This year promises to become a turning point in logistics. More and more supply chain leaders are considering AI-powered technologies for streamlining logistics operations and maximizing performance. No wonder that half of these logistics companies will make investments in applications specifically designed to support the implementation of AI and advanced analytics capabilities by 2024.
The trend is due to the fact that AI solutions keep evolving, along with more data being created and processed daily. And so the demand for novel solutions in the field, including machine learning, is growing exponentially. From optimizing supply chains to enhancing last-mile delivery, the integration of machine learning in the logistics industry is revolutionizing the way goods are moved, stored, and managed.
As we step into a new era in logistics, with data-driven decision-making taking center stage, businesses worldwide are leveraging the power of machine learning. Let’s see how technology and advanced algorithms help them streamline operations, reduce costs, and gain an unprecedented competitive edge.
Why Is Machine Learning in the Logistics Industry So Promising?
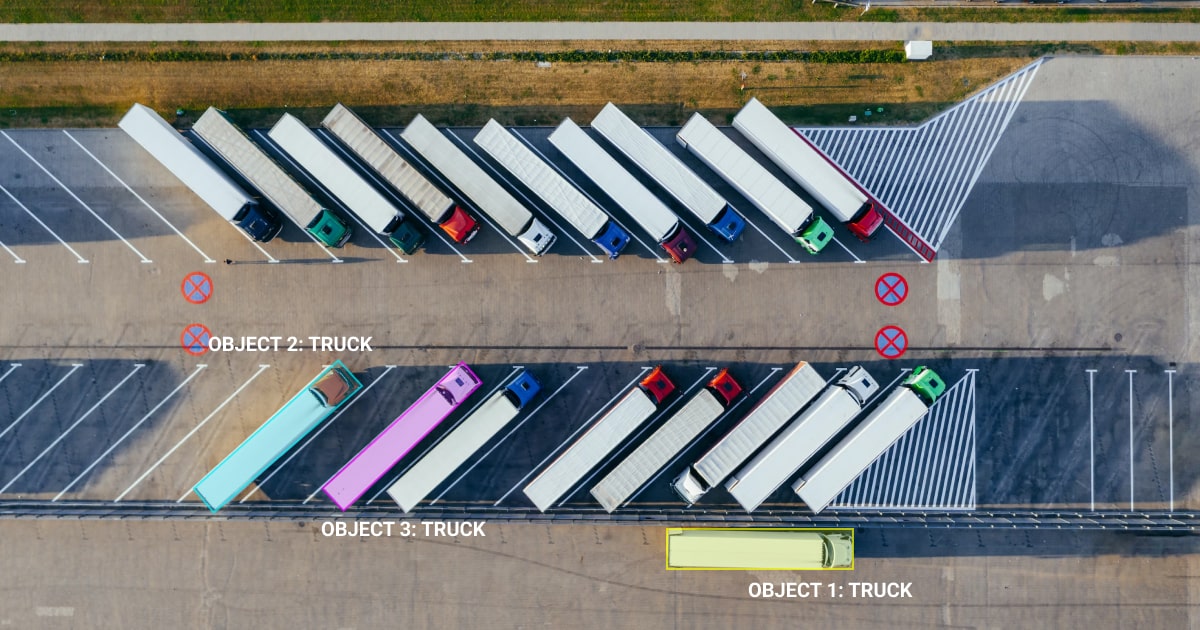
Let’s imagine a world where warehouses anticipate demand and delivery routes self-optimize in real-time. Or perhaps customer preferences that are accurately predicted before they even make a purchase. Machine learning turns this all into a reality.
By analyzing vast amounts of historical and real-time data, and applying pattern recognition in machine learning, algorithms are able to uncover hidden insights and make accurate predictions. This way, machine learning drives logistics operations to unprecedented levels of efficiency, whether it’s predicting demand fluctuations, optimizing inventory management, or minimizing delivery times.
Moreover, machine learning empowers logistics professionals with the foresight and agility to make informed decisions, adapt to dynamic market conditions, and meet ever-changing customer expectations.
What Are Key Technologies Empowering Machine Learning in Logistics?
Machine learning technologies have revolutionized the field of logistics, offering advanced tools and techniques to optimize operations and enhance decision-making. The most common technologies used in this area are:
Predictive analytics uses models and algorithms to analyze data and make predictions about the future scenarios. It helps logistics by identifying patterns, anticipating problems, and taking action.
Computer vision allows computers to identify objects in images and videos autonomously, without the need for human involvement. It benefits logistics by tracking inventory and identifying damaged parcels, while also aiding robotic navigation in warehouses.
Machine learning and the Internet of Things (IoT) coming together can revolutionize logistics. It enables the efficient planning of supply chain routes, accurate demand prediction, proactive identification of disruptions, and overall improvement of the customer experience.
Logistics Problems That Machine Learning Can Solve
Supply chain companies on a global scale perpetually encounter challenges in their dynamic niche. Ensuring seamless delivery of items from manufacturers to end users is crucial, but constant obstacles pose risks and undesirable outcomes. To address these pressing issues, companies look for advanced AI solutions for various urgent matters, such as:
Inaccurate demand forecasting;
Inefficient route planning;
Lack of real-time visibility;
Manual data analysis;
Poor inventory management;
Unoptimized transportation schedules;
High maintenance costs;
Limited risk management capabilities;
Difficulty in detecting fraud and security breaches.
These problems can lead to unsatisfied customers, downtimes, delays, and breaches of contracts. Manual efforts and basic tools are not enough to address them. Hence, logistics firms are turning to solutions like machine learning to predict future events and protect themselves from unforeseen disruptions and logistical challenges.
We can see that the traditional supply chain as we know it is no longer viable. It fails to align with the requirements of time and demand. The future belongs to a more adaptable and digitally-driven supply chain, and ML in logistics plays a crucial role in enabling companies to construct it.
Top 8 Machine Learning Use Cases in Logistics to Keep an Eye On
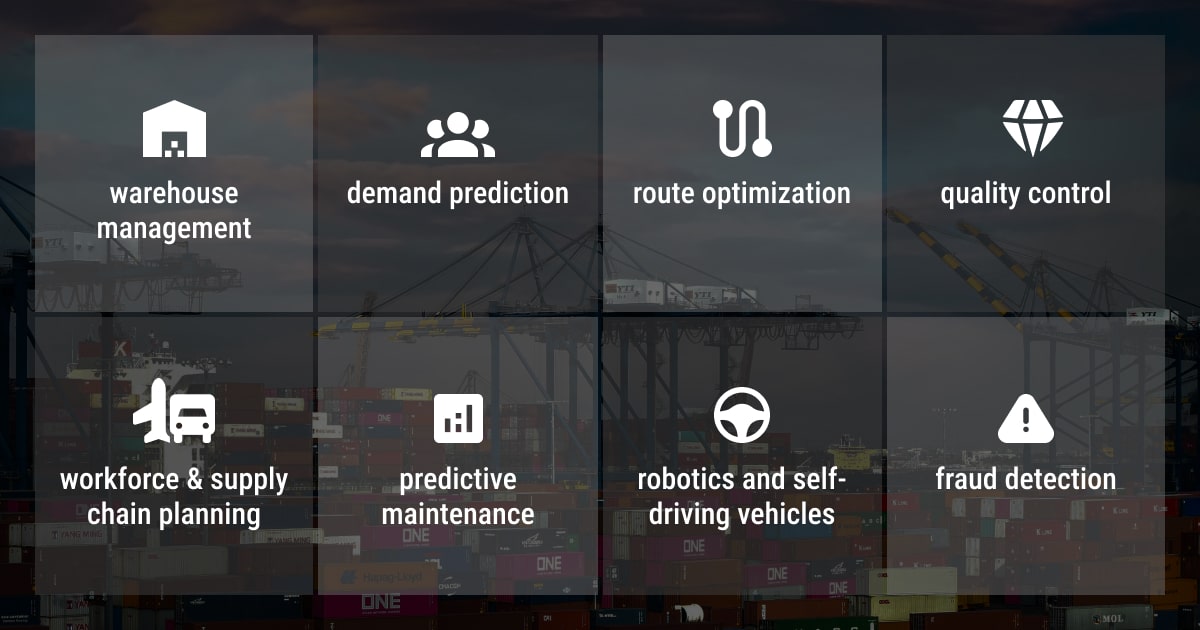
Today, businesses are actively embracing digital transformation in their supply chain to enhance operational efficiency, customer satisfaction, and cost-effectiveness. In fact, Gartner estimates that by 2026, AI and data science will be utilized in more than 75% of commercial supply chain management applications.
Here are the key machine learning use cases in logistics:
Warehouse management
Machine learning has revolutionized logistics management and analysis of warehouses by providing valuable insights into inventory levels, stock availability, fulfillment rates, shipment time frames, and other critical metrics. This eliminates manual errors causing delays or lost shipments, optimizes inventory by preventing over and under-stocking, and automates manual tasks.
Moreover, one can deploy computer vision services to detect arriving packages, scan barcodes, monitor the warehouse perimeter, track employees, and prevent thefts and violations. In addition, the integration of ML into security camera analytics enables real-time monitoring, immediate alerts, and appropriate actions to ensure only authorized personnel access the warehouse.
Demand prediction
By generating more accurate predictions compared to traditional methods solely reliant on historical data, ML algorithms enhance AI-driven demand forecasting in supply chains. This approach can reduce errors by up to 50%, enabling logistics companies to proactively adjust their operations and be better prepared for sudden increases or decreases in demand.
They can analyze various factors such as past orders, traffic patterns, customer behavior, inventory trends, and even weather conditions to create models that effectively anticipate demand fluctuations. By processing vast datasets using additional services, ML systems identify patterns, detect demand signals, and establish correlations between variables. Not to mention their ability to continually update and retrain the models, ensuring adaptability to changing scenarios and optimal performance across diverse settings.
Route optimization
Data analysis of traffic patterns and location distances helps companies compute optimal routes for efficient freight delivery in real-time. This ML-powered route optimization, when coupled with predictive analytics, assists logistics companies in saving valuable resources by promptly notifying them about costly traffic congestions or delays caused by weather or unexpected circumstances.
The trucking industry incurs significant losses, ranging from nearly $75 billion to over $42 billion (the most recent available data), due to bottlenecks. However, with the advancement of ML in the logistics industry, route optimization is becoming increasingly simplified, enabling the determination of the most effective order for stops while minimizing driving time and distance. Moreover, ML algorithms can monitor and predict traffic conditions and other variables, such as peak hours in logistics centers, allowing companies to proactively avoid potential disruptions and accurately forecast shipment times.
Quality control
ML in logistics is highly effective for quality control as it excels in recognizing visual patterns, addressing the common issue of receiving damaged products that lead to negative customer reviews. It offers numerous possibilities for physical inspection throughout the supply chain. By swiftly analyzing extensive datasets, machine learning algorithms have proven valuable in automating quality management processes within logistics centers.
These algorithms efficiently identify common patterns, enabling the segregation of packages containing damaged items, thus enhancing overall quality control. As a result, logistics firms make sure only the best products reach customers, reducing the need for returns and replacements. This, in turn, leads to cost savings and enhances the overall reputation of the company in the eyes of consumers.
Workforce & supply chain planning
Advanced technology plays a vital role in workforce and logistics planning by facilitating efficient staffing and delivery optimization. Through the analysis of historical data encompassing traffic patterns, customer orders, and shipment demand, ML algorithms can accurately predict optimal delivery timings.
This enables logistics companies to appropriately allocate staff resources during peak periods and avoid overstaffing during off-peak periods, ensuring workforce optimization. ML-driven decision-making processes empower supply chain management by balancing demand and supply, optimizing delivery procedures, and leveraging datasets and advanced algorithms to enhance overall operational efficiency.
Predictive maintenance
Machine learning allows analyzing data from on-board sensors and external factors such as road conditions, enabling the identification of upcoming maintenance requirements. This predictive maintenance solution empowers logistics companies to service their vehicles at the most optimal time, effectively avoiding costly breakdowns and unforeseen repairs.
Previously, logistics planners relied on manual assessments using traditional methods. But they were time-consuming and prone to inefficiencies. Now, however, the integration of machine learning in transportation and logistics enables automation and more accurate data collection. This enables logistics technology companies to boost efficiency, cut maintenance costs, and ensure optimal performance and longevity of their vehicle fleet.
Robotics and self-driving vehicles
Robotic process automation has particularly evolved in online retail and shipping sectors. Case in point, industry giants like Amazon and Alibaba have pioneered the automation of their warehouses using intelligent robotic process automation, with robots handling 70% of the workload. They collect and pack products for delivery. These robots are equipped with sensors to prevent collisions, can lift heavy loads up to 500 kilograms, and are accessible to workers through Wi-Fi.
Similarly, in the realm of delivery, ML-powered autonomous vehicles are capable of navigating complex urban and rural areas. They help companies reduce labor costs, optimize fuel usage, ensure driver safety, plan optimal routes, and even predict traffic patterns. Additionally, the utilization of unmanned operations in logistics through drones enables faster and more secure package deliveries by leveraging real-time data and advanced navigation systems.
Fraud detection
Fraud incidents within the supply chain are a frequent occurrence, often due to inadequate shipment tracking and limited visibility into employee activities within warehouses. In such a complex and high-stakes environment, trust alone is insufficient, necessitating the implementation of machine learning to safeguard assets.
ML ensures end-to-end transparency throughout the supply chain, allowing business leaders to closely monitor each step and route. By utilizing risk rules and comparing them with incoming data, ML models promptly identify inconsistencies, triggering real-time alerts for employees. This proactive approach, enabled by machine learning in logistics, enhances supply chain security and empowers companies to prevent financial losses and potential customer data breaches.
Machine Learning in Logistics: Datasets & Steps for Efficient Implementation
The availability of large and diverse machine learning datasets has played a vital role in the development of AI-driven logistics. Machine learning algorithms depend on extensive data sets to discover patterns and gain valuable insights, which can then inform decision-making in the field of logistics. By leveraging large-scale datasets, companies can gain valuable information about customer preferences, demand patterns, transportation routes, and inventory levels.
As such, these insights enable companies to make proactive adjustments to their logistics operations. Furthermore, the analysis of machine learning in logistics datasets containing historical data on order volumes, transportation routes, and delivery times, helps identify patterns and optimize scheduling and resource allocation. The analysis of logistics datasets also strengthens supply chain management practices by providing valuable insights into demand forecasting, inventory management, and risk assessment.
But how do logistics firms embrace machine learning in the face of evolving customer expectations and market demands? These are the steps we suggest for the most successful integration of machine learning in logistics industry:
Problem definition: Collaborate with supply chain experts to identify critical business challenges that can be addressed by ML implementation.
ML capacity: Evaluate workforce impact, identify skill gaps, assess long-term ROI, and set realistic expectations for ML integration.
Data governance: Strategically acquire and organize relevant datasets for ML training, focusing on the target issue at hand.
Collaboration: Build partnerships to exchange region-specific information, leveraging abundant data for enhanced ML performance.
Industry experts: Involve supply chain professionals and data labeling experts, like Label Your Data, early on to leverage their expertise in developing accurate ML forecasting models.
Final Thoughts on Tech-Managed Supply Chains
Machine learning offers unprecedented opportunities for the development and improvement of the logistics sector. Once again, the integration of ML in the logistics industry enables real-time decision-making, demand forecasting, efficient route planning, predictive maintenance, and numerous other solutions that make it easier for logistics companies to adjust to the massive AI breakthrough.
By embracing these technologies and advanced ML models, logistics can open new levels of managing their supply chains, adapt to market dynamics, and meet evolving customer expectations. But before they get started, they need expert annotations to fuel their ML models with the best quality data for the most optimal results in AI-driven logistics.
Contact our data labeling team to take your AI initiatives to new heights with our expert guidance!
FAQ
How is machine learning used in logistics?
Machine learning in logistics is used to optimize route planning, enhance demand forecasting accuracy, automate warehouse operations, enable real-time tracking and visibility, and mitigate supply chain risks.
What is the role of AI in logistics?
AI plays a crucial role in logistics by allocating the right resources to each day-to-day activity, enabling autonomous decision-making, real-time visibility, and optimizing supply chain operations. This makes customer service better and allows for predicting demand and managing risks using predictive analytics.
How does machine learning in the supply chain improve operational efficiency and drive innovation?
By leveraging machine learning in the supply chain, operational efficiency can be enhanced through intelligent decision-making, automated process optimization, predictive insights, and improved resource allocation.
Table of Contents
Get Notified ⤵
Receive weekly email each time we publish something new: