Artificial Intelligence in Healthcare: What’s New in the Industry?
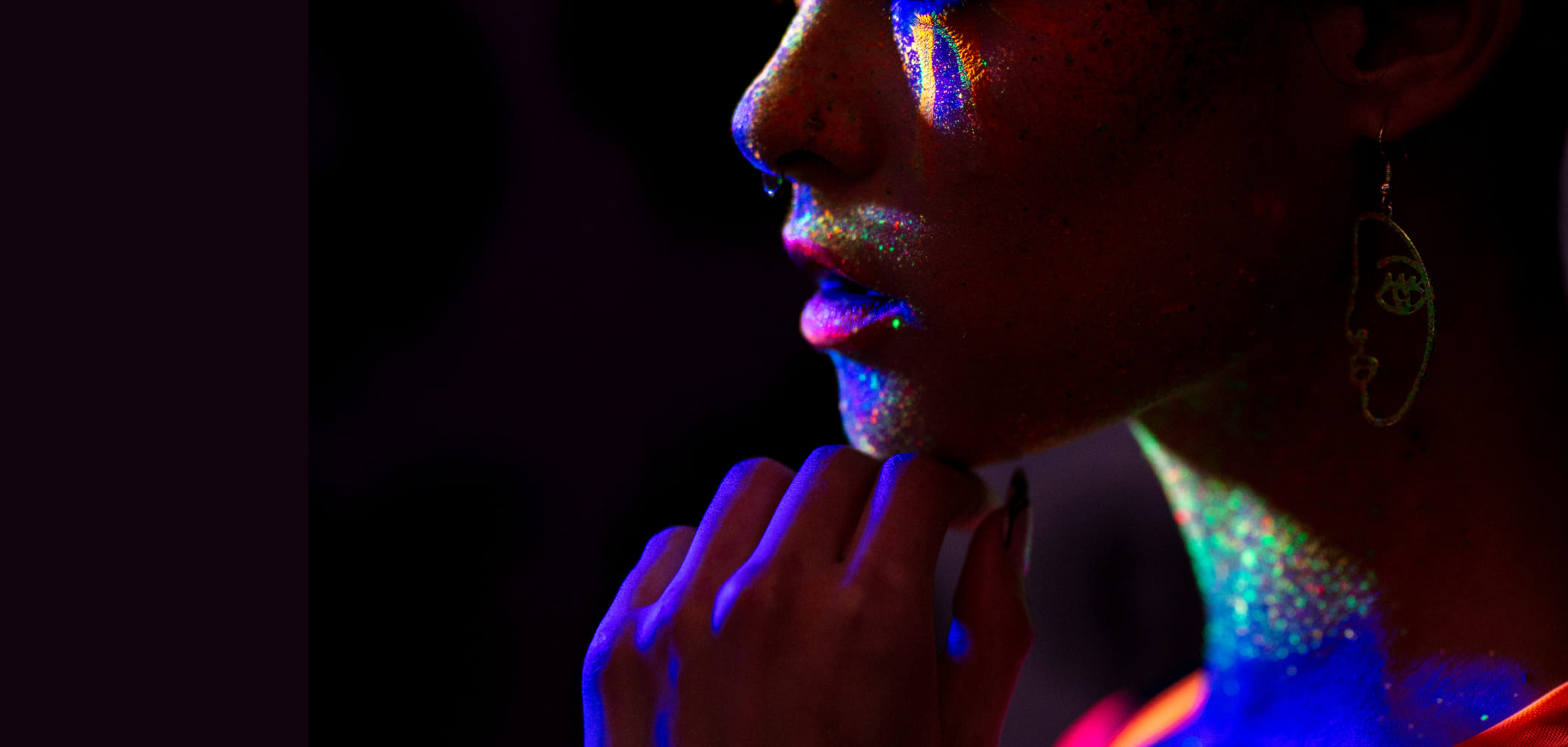
Medical AI figures prominently in the global economy and is no less important in everyone’s life. The brave struggle against the virus throughout the last two years of the pandemic only confirms this.
According to the market forecast, the global AI in healthcare market is expected to reach USD 95.65 billion by 2028, at a CAGR of 46.1%. The main reason for this is the soaring demand for improved, faster, more accurate, and personalized patient treatment. In addition, the increased adoption of advanced technologies in healthcare is due to the growing potential of artificial intelligence in drug discovery and genomics.
However, artificial intelligence covers a much broader spectrum of medical services and offers significant improvements in automated patient care, as well as a reduction in manual work and healthcare expenses. It’s also a viable tool for managing complex medical data, which machines use to learn to detect diagnoses at an early stage and even provide treatment recommendations. Here, the analytical, predictive, and recommendation capabilities of machine learning are at their best.
Among the many interesting facts about AI in healthcare, we want to dive deeper to understand why AI is so paramount and what mechanisms enable its effective employment across the medical field. We’ll also look into the role of data annotators in this sector and explain why they cannot do without the experts in the field to label medical data. Continue reading to learn more!
How Is Machine Learning Used for Healthcare?
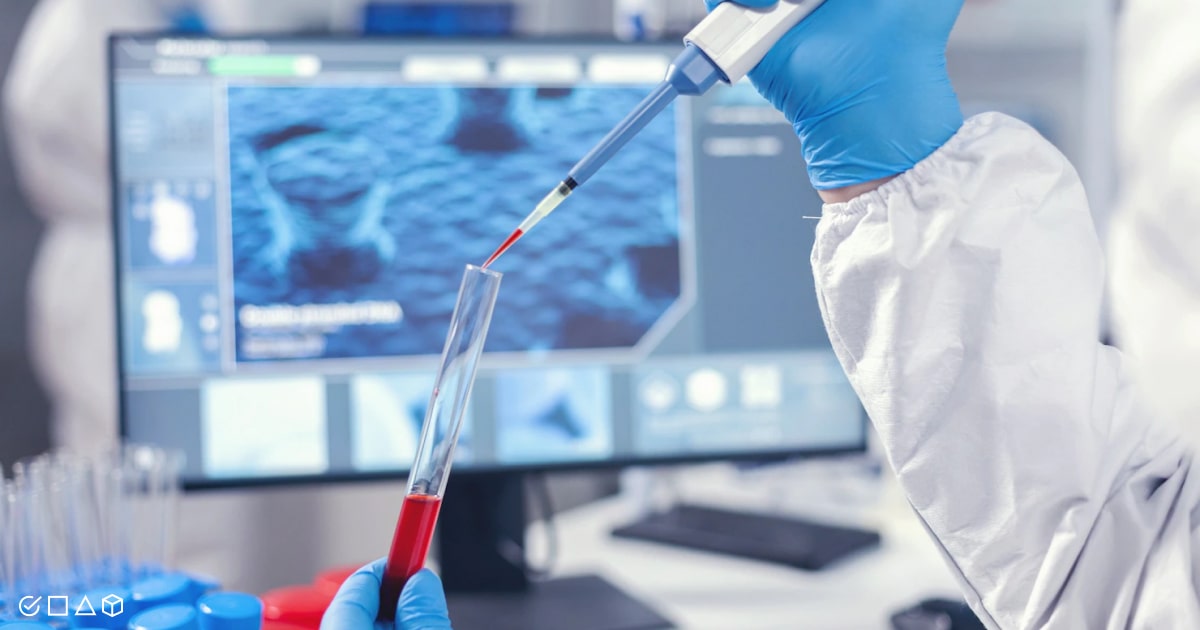
Machine learning is a crucial discipline in artificial intelligence that aims to mimic human intelligence and behavior in a way that it becomes a useful asset for humanity. For now, there are only a few cases of machines surpassing humans, but it doesn’t seem like AI will ever be able to completely replace humans. In the field of medicine, though, attempts are already being made.
However, efficient patient care is the one based on personal touch and emotional support, which is something that machines still lack (for now). Meanwhile, the data is tremendously growing and requires even more robust computational power to process it and build various ML algorithms. They have grown inseparable from our daily lives as a result of their widespread use in numerous fields.
Modern healthcare, which has previously been immune to large-scale technology upheavals, is increasingly being influenced by machine learning. Its many methods have demonstrated exceptional results in performing medical tasks, such as medical image recognition of body organs, classification of interstitial lung diseases, detection of lung nodules, medical image reconstruction, and brain tumor segmentation, to mention a few. So it makes sense that machines are soon to revolutionize medical research and practice by assisting radiologists and physicians in the clinical examination of patients.
Moreover, machine learning has contributed to the emergence of clinical medicine, a thorough examination of highly technical medical issues and hands-on clinical experience. This discipline presents a wide scope of applications for the ML models, which have already attained a human-level performance in clinical pathology, radiology, ophthalmology, and dermatology.
Some of these models managed to outperform human healthcare professionals on average. This fact underpins the development of computer-assisted diagnosis systems. Case in point, the U.S. Food and Drug Administration (FDA) introduced one such system in 2018, which was trained to detect diabetes-related eye issues on medical images with no human intervention.
ML is inevitable for the future of intelligent healthcare, which will become more and more dependent on ever-advancing technologies, like edge computing, mobile communication, and big data technology. When combined with these technologies, ML is able to generate highly accurate outcomes and set a new milestone in human-centered intelligent solutions.
The most commonly used ML methods have the potential to revitalize medical services, especially in remote rural areas, and reinvigorate more accurate, accessible, secure, and personalized patient care.
Supervised Learning
Supervised ML tasks in healthcare, which use labeled training data, include the classification of different types of lung diseases (aka nodules) and medical image recognition of various body organs.
Unsupervised Learning
Unsupervised ML methods are based on unlabeled data and are most widely used in the medical settings for heart disease prediction by performing clustering and hepatitis disease prediction using principal component analysis (PCA).
Semi-Supervised Learning
When you work both with labeled and unlabeled data, you are dealing with semi-supervised ML, which is quite beneficial for a range of medical applications. The reason for this is that obtaining a significant amount of annotated data for ML model training is challenging in healthcare. Here, the semi-supervised tasks include clustering for healthcare data, activity recognition using sensor data, and medical image segmentation.
Reinforcement Learning
Reinforcement ML refers to the methods that examine how intelligent agents should operate in a given environment to optimize the concept of cumulative reward. Many existing technologies and apps in healthcare might benefit from RL, and it has recently been employed for context-aware symptom assessment for illness diagnosis.
Machine Learning Applications in Healthcare
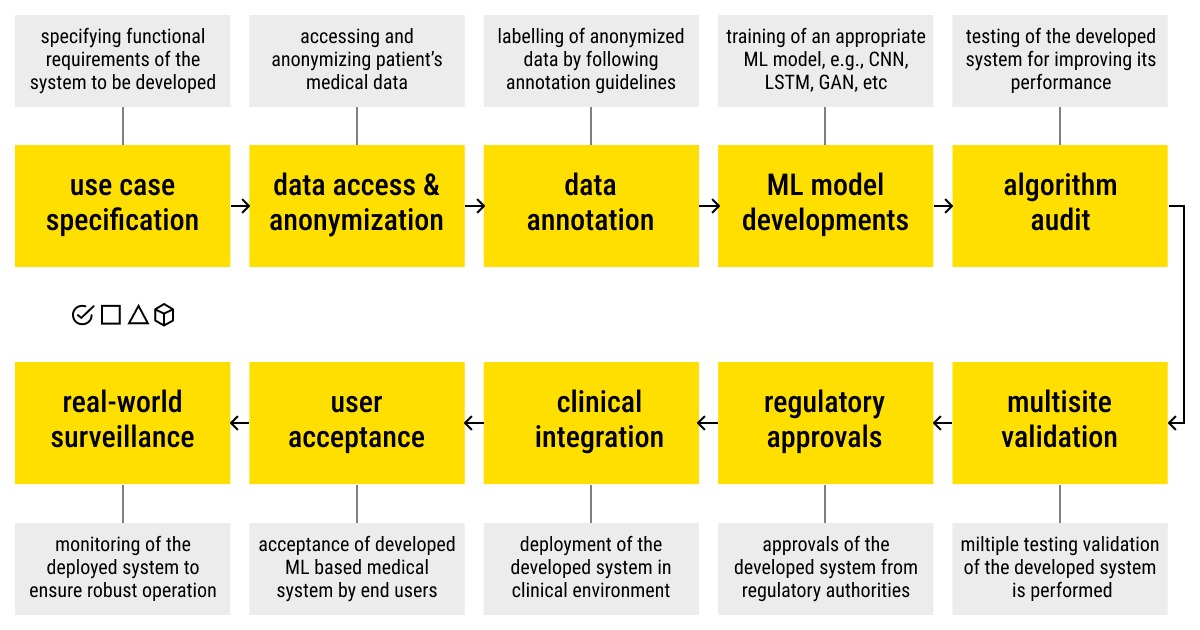
Medical services, and healthcare in general, revolve around tremendous volumes of patient data on a daily basis. Therefore, the traditional methods in healthcare turned out to be inefficient in dealing with mass medical data. And so machine learning techniques are becoming increasingly deployed to handle this data by analyzing it and generating actionable insights.
Besides, heterogeneous data sources exist to amplify healthcare data, including genomics, medical data, social media data, environmental data, and so on. That said, there are four main areas of efficient application for ML in healthcare, such as prognosis, diagnosis, treatment, and clinical workflow. Let’s take a look at each of the machine learning use cases in more detail!
However, if you already have a project in progress, and you know exactly what kind of ML task is performed, send the request to our team of data annotation experts to prepare your medical data for model training and receive the most effective and accurate results!
— Prognosis
Healthcare prognosis and machine learning are a fantastic combination since they both deal with prediction. Prognosis is the technique of predicting how an illness will progress in the future. Isn’t ML based on the same idea?
In this case, ML models are trained on multi-modal patients’ data, including phenotypic, genomic, proteomic, and pathology test results, as well as medical imaging data, to improve disease prognosis outcomes, diagnosis, and treatment. Current ML applications for disease prognosis encompass the prediction of disease symptoms, risks, survivability, and recurrence. For example, machine learning has been already used to identify and classify different types of cancers (e.g., brain tumors and lung nodules).
— Diagnosis
Electronic Health Records (EHRs): Every day, a large portion of EHRs is produced by hospitals and other clinical service providers. They consist of both structured and unstructured data on patients’ complete medication history. Machine learning is used to extract valuable information from this data, that is clinical features, to augment the diagnosis process.
Medical Image Analysis: Among many AI use cases in healthcare, ML methods are fundamental for efficient and effective extraction of information from medical images obtained from magnetic resonance imaging (MRI), computed tomography (CT), ultrasound, positron emission tomography (PET), etc. It’s an essential ML application in healthcare because medical images contain crucial information on functional and anatomical characteristics of body organs, which helps detect, localize, and diagnose abnormalities.
Here, ML proves to be a useful asset for clinicians and radiologists to successfully diagnose and predict the disease at an early stage of its development. The key ML tasks in medical image analysis include detection, classification, segmentation, retrieval, reconstruction, and image registration.
— Treatment
Image Interpretation: Medical images contain valuable information about patient health, and it’s a common clinical practice to analyze and interpret them by expert physicians and radiologists. However, this process is tedious and takes a lot of time that physicians could rather dedicate to their patients.
ML has been applied to solve this problem using natural language processing (NLP) and other techniques. Some machine learning in healthcare examples include annotating clinical radiology reports for NLP tasks, automatic tagging and description of medical images, an end-to-end architecture using CNN and RNN for chest X-rays, and a long short-term memory (LSTM) network for automatic report generation.
Real-time Health Monitoring: Critical patients require continuous, real-time health monitoring, which is an important part of the therapy process. Different technologies are used to support this process, like wearable devices, IoT sensors, and smartphones. All the devices are based on health data that is collected and transmitted to the cloud for further analysis using machine learning. The results are sent back to the device for further processing and relevant actions.
— Clinical Workflows
Disease Prediction and Diagnosis: ML plays a pivotal role in predictive healthcare. Its algorithms can be used for an effective cardiovascular risk prediction, as well as the detection and diagnosis of different diseases, including cancer.
Computer-Aided Detection or Diagnosis (CADx): These ML systems are designed for the automatic interpretation of medical images to assist radiologists in their clinical practice. Different ML techniques are used to run the systems, like traditional computer vision and image processing. IBM’s Watson is one such example of a CADx system.
Clinical Time-Series Data: ML applications of clinical time-series data include prediction of clinical interventions in intensive care units with CNN and LSTM, mortality prediction in patients with traumatic brain injury (TBI), and measuring of mean arterial blood pressure (ABP) and intracranial pressure (ICP).
Clinical NLP: Clinical NLP advancements are expected to be implemented into future clinical software for extracting meaningful information from unstructured clinical notes in order to improve clinical practice and research.
Clinical Speech and Audio Processing: Clinical speech and audio processing provide new potential to solve the constraints of time-consuming clinical documentation, such as speech interfaces for interaction-free services, automatic transcription of patient talks, and synthesis of clinical notes, among others. Speech processing has been used to diagnose speech-related illnesses, including Alzheimer’s disease.
Altogether, these ML solutions benefit all the stakeholders in healthcare, including patients, clinicians, and the industry as a whole.
How Is Medicine Transformed with Machine Learning?
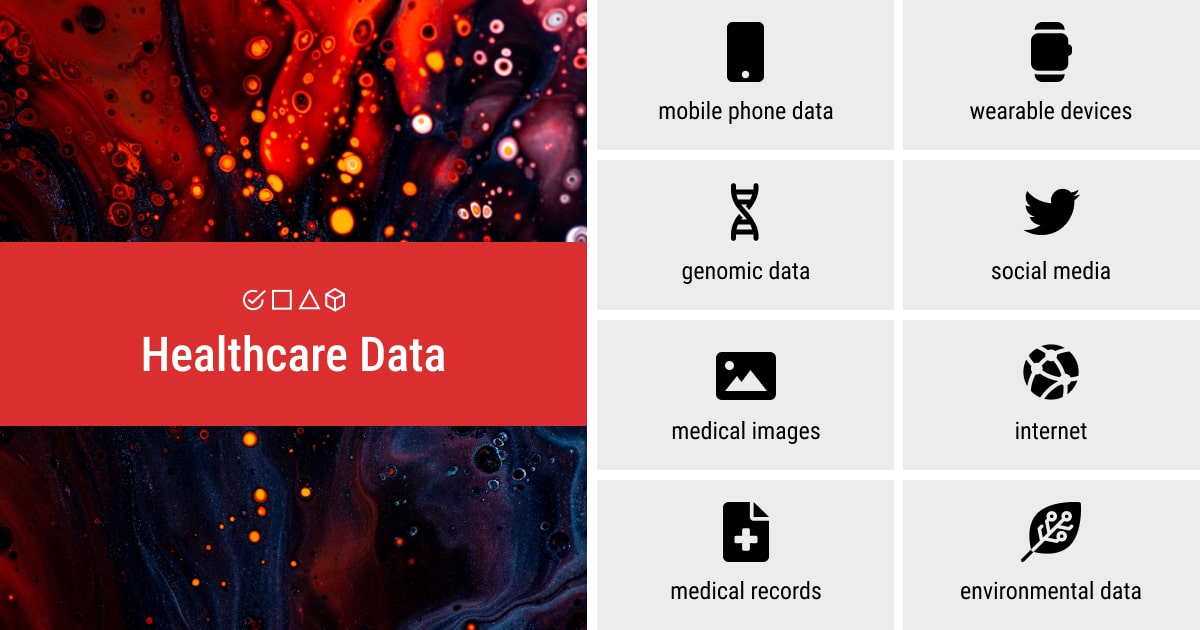
- Trustworthy ML
For effective machine learning deployment in healthcare, both the physicians and the patients must trust the output of the ML systems. Thus, ML needs to be consistently applied within the healthcare settings to ensure that the outputs are reliable and can be used for clinical decision-making. Trust in machine learning depends directly on complete, labeled data, technical robustness of the system, human control, and the general workflow that requires continuous feedback loops and monitoring of long-term outcomes.
- Transparent and fair ML
Machine learning is notoriously prone to bias, a disturbing issue for healthcare-related systems, where human life depends on the accuracy and precision of ML algorithms. For machine learning to become transparent and fair, both education on bias and a number of measures with data need to be taken. In medicine, transparency of ML systems revolves around equity and equality of patient treatment, which can be feasible through an easy-to-access, open, and uniform (yet personalized to each patient) ML framework in healthcare.
- Easy-to-use and effective ML
The usability of machine learning indicates the degree to which a model can help reach specific goals in the most efficient way to satisfy the needs of patients. Such ML must be scalable across different medical settings and improve traditional patient care. This can be achieved through system feedback, understanding of current clinical workflow, and interdisciplinary communication across organizations, sectors, and groups of healthcare providers.
- Clear ML
The explainability of machine learning in healthcare implies the two main requirements: completeness and interpretability. To achieve this, one must ensure that data processing is clear and that various techniques are applied for the visibility of inputs and outputs. Clear and explainable ML healthcare is important for the development of therapeutics and diagnostic tests. However, it’s not always required, especially if the ML system has demonstrated a high level of trust.
- Responsible and ethical ML
The ML systems developed for the clinical settings are based on the idea of serving humanity by improving patient care and upgrading healthcare to the point where technology can save human lives. This is indeed a great responsibility for machine learning. Safe, meaningful, and responsible ML for healthcare must engage an interdisciplinary team, consisting of different stakeholders, like knowledge experts, decision-makers, and users. Responsible ML practices in medicine establish a set of core processes, such as:
- Defining the problem
- Finding the solution
- Considering ethical implications
- Evaluating the model
- Reporting outcomes
- Deploying the system responsibly
Patient Data and Medical Annotation
AI in healthcare is a major breakthrough, which benefits both patients and healthcare providers by enhancing efficiency and lowering costs. However, as in any other industry, machine learning in healthcare requires annotated data to build effective systems for automated and personalized patient treatment or medical research.
Expert data labeling is essential for producing high-quality training data for AI-assisted healthcare. Let’s explore some of the most popular AI use cases in healthcare, as well as how data annotation helps them grow.
- Surgery: Precision data labeling is used for robotic surgery.
- Medicine:Pattern recognition systems are applied for advanced research and drug discovery, as well as personalized drug treatment.
- Diagnosis: Medical image annotation of MRIs, X-rays, and CT scans is used for diagnostic support; object detection on thermal images is applied for early diagnosis of diseases (e.g., breast cancer).
- Virtual Assistance: Labeled data is used to train chatbots, conversational robots, and virtual assistants to monitor and assess health status, schedule doctor appointments, and send prescription reminders.
- Patient Engagement: Annotated data helps to improve patient follow-up and maintenance after therapy with entity recognition for creating chatbots and audio and text transcription to digitize record management.
Label Your Data & Medical AI
Are you involved in a healthcare-related initiative in AI? If you are, make sure to find a reliable annotation partner for your project. Label Your Data can become your trusted guide into medical AI since we have vast experience in working with healthcare data for machine learning and value security and accuracy above all.
We have annotators with a medical background on board who have annotated more than 50,000 medical records for one of our clients using OCR and text classification and classified more than 100,000 images of blood cells. We are a flexible team that can work both using our own labeling tools and the external ones provided by the client.
Contact our team to reap all the benefits of the efficient and scalable data annotation process that we provide and explore the unlimited potential of AI in healthcare!
Final Thoughts: Defining the Future of Healthcare with AI
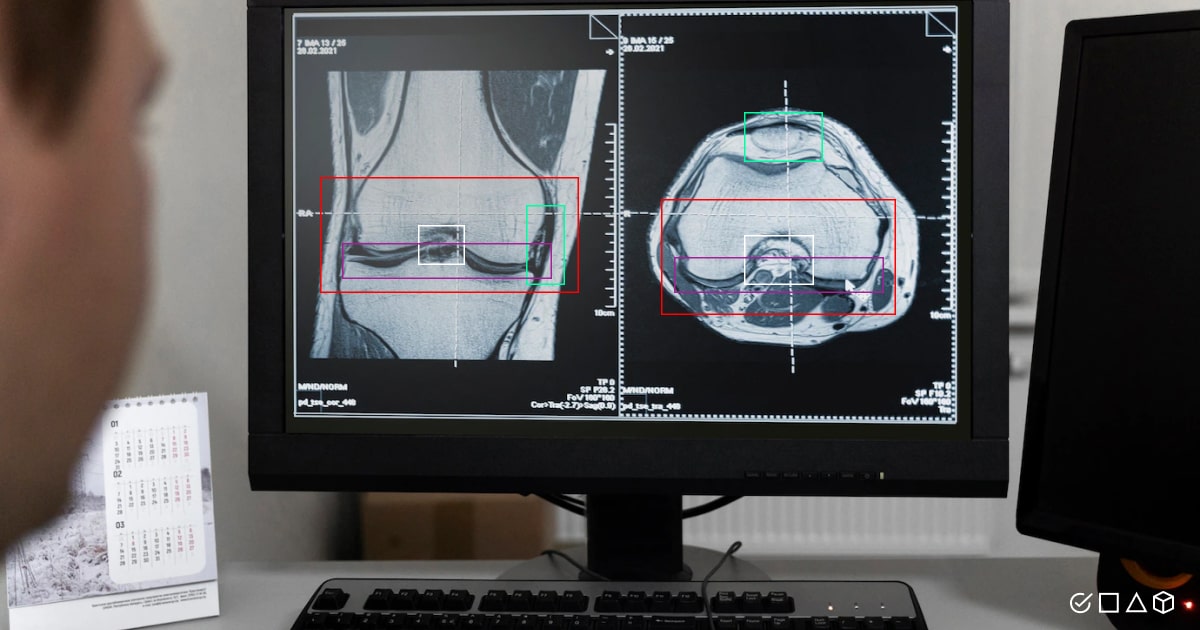
Finding the golden mean between technology and the value it adds to human life is the key to combining healthcare with artificial intelligence.
With the growing need for machine learning algorithms in medical research and clinical practice, healthcare providers must undergo the appropriate training to grasp the main idea behind ML and beware of risks. Thus, it’s a collaborative effort between data scientists and physicians to create the most optimal and reliable ML systems for improved patient care.
But we shouldn’t forget that data is at the heart of every AI project, especially if we are dealing with supervised algorithms. Hence, the lack of annotated data is a challenging issue in healthcare settings, where ML is used to devise efficient tools and systems for better patient care and to assist healthcare professionals.
Finally, while using AI in healthcare and bringing technology closer to patients, it’s imperative to follow ethical guidelines and the main principles of equal healthcare for all.
Written by
Karyna is the CEO of Label Your Data, a company specializing in data labeling solutions for machine learning projects. With a strong background in machine learning, she frequently collaborates with editors to share her expertise through articles, whitepapers, and presentations.