Machine Learning Algorithm: When to Use Which One
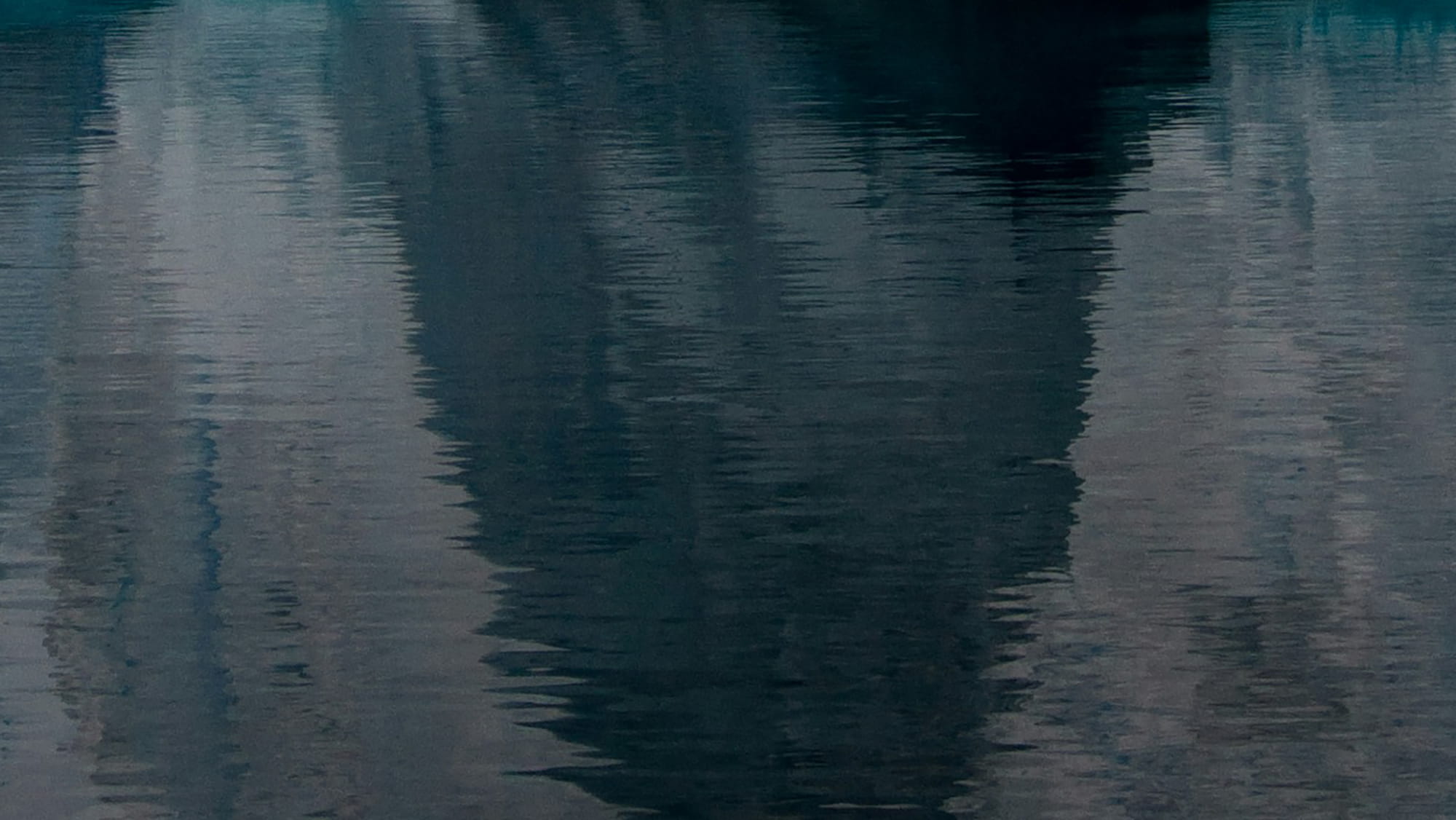
TL;DR
The variety of tasks that machine learning can help you with may be overwhelming. Despite this, the majority of tasks can be solved using a limited number of ML algorithms. Still, you need to know, which of them to choose, when to use them, what parameters to take into consideration, and how to test the ML algorithms. We’ve composed this guide to help you with this specific problem in a pragmatic and easy way.
What Is a Machine Learning Algorithm?
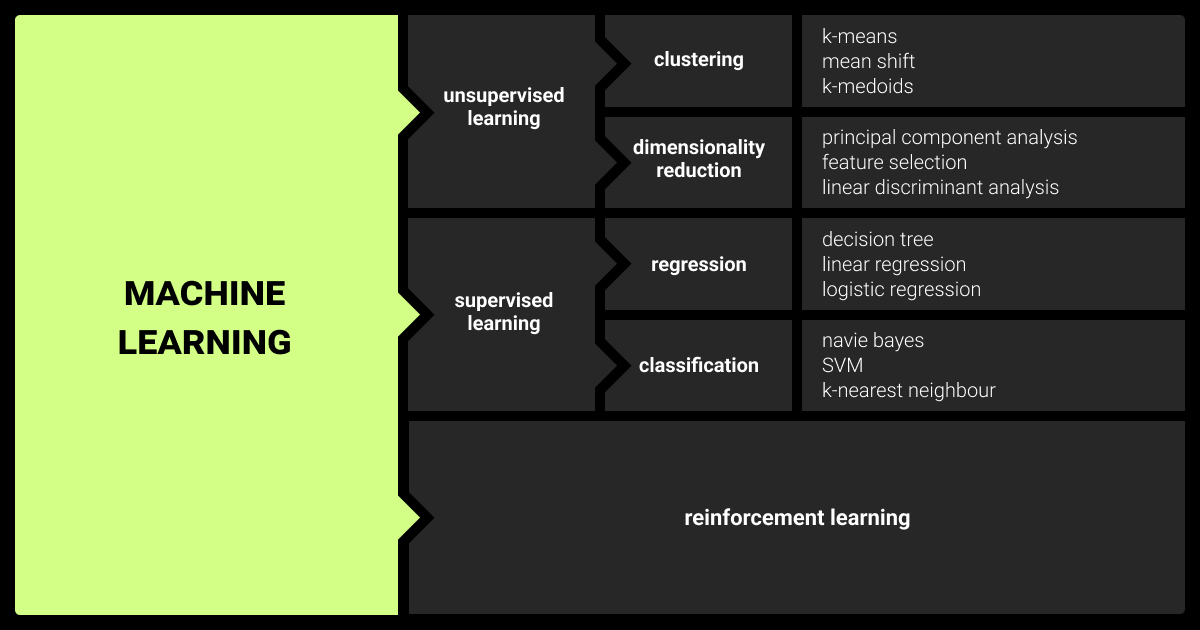
The variety of tasks that machine learning can help you with may be overwhelming. Despite this, the majority of tasks can be solved using a limited number of ML algorithms.
Still, you need to know, which of them to choose, when to use them, what parameters to take into consideration, and how to test the ML algorithms. We’ve composed this guide to help you with this specific problem in a pragmatic and easy way. Let’s start with the basics in case you’re still a bit in the dark about what this all is and why you might need it.
Machine learning is an algorithm-based method for analyzing data with the goal of looking for patterns and making accurate predictions. As the name suggests, ML algorithms are basically computers trained in different ways. These ways are the types of ML algorithms that fall into three and a half broad categories (we’ll explain the “and a half” part a bit later, be patient).
Humanity creates more and more data every day. It comes from a variety of sources: business data, personal social media activity, sensors of IoT, etc. Machine learning algorithms are used to take this data and turn it into something useful that can serve to automate processes, personalize experiences, and make complex predictions that human brains cannot do on their own.
Given the variety of tasks that ML algorithms solve, each type specializes in certain tasks, taking into consideration the features of the data that you have and the requirements of your project. Let’s take a look at each of the major types of ML algorithms and certain examples used for the most common tasks.
Types of Machine Learning Algorithms
There are three major types of algorithms in machine learning: unsupervised, supervised, and reinforcement. An additional one (that we previously counted as “and a half”) is semi-supervised and comes from the combination of supervised and unsupervised. We’ll talk about the unique features and examples of each of these types.
Unsupervised Types of ML Algorithms
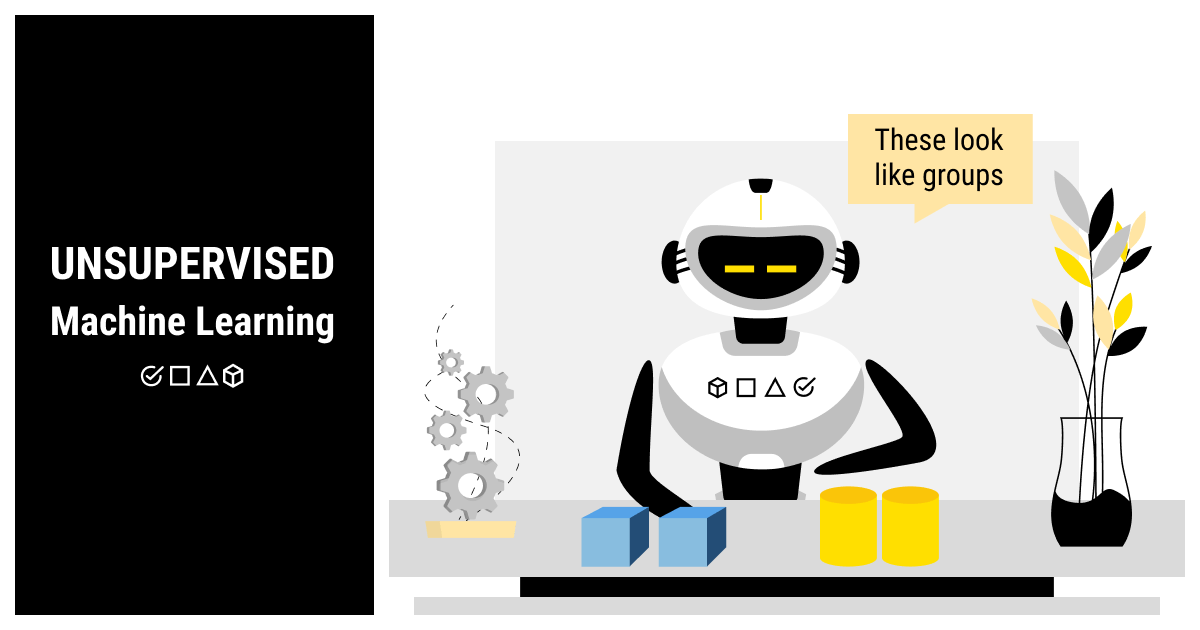
This type of machine learning algorithm arguably represents artificial intelligence in its true form. Unsupervised ML is based on the idea that a machine can learn without any guidance from humans. For learning, it uses unlabeled data, which is basically raw data that can be found “in the wild” and is usually unstructured and unprocessed.
Naturally, unsupervised machine learning algorithms have a lot of limitations. As they don’t have any starting point for their training, there are only a few types of tasks that they can perform. The two major ones that we’ll highlight are clustering and dimensionality reduction.
Clustering
While a clustering algorithm won’t be able to tell if you show it the photo of a cat, it can definitely learn to tell a cat from a tree. This means that your computer can tell two different things apart based on their naturally different features and put them into separate groups (clusters). At the same time, it won’t be able to tell you what type of object is in each cluster.
Clustering is great for solving tasks such as spam filtering, fraud detection, primary personalization for marketing, hierarchical clustering for document analysis, etc.
Dimensionality Reduction
Look for dimensionality reduction algorithms in projects that deal with the data that has lots of features and/or variables. The major idea behind this type of algorithm is processing and simplification of the data by decreasing the number of features. The dimensionality reduction model reduces the features that are not essential for the task at hand but leaves the structure and main features of the data intact.
Noise reduction and data visualization are common tasks for dimensionality reduction algorithms. It is also commonly used as an intermediate step in more complex ML projects.
Supervised Types of ML Algorithms
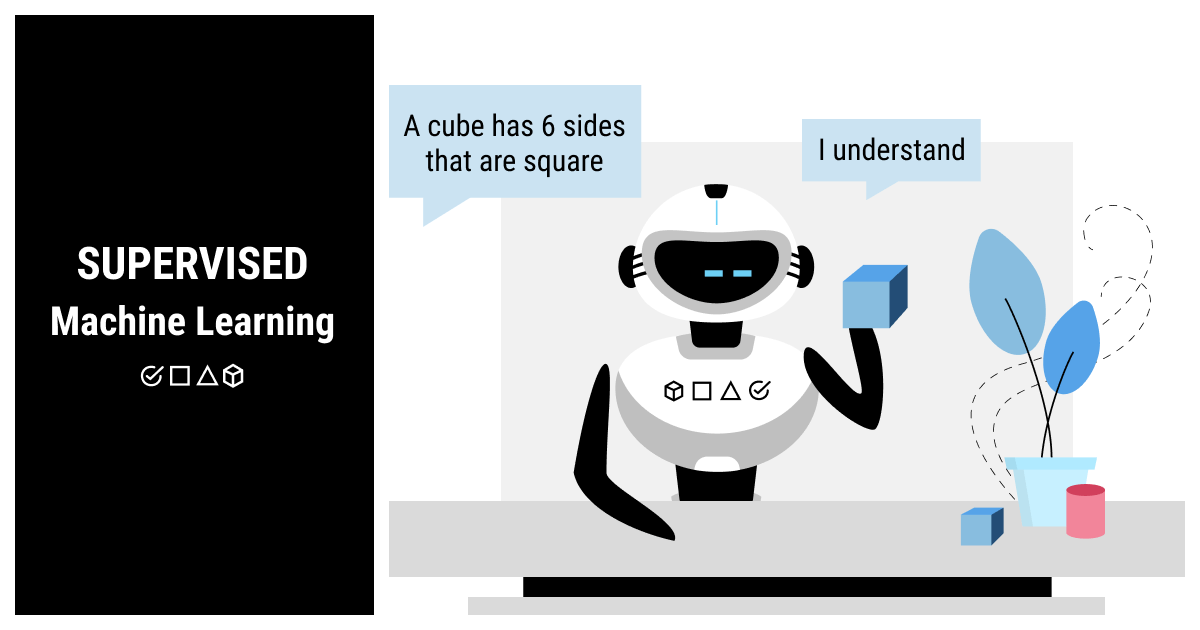
This is arguably the largest and most popular group of machine learning algorithms. And no wonder: supervised learning is flexible, comprehensive, and covers a lot of the common ML tasks that are in high demand today.
In opposition to unsupervised learning, supervised algorithms require labeled data. This means that the models train based on the data that has been processed (cleaned, randomized, and structured) and annotated. The processing and annotation of the data is supervision that a human has over the training process (hence the name of supervised learning).
Data annotation is an essential process for building a supervised ML algorithm. In a nutshell, it requires adding labels or tags to the pieces of data, which will tell the algorithm how to make sense of it. It’s quite a time-consuming and labor-intensive process that usually gets outsourced to save time for the core business tasks.
There are quite a few interesting algorithm types in supervised learning. For the purposes of brevity, we’ll discuss regression, classification, and forecasting.
Regression
It’s a common case that analysis is required for continuous values to find a correlation between different variables. Regression helps to look for this correlation and predict an output.
This type of supervised algorithm is commonly used to predict the prices or value of certain objects based on a set of their features. Thus, a house will be evaluated based on its location, the number of bedrooms, and if anyone died in it ;)
Classification
Similar to clustering that we’ve already seen in unsupervised machine learning algorithms, classification allows training the AI to group different objects (values) into categories (or classes). The difference is that, now, the machine knows which class contains which objects. If, after training, you show the computer a photo of a cat and ask what it is, it will tell you it’s a cat and not just group it with other cat photos. This capability is essential for AI image recognition tasks, where accurately identifying and classifying objects in images is crucial.
Unlike regression, classification is based on a limited number of values. It can be binary (when there are only two classes, e.g., cats or dogs) or multi-class (when there are more than two categories to classify the values).
Forecasting
When you have past and present data, it’s natural that you’d want to predict the future at some point. Forecasting algorithms can help you with this task as they are able to analyze the data in-depth, looking for hidden patterns, and make predictions based on this analysis.
The trends analysis is obviously the forte of this type of machine learning algorithm. That’s why forecasting is commonly used in business and finance.
Semi-Supervised Types of Algorithms in Machine Learning
Supervised and unsupervised machine learning algorithms are very common for the majority of AI tasks today. Here’s a simple cheat sheet to facilitate your choice of a machine learning algorithm:
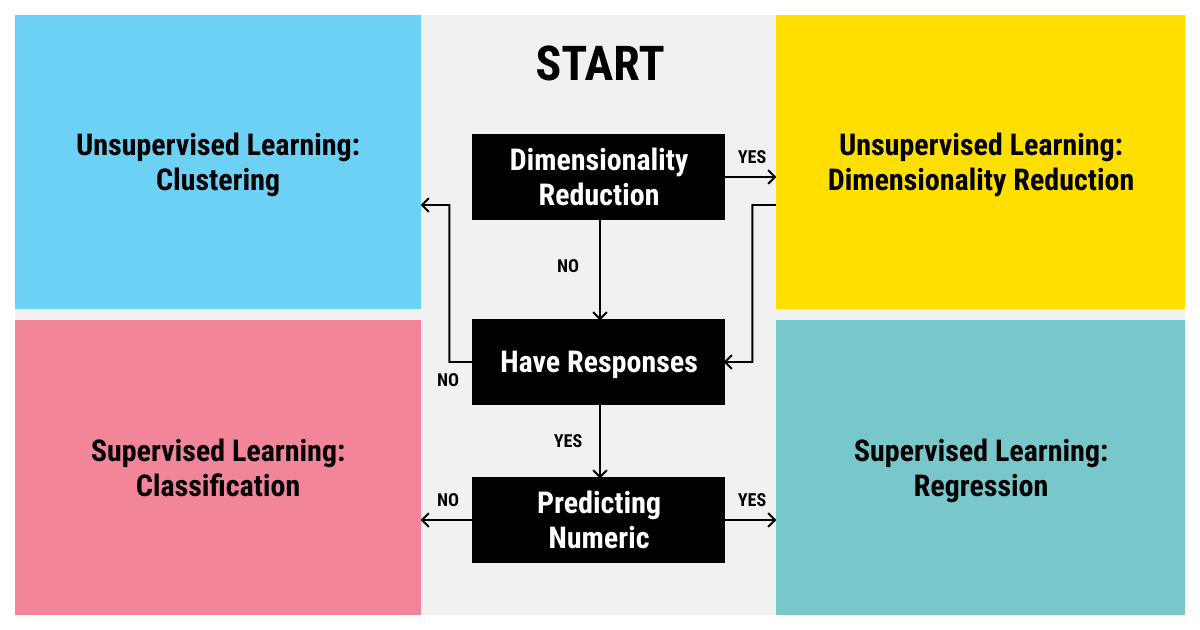
However, sometimes you cannot choose between either an unsupervised or a supervised ML algorithm. There are cases where combining the two algorithms can bring you more benefits even with regard to the growing complexity of your ML model. That’s because of the core features of each type of algorithm: unsupervised learning brings in simplicity and efficiency while supervised learning is all about flexibility and comprehensive goals.
When you combine two different types of algorithms, you get semi-supervised learning. This type of ML algorithm allows you to significantly cut down the financial, human, and time cost for annotating the data. At the same time, semi-supervised learning algorithms are not as restricted in the choice of tasks as supervised learning algorithms.
Reinforcement ML Algorithms
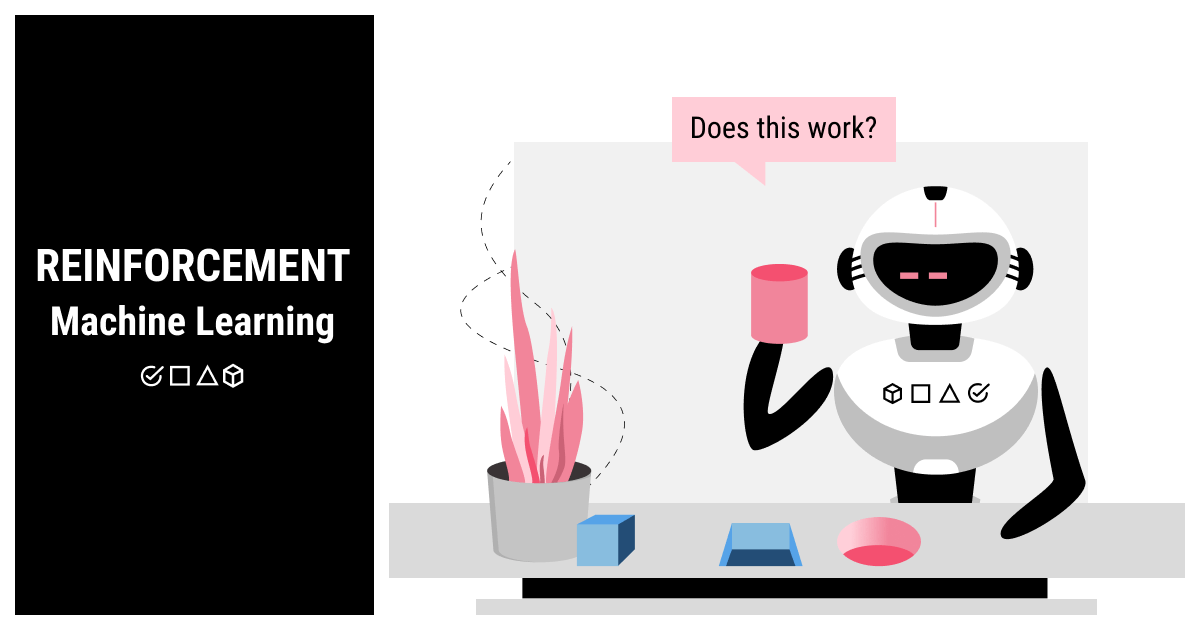
And now for something completely different. Unsupervised and supervised algorithms both work with the data, either unlabeled or labeled. A reinforcement algorithm trains within an environment with a set of rules and a defined goal.
Reinforcement learning algorithms are usually based on dynamic programming techniques. The idea behind this type of ML algorithm is balancing exploration and exploitation. There is some uncharted territory that an algorithm can explore but every action will be followed by a response from a system, either positive or negative. Training on these responses, the algorithm will learn to choose the best set of actions to achieve the set goal.
A classic reinforcement learning application is games such as chess or Go. Learning to play (and win) these games requires the algorithm to understand the environment (the board, the set of rules, and the actions that can be either punished (by the other player taking the pieces) or rewarded (by winning the opponent’s pieces). A more modern and fascinating example of a reinforcement algorithm is training autonomous vehicles. The algorithm is required to navigate the environment without hitting anything and obeying the traffic rules.
How to Choose Machine Learning Algorithm
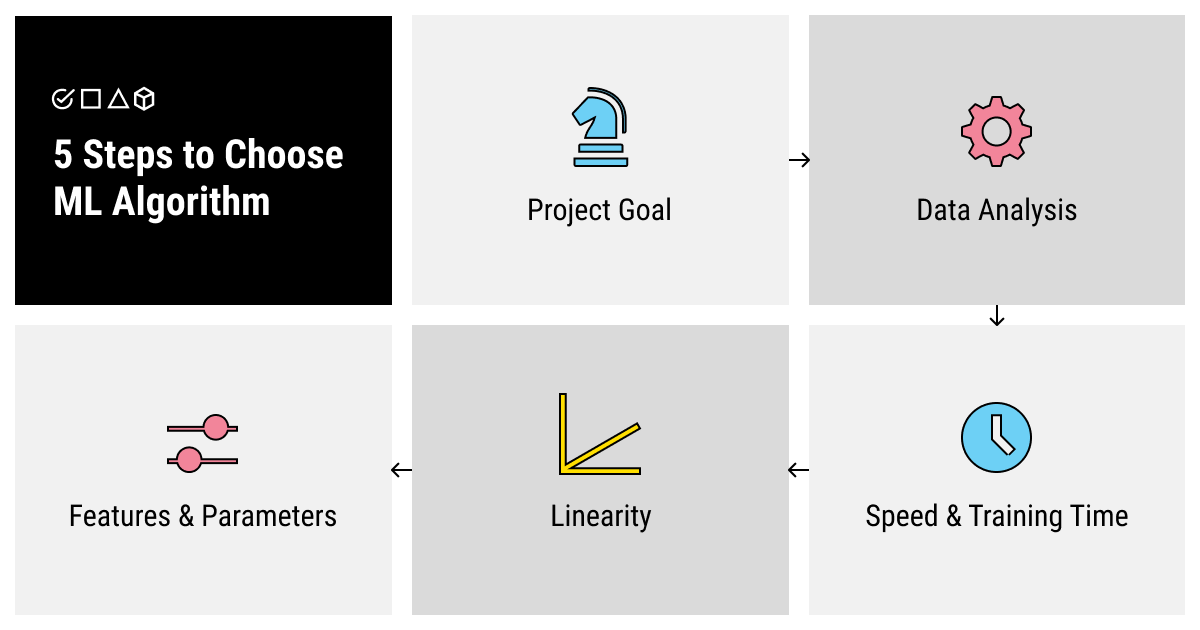
Learning about the different types of machine learning algorithms is not enough to understand how to choose the one that fits your specific purpose. So let’s stick to an incremental method and see how exactly you can approach this problem.
Step 1. Understand Your Project Goal
As it has already become apparent, each machine learning algorithm was designed to solve a specific problem. So, first of all, you should consider the type of project that you’re dealing with.
To determine the right algorithm, start by asking whether the problem involves labeled data (supervised learning) or unlabeled data (unsupervised learning). If you have labeled data, further decide if the task is predicting continuous numeric values (regression) or discrete categories (classification). On the other hand, if the data is unlabeled, clustering techniques may be used to group similar data points. Additionally, consider whether dimensionality reduction is needed to simplify the data before applying other algorithms.
Answer this question: what kind of an output do you need? Do you need an algorithm for prediction based on previous data? Turn to supervised forecasting algorithms, such as regression for numeric predictions or classification for categorical outcomes. Are you looking for an image recognition model that will work with poor-quality photos? Dimensionality reduction in combination with classification will help you with it. Do you need to teach your model to play a new game? A reinforcement algorithm will be your best bet.
Step 2. Analyze Your Data by Size, Processing, and Annotation Required
When you’ve answered the question of what type of output you need, ask yourself what input do you have. What is your data like? Is it raw, just collected from wherever, and requires processing? Is it biased, dirty, and unstructured? Or do you already have a big annotated dataset on your hands? Do you have enough data or is additional collecting (or even collecting from scratch) required? Do you need to spend time preparing your data for the training process or are you good to go?
If your data lacks structure or labels, unsupervised learning techniques like clustering may be more suitable. Alternatively, if you have a well-prepared and annotated dataset, supervised learning approaches will help achieve more accurate predictions. Insufficient, poor-quality, unprocessed data usually doesn’t lend itself to great training of a supervised algorithm. You should decide if you want to spend time and resources on preparing the best data you can before starting the training process. If not, you can opt for unsupervised algorithms but keep in mind the limitations of such a choice.
Step 3. Evaluate the Speed and Training Time
Here’s another question for you to answer that can help you understand what type of machine learning algorithm you need. Do you need it fast even if it means lower quality of training (and, respectively, predictions)? More and higher-quality data lead to better training. Can you allocate the required time for proper training?
Step 4. Find Out the Linearity of Your Data
Another important question is what the environment of your problem is like? Linear algorithms (such as linear regression or support vector machines) are simpler and faster to train. However, they are not usually used for more complex problems as they deal with linear data. If the data is multifaceted, multidimensional, and has many intersecting correlations, linear algorithms might not be sufficient for your task.
Step 5. Decide on the Number of Features and Parameters
Finally, how complex and accurate your final AI model should be? Don’t forget that longer training usually leads to better, more accurate performance when the AI model is deployed. If you’re working with high-dimensional data, consider dimensionality reduction to simplify the problem and speed up the training process. You can specify more features and parameters for your model to interpret if you have time to let it train longer. So giving your algorithm more time to learn may be a good investment into your future output accuracy and interpretability.
Top Machine Learning Algorithms and Use Cases
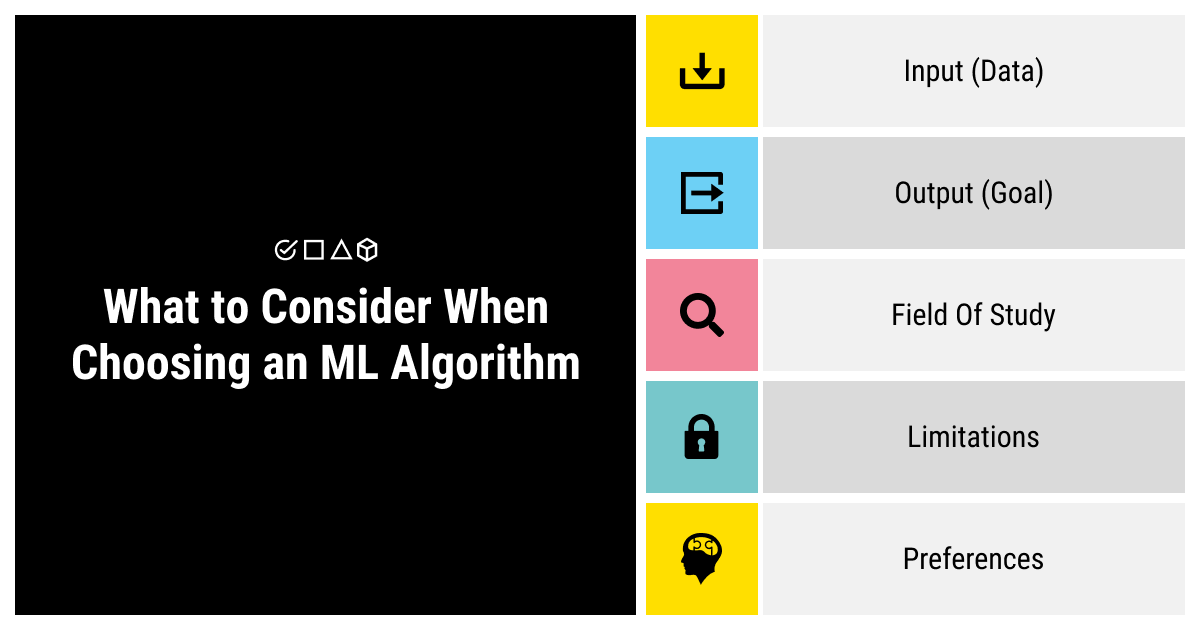
For ML practitioners and data scientists, selecting the right algorithm is crucial for optimizing model performance and efficiency. This table offers a detailed overview of essential ML algorithms to enhance your ML workflows.
When to Use Which Algorithm in Machine Learning
About Label Your Data
If you choose to delegate data annotation, run a free data pilot with Label Your Data. Our outsourcing strategy has helped many companies scale their ML projects. Here’s why:
No Commitment
Check our performance based on a free trial
Flexible Pricing
Pay per labeled object or per annotation hour
Tool-Agnostic
Working with every annotation tool, even your custom tools
Data Compliance
Work with a data-certified vendor: PCI DSS Level 1, ISO:2700, GDPR, CCPA
FAQ
What is the machine learning algorithm?
A machine learning algorithm is a set of rules that helps a computer learn from data. It finds patterns and makes decisions without needing direct programming. Examples include decision trees, neural networks, and support vector machines.
What are the 4 types of machine learning algorithms?
There are four types of machine learning. Supervised learning uses labeled data to make predictions. Unsupervised learning finds patterns in data without labels. Semi-supervised learning uses both labeled and unlabeled data. Reinforcement learning learns by trial and error to maximize rewards.
What are the 5 popular algorithms of machine learning?
Linear regression predicts numbers by drawing a straight line through data. Logistic regression helps sort things into two categories. Decision trees make choices using a step-by-step approach. Random forests combine many decision trees for better accuracy. Neural networks work like the human brain to recognize patterns.
What is the simplest machine learning algorithm?
Linear regression is the simplest algorithm. It predicts values by drawing a straight line through data points. It is easy to use and great for basic predictions.
When to use which machine learning algorithm?
The right algorithm depends on the problem you’re solving. If you need to predict numbers, like house prices, linear regression is a good choice. For yes-or-no decisions, such as detecting spam, logistic regression works well.
When the task involves making structured decisions, decision trees provide a clear, step-by-step approach. Random forests improve accuracy when dealing with complex data. Neural networks are ideal for recognizing patterns in images, speech, or text.
Written by
Karyna is the CEO of Label Your Data, a company specializing in data labeling solutions for machine learning projects. With a strong background in machine learning, she frequently collaborates with editors to share her expertise through articles, whitepapers, and presentations.