Data Annotation Is The Key Ally in Supporting AI Initiatives
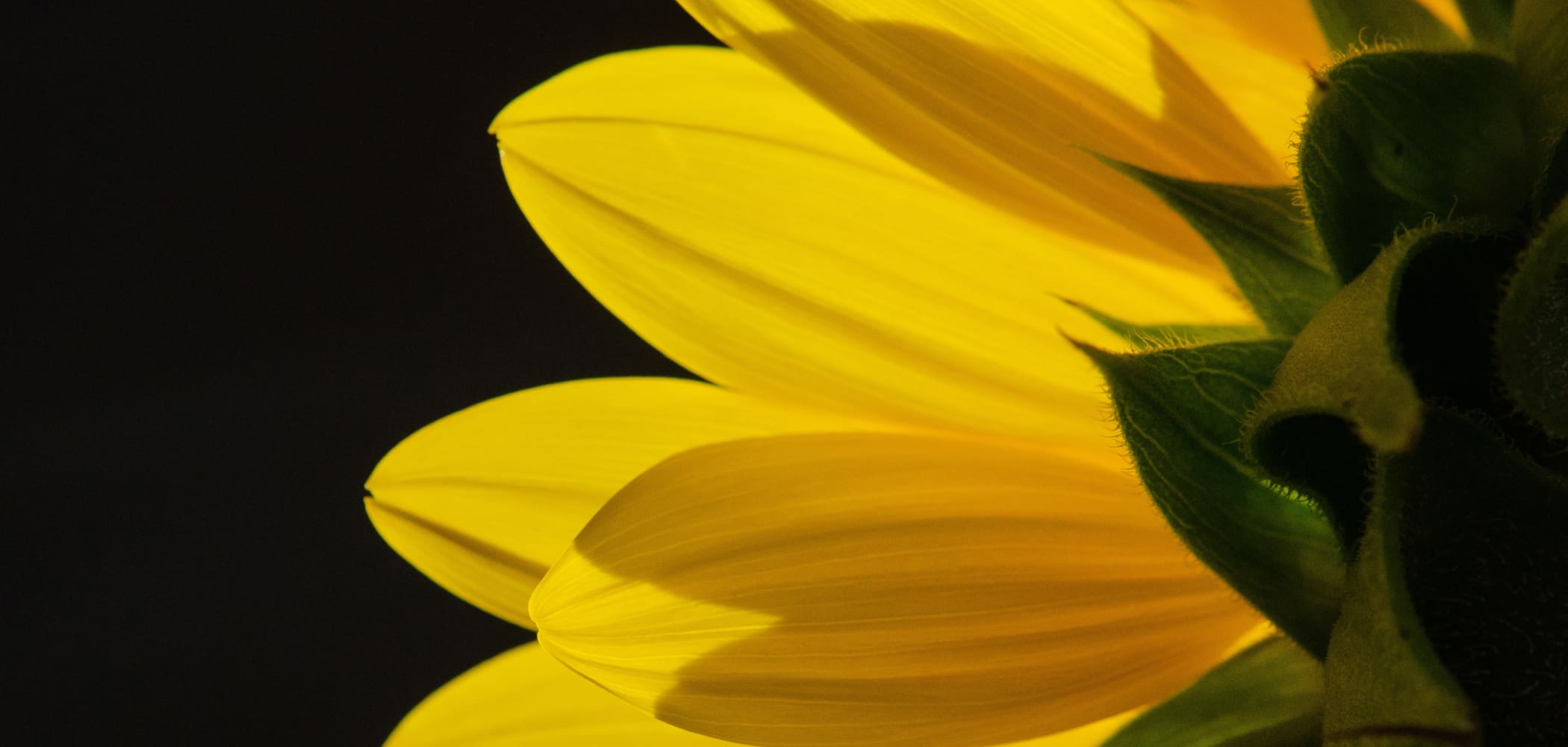
According to current research, data preparation and labeling accounts for over 80% of engineering work in machine learning projects. Besides, the third-party data annotation market is predicted to almost triple by 2024. As a data annotation services provider ourselves, we couldn’t be more interested in learning more on the subject.
The data annotation market is growing by leaps and bounds from year to year. For companies, this means they have no choice but to start mastering the art of data annotation and integrate it into their AI-focused startups.
Yet, we rarely see the behind-the-scenes of how today’s businesses work. Thus, we don’t even recognize how most of them are already either fully or partially automated. The bottom line is that modern leading industries heavily rely on artificial intelligence and the capabilities it provides. And, of course, they cannot do without expert data annotation, something that no successful ML project can be built without.
In this article, we aim to emphasize the importance of data annotation in the development of some of the major industries today, based on our long-term experience with many sectors at Label Your Data. Keep reading to learn more about the soaring demand for annotated services across the global industry!
Applications, Types, and Challenges of Data Annotation in Different Industries
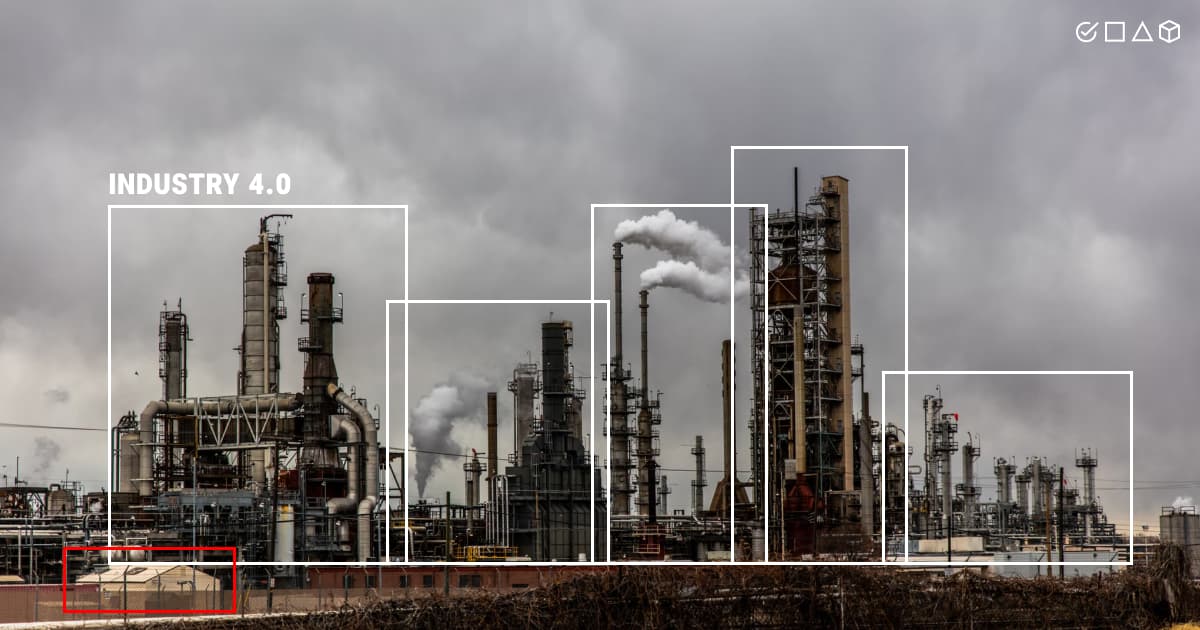
To our knowledge, almost 95% of all the ML algorithms used by major industries today are supervised. This means that most of the ML algorithms and, thus, the industrial projects to which they contribute, rely on labeled data.
Therefore, each large-scale AI project deployed for a particular industry must be provided with high-quality and secure annotated data relevant to the project’s goals. And each dataset should be complete with labeled instances. The industrial data (i.e., medical data, customer data, vehicle data, crop production data, etc.) would be incomplete otherwise, and supervised learning would be impossible.
What are the main applications of annotated data for the biggest industries?
Industrial Applications of Data Annotation
Machine learning solutions can bring many advantages to the key players in the global industry. Sophisticated ML algorithms can be applied to different sectors and solve different problems that the industry has raised. However, industrial data used for the ML models must be carefully processed and annotated by experts. If you need high-quality, secure, and accurate annotation for your ML project, contact us for a quote.
In AI, knowing how to handle industrial data generated by systems, sensors, and assets can be a real game-changer for enhancing the majority of industrial operations and helping industries succeed. So, let’s take a look at the main industrial applications of data annotation!
Industrial Robots
AI-enhanced industrial robots are used for a variety of tasks in manufacturing. These smart machines can be used for performing visual functions and, thus, enhancing visual performance in a specific field. Such tasks include monitoring, analyzing, and processing moving objects. Also, industrial robots can do more complex work, like actual detection, measurement, and control.
High-quality data annotation is the main driving force behind advanced technology such as the industrial robot. Labeled data is commonly used for tasks such as training and validating computer vision (CV) models for robotic navigation systems. Therefore, having annotated data on hand helps one ensure that the robots can interact with humans, objects, and the environment safely and that operations run smoothly. Labeled data is used to train an ML model for the industrial robot to perform the following tasks:
- Object recognition
- Object tracking
- Industrial robot navigation
- Robot arm guidance
- Crack detection
Predictive Maintenance
Annotated data is crucial for creating and integrating predictive maintenance techniques in the industry. This is primarily used for analyzing the condition of the equipment in service and determining when to perform maintenance. Such a data-driven method requires high-quality labeled data to build ML models that will predict potential issues with equipment at an early stage.
This way, annotated data helps maintenance teams stay alert and better control the operation and serviceability of their equipment. With labeled data, these teams get accurate predictions from ML models and can promptly respond to possible technical malfunctions. Plus, it increases the longevity of the inventory used during the manufacturing stage.
Quality Control
CV technology has already proven to surpass the capabilities of human vision. It’s, by far, the decade’s most important AI-based technology. For this reason, computer vision is commonly used by many leading industries through cameras and edge devices. They are more effective, precise, and more detailed than human sight (though, you can argue with that).
As we already know, annotated data is a valuable resource for an ML algorithm that is used to teach machines a human-like vision of the surrounding world (in our case, the industrial environment). When the CV model is combined with a cloud-based data processing framework, it can quickly detect errors and automatically coordinate the response.
The Main Data Annotation Types for Industrial Purposes
In industrial settings, AI takes the lead in ensuring safety, security, as well as fast and efficient production due to the many automation capabilities it can provide. Moreover, AI has enabled advanced quality control systems in the industrial environment, putting human workers’ minds at ease.
Some of the main data annotation types that the key industries today use are:
- Object detection/Object tracking. 2D boxes and 3D cuboids are used to draw frames around the objects on an image or add a dimension to the initial frame around the object.
- Instance segmentation. Polygonal annotation with more complex outlines around the objects to define them more precisely.
- Semantic segmentation. Labels each pixel of an image with a class and then clusters them together.
The Industry-Specific Overview of Data Labeling Needs
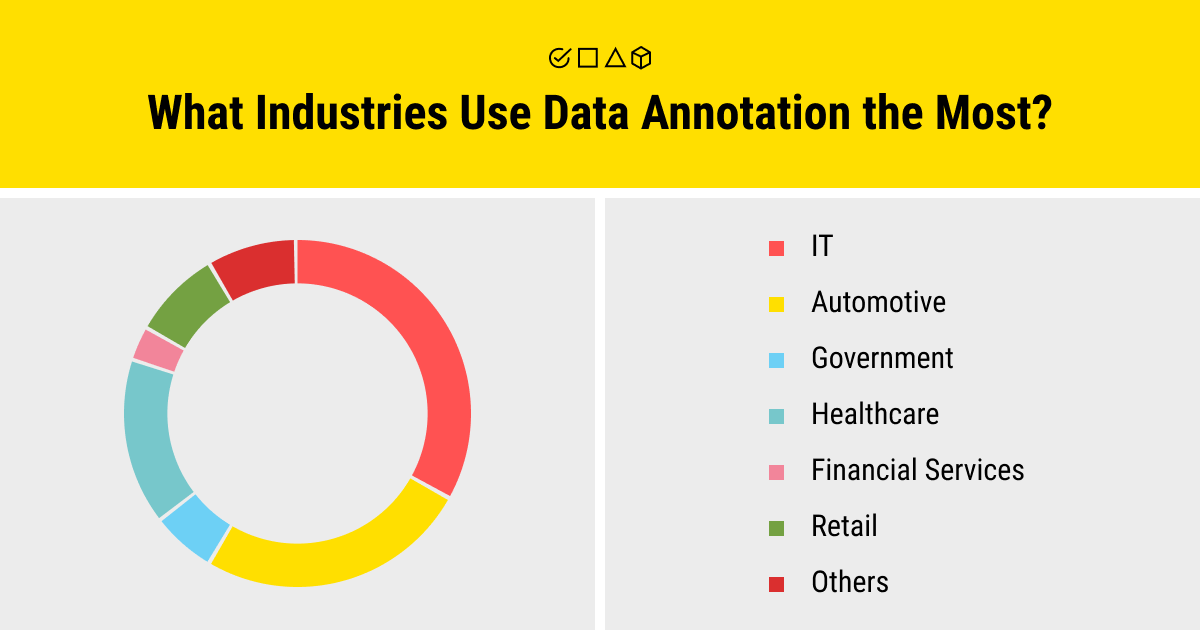
Organizing a well-balanced labeling process is not an easy task for a company that has just started learning the ropes of AI. We at Label Your Data know that this process requires a systematic approach to annotation, as well as analyzing the data that you work with and selecting the optimal workflow.
To relieve yourself of all the hassle of the labeling process and dedicate time to your business, you need a reliable data annotation partner. If you are in search of one, contact our team of skilled annotators and get your quote. But if you want to explore how annotation is applied by different industries in the meantime, let’s get straight to the point!
How do modern industries accelerate their ML initiatives with training data and leverage data annotation to achieve success?
— Automotive
Our team at Label Your Data has always expressed a keen interest in the automotive industry, especially since we started working with our partners from Germany. Labeled data is the cornerstone of safe, secure, and accurate driverless experience, an ever-growing trend today.
AI and self-driving vehicles is a match made in heaven. But there’s even a stronger bond between the self-driving cars companies and data annotators. To build an autonomous car and mitigate all the safety concerns, a properly trained ML algorithm is needed to power the vehicle. It requires a lot of image and video data to be labeled so that the self-driving system can be trained to recognize objects (e.g., other cars or pedestrians) on the roads, streets, and many other things that are necessary for a safe driverless model.
We believe that the future of the transportation industry as a whole is highly dependent on machine learning and expert data annotation. Driverless cars require professionally annotated images and videos provided by multi-sensor cameras so that the vehicle can engage with the environment in the same way as human drivers do.
— Healthcare
Another major industry that has been long reaping the benefits of AI is the medical sector. Advanced technology has been particularly useful during the recent pandemic. AI alleviates the burden weighing on healthcare providers, so they can dedicate more time to patients. We can assume that AI helps maintain the personal touch in the medical setting.
What role does labeled data play in medicine? Healthcare isn’t an easy field to deal with, however, the right data labeling partner can be a big help here. Medical companies are creating AI-powered products that can recognize patterns in the medical images of CT scans, X-rays, MRI reports, ultrasounds, and mammograms. They need this to train the ML models to set a diagnosis and suggest treatment.
To make it work, these AI systems require annotated data of medical images and scans. It’s also mandatory to engage subject-matter specialists (aka medical personnel) to check the quality and correctness of annotated data. They need to confirm the diagnosis generated by machines because the final decision on patient treatment is still up to the doctors.
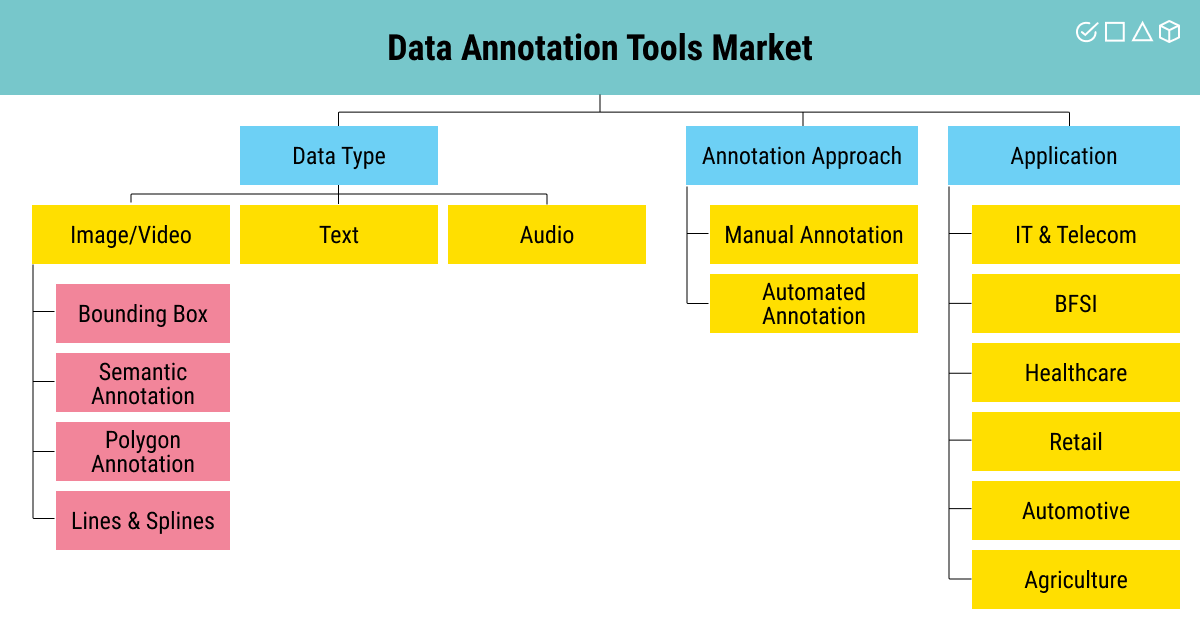
— Agriculture
Speaking of industries that impact our lives the most, we couldn’t get past the agricultural sector. It’s the one that ensures a sustainable food supply for our growing population. Can you imagine data annotation having something to do with that?
Agriculture significantly relies on automation technology, such as robotics and drones, that help monitor, analyze, and manage large amounts of crops. Labeled data helps farmers predict harvest yields, protect the crops, and cut water consumption. Sounds like data annotation supports ecologically sustainable development. AI-based agricultural robots can harvest ripe crops, provide aerial monitoring of the field, fertilize the soil, and even assess crop growth. They use LiDAR technology that generates a 3D Point Cloud, which needs to be labeled so that the robot can recognize the objects and the environment it operates in.
— Retail
AI has found its way into the modern retail industry, and data annotation made it possible. Since AI offers a serious boost for the retail sector, and large distributors massively integrate it into their business operations, the demand for expert data annotation grows too.
For example, facial recognition is a crucial task for building self-checkout systems, so facial annotations are required for this process. Another example is an automated warehouse, where AI-powered robots are commonplace and need labeled data for the LiDAR technology that they rely on. Data annotation helps the retail industry develop cutting-edge technology by generating machine-readable data for the ML algorithms that make this technology function properly.
— Social Media
Social media networks comprise a lot of sensitive user data, like images, videos, and text messages or posts. This is the data that the AI-based algorithms process with every single user’s action on social networks, like Facebook, Twitter, or Instagram. Data annotation is a crucial player here and can be applied for different tasks, including sentiment analysis and natural language processing (NLP).
Companies are using these AI solutions to monitor the emotional perception of their brand, product, or service on different social media platforms, instead of wasting time manually counting likes or reading reviews. Also, the growing tendency towards CV solutions (e.g., facial recognition, tag suggestions) has raised the demand for high-quality annotation for social media to analyze user interaction via different channels with no human intervention at all.
Why Should Industries Care About Data Labeling?
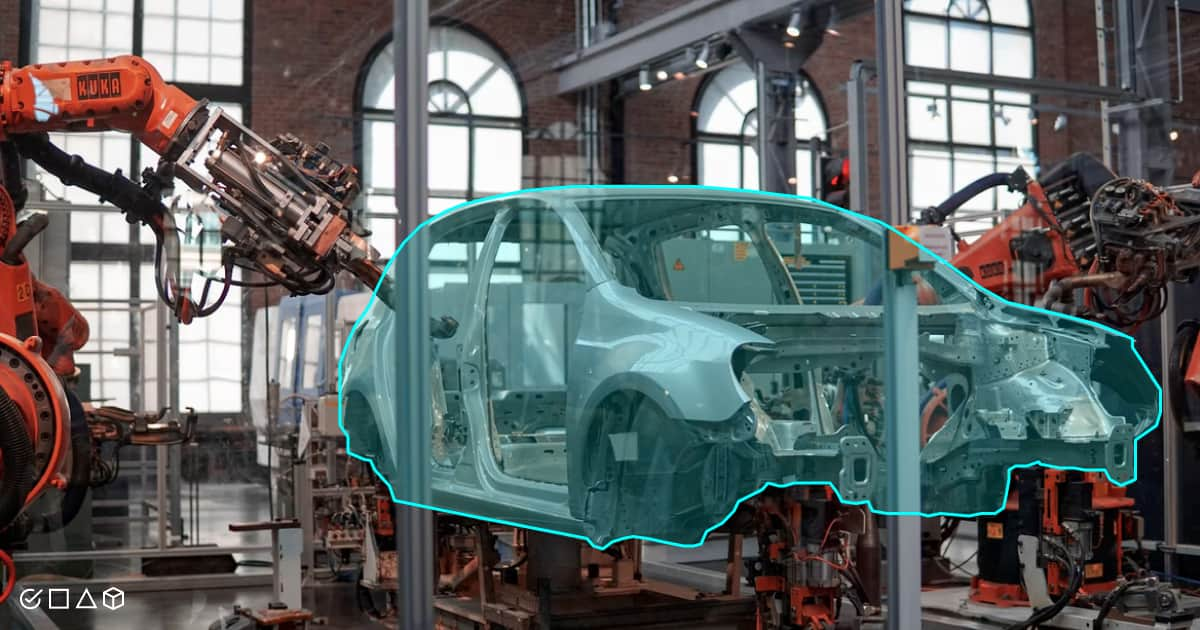
Data is the most valuable asset a business can have. Therefore, learning how to deal with data in today’s digital environment makes perfect sense for AI-oriented industries, including healthcare, transportation, retail, agriculture, and many others. And very soon, this will apply to all industries that wish to expand and control the global economy.
Data annotators have made a commendable effort to make this work. By providing high-quality, secure, and accurate labeled data, annotators help the largest industries today perform supervised ML. Simply put, if the data isn’t labeled properly, it’s hard to use it for machine learning the way it should be used.
While the industry is still heavily reliant on manual data annotation, it’s crucial to enlist the support of a trustworthy annotation partner, like Label Your Data. Request your quote, so you don’t have to deal with all the challenges that companies experience when annotating their data.
Label Your Data will help your business seize all the benefits of AI to the fullest!
Written by
One of the technical writers at Label Your Data, Yuliia has been gradually delving into the intricate aspects of AI. With her strong passion for the written word and technical expertise, Yuliia has developed a keen interest in the evolving field of data annotation and the power of machine learning in today's tech-savvy world. Check out her articles to learn more about the complex world of technology and find the solutions that work best for your AI project!