Overcoming 5 Common Data Labeling Challenges
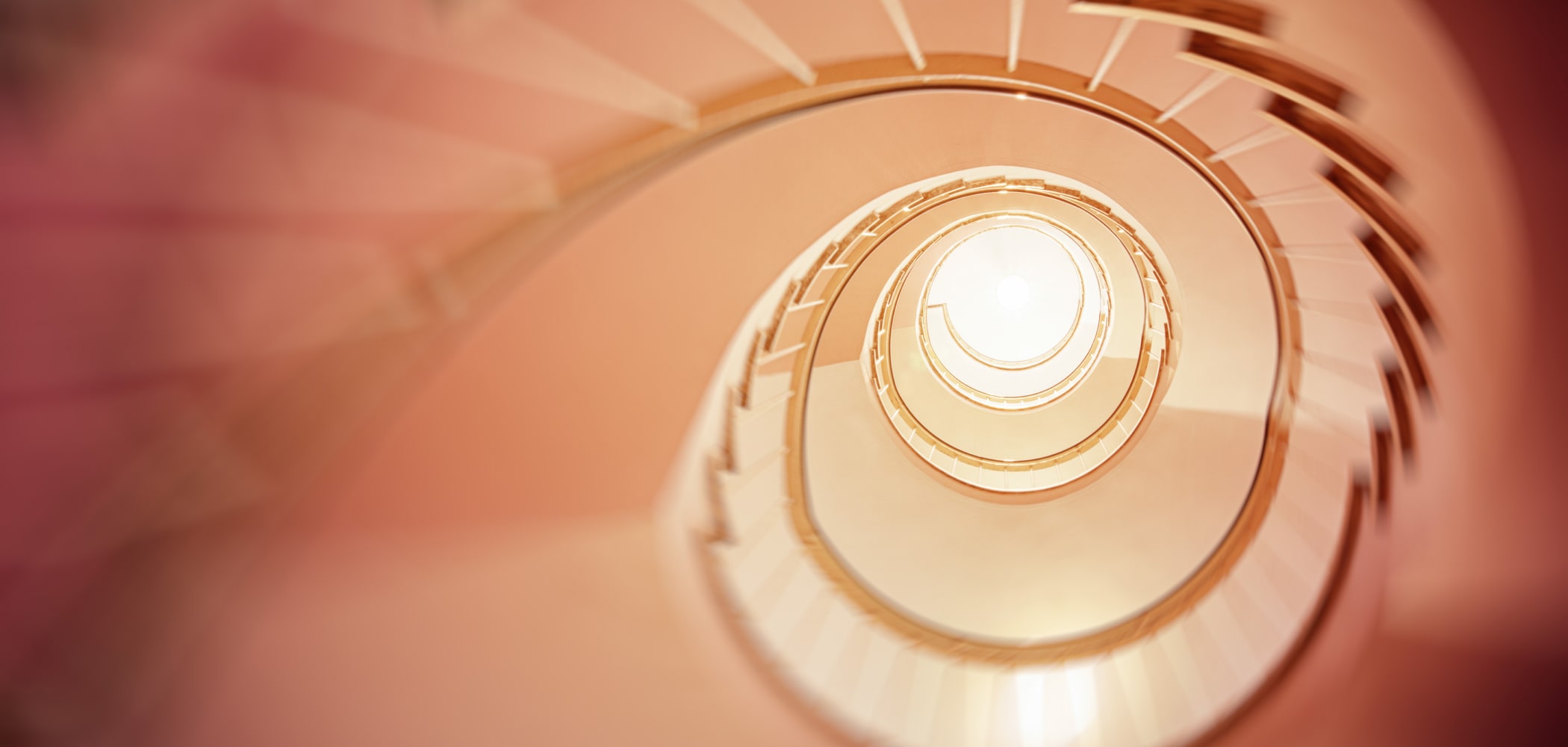
AI propels human progress. But there’s still untapped potential that could be realized if we could address lingering security and data quality issues, as well as inefficient labeling.
Unfortunately, data labeling remains a recurrent issue today due to several reasons. First, it’s the subjective nature of the annotation process. Second, many projects require domain-specific expertise, which may be often overlooked. The third reason is the cost and time associated with labeling, obviously. Last but not least, data annotators typically deal with a vast scale of data and so the accuracy of the final output is often dubious.
Not to mention the continuous generation of new data and the evolution of novel data types and formats. We call them the data labeling bottleneck, but there are some helpful tips on how to avoid them. With that said, let’s explore the most pressing data labeling challenges and our expert tips on how to tackle them.
Top 5 Challenges in Data Labeling Stifling AI Progress
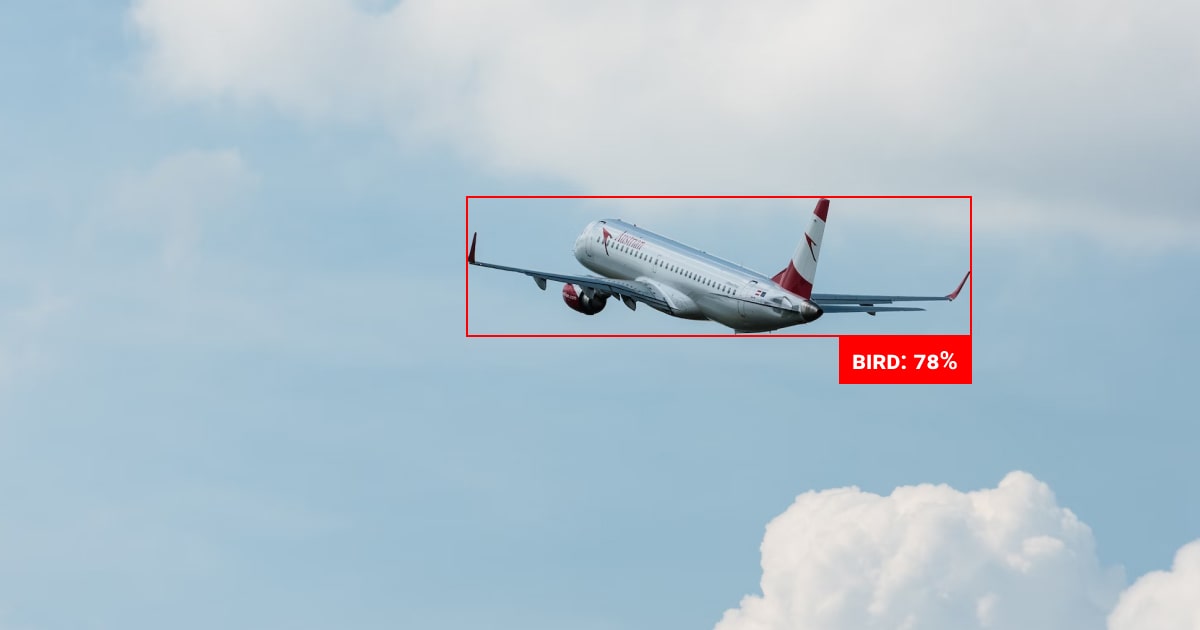
AI development is picking up speed, leading to the emergence of cutting-edge, transformative, and data-driven solutions. Like the recent ChatGPT breakthrough, for example, that made quite a splash in the AI world. However, data labeling, which is a crucial step in training these systems, has remained a significant bottleneck in AI progress.
Having a clear understanding of the key data labeling challenges and how to mitigate them is crucial, as this knowledge has a direct impact on your capacity to build and train robust and reliable machine learning models.
Challenge #1: The lack of data security compliance
The GDPR, DPA, and CCPA are data privacy requirements designed to safeguard the personal data of individuals. Data annotation companies are required to abide by their principles for handling sensitive client data. This means preventing annotators from accessing this data on an unsecured device, transmitting it to an unknown location, or working on it in a public area where it can be viewed by unauthorized persons. For instance, in AI data labeling in MilTech projects, data security is paramount as military data is not only sensitive, but it is also mission-critical.
Solution:
As an expert data annotation service provider, Label Your Data is certified with PCI DSS (level 1) and ISO:27001, and comply with GDPR, CCPA and HIPAA. Such measures help our team to adhere to strict data security and privacy regulations and deliver secure annotations for our clients. Also, managing and storing data on approved devices on-site can be beneficial for handling sensitive information.
Challenge #2: Low dataset quality
Achieving high dataset quality is important, but it’s not always easy. Companies need to make sure that data annotators are capable of producing high-quality results consistently according to the set quality standards. Thus, it’s essential to distinguish between two main types of dataset quality: subjective and objective.
Subjective data quality is when data annotators define which labels to put themselves, as there’s no one definitive source of truth. In this case, the data is interpreted based on the linguistic, geographical, cultural, and other elements associated with annotators performing the task. Objective data, in turn, means that there’s one right answer for each data point. However, the main challenges here are the lack of domain expertise or insufficient guidelines for annotators.
Solution:
The quality of the final labeled dataset depends on the clear and detailed instructions that must be set to guide the entire process. This is also crucial for the data labeling workforce to comprehend each data point correctly. A closed-loop feedback process will help to address the subjectivity and objectivity of the annotated dataset quality.
Challenge #3: Poor workforce management
Inadequate workforce management leads to inability of the annotation team to handle vast amounts of unstructured client data and deliver high quality and security across the data labeling workflow. Therefore, companies need to strike a delicate balance between expanding their workforce and training and supervising a large, diverse group. Some startups and companies have successfully managed their data labeling and other data processing requirements in-house. However, this approach is only feasible when the datasets are still relatively small.
Solution:
With over 10 years of experience managing large annotation teams both on-site and remote, we highly recommend hiring an expert third-party service provider for efficient and accurate data labeling. They offer a larger team of professionals skilled in correct data labeling and help you tackle the challenge of workforce management.
If you lead a data labeling company, provide organized and detailed training for annotators on each project. Distribute tasks based on individual strengths and weaknesses, and track progress while ensuring seamless cooperation between teams.
Challenge #4: Inefficient QA or no QA at all
Among other challenges faced in data labeling is quality assurance, aka QA. In its essence, data annotation is a manual process. This means that at each stage, annotations are constantly being reviewed by human experts, starting from data collection and ending with actual full-scale data labeling. However, since automation has reached this area as well, human involvement is often overlooked.
Solution:
At Label Your Data, not a single project that we work on is without a thorough QA procedure conducted by a specific team of data annotation leads. Data QA requires continuous cross-functional collaboration, since it is a clear plan to follow to address data errors promptly. Manual review of the final annotations is obligatory to deliver outstanding data quality.
Challenge #5: Automation dependence
Automated data annotation is no longer new, but companies often make the mistake of relying too heavily on it to save time and money. However, at Label Your Data, we caution against this approach as it is a sure-fire way to fail in data annotation. This task requires precise algorithms to quickly and accurately label data, without human intervention. However, language interpretation makes it challenging to develop reliable algorithms. Additionally, automated annotation must cope with incomplete or erroneous data, which further complicates the process.
Solution:
To create accurate ML models for automated annotation, large datasets are needed to identify patterns correctly and predict new data labels. Yet, it’s important to remember that automated data annotation cannot fully replace human involvement. Humans offer critical context, domain knowledge, and judgment that automated systems can’t provide. So, it's best to combine automated and human annotation methods to ensure the labels generated are precise and meaningful.
The High Stakes of Poor Data Labeling in AI
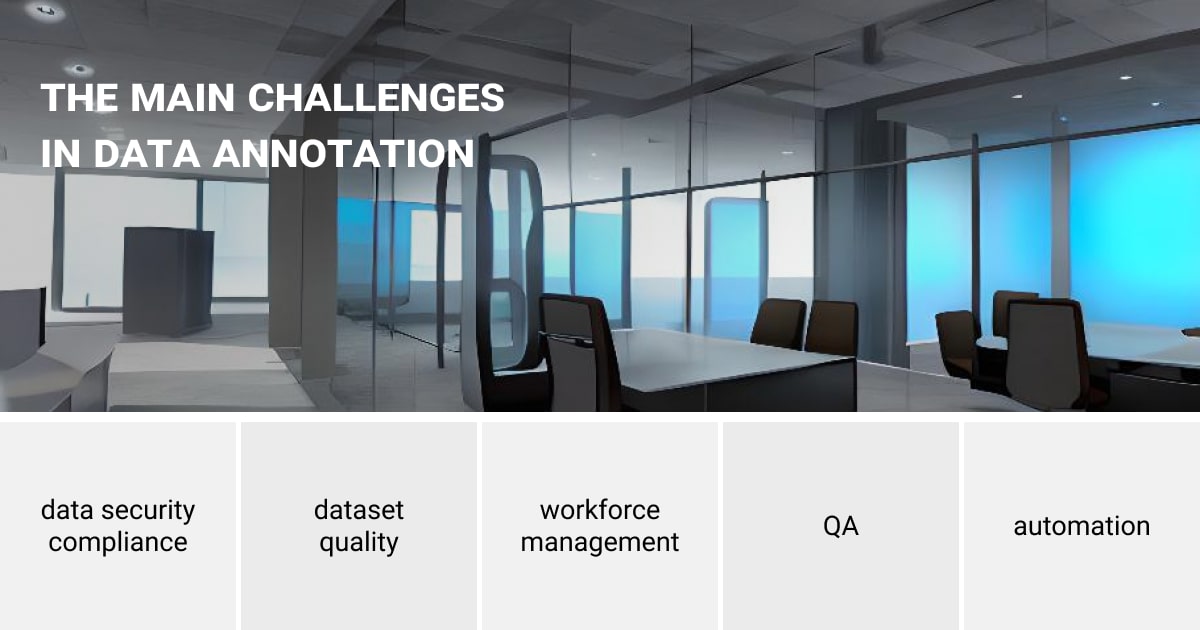
Accurate data labeling is crucial to creating AI models that are fair, accurate, and transparent. Poor quality data can significantly impact an algorithm’s effectiveness and cause losses for a company. Inaccurate labeling can even result in fatal consequences, as with self-driving cars.
Here are a few crucial points to remember:
- Label inconsistency is a major challenge, with conflicting or inconsistent annotations arising from human error, unclear instructions, and subjective labeling.
- Training annotators to follow strict guidelines for each new label, class, and scenario can minimize errors and biases.
- Too much noise and error can harm an algorithm’s performance, making precise labeling essential.
- Quality checks and revisions can ensure clean labels for training, although this process may increase turnaround times.
- Precise labeling is vital for AI performance and positive outcomes for individuals and society.
What’s our approach to overcome data labeling challenges at Label Your Data? We have developed an error-proof data annotation process that addresses all issues that may arise during data labeling. Our team of experts comes from diverse backgrounds, with expertise in various fields such as self-driving cars, AR, retail, and healthcare. To overcome data labeling challenges, we perform multiple rounds of QA until all potential errors are eliminated.
On a Final Note
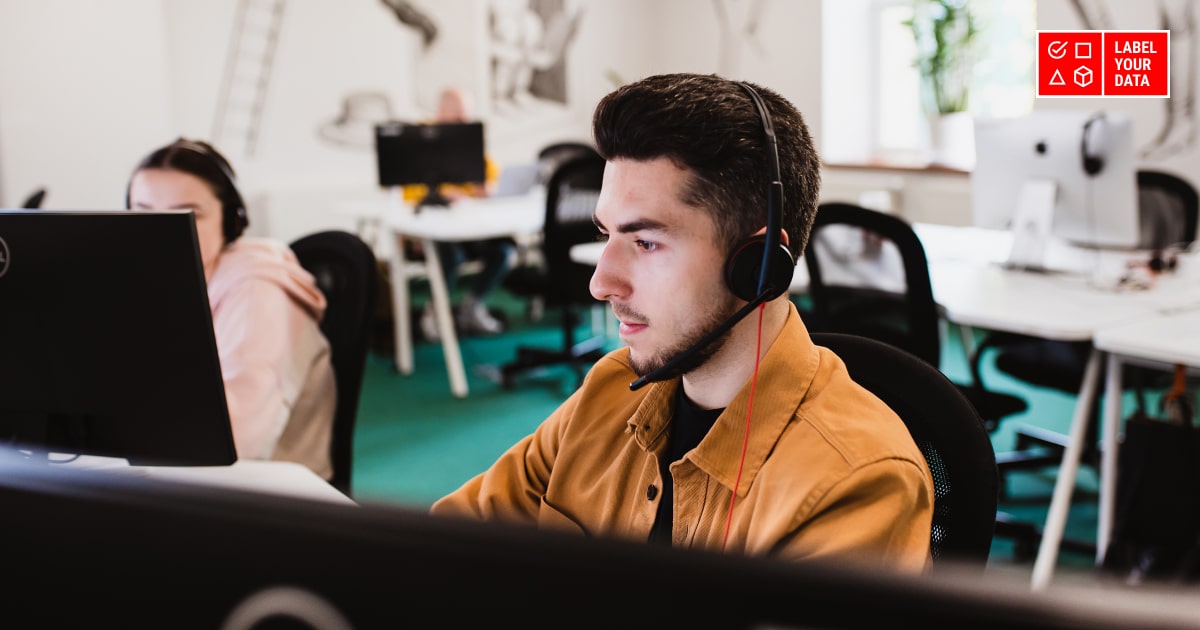
While the development of AI technologies is rapidly advancing, the challenges faced in data labeling continue to hinder progress. Overcoming these challenges requires a thorough understanding of the issues at hand and implementing effective solutions.
In this article, we shared our expert tips and solutions to navigate common data labeling challenges, such as data security compliance, low dataset quality, QA procedures, automation, and poor workforce management. By partnering with a trusted data annotation service provider, like Label Your Data, you can ensure the quality and security of your labeled data, ultimately achieving robust and reliable machine learning models.
FAQ
What are some effective methods for overcoming data labeling challenges in ML?
In general, some useful approaches to address data labeling challenges include using data augmentation, annotation guidelines, human-in-the-loop techniques, and automated labeling.
What are the main challenges in automated data labeling?
The main challenges in data labeling, which has been automated, include label noise, class imbalance, and ensuring that the labeling process is accurate and consistent.
What recent advancements in data labeling have improved this process?
Advancements such as active learning, human-in-the-loop techniques, and transfer learning have improved the labeling process in machine learning by reducing the required amount of labeled data and overcoming challenges in data labeling.
How data labeling challenges impact the accuracy of ML models?
Challenges in data labeling can significantly impact the accuracy of ML models, as they can result in incorrect or inconsistent labeling, leading to poor training and prediction performance.
Written by
One of the technical writers at Label Your Data, Yuliia has been gradually delving into the intricate aspects of AI. With her strong passion for the written word and technical expertise, Yuliia has developed a keen interest in the evolving field of data annotation and the power of machine learning in today's tech-savvy world. Check out her articles to learn more about the complex world of technology and find the solutions that work best for your AI project!