Computer Vision in Agriculture: AI for the Ultimate Transformation in Farming
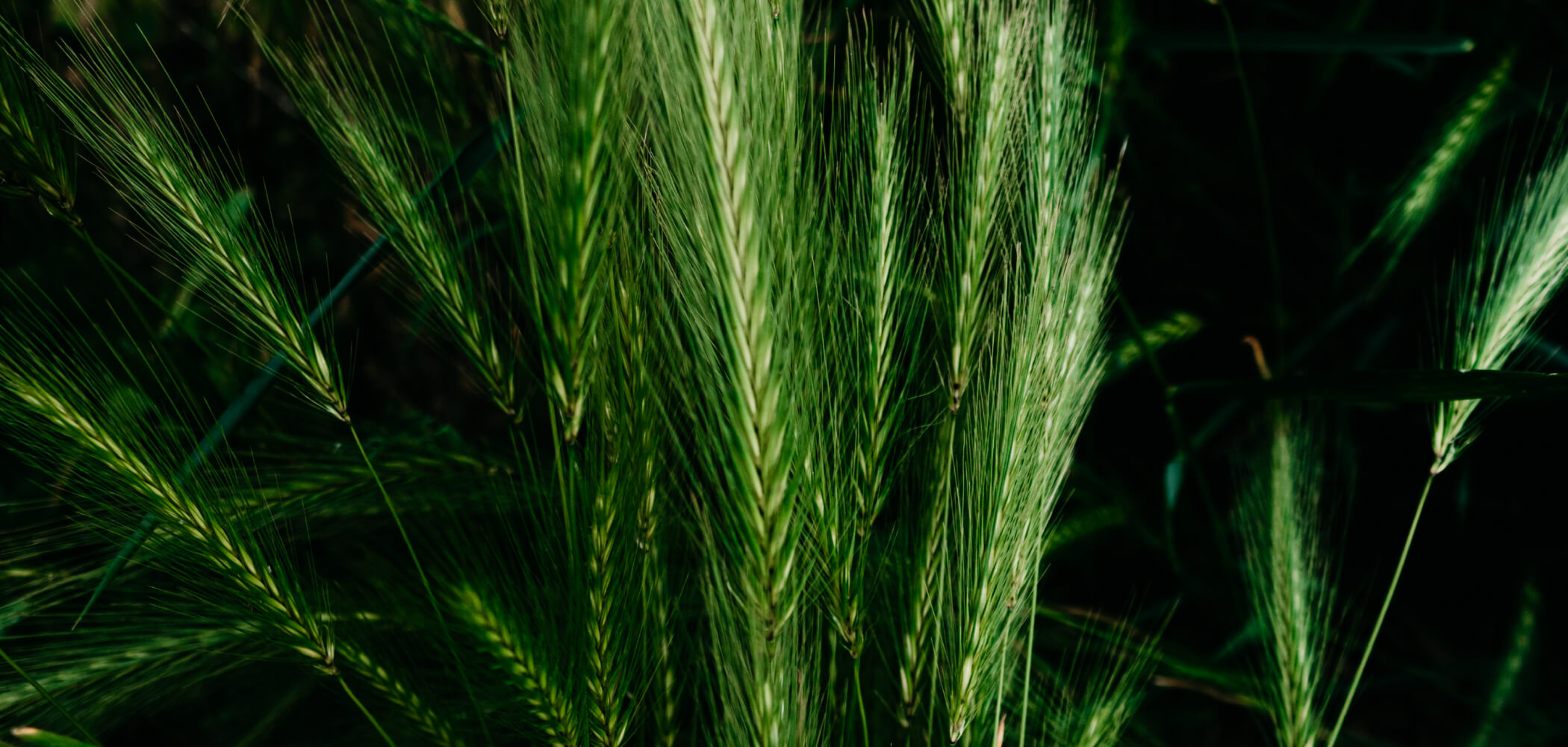
Almost 10 billion people — this is the expected population of our planet by 2050. This poses a great challenge for global food production, which will need to double within a very short time to fulfill the rising food demand.
Now, around 80% of the food consumed worldwide is produced on farms. So, today, enhancing agricultural practices and embracing artificial intelligence is a viable approach towards sustainable food production. Automation makes a world of difference for major industries today. Agriculture is one such industry.
The adoption of AI in agriculture projects has brought many great benefits to the industry. In fact, AI has spawned a number of the newest trends and technologies in the agricultural sector, such as precision farming, or in other words, smart farming. Most of the technologies used for computer vision in agriculture are rapidly revolutionizing modern farming by enabling farmers to monitor crops more effectively and improve their yields.
What lies behind such a complex technology of machine vision in agriculture, and how is the sector transforming as a result? Let’s see!
Why Computer Vision in Agriculture Is Used in Precision Agriculture?
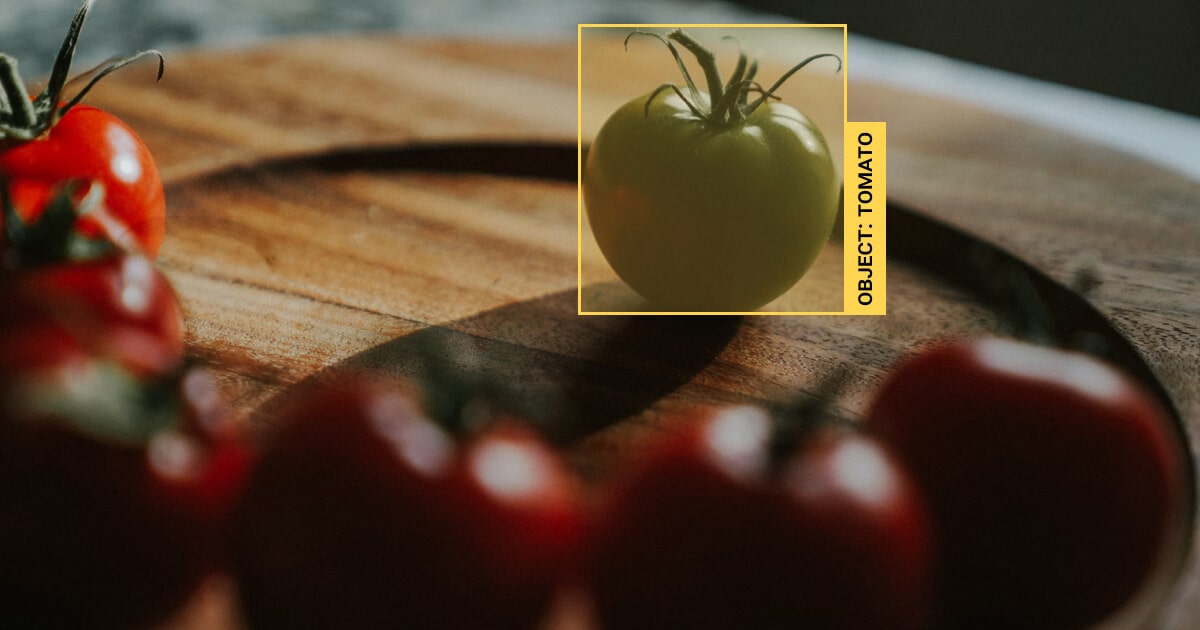
Agriculture has been the backbone of human civilization. It was the first industry to develop and has helped us sustain ourselves for thousands of years. However, increasing population, climatic changes, and limited resources have made agriculture a challenging endeavor.
According to different data, the rising food demand by 2050 necessitates from 60% to 70% increase in crop yields. For this reason, more and more farmers turn to computer vision, given that at least 30% of the increase in crop production can be achieved by this AI solution. Computer vision in machine learning is the top-level image processing that can automatically carry out a variety of activities such as automatic object detection, recognition, categorization, and monitoring.
Numerous machine learning and deep learning (CNN/RNN) techniques are used to ensure higher accuracy of computer vision in agriculture industry. The higher accuracy of CV algorithms helps farmers more efficiently keep track of illnesses, pests, degrees of water stress, soil nutrients, and other factors impacting production. By combining this technology with other data sources, such as weather predictions or satellite imaging, they can get crucial insights into crop health and improve farm-level operations.
Computer vision in the agriculture field has enormous potential when used for livestock monitoring in addition to revealing the best ways to manage crops. The use of drones flying overhead or cameras set on farms to distinguish individual animals from one another based on their distinctive characteristics has become possible thanks to machine learning algorithms. This frees farmers from having to rely purely on human observation, which would be a far less precise (and time-consuming) procedure overall.
Additionally, computer vision in agriculture sector enhances sustainability measures, such as lowering chemical inputs through precise application methods. Future developments in sustainable farming techniques and precision agriculture will offer tremendous potential to significantly lower the carbon intensity of biofuels.
Top 9 Applications of Computer Vision in Agriculture Sector in 2023
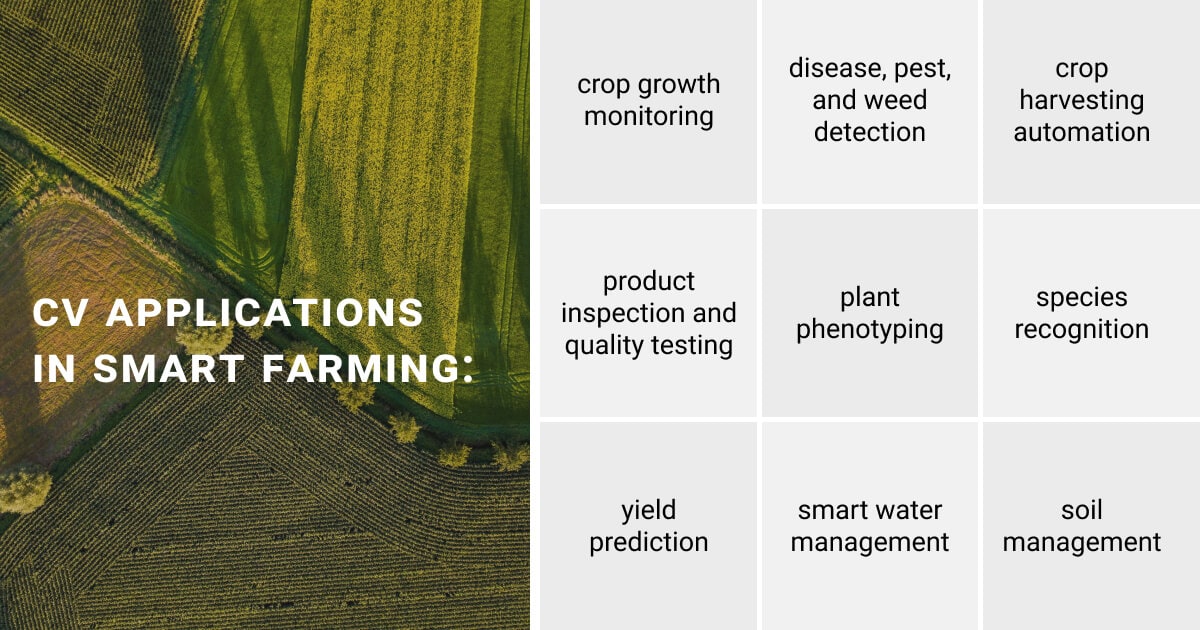
Computer vision in agriculture gathers essential visual information about crops, livestock, farms, and gardens. This enables farmers to recognize, detect, and track certain items using visual cues as well as to understand complex visual data for automated agricultural activities.
Moreover, combining CV methods with high-throughput molecular DNA sequencing methodologies helps understand complex traits like plant yield and productivity, stress tolerance, biotic and abiotic stress management, etc. Yet, most of the current applications of computer vision in agriculture sector are based on sensing technology, underpinning data-driven precision agriculture. They are used directly on the fields and can be accessed through mobile or web applications.
Let’s talk about the main applications of computer vision in agronomy sector in more detail:
- Crop growth monitoring
One of the key applications of computer vision in agriculture industry is crop health monitoring. It employs cameras to capture various crop growth stages and increase production efficiency. Farmers can better identify subtle abnormalities in crop development caused by malnutrition at an initial phase.
- Disease, pest, and weed detection
Farmers can automate crop inspection with the use of CV imaging methods. By processing data from photos taken by RGB cameras, drones (UAV), satellites, and mobile ground robots, it can more precisely anticipate crop issues. Pests, illnesses, and weed infestations may be found using satellite imagery on a bigger scale and with less complexity.
- Crop harvesting automation
Robotic systems for the automated harvesting of various fruits and vegetables, such as cucumber, apple, and cherry, have undergone revolutionary modifications thanks to computer vision.
- Product inspection and quality testing
Given the rising consumer demand, one of the most crucial concerns for the agriculture sector is the capacity to visually assess a product’s quality. To improve the capability of the classification systems, computer vision in agronomy layers symbolic knowledge from human experts on top of the numerical data. It is known as a neuro-symbolic hybrid system (NSHS).
- Plant phenotyping
It is the method of determining the physical plant traits and functions that may be mutually influenced by genotype and environment, and is based on science. Deep learning CV technologies are now often utilized in plant phenology and phenotyping to increase plant production.
- Species recognition
Without the need for human labor, computer vision in agronomy may be utilized to identify and categorize plant species more quickly compared to humans.
- Yield prediction
As yield prediction has exceeded the conventional prediction-based traditional approaches for crop production, it has become one of the most well-liked study subjects in precision agriculture. Over the past several years, yield prediction has been greatly advanced by using machine learning algorithms.
- Smart water management
Agronomic, hydrological, and climatological balance in agriculture is significantly impacted by water management. To create a regular irrigation system that is effective, several computer vision algorithms have been created.
- Soil management
To better comprehend current ecological practices in agriculture, computer vision algorithms are utilized to examine evaporation processes and assess soil moisture and temperature.
Data Annotation for Computer Vision in Farming
Traditionally, farmers relied on their human vision to monitor the amount of crop, detect the infected crop, or prevent natural disruptions. However, AI breakthroughs go beyond what the human eye can see. Process automation and increased visibility are made possible by computer vision in agriculture using cutting-edge cameras, sensors, and software. But training datasets for agricultural applications must first be prepared to make these technologies work.
Labeling agricultural data for training computer vision models is widely applied across the sector. This can be anything from scanning and labeling of fruit and vegetables to annotating fields and farming equipment. Data annotation in AI automates processes like livestock management, fructification detection, undesired weed detection, crop health and soil monitoring, and makes them much more efficient.
Here are the common data labeling types used for computer vision in farming:
- Bounding Boxes for Crop Detection
Bounding box annotation can simplify the crop detection process. It helps create perception models that can distinguish weeds from crops and get rid of the grass.
- Keypoints for Detecting Shape and Size of Fruits & Vegetables
Keypoint aka landmark annotation is great for analyzing the size and shape of an object, like a fruit or vegetable, as well as signs of ripening and fructification.
- Semantic Segmentation for Crop Inspection
Precise semantic segmentation in computer vision addresses various segmentation and classification issues in precision agriculture. This covers crop detection, cow respiration rate detection, semantic text segmentation and localization, as well as semantic picture and video segmentation of agricultural datasets and semantic segmentation of 3D point clouds. You can also use semantic segmentation to eliminate unwanted crop data from the image.
- Polylines for Classifying Crops Lanes in Big Farms
Polyline annotation can classify objects and, thus, help develop robust models for computer vision in agronomy industry that can carry out disease detection, irrigation pattern analysis, and harvest prediction.
- GIS & Geospatial Data Annotation
This type of data labeling assists AI-powered farming drones to keep track of farm conditions and crop health. It also helps monitor the condition of agricultural equipment and boosts the agriculture output overall.
AI Behind the Future of Smart Farming
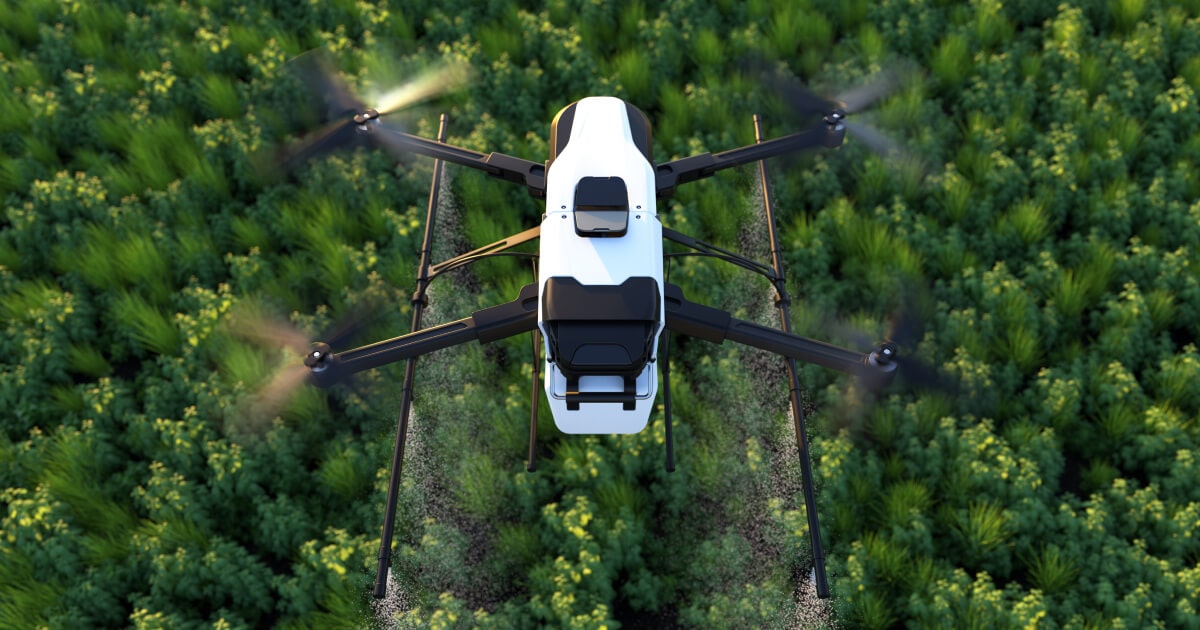
The potential to improve human vision is one of the most intriguing features of computer vision technology.
Technical developments like machine learning and deep learning are now more widely available and reasonably priced, making them useful for the agricultural sector. There is no doubt that with all of these great technologies at our disposal, AI will play a crucial part in helping us meet our expanding food demand while minimizing environmental effects.
FAQ
How computer vision is used in agriculture?
Computer vision in agriculture is a great way for farmers to identify product defects, sort the produce by weight, color, size, ripeness, and many other factors. CV solutions offer significant benefits when used in conjunction with appropriate machinery. For example, you can build a fruit classification system using CV by performing the labeling of fruit and vegetables.
What are the positive impacts of using computers in agriculture?
Thanks to computers, farmers can stay updated with all recent information about their crops. This is inclusive of data about harvest, weather conditions, and more advanced ways of enhancing crop quality and production.
Written by
Karyna is the CEO of Label Your Data, a company specializing in data labeling solutions for machine learning projects. With a strong background in machine learning, she frequently collaborates with editors to share her expertise through articles, whitepapers, and presentations.