The Role of Data in Constructing Autonomous Vehicles
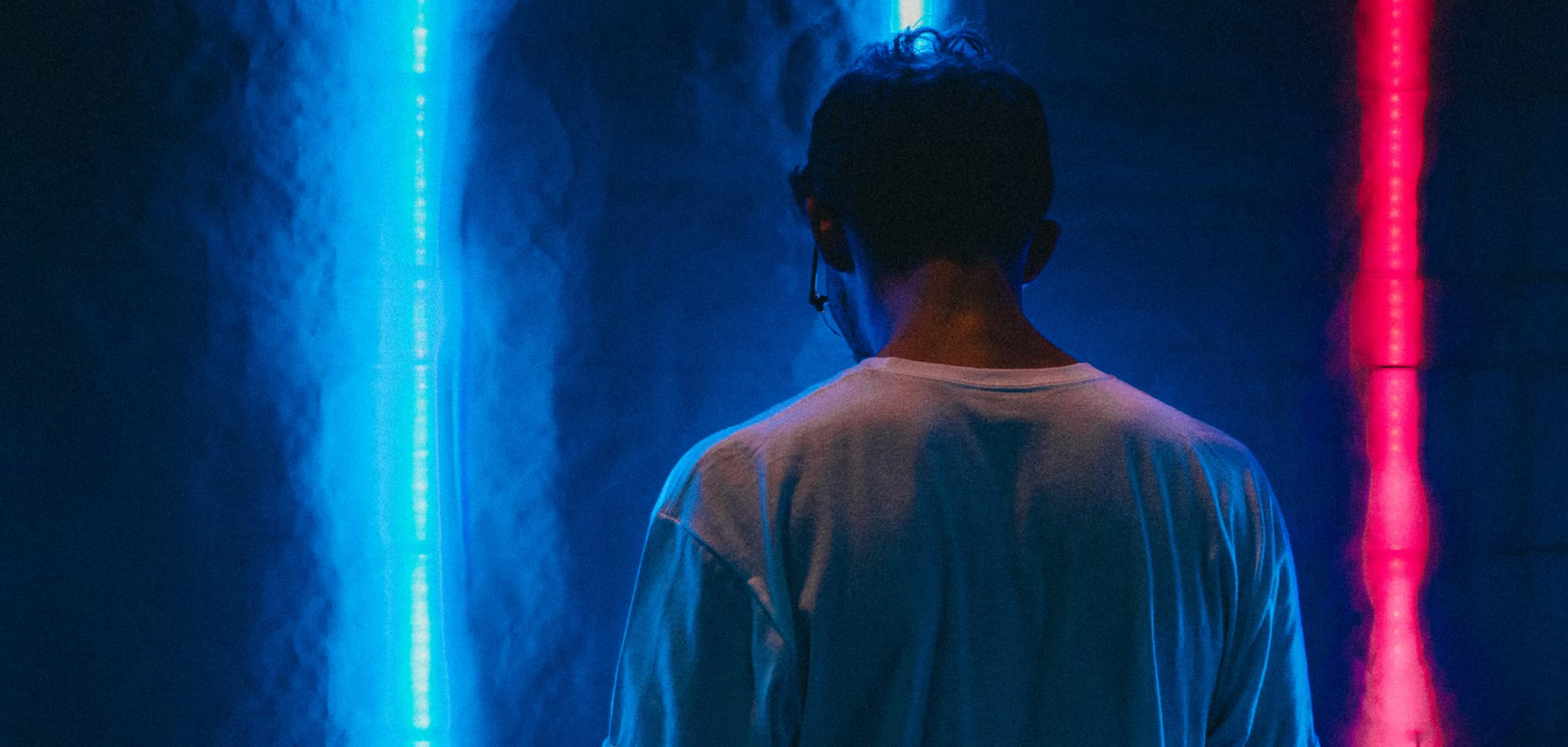
Data is at the core of all modern technologies, but for some, it plays a decisive role in keeping such technology safe for humans. Driverless cars have gained quite a foothold in our daily lives, and have even become mandatory in some countries for environmental purposes. The current statistics on autonomous driving demonstrate that the rising deployment of unmanned cars has resulted in a nearly 60% reduction in hazardous emissions.
However, even last year, customers views on autonomous cars varied, with more of them willing to use a semi-automated vehicle instead of a fully automated version. So, why is there still so little trust in AI-driven automated technology in today's tech-savvy world?
There's a wide range of machine learning applications in autonomous cars. Nevertheless, to build a safe autonomous vehicle, it's crucial to provide the driverless algorithm with a sufficient amount of well-annotated data. Labeled images or videos are integral in this case because they help train driverless cars to better recognize various objects on the road. Thus, accurate data annotation for self-driving cars became imperative in training a driverless ML model using supervised approaches. Poor data labeling practices in autonomous driving can cause serious bottlenecks in the development and production stages.
So, it's vitally important to learn and understand how such automated technologies work, so as not to let AI cross the line and let it remain a safe invention of mankind. We'll start from the most paramount aspect of autonomous driving, that is data annotation. Both data collection and data annotation are quite challenging, resource-intensive, and costly tasks in developing machine learning algorithms or deep learning networks for driverless systems. Hence, end-to-end solutions are essential in the autonomous sector, which is why automation of the data labeling process became so popular today.
Having successfully worked on one of our major driverless projects with the Elefant Racing team, our Label Your Data annotators have some wisdom to share with you. It’s still an ongoing project for our team and a telling case worth learning from. Keep reading to find out all the ins and outs of autonomous driving and the role that data annotators hold in this story!
In case you already know what you need in your driverless project, contact our team to enlist the support of professional data annotators!
Data Labeling for Autonomous Vehicles Technology Explained
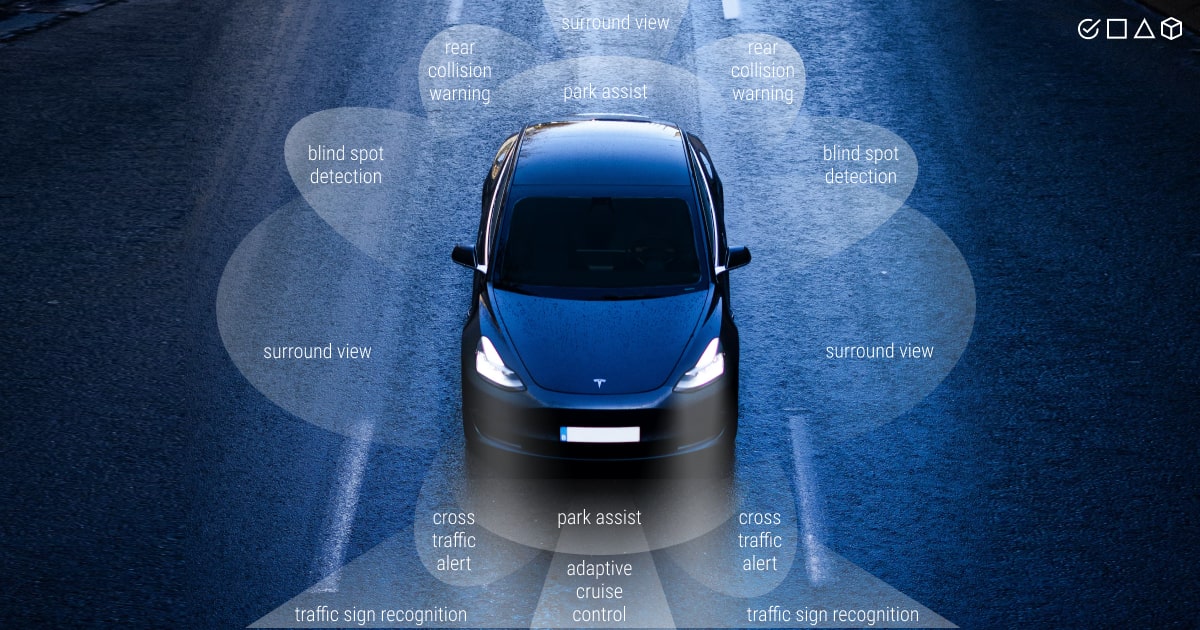
According to Statista, around 58 million autonomous vehicles would be sold globally by 2030. Basically, we have less than 10 years to figure out how to build driverless AI systems that are as accurate, ecological, safe, and secure as possible.
For safe autonomous driving, it's crucial to feed an ML algorithm with large volumes of labeled training datasets. This data usually includes images and videos of other cars, cyclists, pedestrians, animals, traffic lights, police traffic checks, construction sites, potholes, etc. Many already-existing autonomous driving platforms have focused their efforts on data annotation for machine learning, and even developed new platforms to meet the demands of software developers of self-driving cars. These are the effects of the mass amounts of training data needed for this process and the newly introduced regulations concerning the precision and accuracy of the self-driving car algorithms.
To train a driverless algorithm and process sensory input from its immediate environment, autonomous vehicle engineers require annotated data. This is similar to how a human brain would process this information, more specifically:
- Recognize objects and terrain;
- Measure distances, direction, and speed;
- Plan its own direction and speed based on environmental factors;
- Infer missing data based on acquired experience.
Now, let's go through some of the most frequently asked questions about the main problems with autonomous cars and data annotation approaches to solving them!
1. What Data Is Used for Autonomous Vehicles?
Overall, you deal with computer vision when annotating data for autonomous vehicles. So, once we solve the computer vision issues prevalent today in the tech world, we can start building self-driving systems we can truly trust.
As a rule of thumb, the driving data underpinning complex algorithms used for unmanned vehicles is produced by sensors and cameras, mounted on different parts of the car. This usually includes information from outside the vehicle and represents a complex set of network-based structures. How does this work? Cameras pick up traffic signals and road signs, while sensors keep track of the car's location with respect to other vehicles and pedestrians. Besides, autonomous driving systems harness the benefits of light detection and ranging (LiDAR) technology, radars, or sonars, including 2D and 3D data they provide.
So, if you're working on a driverless project, you need to collect data from sensors and other sources, including vehicle sensor configurations and map databases. After that, you must create a model of the world and a visual representation of the car's condition. To make this happen, there are two types of sensors: proprioceptive and exteroceptive sensors.
Proprioceptive sensors, such as Global Navigation Satellite Systems (GNSS), Inertial Measurement Units (IMUs), Inertial Navigation Systems (INS), and Encoders, are those that sense the condition of the vehicle. They are used to obtain information on the platform's position, motion, and odometry. Exteroceptive sensors track the surroundings of the vehicles to collect data on the terrain, environment, and other objects. This category includes sensors such as cameras, radar, LiDARs, and ultrasonic ones. After the data is collected from these sensors, we can move on to data annotation.
That said, before the driving data brings any real benefit to autonomous systems, it has to be properly labeled for better scene understanding and a safe driverless mode. It's useful to use datasets that include many levels of annotation, such as low-level object annotation, mid-level trajectory annotation, and high-level behavior and relationship annotation.
Data annotation is a fundamental component of autonomous driving, since it allows training the vehicles so that they can safely navigate a real-world environment and see the road similarly to humans. To ensure the safety of a self-driving vehicle, it needs to be trained on labeled data to be able to:
- Respond to objects on the road and their nature;
- Understand the difference between static and moving objects;
- Feel the speed and object's direction;
- Recognize potential threats caused by these objects.
2. What Objects Are Annotated for Autonomous Driving?
Let's see what are the main labeling attributes that guide the annotators' work on driverless projects in AI:
— Vehicle sensors
- Longitudinal acceleration;
- Engine torque and estimate;
- Steer angle and steering wheel angle;
- Engine RPM, odometer, and pedal gas;
- Throttle and brake;
- Front left and right wheel speed;
- Rear left and right wheel speed.
— Phones and dashboard cameras
- Images and GPS;
- Speed, drift, and heading angle;
- Estimated attitude: roll, pitch, yaw;
- Gyro and accelerometer metrics;
- Vertical, horizontal, and heading accuracy;
- Moving traffic.
— OpenStreetMap and online APIs
- Number of lanes;
- Road type;
- Intersection type and distance;
- Bike lanes;
- Weather index and road condition;
- Stops signs, bus stop;
- Wind speed and visibility.
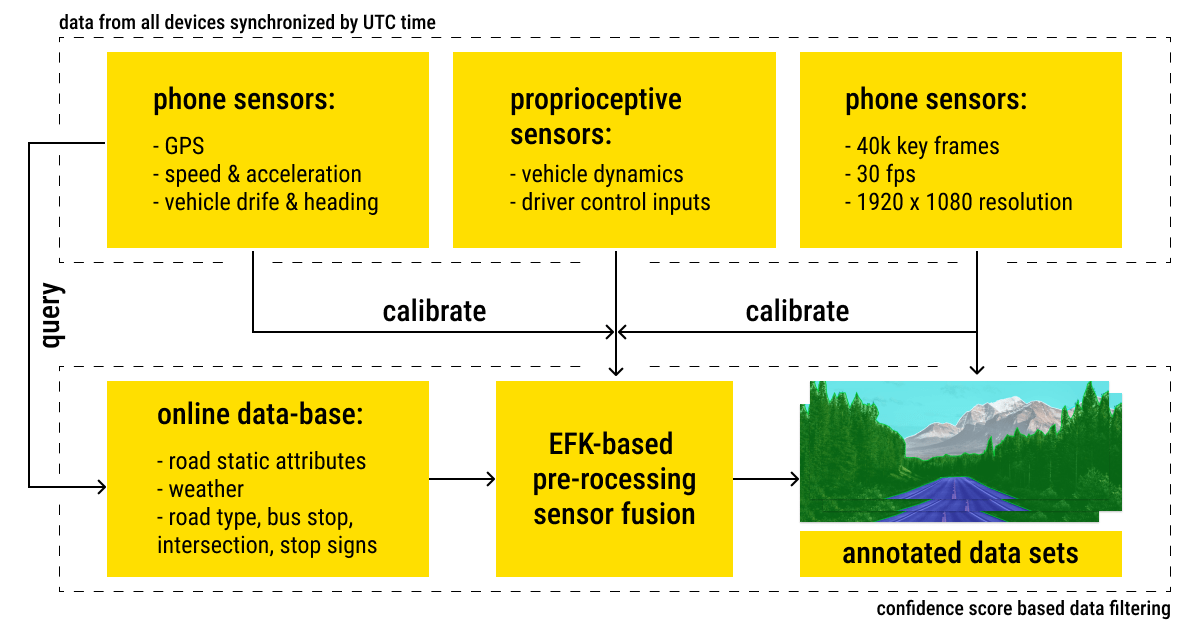
3. What Types of Data Annotation Are Used for Self-Driving Cars?
An autonomous vehicle can drive you safely on the sole condition that the ML algorithm it was trained on is as accurate as possible (i.e., trained on annotated data). For instance, our Label Your Data team spends thousands of hours providing the correct labels on image or video data, including 3D LiDAR data. This way, the sensors can recognize another moving car, a pedestrian, or lane making.
Of course, training data for autonomous vehicles doesn’t collect and label itself. Here, human intelligence plays a fundamental role in providing complete and accurate annotations for autonomous driving, a data-hungry field for sure.
Annotated datasets with images of all sorts of objects, which cars encounter on the road, constitute a vital part of the fierce competition in the automotive sector. They are used to train the driverless algorithm to detect and recognize different objects in the traffic, in all possible scenarios.
Data annotation powers supervised ML tasks, where the quality of a labeled dataset has a direct impact on the quality of the ML model itself and the final outcomes on the road. Here's the list of the major annotation types applied in autonomous driving:
- Bounding boxes. The most popular annotation method that uses rectangular boxes for object detection to identify the location of the target object.
- Polygonal segmentation. Objects come in different shapes, so there’s no single one-size-fits-all solution. When an annotator deals with a complex object, they use polygons to define the object’s shape and location as accurately as possible.
- Semantic segmentation. A pixel-wise annotation that assigns each pixel to a certain class, which is a common method when environmental context comes into play.
- 3D cuboids. As the name suggests, this type of data labeling is used to provide a 3D representation of the object, a crucial measure to define volume and object position in a 3D space and obtain spatial-visual models.
- Key-point and landmark. A data annotation technique used to define small objects and shape variations by using multiple consecutive points to outline the object on the image or video.
You might be wondering what’s the further use of labeled data and how exactly these algorithms help a vehicle become driverless. Here are the main tasks that the ML algorithms (fed with annotated datasets) perform in autonomous driving:
- Object detection: Precise object detection in real-world scenarios can be carried out by complex deep learning algorithms. Such algorithms usually undergo two phases: conventional object detection and DL-supported object detection.
- Lane detection and marking: Sophisticated self-driving systems require robust and accurate lane detection. This helps autonomous vehicles drive safely within the road lanes and avoid collisions. Additionally, this ML task ensures efficient trajectory planning and lane departure. The algorithms rely on color-based features, the structure tensor, the bar filter, and ridge features. However, the effectiveness of lane detection is often undermined by road scene variations.
- Localization and mapping: The accuracy of localization and mapping has a strong impact on the safety and visibility of path planning of a self-driving vehicle. This is especially true for urban autonomous driving. Here, multi-layer high-definition (HD) maps are used for path planning and are classified as follows: landmark-based, cloud-based, and vision-based. In addition, DL methods are used for feature extraction, motion estimation, and long-term localization.
- Prediction and planning: Prediction models are crucial for analyzing the driving behaviors of the nearby cars and pedestrians for risk assessment, including unsafe lane changes. Planning is locating possible routes on a map that connect points of origin to points of destination, much as how GPS navigators assist in planning a feasible global route. There are three categories of local planners: curve interpolators, graph-based planners, and sampling-based planners.
- Vehicle control: Drive-by-wire and autonomous driving computing systems are linked through vehicle control. This work aids the machine in maintaining the required speed as it follows the trajectory of the planning module. Usually, two controllers, a lateral controller and a longitudinal controller, are used to operate a vehicle.
- Sensor fusion: Researchers can experiment with perception techniques like sensor fusion if the data from the sensors is available within the simulation environment. To understand ambient circumstances for detection confidence, sensor fusion processes inputs from radars, LiDAR, cameras, and ultrasonic sensors together.
Data annotation, in its most basic form, is the process of labeling or classifying objects that have been recorded by an autonomous vehicle in a frame. This annotated data (manually or automatically) is further selected to feed ML and DL models. Therefore, data labeling is a crucial technique to assist self-driving cars in recognizing patterns in data and properly categorizing them to make the correct and safe decisions on the road. To obtain the best data, it's also important to use the proper type of annotation.
4. Why Do You Need Data Labeling for Autonomous Cars?
As you can see, advances in self-driving car technology heavily rely on data annotation. A self-driving car must be trained to recognize various road signs, including colors, shapes, lights, and electronic messages conveyed by these signs. Understanding these elements ensure safe pilotless movement on the road. But how do cars manage to recognize all these complex signs and objects? They do so by training on a labeled dataset.
AI systems that power autonomous cars need to learn how to distinguish between all these variables to produce smart, safe, and accurate technology in the end. In this case, AI learns from an immense amount of data. And as in any other case with AI-driven technologies, they require this data to be labeled. This way, annotation helps autonomous cars better identify different road conditions and unanticipated obstacles.
Moreover, in machine learning, data is usually labeled manually, which involves a considerable effort from human annotators, time, annotating tools, and other resources. However, such methods are not always error-proof and can be biased. So, sometimes, automated annotation performed by AI can be faster, cost-efficient, and even more accurate, but it always requires human control for the most optimal results.
To put it briefly, data annotation provides the ability for the vehicles to comprehend diverse driving environments as similar to humans as possible. The modular pipeline approach is one of the most widely used autonomous driving frameworks. It combines expensive LIDAR, a highly precise global navigation satellite system (GNSS), and 3D high-definition maps to reconstruct a consistent representation of the surrounding environments.
At the same time, vehicle data collection and annotation methods applied in autonomous driving have raised several concerns. Among the most critical ones are the scalability of this process in a diverse environment and the shift towards more automated options to reduce the burden on human annotators.
As of right now, the majority of autonomous cars use pricey, high-quality sensor systems, such as LiDAR and HD maps with detailed annotations. The quality of the data and labeling accuracy are two main problems with labeling in the automotive sector. Additionally, the annotated label must be highly accurate to prevent adding bias to its use in supervised learning in the future.
How Label Your Data Handles Autonomous Vehicle Data
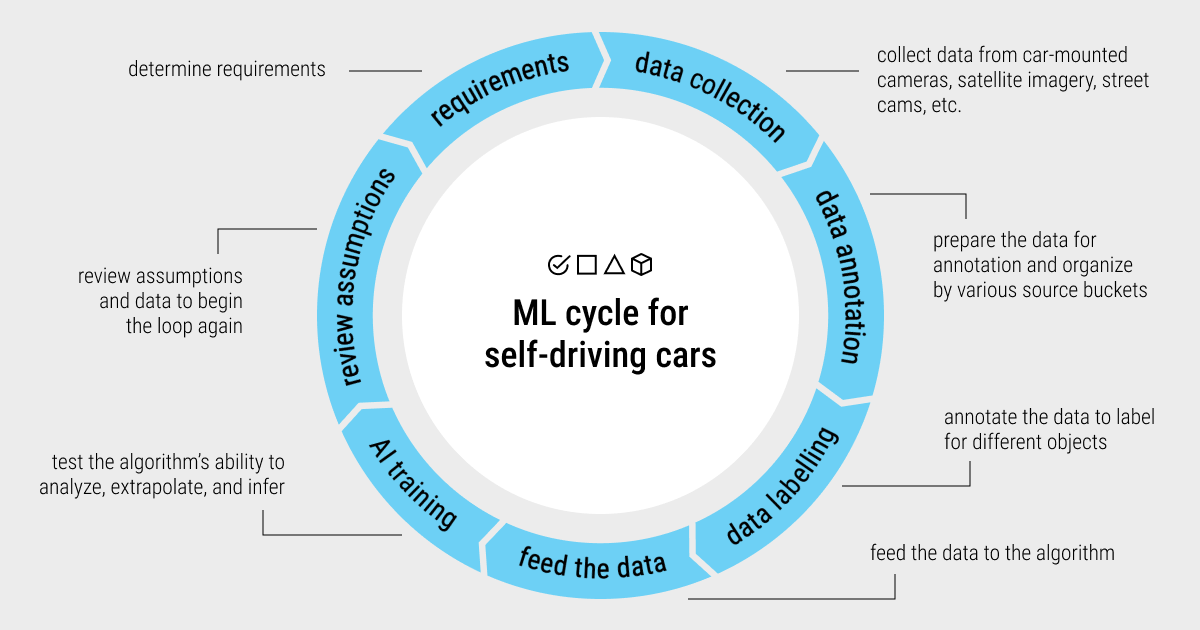
Two years ago, our Label Your Data team embarked on a journey with the true driverless fans of Formula Student — our partners from Germany, Elefant Racing. You can read a short overview of our successful collaboration, or skip to the full case study that we have recently published and know more about all the intricacies of pilotless racing!
The trust between our crews was quickly established, and the tests began in the spring of 2021 and continued until the launch in late June. It was a turning point for both teams when the public first saw FR20 Ragnarök in action. By that point, our annotators had put in about 2000 hours on labeling tasks using bounding boxes and keypoint annotation of traffic cones. This year, our annotators work on the new driverless race car by the Elefant team, named Thor. The long-awaited results and new achievements are on the way.
We also worked with semantic segmentation for a client that does the long-range 3D vision for autonomous vehicles. Our team annotated very small objects and obstacles for the cars on the roads. And just to remind you, the annotators at Label Your Data are well-versed in working with 3D LiDAR data, since we have already labeled many scans from LiDAR technology for remote-sensing systems.
Aside from autonomous cars, Label Your Data provides a variety of data annotation services, so you can find something for your unique AI project! Send your data to us and receive a free pilot project to make sure your data is in safe and professional hands!
Concluding Thoughts on AI-Assisted Driving and The Value of Data
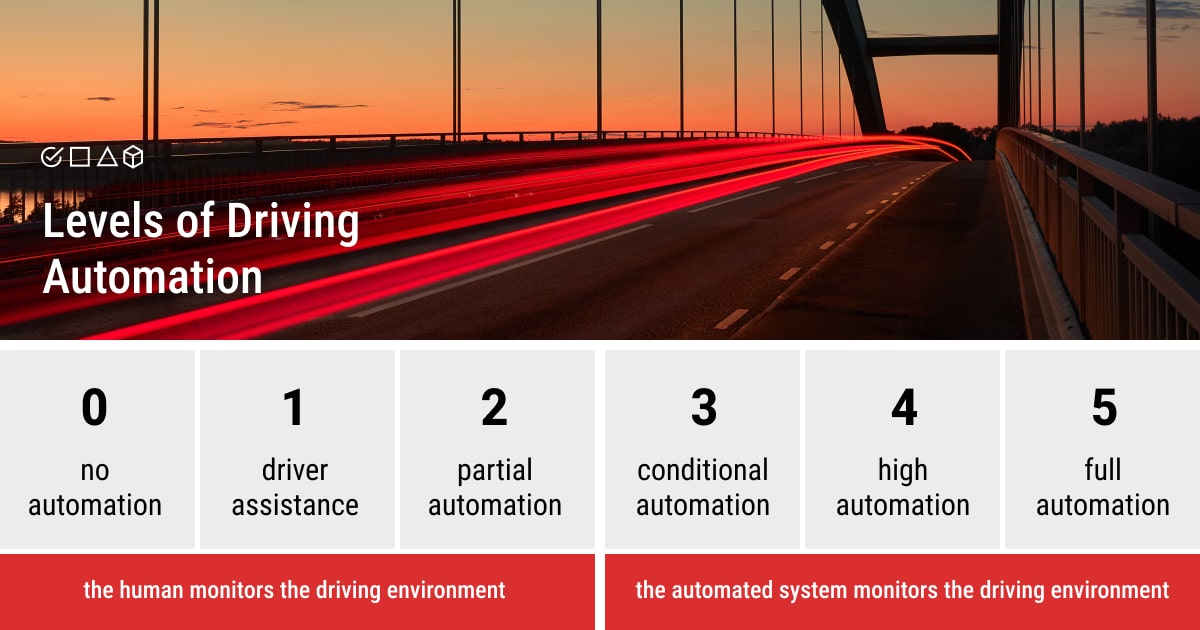
You can never go wrong with the main principle in machine learning: garbage in, garbage out. Poor annotation practices in autonomous driving lead to hazardous outcomes on the road. As such, expert data annotation is one of the most pivotal tasks of machine learning for AI-powered self-driving cars.
What should be kept in mind about autonomous driving is that for such a vehicle to navigate safely in the surrounding environment, it must learn the nature of the objects it encounters on the road in-depth. Using data annotation is one of the proven methods to do this. Thus, labeling individual objects, the differences between them, and the dynamic changes on the road, is deeply embedded in the process of training an algorithm when autonomous cars teach themselves to drive (yet, safely).
Data labeling specialists mostly work manually, but they also use software tools to semi-automate their work, as we do at Label Your Data. No one said AI-assisted driving would be easy, but annotators are always here to help!
Written by
One of the technical writers at Label Your Data, Yuliia has been gradually delving into the intricate aspects of AI. With her strong passion for the written word and technical expertise, Yuliia has developed a keen interest in the evolving field of data annotation and the power of machine learning in today's tech-savvy world. Check out her articles to learn more about the complex world of technology and find the solutions that work best for your AI project!