- TL;DR
- Top Financial Dataset Resources
- How to Stay Updated on New ML Datasets
- What Data Should Be Labeled in Financial Data Sets
- FAQ
TL;DR
Discover top resources for financial data sets, including Kaggle and Quandl.
Learn how to stay updated on new data releases for ML projects.
Understand what types of financial data should be labeled for accurate modeling.
Expert tips: prioritize data quality, relevance, and up-to-date information.
Access insights on balancing data granularity and consistency for better predictions.
Top Financial Dataset Resources
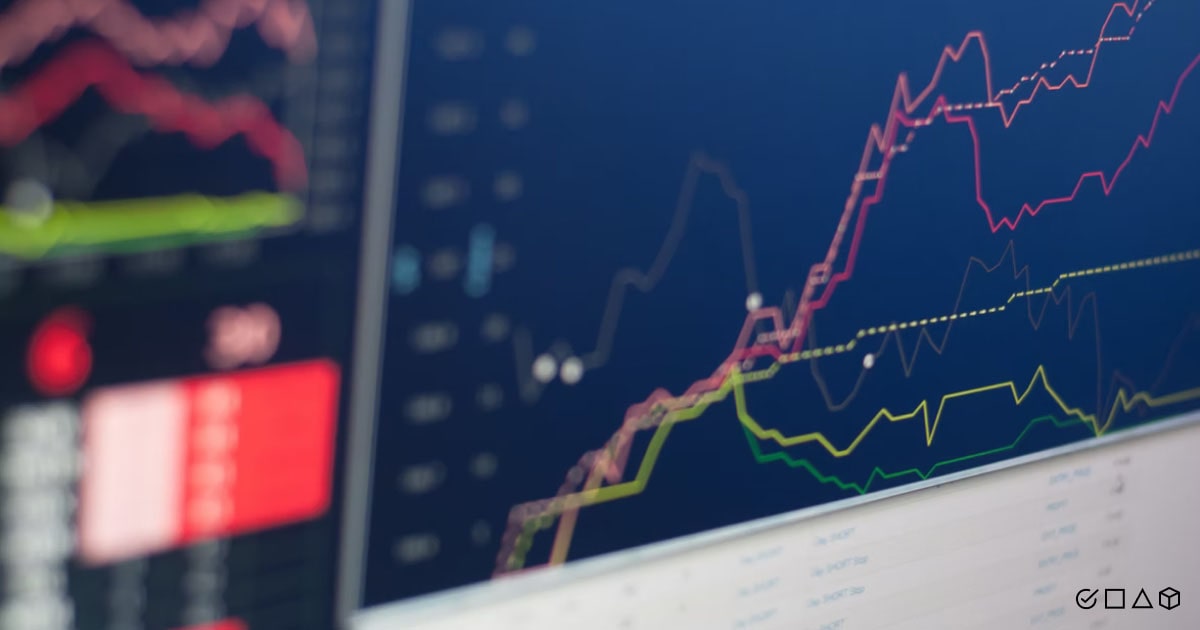
As the financial sector evolves, accessing high-quality data is essential for training machine learning models. Financial data sets, combined with accurate data annotation, provide valuable information to drive predictive modeling, automate trading, detect fraud, and improve financial decision-making.
“Historical consistency is crucial when selecting financial datasets for predictive modeling. Datasets must demonstrate reliability over time and have a proven track record of forecasting outcomes correctly. Regulatory compliance is also nonnegotiable to protect clients’ interests.”
President, RVW Wealth
Below, we’ll explore the top resources where you can find financial data sets, how to stay updated on new data releases, and the types of data that should be labeled for financial projects.
Here’s a list of the top platforms offering financial data sets for machine learning:
Let’s delve into some of these platforms and what they offer.
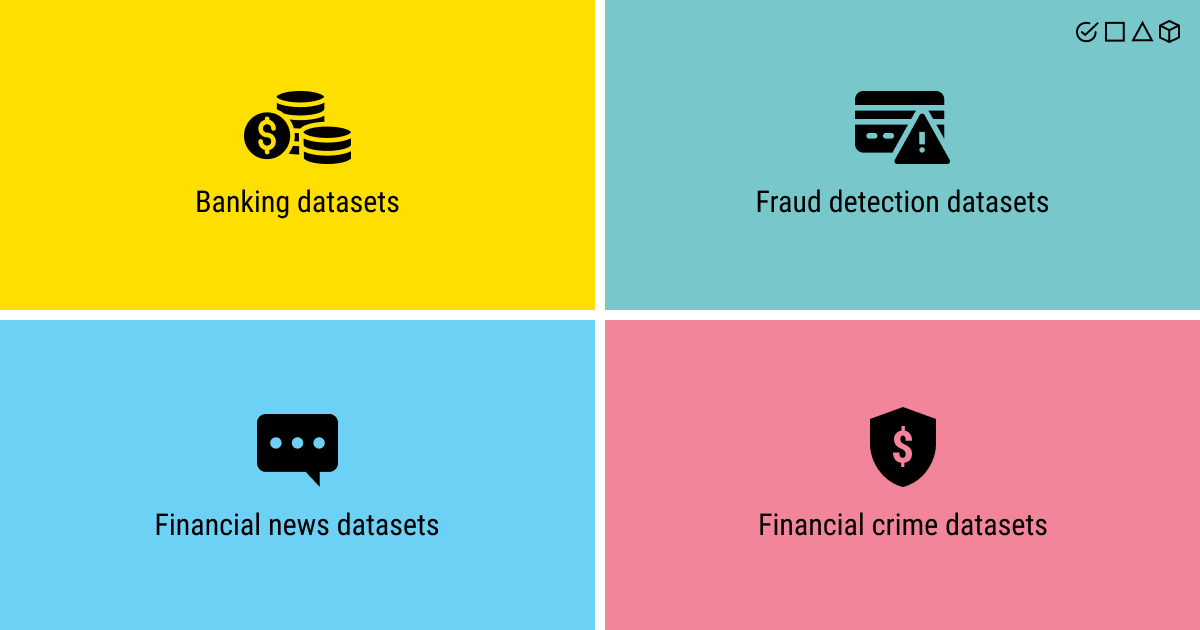
Looking for a reliable partner for collecting or labeling financial documentation? Contact our team and get your quote to find the best option for your unique ML project!
Kaggle
Kaggle is a popular hub for data scientists and ML engineers looking for high-quality datasets. It features a variety of financial data, including stock prices, cryptocurrencies, credit card fraud detection, and loan predictions.
The platform’s community shares valuable insights and solutions, making it easier for you to get started on projects. Kaggle’s financial data sets are diverse and continuously updated, giving you access to fresh information for your ML projects.
Top Kaggle financial data sets:
Credit Card Fraud Detection: Training models to detect fraudulent transactions.
Stock Market Data: Contains historical stock prices for building trading algorithms.
Bitcoin Historical Data: Useful for analyzing cryptocurrency trends.
Loan Default Prediction: Helps in modeling credit risk.
Financial News Sentiment Analysis: Predicting market movements based on news sentiment.
Quandl
Quandl provides reliable financial and economic datasets, suitable for predictive modeling and research. It offers both premium and free financial data sets, sourced from well-established financial institutions. The finance data covers various topics, including stock performance, commodity prices, real estate trends, and alternative metrics like social sentiment.
You can use Quandl’s data for algorithmic trading, economic forecasting, or quantitative research. Its high-quality, clean data helps you avoid spending too much time preparing data.
Top financial data sets available on Quandl:
End-of-Day US Stock Prices: Data on daily stock prices from major exchanges.
Global Futures Prices: Information on future contracts across various commodities.
Housing Price Index: Historical data on real estate trends.
Economic Indicators by Country: Covers GDP, inflation, and employment data.
Oil Prices Data: Tracks the historical prices of crude oil and other energy sources.
Data.gov
Data.gov is the U.S. government’s open data platform. You’ll find data on consumer spending, economic indicators, federal budgets, and more. The platform’s machine-readable data is valuable for financial modeling and policy analysis.
Updated regularly, Data.gov ensures that you work with the latest information available. Whether you’re doing research, building financial models, or analyzing economic trends, it’s a go-to source for public financial data sets.
Recommended financial data sets from Data.gov:
Consumer Spending Data: Useful for understanding trends in consumer behavior.
U.S. Federal Budget Data: Provides details on government spending and revenue.
Unemployment Insurance Weekly Claims: Offers insights into job market trends.
Bank Financial Reports: Includes information on the performance of U.S. banks.
Federal Reserve Economic Data (FRED): Data on economic indicators like inflation and interest rates.
World Bank Open Data
World Bank Open Data gives you access to a vast collection of global financial and development data. It includes economic indicators, poverty statistics, and country-specific financial metrics.
The data is highly useful for modeling economic forecasts and assessing risks. You can leverage it for credit risk analysis, economic development studies, or market predictions. It’s a must-have resource if your projects involve global financial or economic modeling.
Top datasets from World Bank Open Data:
Global Financial Development Database: Information on financial institutions and markets.
World Development Indicators: Comprehensive data on economic development and global growth.
International Debt Statistics: Covers debt across developing countries.
Doing Business Reports: Insights into the regulatory environment and ease of business.
Poverty and Equity Database: Tracks trends in poverty and income distribution.
IMF Data
IMF Data provides a range of financial statistics, focusing on global economic trends. You’ll find information on exchange rates, commodity prices, and government finances. It’s useful for macroeconomic analysis, financial stability assessments, and understanding the impact of international policies.
For ML projects, IMF Data can help you build models that predict economic outcomes or assess financial risks. Its international focus allows you to analyze trends across different countries.
Notable datasets from IMF Data:
World Economic Outlook Database: Forecasts of global economic trends.
International Financial Statistics (IFS): Includes data on exchange rates, GDP, and trade.
Global Debt Database: Information on the debt levels of various countries.
Currency Composition of Official Foreign Exchange Reserves (COFER): Tracks central banks' reserve holdings.
Commodity Price Data: Covers prices of goods like metals, oil, and agricultural products.
Financial Times/Markets Data
Financial Times/Markets Data is your source for real-time financial market data. It covers stock prices, commodities, currencies, and market indices, updated frequently to reflect the latest conditions.
By combining this data with news coverage from the Financial Times, you can get a comprehensive view of market trends. This makes it suitable for investment analysis, risk management, and financial forecasting.
Top datasets from Financial Times/Markets Data:
FTSE Index Data: Provides details on stock market indices.
Foreign Exchange Rates: Daily updates on currency values.
Commodities Data: Information on prices of gold, oil, and other commodities.
Global Market Trends: Analyzes shifts in major financial markets.
Corporate Bond Yields: Tracks bond performance and yields.
Google Trends
Google Trends offers insights into what people are searching for, including financial topics. You can track search trends for companies, stocks, or economic events. This helps gauge public interest and market sentiment.
Using Google Trends, you can predict market movements or identify emerging financial topics. This data is helpful for ML projects focused on sentiment analysis, market forecasting, or even algorithmic trading based on public behavior trends.
Popular financial data sets to explore with Google Trends:
Company Search Trends: Gauging interest in specific companies or stocks.
Cryptocurrency Search Trends: Measuring public interest in various cryptocurrencies.
Economic Event Searches: Tracking searches for events like stock market crashes or economic policies.
Sector-Based Trends: Interest in sectors like finance, real estate, or commodities.
Investment Keywords: Insights into what people are searching for related to investments.
EU Open Data Portal
The EU Open Data Portal offers financial data from European institutions. It covers topics like economics, trade, and government budgets. An essential resource if your work involves analyzing financial policies or economic activities within the European Union.
Use this platform to model economic trends, assess policy impacts, or analyze trade dynamics across Europe. The wide variety of datasets makes it suitable for different financial modeling projects.
Recommended data sets from the EU Open Data Portal:
Eurostat Financial Data: Provides economic statistics for the EU, including GDP, inflation, and trade.
Government Budget Data: Tracks public finance across EU countries.
EU Trade Data: Covers imports and exports by country.
Employment Statistics: Data on labor markets and job trends.
Inflation and Price Indices: Information on cost-of-living changes.
American Economic Association (AEA)
The American Economic Association provides access to various economic data, particularly U.S. macroeconomic data. You’ll find datasets on national income, employment, and consumer prices. This data is frequently used in academic research and financial analysis.
The platform is ideal for building economic models, conducting policy analysis, or forecasting financial trends. If you’re working on projects that require an in-depth understanding of the U.S. economy, AEA is a great starting point.
Top data sets from AEA:
U.S. Macroeconomic Data: Includes GDP, unemployment, and inflation.
National Income Accounts Data: Details on income distribution and economic growth.
Consumer Price Index (CPI): Measures inflation and changes in purchasing power.
Labor Market Data: Information on employment rates and wage trends.
Housing Market Trends: Data on real estate values and mortgage rates.
Global Financial Data (GDF)
Global Financial Data offers extensive historical economic and financial data. It’s perfect for long-term analysis, such as back-testing trading strategies or understanding the impact of major economic events. The data covers financial instruments, economic indicators, and global market trends.
If your projects involve historical modeling or require in-depth financial insights, GDF is a go-to resource. The long-term data can help you uncover patterns and build more accurate predictive models. It’s particularly useful for financial researchers and quantitative analysts.
Popular datasets available through GDF:
Long-Term Stock Market Data: Historical stock price information dating back decades.
Global Economic Indicators: Measures of economic health across countries.
Historical Bond Yields: Data on government and corporate bond performance.
Commodity Price History: Tracks the long-term prices of essential commodities.
Interest Rate Data: Historical trends in global interest rates.
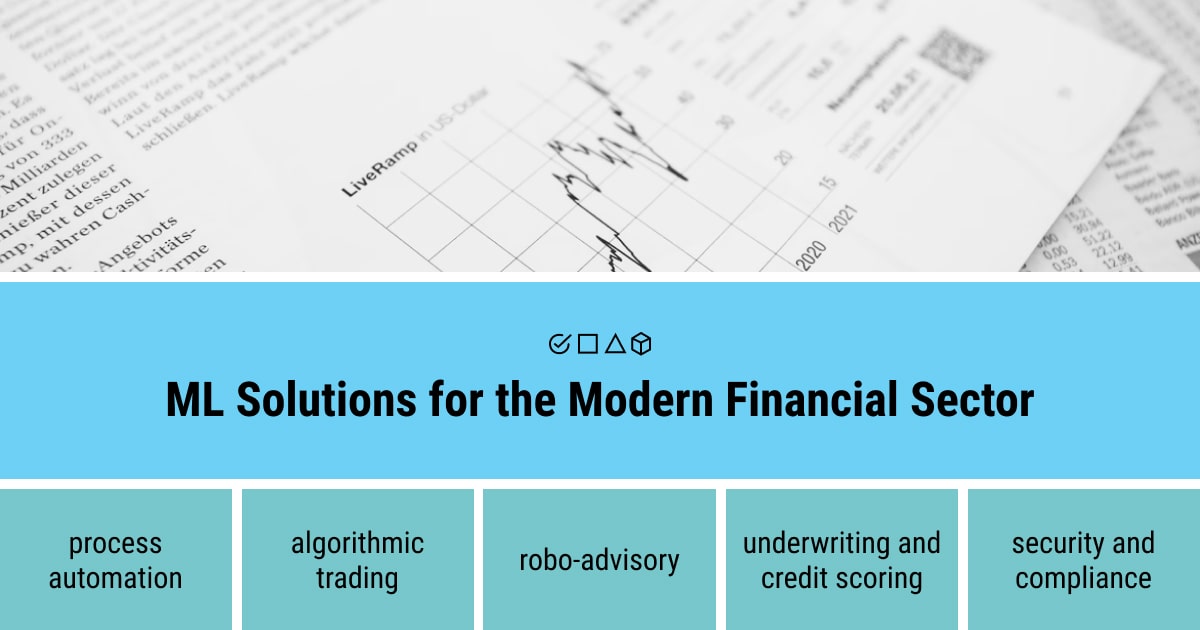
“Accuracy, relevance, and timeliness are key when choosing financial datasets. In finance, even slightly outdated data can lead to inaccurate predictions. Always ensure your data aligns with your model's goals and comes from credible sources.”
President of Tax Crisis Institute, Tax Crisis Institute
How to Stay Updated on New ML Datasets
As an ML engineer, staying ahead means having access to the freshest data for your projects. Subscribe to our blog to get timely updates on new, trending financial data sets that will keep your models performing at their best.
Never miss out on the latest financial data sets! Sign up for our newsletter and keep your ML projects fueled with insights that matter.
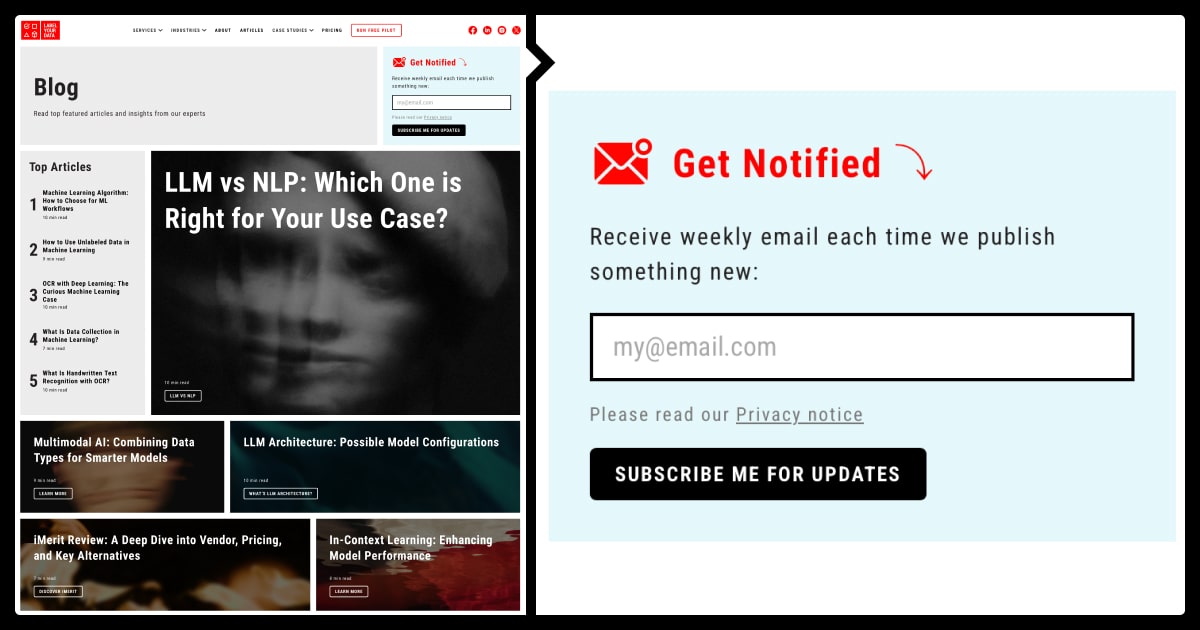
What Data Should Be Labeled in Financial Data Sets
For machine learning models to perform accurately in finance, specific data types must be labeled.
Here are the key categories:
Data Category |
Description |
Labeling Purpose |
Techniques |
Transaction Data |
Payment and transaction info |
Fraud detection |
Anomaly detection, supervised learning |
Financial News |
News articles and social media content |
Sentiment tagging for investments |
NLP, sentiment analysis |
Credit Data |
Customer profiles, credit scores |
Risk classification for loans |
Classification, regression |
Market Data |
Stock prices, trading volumes |
Predictive trading patterns |
Time series, pattern recognition |
Customer Data |
Behavior and transaction history |
Targeted financial products |
Clustering, predictive modeling |
Learn more about our data annotation services in finance and how we can help you prepare financial data sets for machine learning.
FAQ
What is a financial data set?
A financial data set contains information about economic transactions, market trends, asset prices, and other financial activities, which are used for analysis and modeling in finance.
What are examples of finance data?
Examples include stock prices, interest rates, financial transactions, credit scores, and economic indicators like GDP.
Which database is best for financial data?
Kaggle, Quandl, and IMF Data are popular choices for financial data sets, each offering unique data points suited for different financial modeling needs.
Table of Contents
Get Notified ⤵
Receive weekly email each time we publish something new: