NLP Data Labeling for Finance: Deciphering the Language of Money
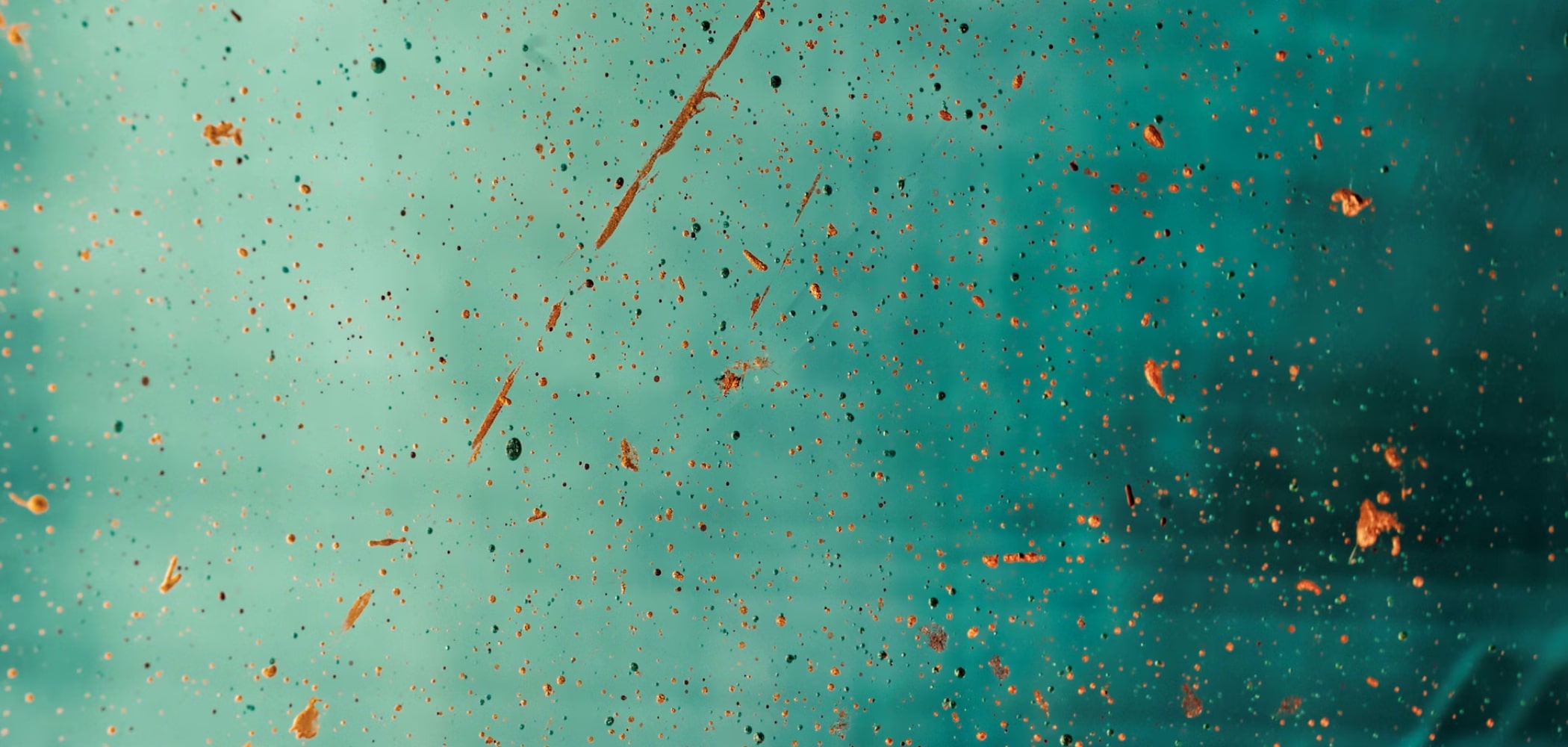
Finance is a hectic market, where every second counts. And so timely and accurate prediction can either make or break the industry. From Wall Street to Main Street, financial players are always on the hunt for novel solutions to lead their game.
This year, machine learning in finance is considered the main trend in the sector, with the potential to revolutionize banking, payments, and investments. More specifically, FinTech companies are massively relying on Natural Language Processing, aka NLP, for its automation capabilities and efficient processing of large volumes of unstructured data. That is, customer interactions, financial reports, regulatory filings, email and chat data, and so on.
Now, imagine being able to talk to your computer as easily as you talk to a friend. That’s the power of NLP technology. By enabling communication between humans and machines, NLP is transforming user experience and making technology more accessible to financial gurus. It’s a game-changer for the finance sector, and the possibilities for innovation are endless. So let’s explore them together!
What You Need to Know About NLP in Finance
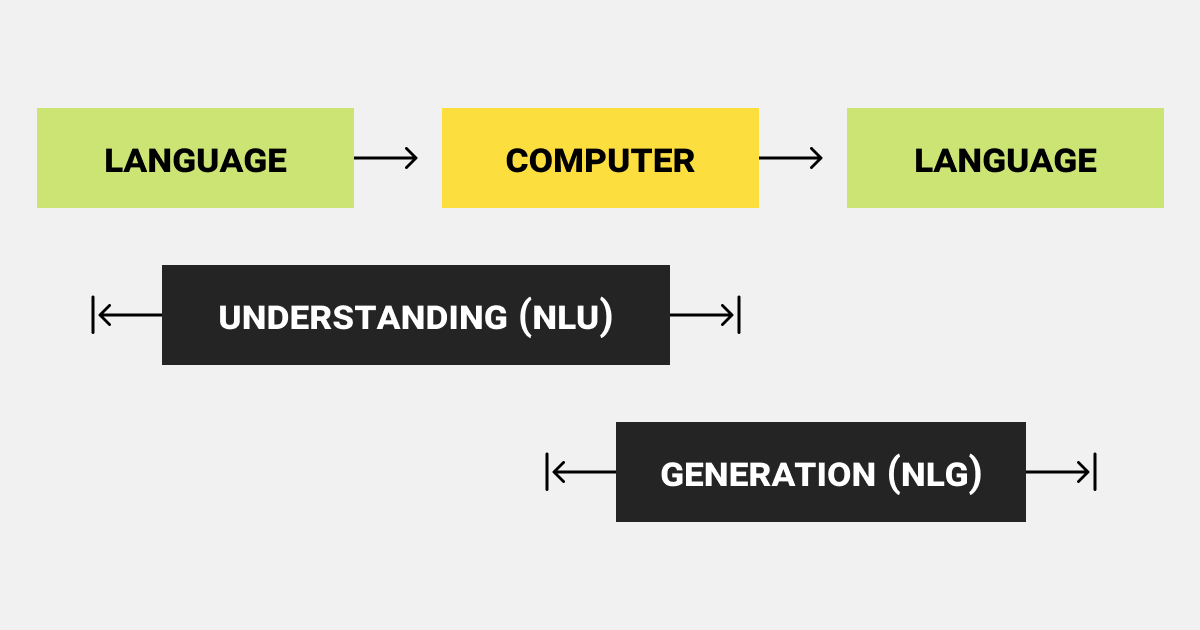
The FinTech realm aims to revolutionize the world by creating value and utility in every activity. With communication becoming increasingly globalized, companies are adopting remote work and turning to FinTech for solutions. Natural language processing (NLP) is one of the cutting-edge technologies driving innovation in this field.
With NLP in the banking and finance sector as a unifying factor, language is no longer a barrier to business success — instead, it is a lever that drives innovation and change. NLP systems are designed to process and interpret a wide range of text-based data, using natural language understanding (NLU), sentiment analysis, entity recognition, and many other techniques to extract valuable insights from unstructured data.
Another important aspect of NLP in finance is its ability to automate tasks that would normally require human intervention, such as fraud detection, risk assessment, and customer support. By choosing the right machine learning algorithms, NLP systems can learn from historical data to make predictions and recommendations based on new data inputs.
However, the main challenge for advanced systems in finance is dealing with the complexity of financial language, which often includes jargon, technical terms, and abbreviations. To overcome this challenge, NLP systems must be trained on labeled data that includes specialized financial lexicons and ontologies that capture the nuances of financial language.
The Main Applications of NLP for Finance and the Role of Labeled Data
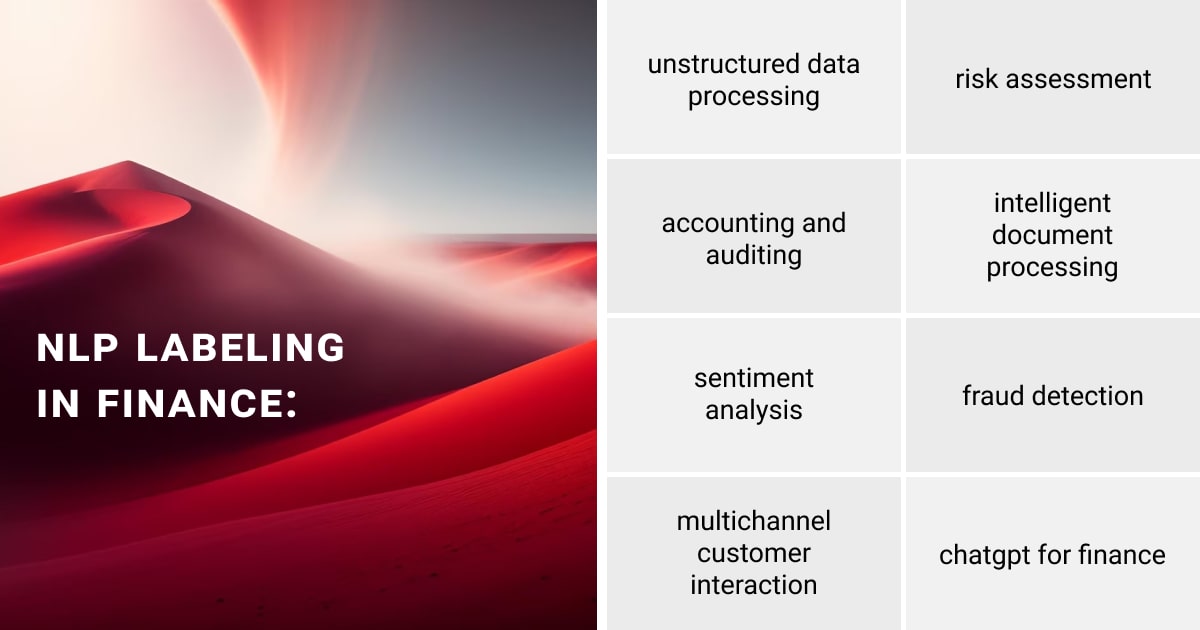
Natural Language Processing is a powerful technology that has the potential to transform the way finance wizards work with data. By providing faster, more accurate insights and automating routine tasks, NLP has proven to have a versatile range of applications in the world of finance.
The practical NLP use cases in finance are numerous, with some notable examples like:
Unstructured Data Processing
Finance is inundated with various data types from different sources, which can be tedious to analyze. NLP helps financial institutions find relevant information across unstructured resources. Although you can use unlabeled data in ML, NLP models are required to analyze and extract valuable information from this data to infer meaningful insights. This makes searching digital documents easier for compliance and regulatory compliance evaluation. NLP also keeps track of system changes, updates rules, and follows up on settlements via different channels.
Risk Assessment
You’ve probably heard the saying: no risk, no money. And that’s where data annotation and NLP can be of great use. To mitigate risk, investment and financial companies use NLP annotation techniques like named entity recognition and document categorization to extract information from customer documents. This information is then used to assess the customer’s risk profile for loans based on established criteria. The NLP credit risk models examine applicant account history, credit history, employment, and education, providing a faster and more comprehensive analysis.
Sentiment Analysis
To keep up with increasing pressure to satisfy customers, many companies use NLP to analyze sentiment. This enables machines to comprehend human emotions expressed in text. However, financial sentiment analysis differs from the regular one in both domain and purpose. Financial sentiment analysis powered by NLP predicts market reactions and stock price changes, while regular sentiment analysis simply determines if the text is positive or negative. This data annotation task helps identify signals of equity performance from research reports and social media, allowing companies to make better investment decisions.
Fraud Detection
NLP algorithms help fraud detection departments to identify relevant data in customer documents (e.g., credit history, account activity, loan transactions, and income history) for banks and investment firms. Any unusual activity is quickly flagged and confirmed with the customer, and law enforcement is alerted if necessary. The labeling types in finance used here are entity annotation, sentiment annotation, classification, sequence annotation, or anomaly detection.
Accounting and Auditing
Numerous financial services companies are prioritizing NLP for conducting efficient audits of their clients’ annual performances. This technology assists in documenting reviews, long-term procurement agreements, and government data, while also aiding in the identification of potential risks such as money laundering and fraud. Named entity recognition (NER), sentiment analysis, topic modeling, and keyword extraction are some of the possible methods here of NLP labeling for finance.
Intelligent Document Processing (IDP)
The BFSI (Banking, Financial Services and Insurance) industry handles many legal documents that need to be processed efficiently. NLP data annotation can simplify the document search and processing. IDP solutions use various labeling types, such as Named Entity Recognition (NER), Optical Character Recognition (OCR), and NLP, to extract and classify data from unstructured documents based on specific parameters. This reduces processing time and increases information accessibility, enabling better compliance understanding.
Multichannel Customer Interaction
Natural Language Processing in finance aids institutions in obtaining timely and relevant information through information filtering. Major institutions use NLP-powered chatbots to engage customers and improve products and services. NLP is an effective tool for enhancing customer service and support.
ChatGPT for Finance
ChatGPT is on everyone’s lips today. This AI language model can enhance existing NLP-based financial applications, including risk management, NLU, NLG (generation), and text-based financial analysis. GPT models have been applied to financial document classification, sentiment analysis, named entity recognition, and text-based financial analysis, such as news and social media analytics. The chatbot can also generate financial reports, summaries, and forecasts, improving accuracy and reliability. However, ethical and regulatory concerns need to be considered when using this technology.
Without a doubt, NLP presents an extensive spectrum of applications in finance. It provides a comprehensive analysis and interpretation of financial text data, which proves beneficial for various tasks, such as sentiment analysis, event detection, risk management, and regulatory compliance. However, the particular use of NLP in finance heavily relies on the specific objectives and demands of the task at hand, and often involves employing an amalgamation of diverse techniques.
The Specific Approach to NLP Labeling for Finance-Related Tasks
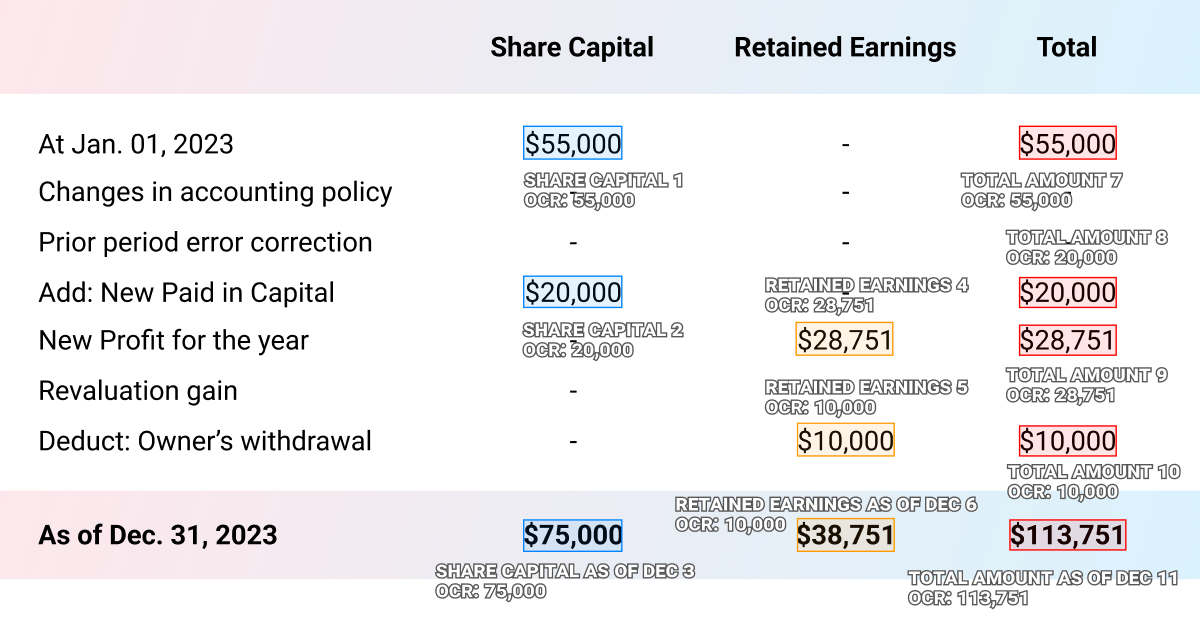
Although NLP labeling is widely used in finance, it still faces challenges and limitations. For instance, there is frequently a lack of accurately labeled training data for specific financial NLP tasks. As such, ML model performance is unlikely to deliver desired results. Additionally, NLP models may not fully comprehend the intricacy and context of human language, resulting in mistakes and prejudices.
As with every other data annotation task in AI, NLP labeling for finance must be performed with due attention to quality, rather than quantity of training data. Luckily, with NLP, we can do so much more than just analyze text. We can extract key information, search through vast amounts of data, and even translate between languages.
From earnings reports and financial statements to news articles and press releases, labeled data helps investors make informed decisions about stock prices and market trends. As an alternative, automated data annotation can also be used to reduce the time and cost associated with manual labeling for NLP tasks in finance.
Let’s take a look at the most common types of data annotation used in finance:
- NER (Named Entity Recognition) for Finance: Identification of important financial indicators from text.
- Sentiment Analysis for Investment: Used to gauge market, investor, and customer sentiment for informed investment decisions.
- Topic Modeling for Trends: The analysis of news and reports to identify emerging trends and market shifts.
- Financial Text Classification: The classification of financial text into different categories for valuable insights and organization.
- KYC Fraud Detection: Used to verify customer identities and detect fraud with KYC (Know Your Customer) automation.
- Legal Documents Extraction: The analysis of legal documents for key information, using clauses and entities Extraction.
- Text Categorization for Organization: The categorization of text data into different categories for better organization and analysis.
However, Natural Language Processing in finance poses various challenges in the context of data labeling, such as the subjectivity of financial data, data quality, and limited data availability.
Final Words
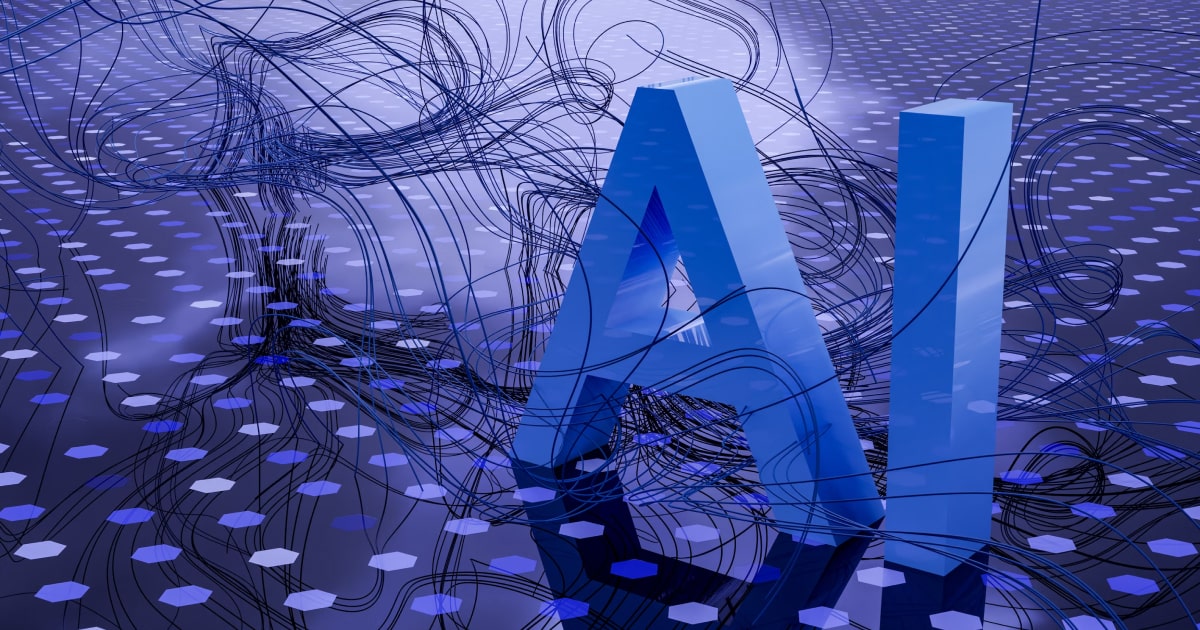
Wrapping up, NLP data labeling is an essential step towards achieving accurate and insightful analysis of financial data. While challenges may arise, they can be overcome with the right approach and tools. Leveraging NLP in the finance sector can lead to better investment decisions, improved risk management, and ultimately drive business success.
So, if you want to stay ahead of the competition and make the most of your financial data, consider exploring the possibilities of NLP for finance today.
Contact our team for expert, end-to-end data labeling service for your NLP initiatives!
FAQ
What are the different labeling types in finance?
There are no standard methods for financial annotation, but some common types include sentiment analysis, NER, document categorization, and OCR.
What are some challenges of implementing NLP in finance?
The main challenges of applying NLP for finance are the complexity and ambiguity of financial language, data quality issues, and the need for domain expertise in both finance and NLP.
How is NLP labeling for finance performed?
Data labeling for NLP in finance sector is performed using manual methods to train machine learning models to classify and extract information from financial texts. However, the labeling tools in finance can be used as well to automate the process.
Written by
Karyna is the CEO of Label Your Data, a company specializing in data labeling solutions for machine learning projects. With a strong background in machine learning, she frequently collaborates with editors to share her expertise through articles, whitepapers, and presentations.