In this article, we’ll define machine learning and MLaaS, give a few examples of these concepts, and provide you with a step-by-step guide on how to use machine learning as a service to gain your business a competitive edge.
MLaaS: What Is Machine Learning as a Service?
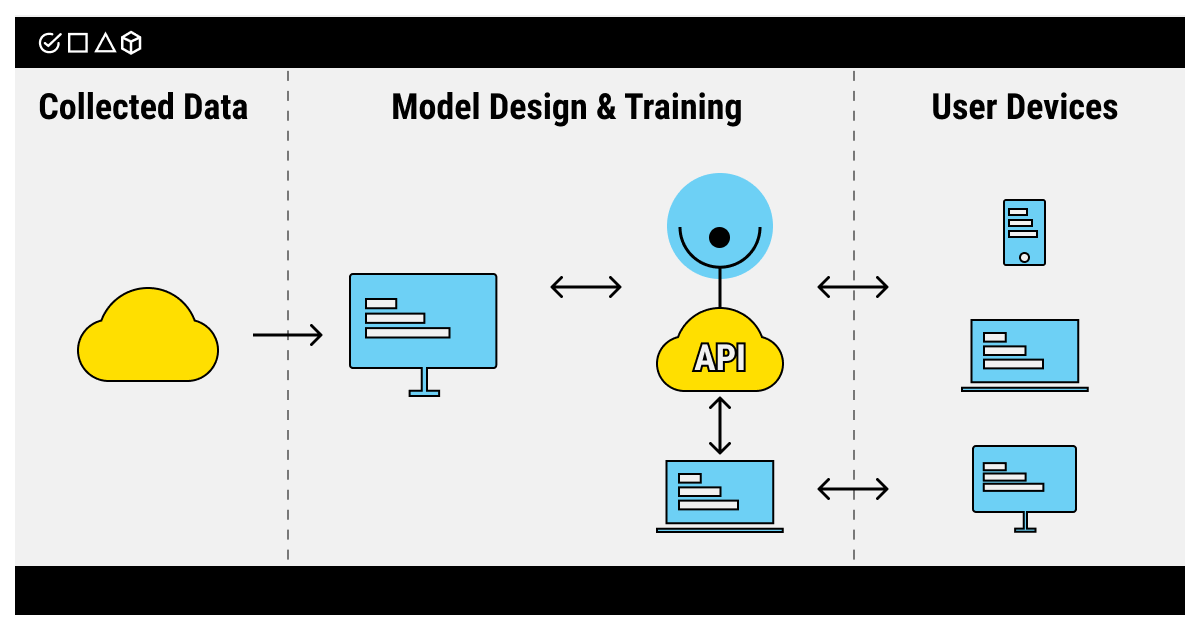
Not all of us specialize in data science and ML engineering. However, artificial intelligence is such a powerful concept today that there is rarely a business case that cannot gain a competitive advantage from adopting expert NLP services or, perhaps, computer vision services. For such cases, the boost in productivity and innovative approach can be reached by taking into the concept of machine learning as a service.
MLaaS is a way to enjoy the benefits of machine learning without bearing the risks of designing your own models and avoiding the costs of collecting an expensive in-house crew of developers and data scientists to build your own ML platform from scratch. Click to TweetExplaining Machine Learning
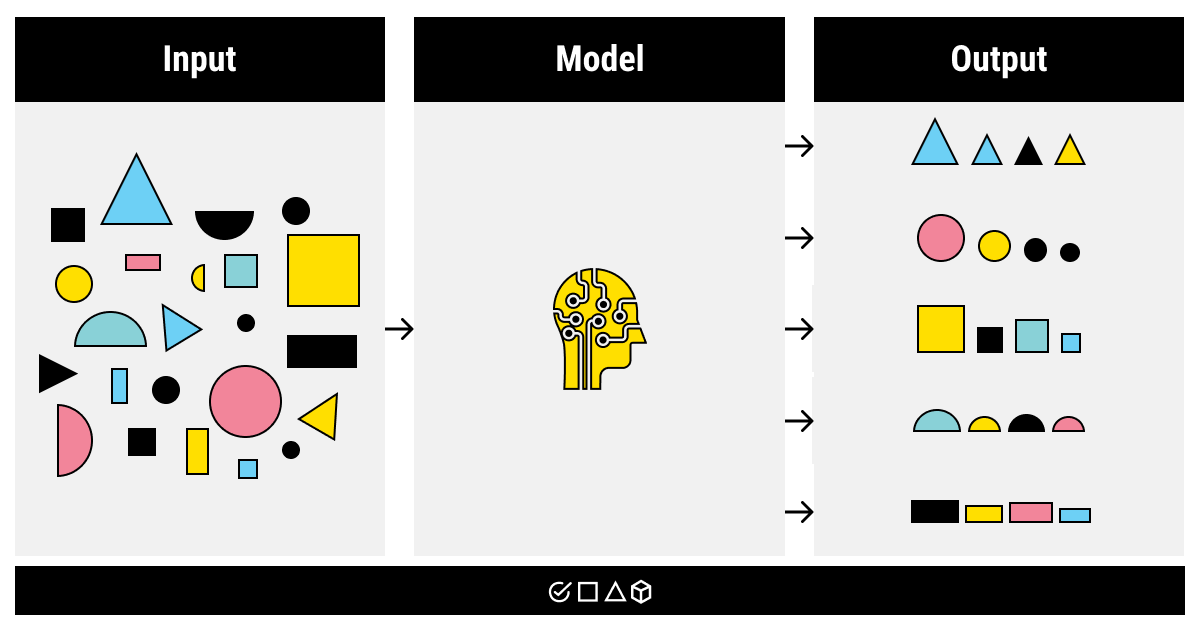
Let’s sidestep for a moment to talk about machine learning (ML). The definition of ML deals with letting the computers analyze big volumes of data with the goal of finding the patterns that could reveal the hidden insights to improve business performance. As we are surrounded by data in the modern world, it makes sense to put it to good use by letting the machines learn from it and make valuable predictions.
For example, let’s say you want to predict the weather. For this, you need to build a model that takes into consideration a variety of factors, from temperature to humidity to atmospheric pressure; you get the idea. Then, you collect the historic data, process and annotate it, and feed it into the computer to train. After the training session, you’ll be able to get the weather predictions. The accuracy will depend on the volume and quality of data you used to train your model.
The Value of MLaaS in Real-Life Scenarios
Getting back to machine learning as a service, let’s now take a look at how MLaaS can help your business. Machine learning as a service is widely applied for a variety of purposes and in a variety of fields, whether it’s health care, telecommunications, fintech, retail, or almost any other industry. There’s virtually no organization that wouldn’t benefit from MLaaS one way or the other (it can be in the form of making analytical predictions or automating the document workflow). Here are a few of the popular applications of MLaaS:
- Designing chatbots and virtual assistants
- Image or facial recognition
- Quality control and detection of manufacturing defects
- Automation of the business documentation workflow
- Natural language processing tasks
- Predictive analytics
- Data protection
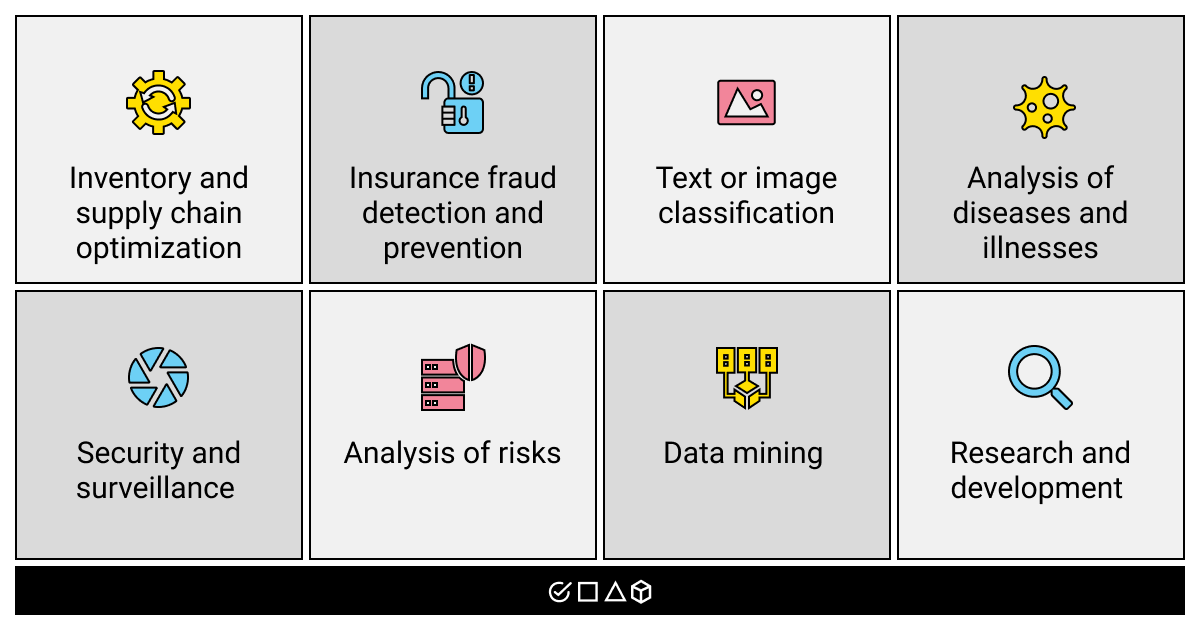
Machine Learning as a Service Market: Expanding Services and Growing Accessibility
The MLaaS market is already quite big and keeps developing at a fast pace. It was estimated at $5.7 billion last year. In seven years, it’s expected to further grow and reach nearly $305.62 billion.
Moreover, while the modern machine learning as a service market is dominated by a few key players, the choice will broaden in the near future to offer the businesses a variety of options to fit their needs. The demand for it will expand exponentially due to the development and spread of IoT devices and systems for enterprise automation.
Even more expressively, the most recent trend for the biggest players on the ML as a service market is switching to cloud-based AI solutions. Such tech giants as Google (Google Cloud), Amazon (SageMaker), Microsoft (Azure), and IBM (Watson) all offer their respective cloud-based MLaaS platforms. This allows satisfying the demand for flexibility, functionality, and accessibility for a business of any size.
In the future, there are two more trends we’d like to highlight. Speaking about the regions of influence, it is expected that North America will keep its dominance over the MLaaS market in the next few years. This trend will be supported by the presence of large companies (a few of them named above) and the inflow of investment due to the high expertise in machine learning development.
Arguably a little bit more surprising, MLaaS for forecasting deals with retail as the leading consumer of machine learning services. This industry will be propelled by the growing demand for personalization and developments in virtual technologies such as AR, virtual assistants, as well as smart storage management, and logistics. Moreover, as COVID-19 has made us embrace the digital lifestyle, more people are leaning into online shopping experiences, further driving the growth of the MLaaS market sharpened to fit the needs of e-commerce businesses.
Step-by-Step Guide to Using MLaaS for Your Business
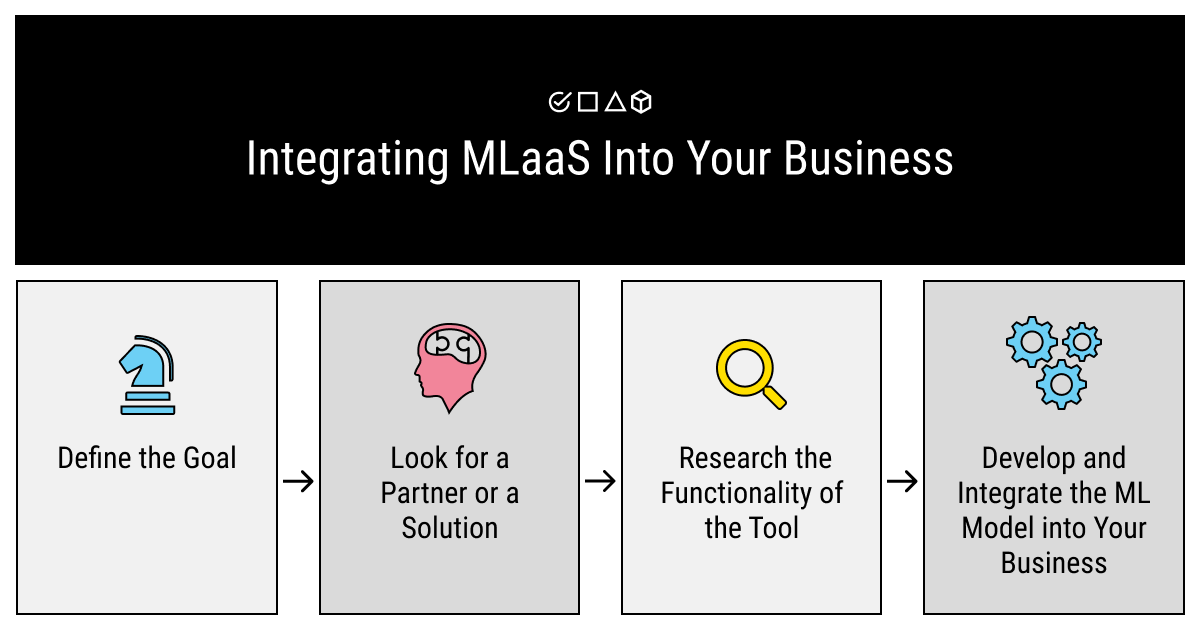
We hope you’ve gained a sufficient understanding of MLaaS both as a technological sphere and as a market. Now, we’d like to give you some practical advice.
Let’s say you’re a business operating in a sphere other than IT, artificial intelligence, or machine learning, but you have a great idea for an AI project and are on the lookout for an MLaaS solution that’ll help you with building a fitting ML algorithm. If you’re at a loss where to start, we’ve prepared a list of steps with a few useful tips for your convenience.
- Define your goal and describe your project in detail. Research the subject. Take into consideration your resources and the desired outcome. Formulate your vision before you plan on taking further action: this can save you a lot of time and resources in the future.
- Look for a suitable MLaaS partner or a ready MLaaS solution. Your choice should take into consideration the goals of your project, your budget, and time constraints. All of these will be essential in the long run. If you don’t have and aren’t planning on hiring a team of ML engineers, outsourcing the project to an MLaaS partner might be your best bet. On the other hand, if you plan to handle the model yourself, there are plenty of flexible and rich MLaaS solutions on the market.
-
When working with a third-party MLaaS business, find a common vision. An experienced partner will help you with deciding on all the required conditions before starting working on the machine learning model. If you opted for a ready MLaaS solution, make sure it offers the functionality you need. While all big machine learning as a service providers cover the majority of machine learning tasks, there are tools that specialize in certain tasks and correspond to the skill level in your possession.
For instance, Cloud AutoML from Google is a great tool for novices, while TensorFlow is better suited for experienced data scientists. SageMaker has some incredible out-of-the-box solutions for retail. Azure’s Bot Service Framework is a solution for the development of chatbots. And TF offers a broad choice of tools for working with deep learning and neural networks, as well as being a source for free annotated datasets for machine learning.
- Integrate the ready model into your system or workflow. Keep in mind that your work isn’t finished after deployment. Make sure you have access to the tools necessary to monitor and manage the working ML algorithm in case you chose the MLaaS solution or your ML partner doesn’t provide the after-launch support service.
These are the general steps any business new to machine learning can follow when tapping into the machine learning as a service market. Depending on the specifics of your project, your experience, and resources, the framework might change. For example, your steps might include data exploration; outsourcing the tasks of data collection, preprocessing, and labeling of your dataset; validating and testing your designed model using professional additional services; or after-deployment maintenance, experimentation, and scaling up, etc.
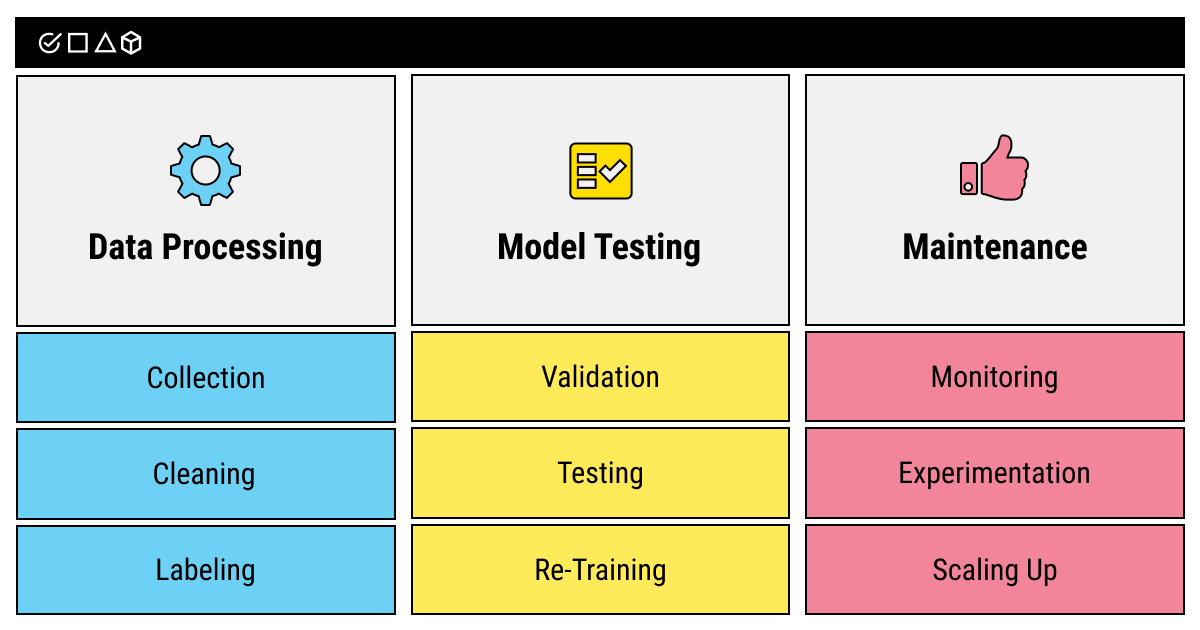
A Short MLaaS Summary
Undoubtedly, artificial intelligence and its subset, machine learning, pave the way for future technology development. The competitive benefits that smart integration might bring are vast and diverse. That’s the reason that so many businesses start adopting an AI-based mindset and ideas.
However, as not all of us can be genius developers, it’s important to acknowledge the recent trend of machine learning becoming a service accessible to any business without regard to the level of technical prowess it possesses. The ML as a service market shows unlimited potential in any sphere, from retail to manufacturing and from fintech to insurance.
In the next few years, we’ll be witnessing the booming growth of MLaaS platforms from both industry giants like Google, Amazon, and Microsoft, to smaller, niche-focused solutions fit to support the growing demand of companies and enterprises of all sizes.
If you want to jump on the bandwagon of AI adoption, there are a few simple steps you should take. First, think about your goal and clarify your vision: what result are you looking for? What services do you want to outsource? After answering the core questions, start looking for an ML as a service partner or a ready MLaaS solution that offers the full functionality to satisfy your requirements. When your model is built, trained, and tested, integrate it into your system. Keep a watchful eye on it and don’t hesitate to experiment, and soon you’ll see the ripening ML fruit of your labor!
FAQ
What factors contribute to machine learning as a service cost structure, and how do providers determine pricing for this type of service?
The cost of MLaaS is influenced by several factors, including
- Complexity of algorithms and models used
- Amount of data processed and stored
- Level of customization and integration required
- Compute resources needed for training and inference
- Support and maintenance services provided by the provider
How to compare and select the most suitable machine learning as a service providers for implementing predictive analytics within a business?
When choosing a ML as a service provider, consider factors like their expertise in your industry, the scalability of their solutions, and the ease of integration with your existing systems. Additionally, evaluate their track record and customer support for a comprehensive decision.
What are some top MLaaS platforms that are widely used and trusted in the industry?
Some of the top MLaaS platforms include Google Cloud AI Platform, Microsoft Azure Machine Learning, Amazon SageMaker, IBM Watson Studio, and Databricks.
Table of Contents
Get Notified ⤵
Receive weekly email each time we publish something new: