- The Role of Artificial Intelligence in Drug Discovery of the Future
- Top 5 Use Cases of AI for Drug Discovery
- Challenges and Solutions of AI-Based Drug Discovery
- What AI in Drug Discovery Will Bring in 2024?
- Final Thoughts
- FAQ
In recent years, AI and drug discovery have already shown exceptional success. Case in point, the pharmaceutical company Insilico Medicine has successfully passed the Phase 1 trials of the AI-designed drug candidate in only 30 months. The results are extraordinary, since usually the drug discovery phase can last from 4 to 7 years. Another example showed Takeda Pharmaceutical Co., which invested $4 billion in an AI-selected drug compound for psoriasis. Instead of years of human work, the compound was selected by AI in only 6 months.
The pharma giants have high expectations that AI in healthcare will speed up the process of drug discovery. Taking into account all the hassle around technologies, can AI be a real game-changer in a very long and expensive process of bringing new treatments to human diseases? Let’s explore.
The Role of Artificial Intelligence in Drug Discovery of the Future
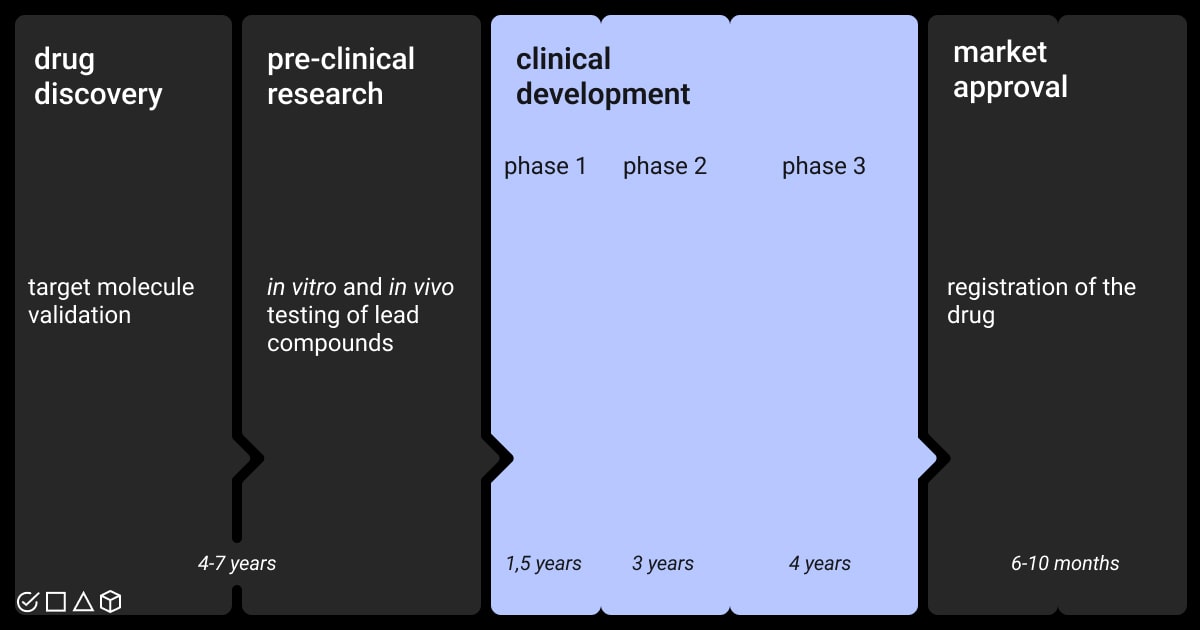
The whole process of drug development can last more than 10 years and follows 4 to 5 steps:
Pre-discovery/drug discovery stage. This stage involves research on target mechanisms (usually proteins or genes) that cause the disease. Further, the scientists search for molecules or components that could cure the disease or relieve its symptoms. Without AI application, this stage can last up to 4 years.
Pre-clinical research. At this stage, the scientists define the mode of the components’ action, their efficiency on in vitro and in vivo models, and their potential toxicity. From the drug discovery stage to the end of the pre-clinical research, the work can last up to 7 years.
Clinical development. This phase consists of three steps, which involve all the regulatory approvals, testing on voluntary individuals, and confirmation of drug efficacy. Even if you use artificial intelligence in drug discovery and development, testing on candidates with diseases is a critical and the most important step.
Market approval. If the previous phases are successful, the drug is then registered and seeks market approvals from local authorities (such as Marketing Authorization Application in the EU or New Drug Application/ Biologics License Application in the US).
The artificial intelligence in drug discovery spreads along all stages of drug development, but significantly saves time on the discovery and pre-clinical research. Let’s take a look at the current application of AI in drug discovery, and how exactly it helps to change the course of the process.
Top 5 Use Cases of AI for Drug Discovery
AI’s role in drug discovery only continues to evolve, and this year will bring even more evidence. AI has a great potential, but for the moment it helps with such elements of drug development:
Disease target identification. AI-driven drug discovery consists of analyzing enormous biological datasets to find potential drug targets. They include the information on genomics, proteomics, and other elements that take part in the disease.
Drug optimization and design. Artificial intelligence for drug discovery helps in analyzing molecule structures, predicting their connection with the targets. This further influences component efficiency and side effects.
Generation of new data on molecules. After defining the molecule properties, AI-based drug discovery helps with optimization. With all the annotated properties, it creates suggestions on physical and chemical features for all similar and new compounds.
Screening accuracy. AI plays an important role in analyzing screening data for efficient and promising compounds. The algorithms and the overall screening process speed up with AI.
If we talk about the whole drug discovery process and not only the first stage, AI also touches such areas as predicting toxicity of drug components and outcomes of clinical trials. It contributes to confirming drug safety and assessing risks and side effects.
To make this usage of AI in drug discovery efficient and successful, you need the right data to start with. In such cases, data collection services may come in handy if you need to get the best quality data for your AI project in healthcare.
If you already have collected data on hand and seek professional assistance with data annotation, our team can help you out. Send us your request!
Challenges and Solutions of AI-Based Drug Discovery
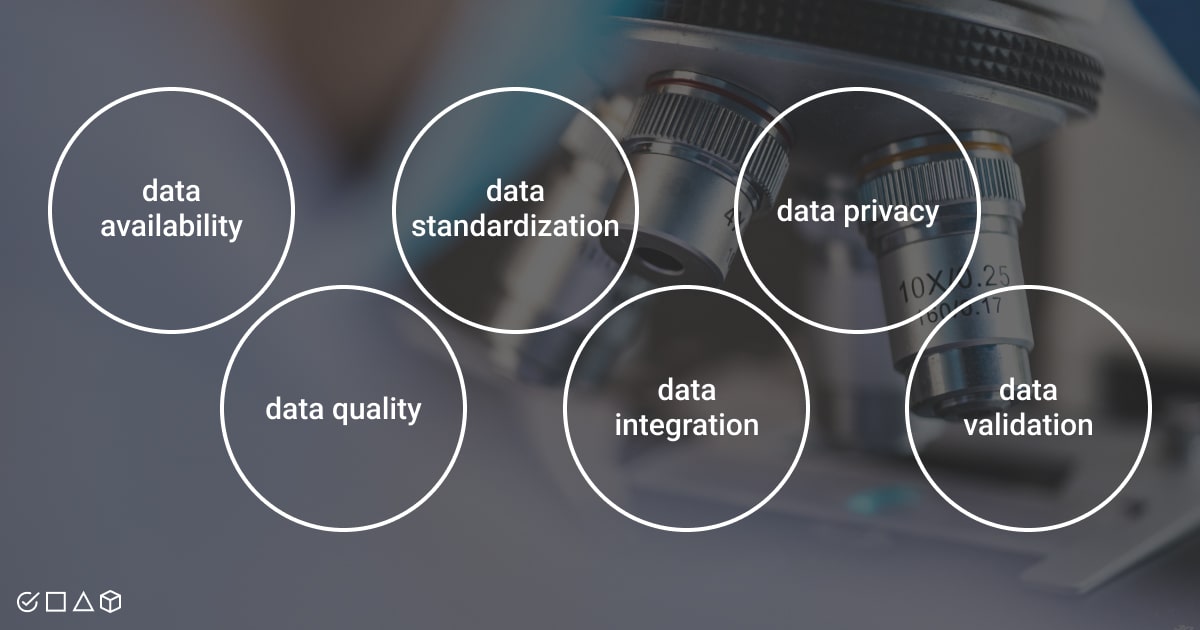
AI in drug discovery can solve multiple problems. The most critical ones are the long development process and high development costs. Others include cumbersome target identification, difficult understanding of the disease’s biology, and development of highly resistant long-term components.
But, even if AI and drug discovery work hand in hand, there are many areas in the process where AI is difficult to apply. The main pain points arise in data-related stages, be it data collection, image annotation services, text labeling, etc.
Challenges of AI and Drug Discovery
The implementation of AI into the drug discovery process happens with the help of tools built on deep learning algorithms. Today, deep learning in drug discovery is one of the most popular applications of AI. However, data in drug discovery, which is the most crucial element for deep learning, is often difficult to collect or read, is not standardized, and causes many biases.
Here are the main challenges we face while implementing an AI-enabled drug discovery:
Data availability and quality. AI for drug discovery requires massive amounts of data for training and supervised learning. Frequently, the datasets in the researchers’ possession are not always available and of low quality.
Data standardization. To make the AI tool effective, the data needs to be in the same format. The tasks for the tool are thus difficult to formulate since the data comes in different formats.
Data annotation. Data annotation services in healthcare help with reducing disparity among data labels, contributing to the formulation of successful tasks. This area is often considered a significant gap, which leads to unsuccessful final results.
Integration into traditional methods. Since AI is integrated only in parts of the drug discovery process, it meets resistance from traditional research methods. Working with both computation and experimental methods slows down the process.
Data privacy. The access to the patient’s information is very sensitive and can often be restricted. This slows down the process of building accurate datasets.
Validation to real-world scenarios. All AI suggestions must be validated. They sometimes offer impossible combinations that are far from real-life applications.
Costs. Healthcare and drug discovery are some of the riskiest areas for investment. Even if the first phase is successful, very few components of the new drug come till the end. The costs for the whole process remain too high.
To find the best solutions for effective deep learning training, contact our annotation professionals by sending your request. We will ensure the accuracy and standardization of your data.
Solutions That AI in Drug Discovery Offers
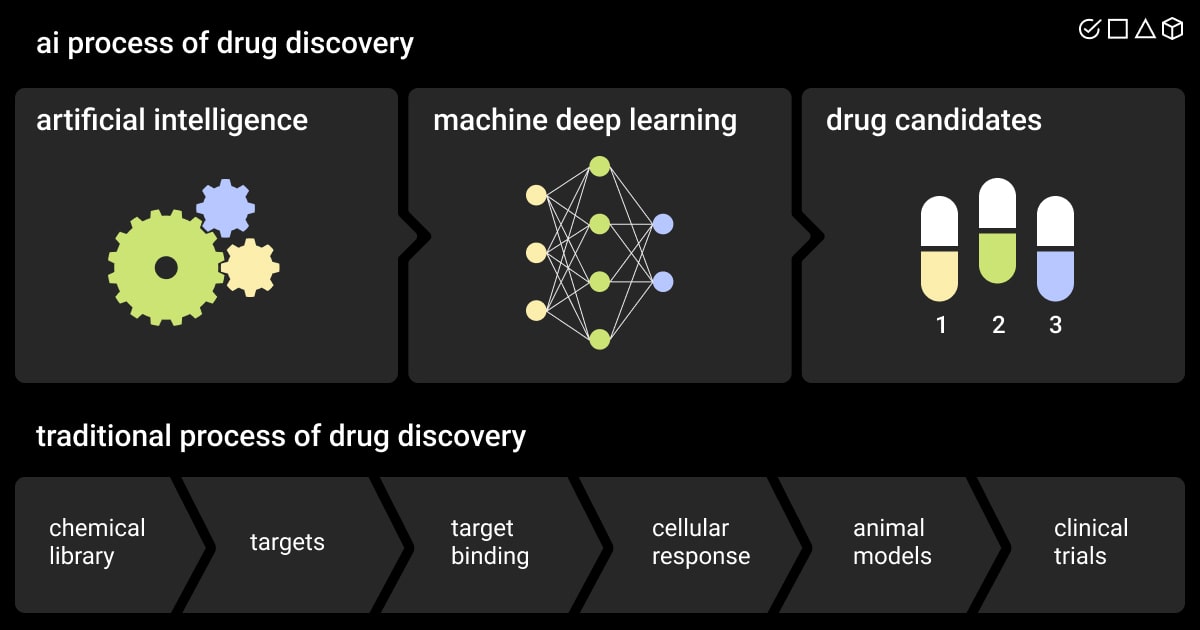
Modern drug discovery landscape is considered so promising with the application of AI because we have already seen multiple successful cases. In addition to saving time and costs, AI solutions in different industries continue to grow.
AI was already used in analysis that identified dual-purpose target candidates for aging and the curation of cancer. Another international group of scientists used AI for drug discovery, working on COVID-19 variants. EVEscape tool based on AI helps to develop new vaccines and therapies for the new variants of SARS-CoV-2.
Apart from ongoing projects that have already shown extraordinary results, the usage of AI brought significant benefits in the data analysis methods in drug discovery. Here is the list of the most widespread benefits from the application of AI:
Automation of manual tasks and acceleration of drug discovery process.
Prediction of toxicity, which helps to prioritize the efficient drug candidates.
Analysis of existing datasets significantly contributes to the repurposing of the existing drugs for other diseases.
Acceleration of screening time.
Optimization of clinical trials thanks to analysis of historical data and prediction of outcomes.
The key to the success of AI in such a complex industry remains data standardization in the same format. Data entry services can help with transforming the data from handwritten or printed sources to a digital format. This is especially important while working with patients’ records.
What AI in Drug Discovery Will Bring in 2024?
The interest in the application of AI among pharma giants is too high, which proves multiple contracts that were signed in 2023.
BioNTech concluded a deal for almost £500 million to acquire AI/ML company InstaDeep. It will help them expand the application of AI in drug development for immunotherapies and vaccines. Another AI deal was concluded between Sanofi and Aqemia, worth $140 million. This will help in discovery of small molecules in multiple therapeutic areas.
Verge Genomics announced positive and safety data from the Phase 1 trials for its AI-candidate in therapeutic development for ASL. The drug development is still ongoing, and we expect new announcements in 2024. Another successful example showed Lantern Pharma with their RADR (Response Algorithm for Drug Positioning & Rescue) AI platform. The platform takes part in multiple ongoing projects. Notably, with novel AI algorithms, they lead preclinical trials in oncology. They already showed exceptional accuracy in predicting blood brain barrier (BBB) permeability.
Overall, Pharma AI Readiness Index launched by CB Insights in 2023 shows that big pharma companies compete in investing millions of dollars in AI technology. The most scoring leaders in terms of innovation, execution, and talent are Roche and Bayer. With all the initiatives and investments, 2024 is promising for new discoveries and new AI solutions in the pharma industry. We witness another exciting transformation of the industry with the help of AI.
Efficient AI application starts with labeling your existing data. If you need the help of professionals to handle the task, look no further. Contact us now!
Final Thoughts
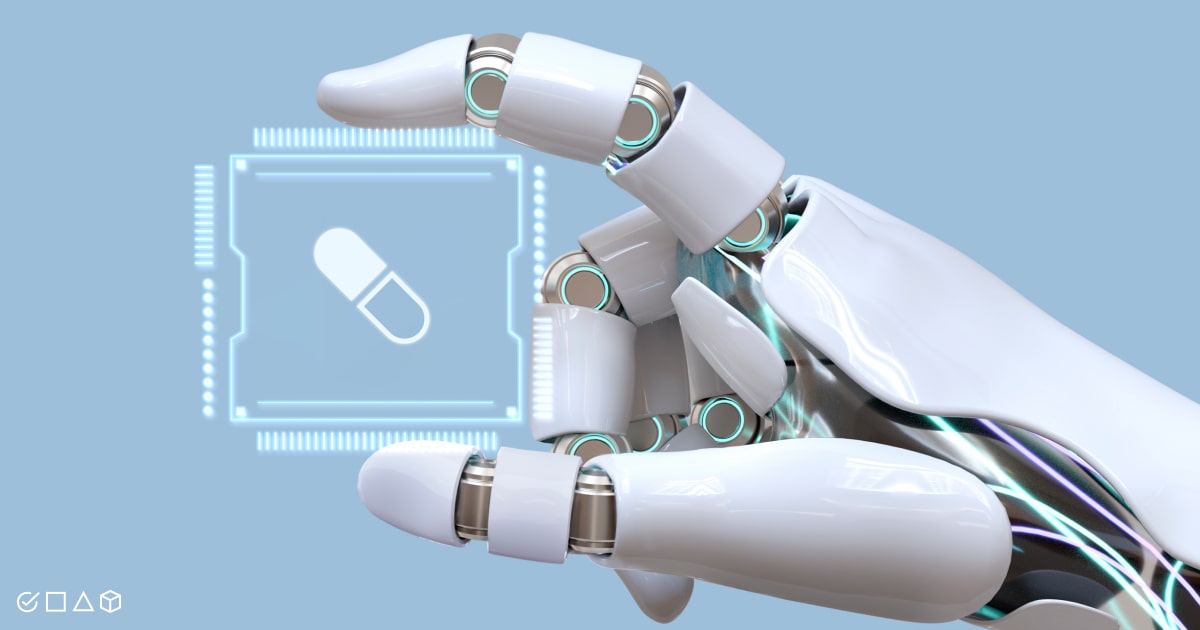
AI in the pharma industry, and in drug discovery in particular, expands, showing wonderful results. With all its challenges, the final outcomes overcome the efforts. The already available solutions in drug discovery and preclinical trials for such diseases as cancer, ASL, or psoriasis give us hope for the new turn in the pharma industry.
The combination of researchers’ expertise and AI technology give us the possibility to grow, designing a more effective curation of diseases in a shorter time. Now is the time when, by managing progress, we create the history of innovation.
FAQ
What is the role of generative AI in drug discovery?
Generative AI is the main contributor to the drug discovery process, leveraging machine learning algorithms to generate and optimize potential drug candidates. This field of artificial intelligence generates new molecule structures, or compounds, based on the analysis of the existing datasets.
What is the new drug found by AI?
AI is used in multiple ongoing projects of drug discovery led by different pharma companies. One of the successful results showed Insilico Medicine, who completed Phase 0 trials and is currently in Phase 1 trials of AI-generated anti-fibrotic drug candidates.
Can AI take over pharmacy?
AI has a potential of influencing the pharmacy, but automation could never truly replace pharmacists and their staff. For now, AI is expected to rather help the pharmacists by automating their routine tasks and optimizing patient cases. AI can also assist with decision-making, but a specialist still needs to validate the final data, suggested by AI.
Table of Contents
Get Notified ⤵
Receive weekly email each time we publish something new: