ChatGPT Data Annotation: How to Automate Text Annotation
Table of Contents
- Why Use ChatGPT for Text Annotation?
-
How to Set Up ChatGPT for Text Annotation Step-by-Step
- Define the Annotation Task
- Select the Appropriate GPT Model
- Infrastructure and API Setup
- Craft High-Quality Prompts
- Utilize Temperature Settings
- Incorporate Domain-Specific Language
- Implement Iterative Feedback Loops
- Leverage Ensemble Methods for Complex Tasks
- Monitor and Adjust Based on Task Complexity
- Optimize for Cost and Scalability
- Effective Prompt Engineering for ChatGPT Data Annotation
- ChatGPT Data Annotation Strategies for NLP Tasks (Prompt Examples Included)
- Challenges and Mitigation Strategies
- Future Directions for ChatGPT Data Annotation
- Final Take
- FAQ
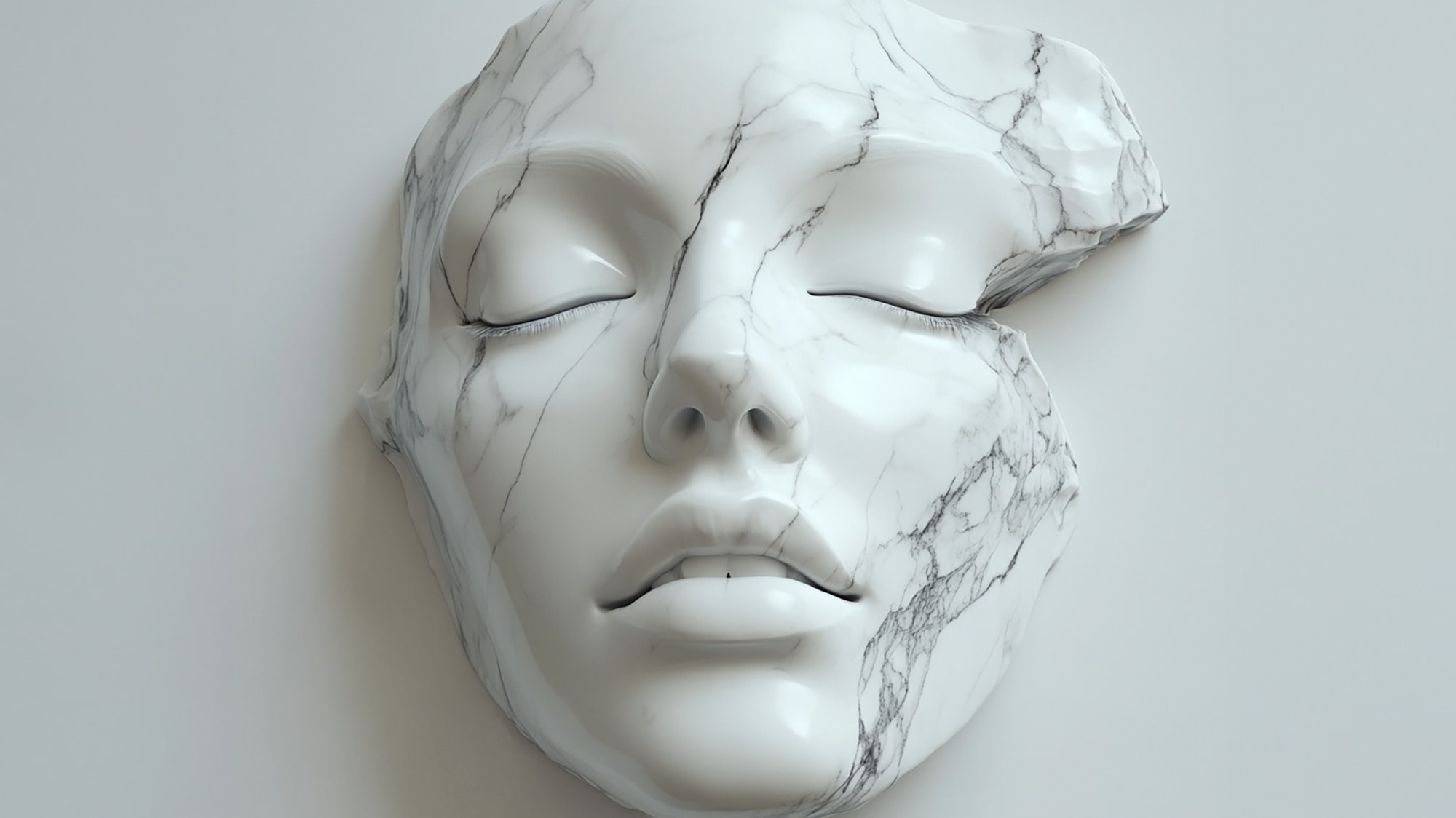
ChatGPT, a top-tier model by OpenAI with 175 billion parameters, continues to lead the AI pack. The chatbot excels in understanding human language and context. Its capability to generate coherent, context-aware text makes it perfect for annotations across various NLP tasks.
This article explores how ChatGPT data annotation automates and optimizes the text annotation process for machine learning projects.
Why Use ChatGPT for Text Annotation?
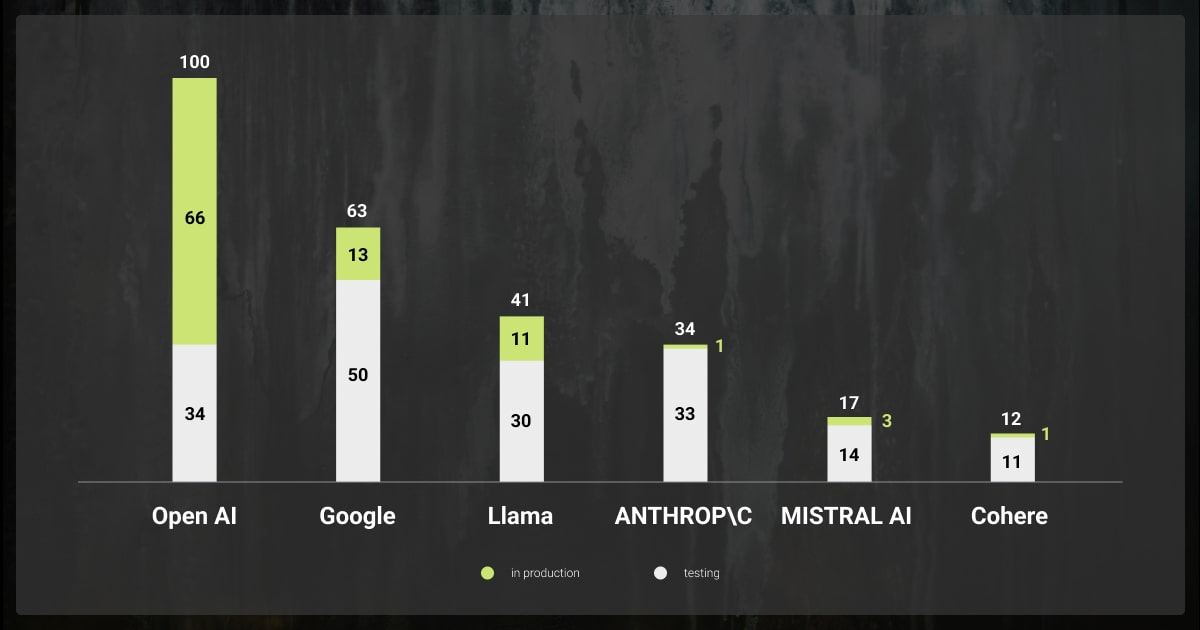
According to Statista, 38% of companies using generative AI report a 40% reduction in manual data annotation time, while 45% see a 30% cost savings. These advancements stem from ChatGPT’s ability to automate repetitive annotation tasks. This, in turn, enables businesses to process large datasets with consistent accuracy and scalability.
Moreover, this trend highlights the growing importance of integrating AI-driven solutions like ChatGPT into data-intensive workflows, particularly in the e-commerce, finance, and healthcare sectors. Businesses are increasingly adopting ChatGPT due to its ability to enhance annotation speed and accuracy.
Annotation teams can leverage ChatGPT’s advanced natural language understanding (NLU). It reduces the manual workload by automating annotation tasks. This shift is driven by the demand for more scalable and cost-effective solutions.
ChatGPT has become a strategic asset for data annotation, helping organizations manage large volumes of data with improved accuracy and reduced operational costs.
Current Research on ChatGPT Data Annotation Capabilities
This study underscores the potential of ChatGPT as a highly efficient and cost-effective tool for text annotation. The chatbot’s capabilities often surpass those of traditional crowd-sourced methods.
Here’s what we learned:
Performance Superiority: ChatGPT outperformed crowd workers on platforms like MTurk in tasks such as relevance, stance, and topic detection, with zero-shot accuracy averaging 25% points higher.
Intercoder Agreement: ChatGPT achieved higher intercoder agreement, with 91% at a default temperature of 1 and 97% at a lower temperature of 0.2, compared to 79% for trained annotators and 56% for crowd workers.
Cost Efficiency: ChatGPT’s cost per annotation was around $0.003, making it approximately 30 times cheaper than using MTurk.
Task Complexity: ChatGPT excelled in simpler tasks and had a more significant advantage over MTurk in complex tasks where human annotators struggled.
Limitations: In one case with 2023 tweets, ChatGPT struggled with relevance classification due to a lack of examples, highlighting the importance of prompt quality rather than memorization.
Temperature Settings: Lower temperature settings improved annotation consistency without compromising accuracy.
However, to fully capitalize on ChatGPT’s capabilities for text annotation, it’s crucial to understand how to set it up for efficient text annotation.
How to Set Up ChatGPT for Text Annotation Step-by-Step
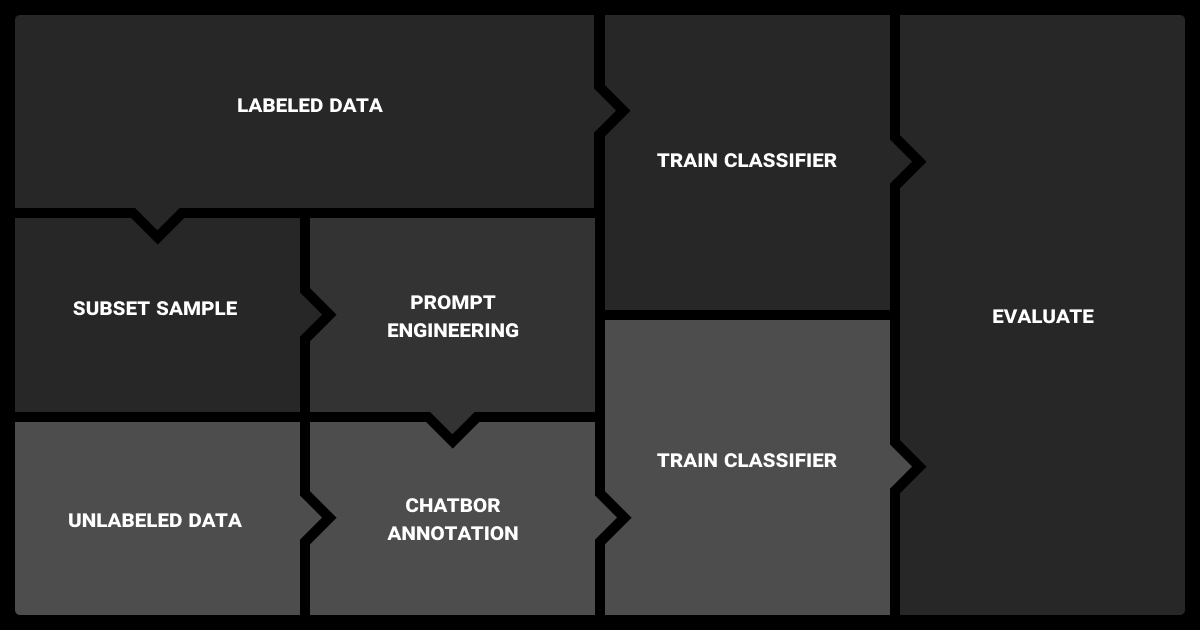
Setting up ChatGPT for text annotation requires a strategic approach that leverages generative AI capabilities and the best data annotation practices.
Here’s how to do it:
Define the Annotation Task
Whether it’s sentiment analysis, entity recognition, or topic classification, a well-defined task ensures that the model’s outputs align with your goals. Breaking complex tasks into simpler sub-tasks can help ChatGPT produce more accurate and consistent results.
Select the Appropriate GPT Model
GPT-4 offers superior language understanding and generation capabilities compared to GPT-3.5, making it more suitable for complex annotation tasks. However, GPT-3.5 might be sufficient for simpler tasks and offers cost savings. Assess the specific needs of your annotation project—such as the complexity of the text and the level of detail required—before selecting the model.
Infrastructure and API Setup
Configure your API access to OpenAI’s models, integrating them into your data annotation pipeline. Make sure your computational resources can handle the demands of large-scale annotation tasks. We suggest automating parts of the pipeline where possible, such as batching requests or using queue systems to manage API calls efficiently.
Craft High-Quality Prompts
The quality of prompts directly impacts the accuracy of annotations. Prompts should be clear and concise, and examples should be included when possible. For instance, if you’re annotating for sentiment, a prompt like [“Classify the sentiment of the following sentence as positive, negative, or neutral”] followed by a few example sentences can guide ChatGPT to produce better annotations.
Utilize Temperature Settings
Temperature settings in ChatGPT control the randomness of the output. Lower temperatures (e.g., 0.2) result in more deterministic and consistent responses. This is ideal for annotation tasks where consistency is critical. However, higher temperatures might be helpful in cases where creative or varied outputs are needed. Experiment with temperature settings to find the optimal balance between creativity and consistency for your specific task.
Incorporate Domain-Specific Language
For tasks requiring domain-specific knowledge, fine-tuning ChatGPT with domain-relevant data can significantly enhance performance. Use domain-specific datasets to train or fine-tune the model, ensuring the language and context used in annotations are accurate and aligned with industry standards.
Implement Iterative Feedback Loops
Regularly review and refine the annotations generated by ChatGPT. Human annotators or domain experts should validate the outputs and provide feedback to fine-tune the model further. This process improves accuracy and helps identify and mitigate any biases in the model’s outputs.
Leverage Ensemble Methods for Complex Tasks
For more complex annotation tasks, consider using an ensemble of models, including ChatGPT, to cross-validate and enhance the accuracy of annotations. This approach combines the strengths of multiple models, leading to more robust and reliable annotations.
Monitor and Adjust Based on Task Complexity
ChatGPT’s performance can vary depending on the complexity of the annotation task. For simpler tasks, ChatGPT may excel with minimal configuration. However, more complex tasks may require more detailed prompts, lower temperature settings, or additional training. Closely monitor the model’s performance and adjust as needed to optimize results.
Optimize for Cost and Scalability
While ChatGPT offers cost-effective annotation, balancing quality with efficiency is essential. We suggest batch-processing large datasets and using automated validation tools to handle high volumes of annotations. This ensures the setup remains scalable without compromising accuracy.
Enhance the quality of your datasets with human annotation tailored to your specific needs. Run a free pilot and see for yourself!
Effective Prompt Engineering for ChatGPT Data Annotation
Understanding how to design prompts for ChatGPT effectively can enhance your data annotation process.
Here’s a short guide to help you get started:
Define Objectives: Start by clarifying what you want the model to accomplish. Whether labeling sentiment, categorizing content, or generating specific types of annotations, having a precise objective will guide the prompt's structure.
Be Explicit with Instructions: ChatGPT works best with clear and explicit instructions. Instead of vague prompts like "Label this text," use specific instructions like "Identify the sentiment in this sentence as positive, negative, or neutral."
Provide Context: Sometimes, the task requires more context for the model to perform accurately. If you're annotating customer service interactions, include relevant background information in the prompt to help the model understand the nuances of the conversation.
Iterate and Refine: Prompt engineering is an iterative process. Start with a basic prompt, review the outputs, and refine the prompt to improve the accuracy of the annotations. Minor adjustments can make a significant difference in the quality of the results.
Test with Diverse Data: Ensure your prompts are robust by testing them across a diverse dataset. This helps identify any biases or inconsistencies in the model's responses, allowing you to refine the prompts further.
Following these guidelines, you can optimize ChatGPT for data annotation tasks, ensuring the outputs are accurate, consistent, and aligned with your project's goals.
ChatGPT Data Annotation Strategies for NLP Tasks (Prompt Examples Included)
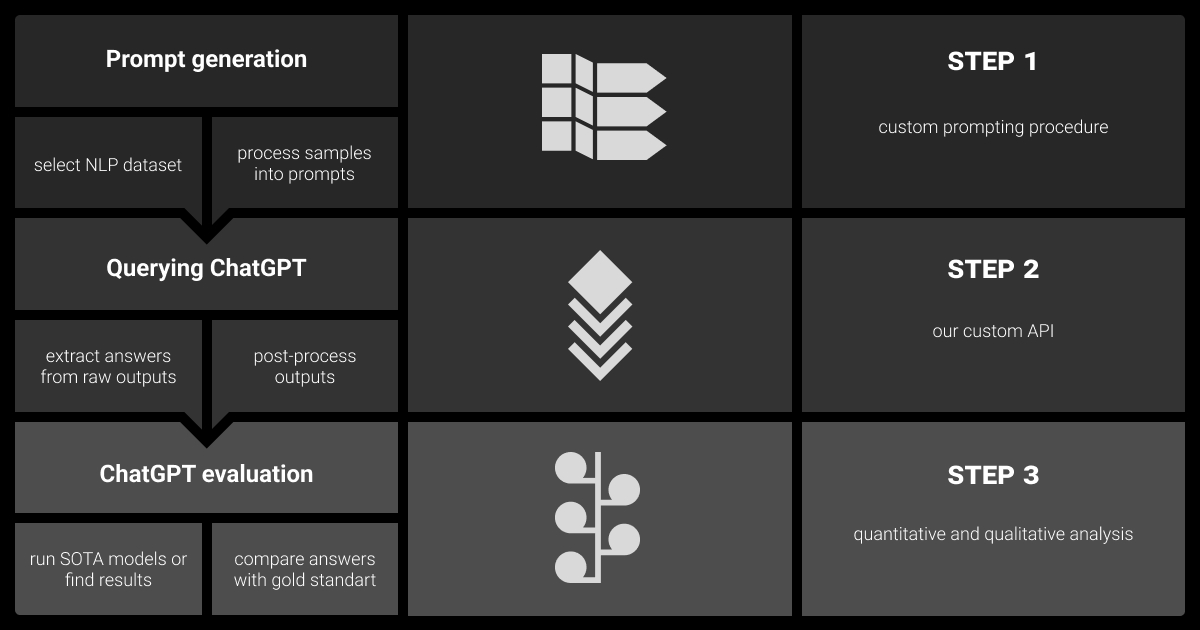
Here’s how to set up and optimize ChatGPT for different types of text annotation:
Sentiment Analysis
For sentiment analysis, define clear criteria for positive, negative, and neutral sentiments. Craft prompts that explicitly ask ChatGPT to classify the sentiment of a given text. Examples within the prompt can help the model produce consistent and accurate labels. Lowering the temperature setting (e.g., 0.2) can further enhance consistency across similar inputs.
Prompt example:“Classify the sentiment of this sentence as positive, negative, or neutral.”
Entity Recognition
To optimize ChatGPT for this task, fine-tune the model with domain-specific data, ensuring it understands the context in which entities appear. Specific prompts help the model deliver precise annotations, especially with specialized domains like healthcare or finance.
Prompt example:“Identify all organizations mentioned in the following text.”
Topic Classification
When using ChatGPT for topic classification, it's important to establish well-defined categories to reduce ambiguity. Start with prompts that ask the model to classify the text into broad topics, and consider using hierarchical prompts that narrow down to subcategories in a second pass. A prompt can be followed by a more detailed classification if needed. A moderate temperature setting (around 0.5) can be used to allow for some flexibility while maintaining coherence.
Prompt example:“Classify this text as related to technology, healthcare, or finance.”
Relevance Detection
For relevance detection, define what makes content relevant, somewhat relevant, or irrelevant within the context of your project. Prompts should clearly articulate the relevance criteria. To ensure accuracy, consider using ensemble methods combining multiple outputs to validate relevant decisions.
Prompt example:“Determine whether the following paragraph is relevant to the topic of climate change.”
Text Summarization
Text summarization requires the model to condense information while retaining the essential meaning. Set up ChatGPT with prompts that ask for a summary of a given text, providing an example of a well-crafted summary to guide the model. A prompt followed by an example summary can lead to more focused and coherent outputs. Lower temperature settings will keep the summaries concise.
Prompt example:“Summarize the following article in two sentences.”
Stance Detection
Stance detection involves determining whether a text expresses support, opposition, or neutrality toward a specific statement or topic. Prompts should be straightforward, asking ChatGPT to identify the stance. Fine-tuning the model with stance-specific datasets can improve its ability to detect subtle nuances in stance expression.
Prompt example:“Does this statement express support, opposition, or neutrality towards the new policy?”
Frame Detection
Frame detection identifies the underlying perspective or angle from which a topic is presented. To optimize ChatGPT for this task, use multi-turn prompts to determine the general topic and specify the frame. Fine-tuning the model on datasets that include annotated frames can enhance its ability to detect and categorize different frames accurately.
Prompt example:“Identify the frame used in this discussion about environmental policy.”
Applying these tailored strategies allows you to use ChatGPT effectively for various text annotation tasks.
Challenges and Mitigation Strategies
While ChPT is a powerful tool for data annotation, several challenges may arise:
Inconsistency in Annotations
ChatGPT may produce inconsistent results, especially in complex tasks.
Mitigation: Use clear, well-structured prompts and lower temperature settings to enhance consistency. Regularly review outputs and provide iterative feedback to fine-tune performance.
Domain-Specific Knowledge Gaps
The model might struggle with domain-specific terminology or concepts.
Mitigation: Fine-tune ChatGPT with domain-specific data or include detailed examples in prompts to improve understanding.
Bias in Annotations
LLMs can inadvertently reflect biases present in training data.
Mitigation: Implement diverse datasets for training and perform regular bias audits on outputs to ensure fair and balanced annotations.
Scalability Concerns
Handling large-scale annotation tasks efficiently can be challenging.
Mitigation: Optimize API usage, batch process annotations, and leverage automation tools to scale operations without compromising quality.
Transform your ChatGPT capabilities with our domain-specific LLM fine-tuning services for optimized data annotation
Future Directions for ChatGPT Data Annotation
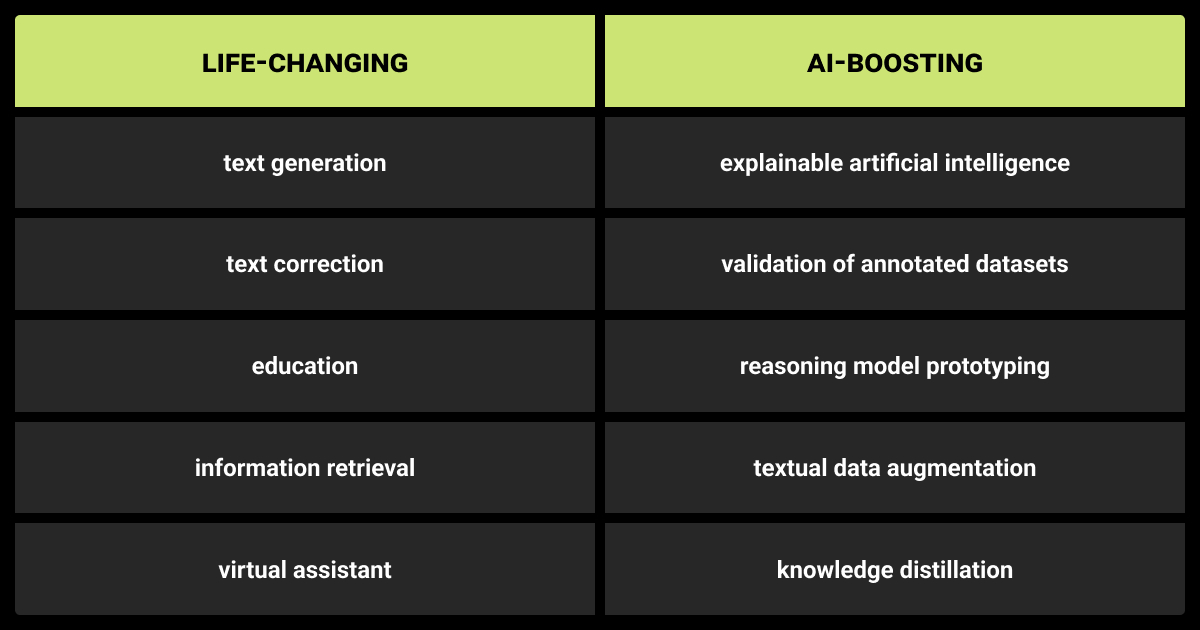
The future of ChatGPT data annotation lies in a hybrid approach that leverages the strengths of LLMs with human expertise. Human-in-the-loop validation, where humans review and re-label low-confidence instances generated by ChatGPT, can enhance the reliability of the annotations. Developing domain-specific LLMs tailored to particular annotation tasks could also improve accuracy and relevance.
Furthermore, ongoing research into optimizing prompt strategies and model configurations is crucial. Refining how ChatGPT is used in different contexts can maximize its effectiveness as a data annotation tool. Additionally, integrating ChatGPT into existing workflows to identify and correct errors in annotated datasets could improve training data quality, leading to better-performing NLP models overall.
Yet, while ChatGPT shows significant potential in automating data annotation, it is not yet ready to fully replace human annotators. The most effective use of ChatGPT in this context will likely involve a combination of automated processing and human oversight.
Final Take
The integration of ChatGPT into text annotation workflows enhances efficiency and scalability and provides a robust annotation framework. It ensures quality and consistency through human-AI collaboration.
This trend underscores the growing importance of LLMs in the evolving landscape of NLP and AI-driven data annotation.
While ChatGPT is powerful, human precision is unmatched—partner with us to ensure your dataset is annotated according to the highest standards. Get a quote!
FAQ
Can I use ChatGPT for data annotation?
Yes, ChatGPT can be a powerful tool for data annotation, especially in tasks like text classification, sentiment analysis, and entity recognition. It can generate initial labels or refine existing ones, significantly speeding up the annotation process.
However, while it can handle straightforward annotations, tasks requiring domain-specific knowledge or nuanced understanding may still require human oversight.
Can I use AI for data annotation?
Absolutely. AI-driven tools can automate many aspects of data annotation, such as labeling images, tagging text, or transcribing audio data. These tools use ML algorithms to learn from existing annotated data and apply this learning to new, unlabeled data.
While AI can handle large volumes of data quickly, monitoring and validating the output is essential, particularly in cases where the data is complex or ambiguous.
What are the benefits of using AI for data annotation?
Using AI for data annotation offers several key benefits. First, it dramatically speeds up the annotation process, allowing handling large datasets that would be time-consuming for humans alone. Second, AI reduces the likelihood of human error, ensuring more consistent and accurate annotations.
Third, AI tools are scalable, meaning they can manage increasing data volumes without a proportional increase in cost or time. However, combining AI and human expertise often yields the best results, particularly in highly specialized domains.
Written by
Karyna is the CEO of Label Your Data, a company specializing in data labeling solutions for machine learning projects. With a strong background in machine learning, she frequently collaborates with editors to share her expertise through articles, whitepapers, and presentations.