What is Data Quality, and Why Should We Care?
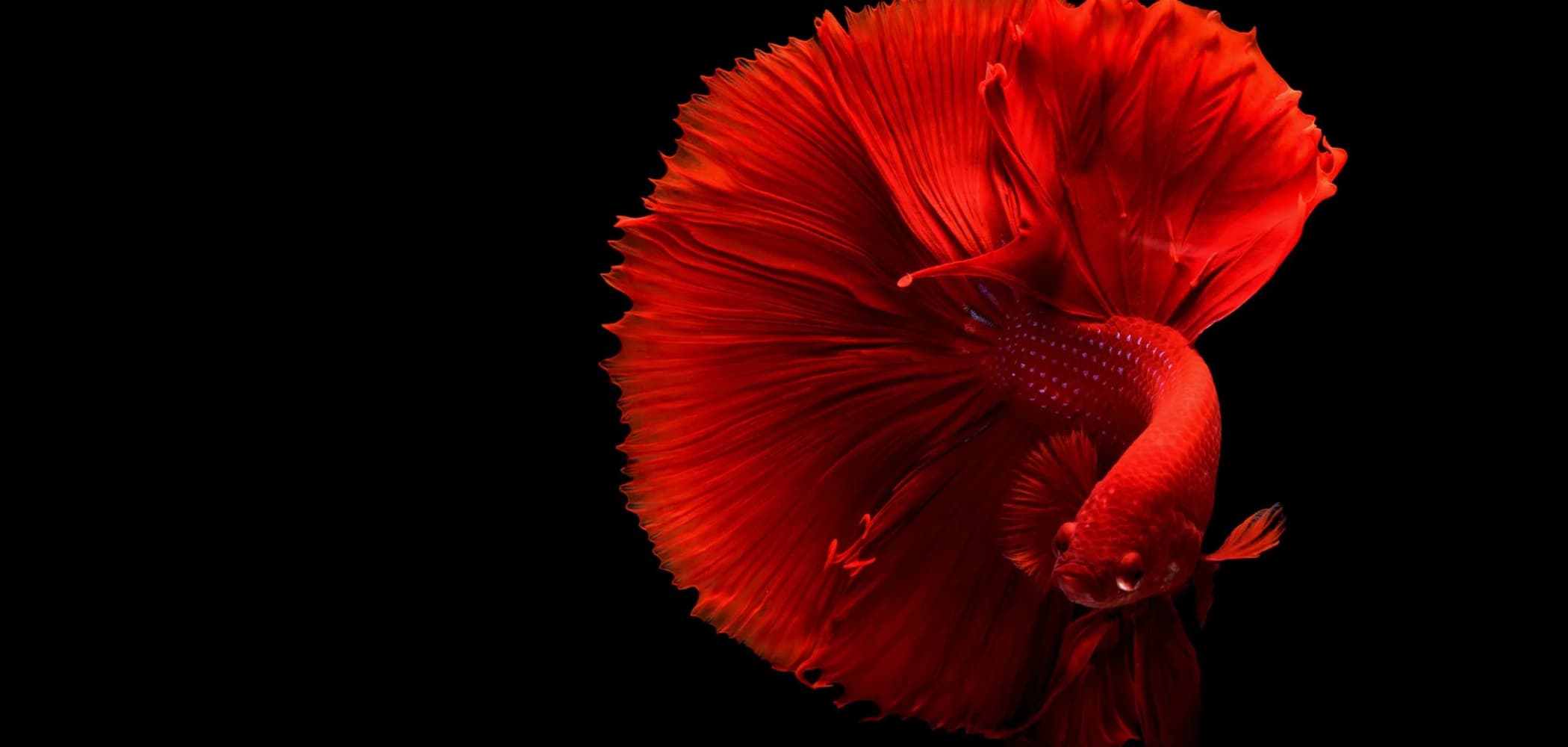
Data is the most valuable currency in today’s digital economy and humanity’s most powerful asset to own, as noted by The Economist. Yet, it’s only powerful when used wisely.
Sophisticated AI-powered systems that we build are based on data. Thus, good data ensures that these systems will generate accurate results and can be used for something truly revolutionary. Having the right data on hand is the only way to build complex models and technologies that we can trust and benefit from.
Data quality refers to the capacity of data to meet an organization’s specified business, system, and technological criteria. It’s an assessment of whether data is appropriate to fulfill a certain function in a given context. However, proper data quality management is a complex skill that not all businesses can master. In fact, poor data quality impedes almost 40% of all business initiatives from achieving their objectives, according to Gartner. Individuals and organizations that rely on data will pay a high price if they don’t maintain the quality of their data.
For such businesses, improving data literacy and committing to high-quality data quality is the best way to avoid the negative consequences of inadequate data quality control. And it starts with a thorough grasp of data, its fundamental components, and what constitutes high-quality data.
We at Label Your Data have gathered the most up-to-date information on current approaches to proper data quality management, seasoned with our years-long data experience. Read on to learn the secrets of high-quality data!
The Growing Demand for Quality Data Practices and Knowledge
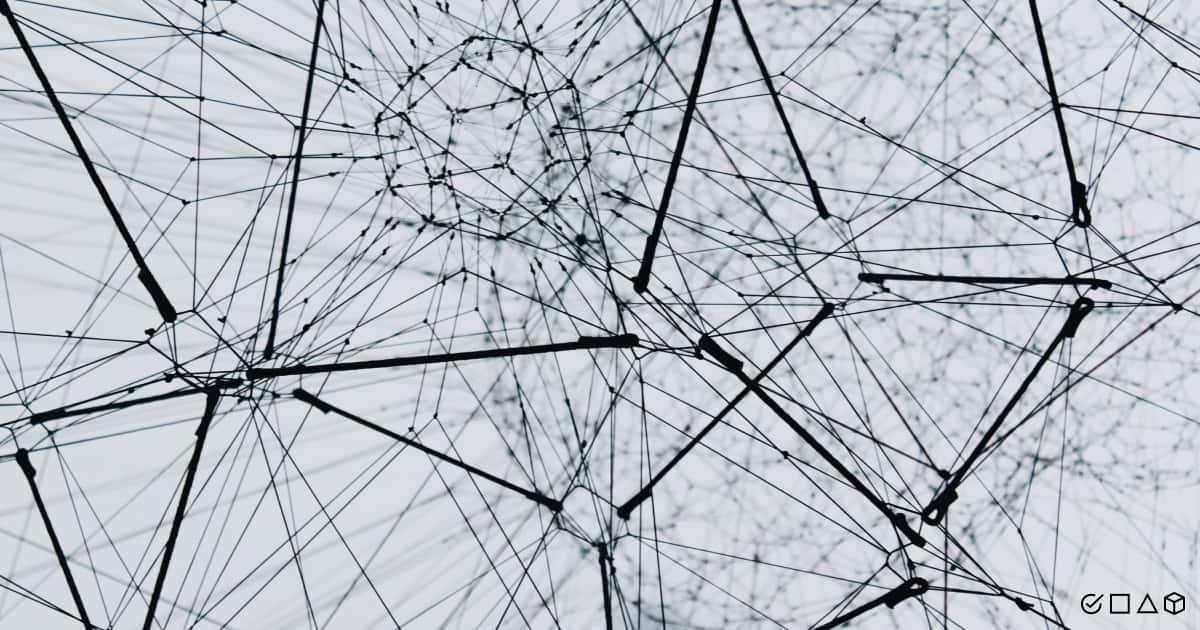
Ideas in artificial intelligence simply don’t work unless they’re based on high-quality data. What makes the data quality?
Before delving into the fundamental features of data quality, it’s important to first understand what the data represents. It never hurts to learn more about data, right? Plus, it’s impossible to establish an organizational commitment to high-quality data practices without knowing the basics.
There are many different categories of data, yet the most important types include master data, reference data, historical data, transactional data, and metadata (data about data). But whatever type of data is used, it still represents the same thing and plays an equally pivotal role for every organization. So, what is data? Data is:
- An asset
- A meta-asset
- An operational necessity
- A sort of organizational expertise
But also, data is a risk. More specifically, poorly managed or unmanaged data is a risk for most data-driven businesses. That’s why learning more about how to achieve quality data and maintain it across the business operations is key to success in today’s AI-driven, data-dependent world.
Having trouble with your data? Don’t hesitate to send your data to our team of expert data annotators, and we'll make a free pilot, customized specifically for your AI project!
How to Measure Data Quality and Why?
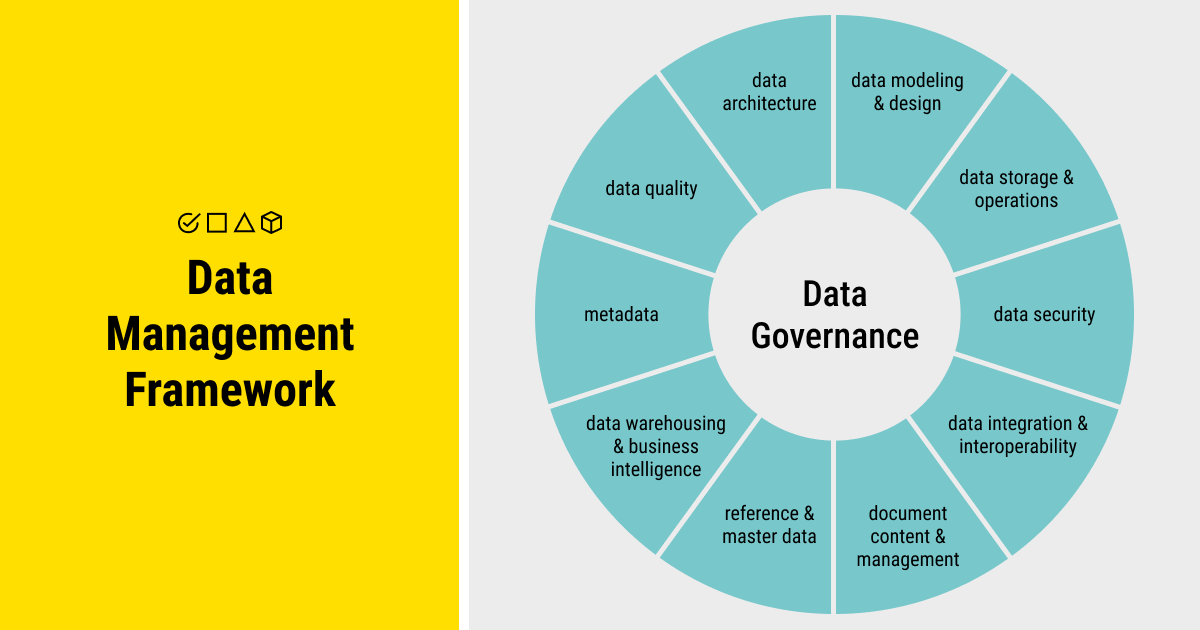
The rising need for high-quality data strategies goes hand-in-hand with ever-increasing volumes of data. There is so much data in the modern tech-oriented environment, which makes it even more challenging to maintain the quality of this data. However, measuring and maintaining good data is important, since the inability to do so is both costly and harmful for individuals and businesses.
What are the primary obstacles to putting good data quality management practices in place? First, it’s the differences in the meaning and interpretation of data. Second, it’s the failure to understand that data quality directly impacts the quality of the process based on this data. Third, it’s the lack of proper data-technology balance, as well as people factor and their inadequate data literacy. The final challenge to proper data quality practices is the culture of an organization that fails to establish leadership and accountability along the data supply chain.
What’s the solution to these challenges? The ultimate goal is to know data-as-data and be aware of all the processes and advanced technologies through which data is created, processed, and deployed. Data literacy and team communication are of the utmost importance here.
— Data Quality Improvement Cycle
Assessment ⟳
Work with your actual data and environment. Compare them to the main requirements and future expectations from the operations based on your data.
Awareness ⟳
Examine the true state of your data and its potential impact on business. Learn the underlying causes of the existing problems with data.
Action ⟳
Prevent yourself from future information and data quality issues, and deal with current data errors. Repeat the steps until you reach the desired state of high-quality data for your business objectives.
— Data Quality Dimensions
There’s a big family of data quality dimensions or metrics that help measure different aspects of the same data in accordance with the project’s goals and technical limitations. Let’s see what they are!
- Completeness: A dimension that measures whether the data is present (non-blank values in data) or absent (null or blank values; the value is missing). There’s schema completeness, column completeness, and population completeness.
- Conformity/Validity: A measure of how well the data acts according to internal and external standards, guidelines, or standard definitions.
- Consistency: A dimension that refers to whether the same data stored in different places does or doesn’t match. A measure of the degree of uniformity of data as it moves across the network or between applications on a computer. Data values in one dataset must match the values in the other datasets.
- Integrity: Captures the relationships between data objects, the validity of data across these relationships, and the guarantee that the data can be traced and connected to other related data.
- Accuracy: A measure of how well the data reflects the real-world scenario and how correctly it can describe the reality; a measure of correctness of the content.
- Correctness: Similar to accuracy. The characteristic of whether the data is free of errors or mistakes.
- Granularity: A measure of the level of detail in a data structure.
- Precision: A degree of detail of the measurement and thoroughness of description for a data element.
- Accessibility: A measure of the extent to which data or metadata is provided and shared in open formats, as well as the suitability of the data.
- Time-related dimensions: Timeliness, volatility, and currency/freshness of data.
Remember, data quality dimensions work together. Having multiple dimensions helps organizations make better data-driven choices about where the time and effort should be devoted to. Only by using several dimensions at a time, one can understand what can be learned from the various data quality evaluations.
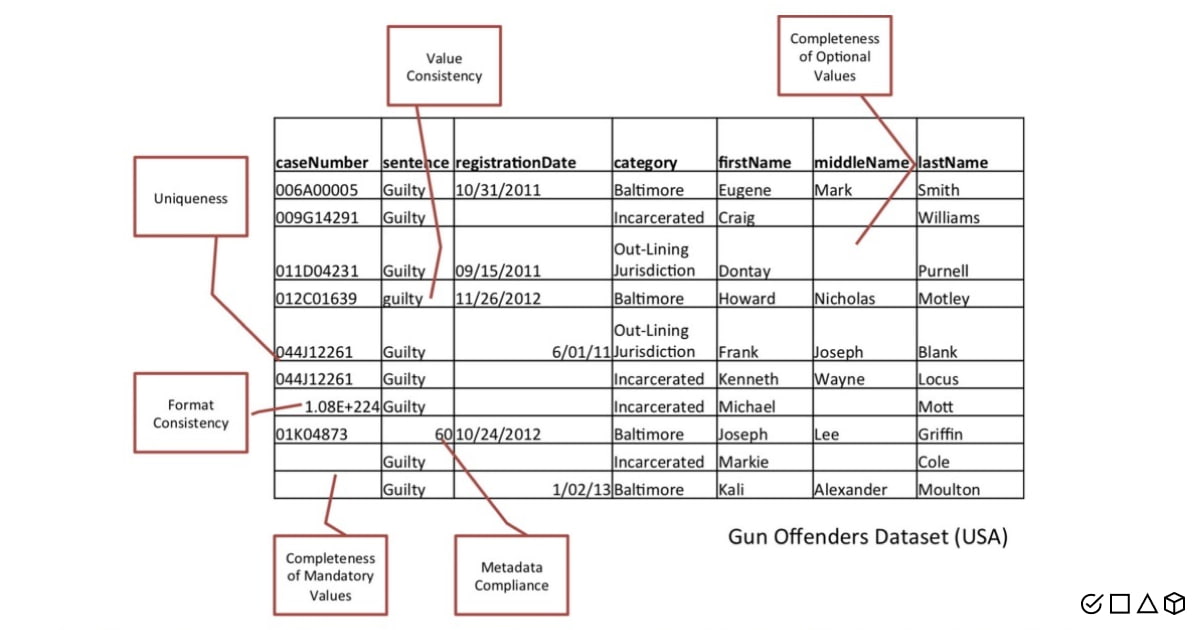
— Data Quality Management Practice
Simply put, good data provides critical insights about markets, consumers, and employees. It also drives corporate success. So, having proper data quality management is required to help companies achieve success and avoid losing costs, time, and other valuable resources.
Among the plethora of approaches to data quality management, we’ve identified the most effective, in our view, methods for tackling the pressing problem of low-quality data. Here are ten steps that will gradually lead organizations to high-quality, trustworthy data that they can use for their business initiatives:
- Clearly define the most important business needs and data governance to know where to concentrate your efforts.
- Describe and analyze the information environment.
- Evaluate the quality of your data and establish rules.
- Assess the business impact of your data, especially of poor-quality data.
- Understand and detect the root causes of data quality issues and their influence on your business.
- Develop data improvement plans and integrate the data quality rules.
- Prevent future data errors and defects.
- Correct current data errors and defects.
- Continuously monitor data quality controls.
- Communicate, regulate, and engage the right people throughout the data quality improvement cycle.
A robust data quality management practice is an end-to-end strategy to define needs, as well as identify and resource the necessary data components. Also, this implies continuous monitoring of data quality, all while integrating and promoting data quality in the company.
— Data Quality Tools and Software
Data-related issues in companies fall under the purview of data quality managers, analysts, and engineers. They are the ones responsible for fixing data errors (e.g., data drift and anomalies) and other data quality problems. Data quality managers find these errors and clean poor-quality data in the company databases or other data repositories. But they don’t work alone. Data quality managers enlist the support of other specialists, such as data stewards and data governance program managers.
To make their job easier, there are many great solutions and data quality monitoring tools that help optimize the main dimensions of data quality. These tools detect, understand, and correct data flaws so that businesses can make better decisions and lead more effective information governance across all operations. What are the best data quality tools on the market?
- IBM InfoSphere QualityStage
- IBM BigQuality
- Informatica Master Data Management
- OpenRefine
- SAS Data Management
- Oracle Cloud Infrastructure Data Catalog
- Precisely Trillium
- Talend Data Quality
- TIBCO Clarity
- Validity DemandTools
- Cloudingo
- Data Ladder
These data quality tools perform a wide scope of functions, including profiling, parsing, standardization, cleansing, matching, enrichment and monitoring. However, there’s a common belief in the corporate world that data quality is the responsibility of everyone in the organization. Everyone in the company must prioritize data because it keeps their work and business alive. Good business choices are made based on quality data.
To ease the burden of finding, analyzing, and using various data analysis tools, you can simply contact our team at Label Your Data and let the professionals do the work!
What is Data Quality Assurance?
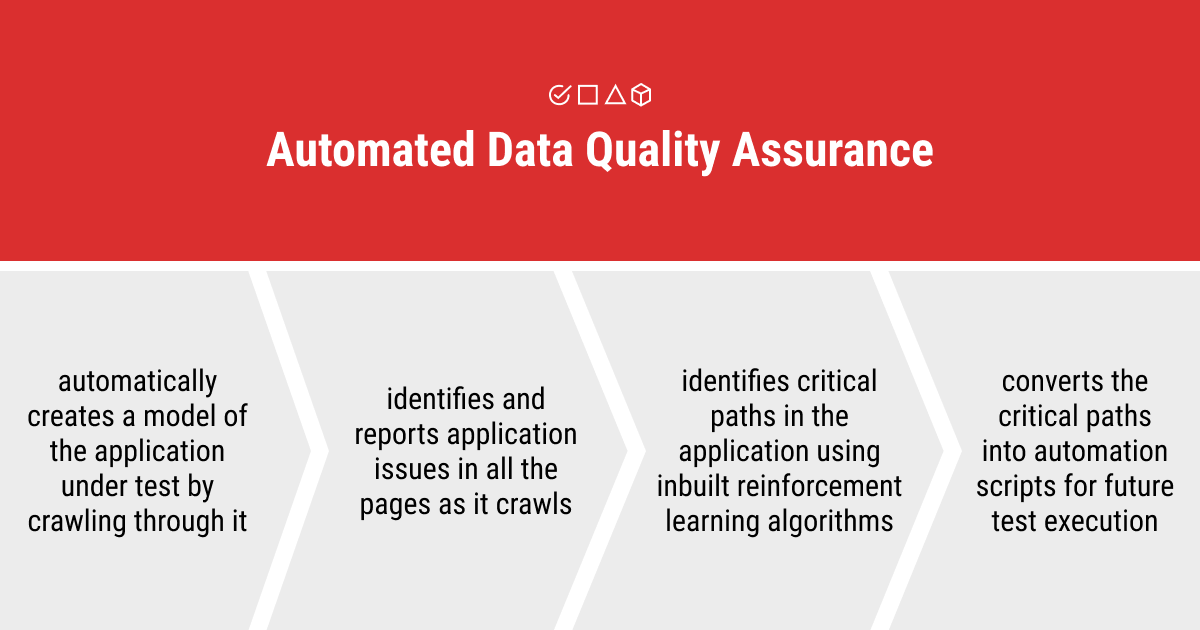
Data quality assurance refers to the process of identifying and screening anomalies by virtue of data profiling, obsolete data removal, and data cleaning. That said, the organization-wide quality assurance (QA) strategy must include corporate data governance measures and technical interventions in order to protect and maintain the value of data.
What’s the key to proper data quality assurance? First and foremost, it requires a certain skill set and human resource capacity to establish adequate supervision and accountability within an organization. Second, data collectors and processors must have access to complete documentation of operational procedures. Data QA is a clear strategy to follow to respond to data issues on time and, therefore, needs cross-checking techniques performed on a regular basis. Last but not least, this strategy isn’t complete without sufficient financial and logistical resources, which guarantee that work is completed on time.
Artificial Intelligence in Data QA
Like any other approach to modern sophisticated systems and technologies, everything becomes much simpler with AI. The same story is true for data quality assurance. Artificial intelligence is used to enhance the automation capabilities of smart data analysis in real-time, agile testing, and third-party system integrations.
With many ML algorithms available today, companies can perform better pattern analysis of their data and process large volumes of data. As a result, they can make better decisions, optimize data analysis, and prevent the risks of business failure.
Summary: A Paradigm Shift Towards Quality Data in AI
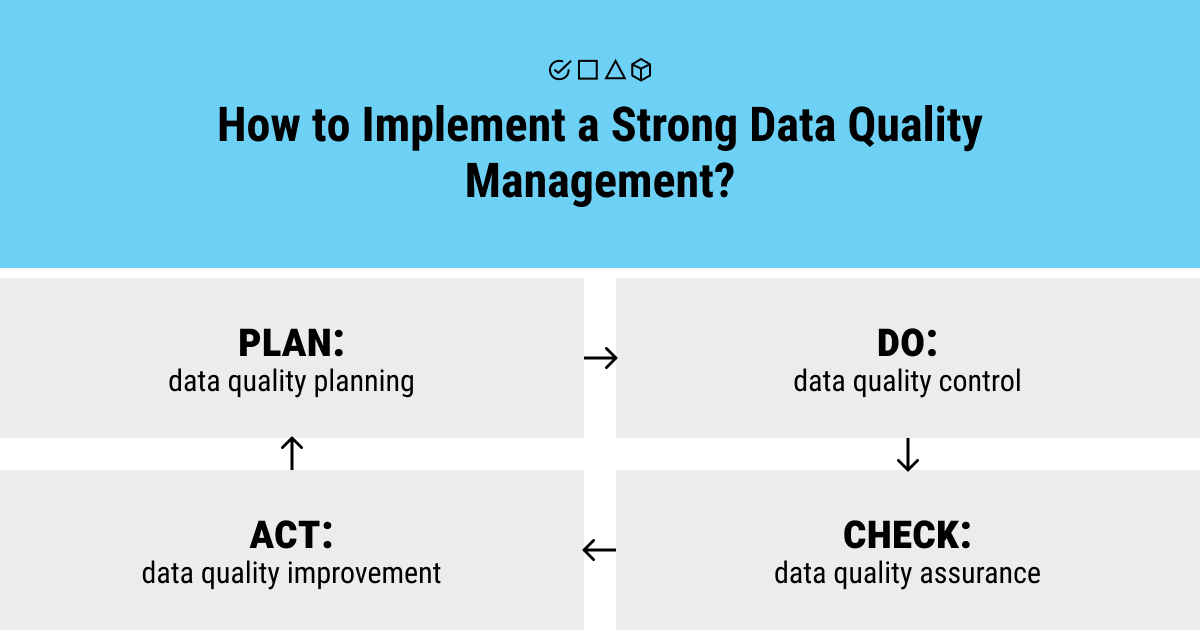
Data, as we all know, is notoriously prone to error. In business settings, if data fails to meet quality standards, corporate decision-making will be inaccurate, leading to untold downstream costs and missed opportunities. Why should we care about data quality?
Today, investing in data quality is a cost-effective strategy to avoid bad decisions and the loss of incalculable expenses and resources. Low-quality data is costly for companies, so it’s crucial to develop and nurture data competency across the business units to achieve robust data quality.
We hope that our short yet detailed overview of the basic principles behind high-quality data will help your business thrive and prevent such negative consequences of bad data. And don’t forget that you can always contact our Label Your Data team to unlock new opportunities with high-quality, secure annotated data.
Written by
One of the technical writers at Label Your Data, Yuliia has been gradually delving into the intricate aspects of AI. With her strong passion for the written word and technical expertise, Yuliia has developed a keen interest in the evolving field of data annotation and the power of machine learning in today's tech-savvy world. Check out her articles to learn more about the complex world of technology and find the solutions that work best for your AI project!