Data Enrichment in the Age of Big Data: Getting the Most Out of Data?
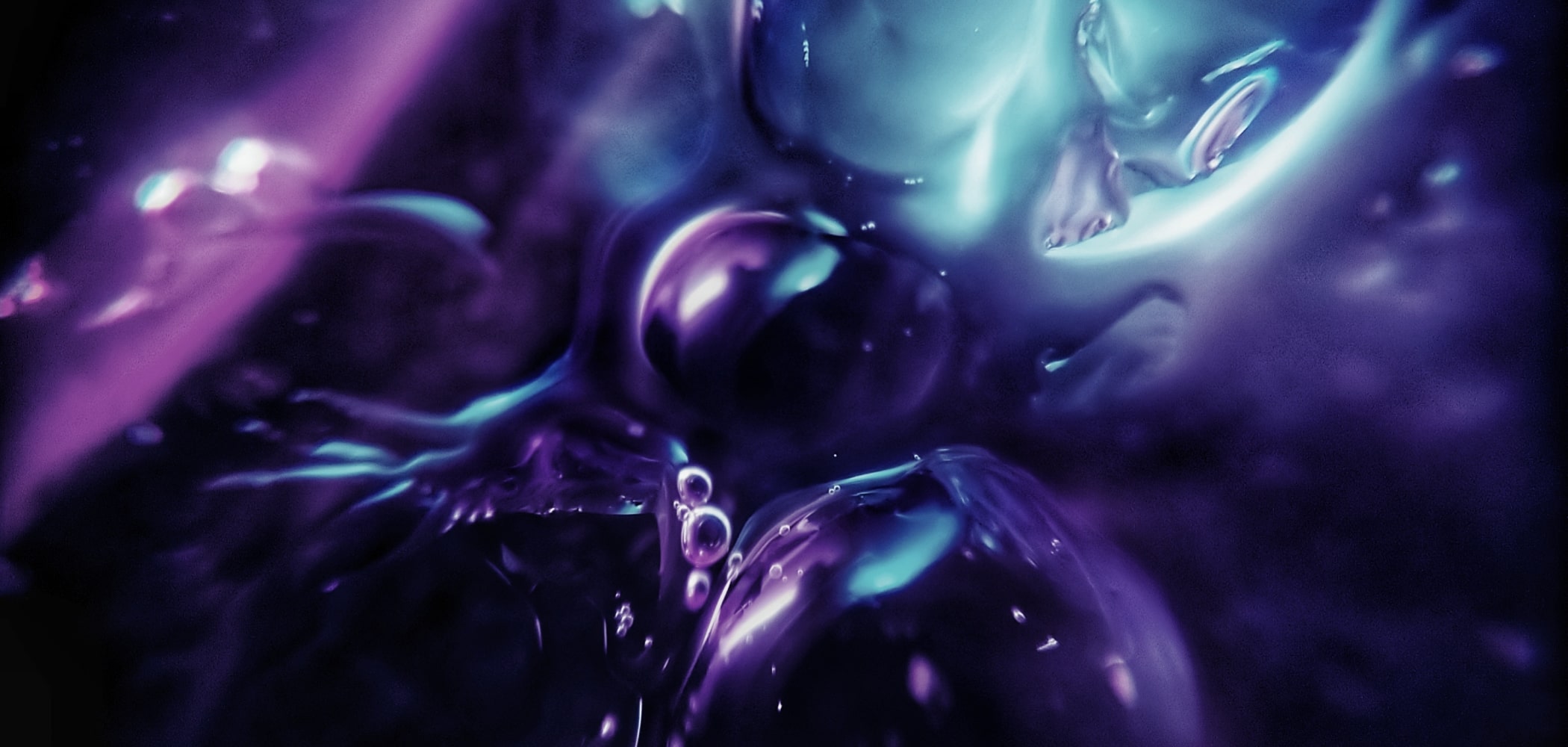
With over a decade of industry expertise, we’ve been mostly talking about ways to enhance data through labeling it. But there are other ways to make data more valuable and insightful. Through data enrichment.
Say you have a customer database, or a CDP (customer data platform), for an online store that includes basic information such as names, email addresses, and purchase history. However, this data doesn’t give a full picture of your customers’ preferences. How do you make it more helpful?
A popular method used for enhancing your customer data is called data enrichment. In this case, the method implies incorporating additional information about the customers. It can be demographic data, social media activity, or even third-party data sources. By doing so, you gain a more complete profile of each customer. And so now your data is rich enough to segment customers based on different criteria, personalize marketing campaigns, or make better product recommendations.
While this is a great illustration of data enrichment examples, the topic is significantly more profound. So, let’s get to the bottom of this.
What Is Data Enrichment and Where Is It Used?
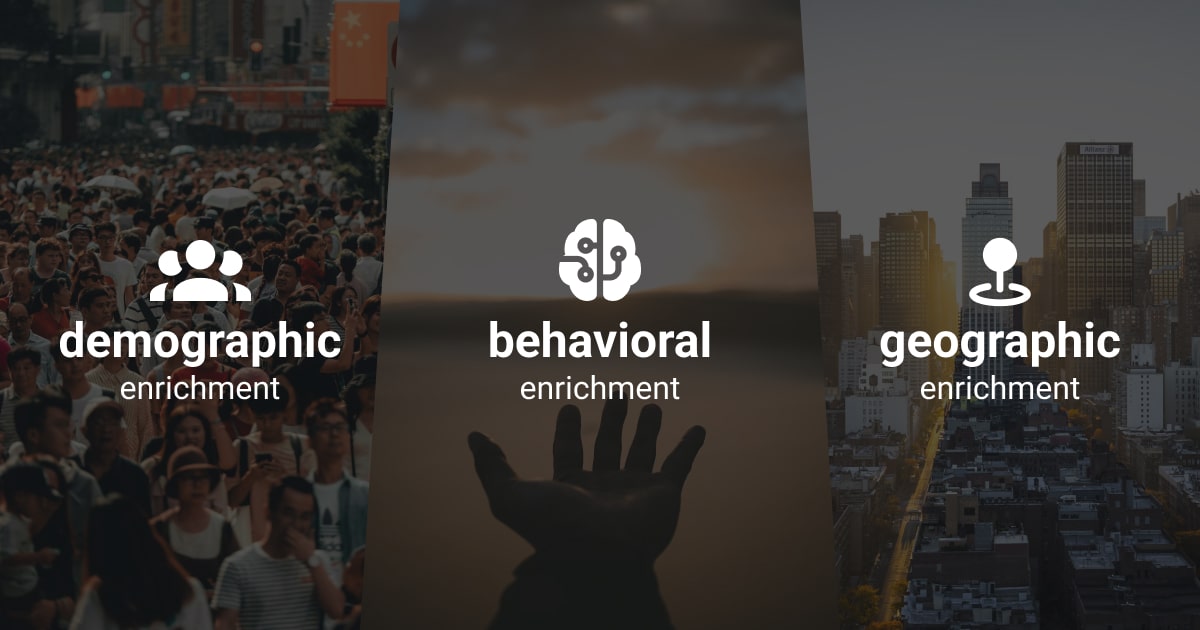
To illustrate what is data enrichment, let’s consider a practical scenario:
Say you’re developing an ML algorithm for predicting stock market trends using financial data. The first step you take is obviously data collection in ML. But you might end up with data that is scattered and lacks unique, crucial insights. To enhance its accuracy, you can enrich the dataset by incorporating additional financial indicators and market sentiment data, resulting in more informed and reliable predictions.
In essence, the data enrichment process is about achieving better quality of the dataset by feeding it with new data. Specifically, it means combining external third-party data from reliable sources with a preexisting repository of customer data owned by the company, thereby enriching the dataset.
Businesses need data enrichment to improve the quality of their existing data, enabling them to make better-informed decisions. Initially, all customer data, regardless of its source, exists in its raw state. As this collected data is consolidated into a central data store, it’s typically ingested as separate datasets. However, this raw information often lacks usefulness beyond specific contexts.
Hence, the data enrichment goal is to augment existing datasets with additional and complementary information. Teams then go through the process of verifying this information against external sources, thereby enhancing the overall value and reliability of the data.
How Is Customer Data Enrichment Different?
66% of consumers anticipate companies to grasp their distinct demands and expectations, with 52% desiring personalized offers across the board. By adopting robust customer data enrichment solutions, companies can meet this demand.
This is a specific type of data enrichment that focuses on enhancing customer-related data with additional information to improve customer-centric strategies. It involves merging your existing first-party customer data with third-party data from external sources to augment the information available. For instance, your first-party consumer data includes details about their shopping behaviors on your website or store, but lacks additional information about their preferences.
Customer data enrichment can enhance the depth and breadth of insights about your customers. Consequently, you can gain additional insights about your customers, including their residential and occupational information, hobbies and interests, as well as their preferred brands. This enriched data helps you make better decisions, reach a wider audience, and provide personalized experiences to a broader audience.
Exploring Data Enrichment and Data Annotation Relationship
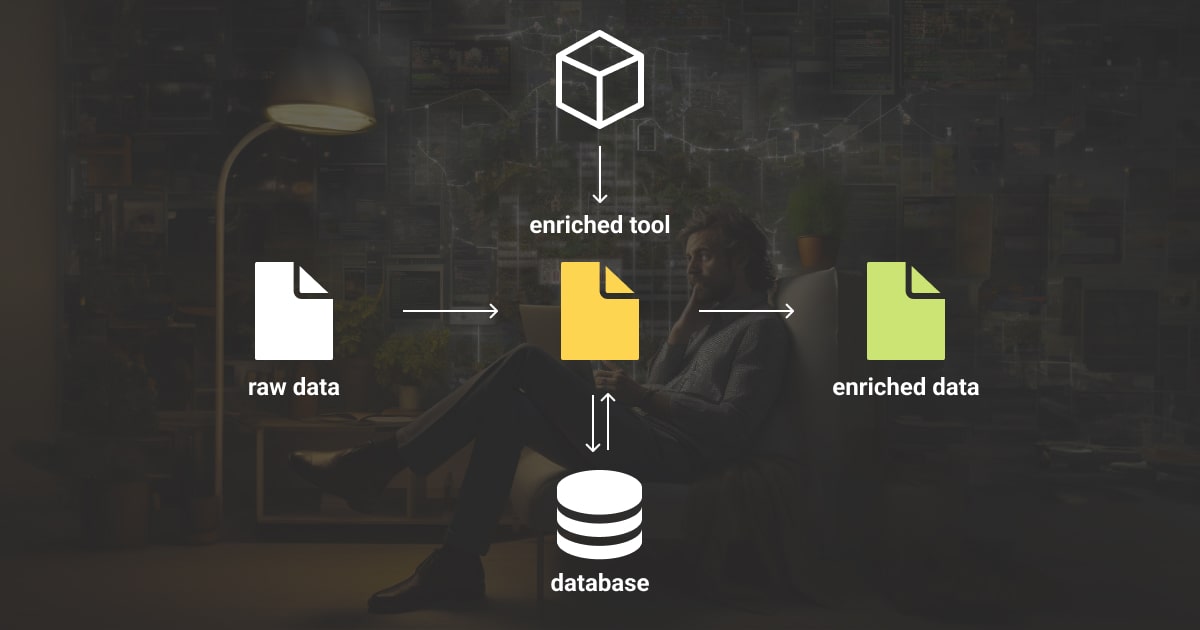
As you now know more about data enrichment, you may be wondering whether it’s the same as data annotation in AI. In fact, both data annotation and data enrichment can be used interchangeably, but this is not always the case.
On one hand, we can say that by annotating data, we enrich it in some way. From the technical perspective, these are two different processes but with very similar meaning.
Data annotation is performed by trained experts who add relevant tags, aka labels, to pieces of data to make it usable for machine learning algorithms. Case in point, in image recognition, annotators may label objects in images with corresponding class labels (e.g., “aircraft,” “vehicle,” or “pedestrian”) or draw bounding boxes around objects of interest.
Data enrichment refers to augmenting the quality and depth of existing data by adding additional information or improving its quality. It involves various techniques such as data augmentation, feature engineering, or integrating external data sources. This way, we receive more comprehensive and accurate data for analysis or ML purposes. For instance, enriching product data with customer reviews and ratings can provide valuable insights about product performance, customer satisfaction, and feature preferences.
Now, onto the interesting part. Data annotation is a vital step in data enrichment. Annotated data is needed to train machine learning models effectively. It enables models to learn patterns and make predictions. After annotation, data enrichment techniques can be applied to enhance the labeled data, leading to improved model performance and more informative analysis.
Data Enrichment Process Overview: Types & Approaches
Data enrichment involves using two types of data: first-party and third-party data. First-party data is the one you get straight from your customers, ensuring a direct and firsthand source of information. This includes purchase history, website activity, or feedback. Third-party data is from external sources not affiliated with your business, like demographic data, public records, or social media data.
Data enrichment combines and enhances first-party data with relevant third-party data. This helps businesses make accurate predictions, personalize customers’ experience, and make informed decisions based on a broader perspective. There are three main types of data enrichment that businesses can use:
Demographic data enrichment
Enriched demographic data targets messaging to specific groups, making advertisements relatable and enabling customized communication based on organization size.
Geographic data enrichment
Enriched geographic data tailors messaging to specific locations for enhanced user experience, ensuring relevant content based on country, time zone, and city.
Behavioral data enrichment
By enriching behavioral data, you incorporate customer behavior patterns into their profile, revealing their interests and purchase journey. This process is crucial for assessing campaign effectiveness and optimizing marketing budgets.
Now that we have covered the main types of data enrichment, let’s move on to the key data enrichment techniques to enhance your data.
Appending Data
This approach involves combining multiple sources to create a comprehensive dataset, enhancing analytics and expanding variables for ML models. Appended data can include both internal and external sources.
Segmentation
Data segmentation entails dividing a dataset based on specific field values, such as demographics, geography, technology, or behavior. In marketing, it helps target specific audiences.
Derived Attributes
Derived attributes are calculated fields in a dataset, like age based on birthdate, and include various time conversions, counts, and classifications.
Data Imputation
Data imputation, a part of data cleansing, involves replacing missing or inconsistent values within fields. This includes estimating the value of a missing field based on other available values.
Entity Extraction
Complex unstructured or semi-structured data may have multiple encoded values in a field. To ensure usability, extract these values and expand them into new columns. For example, OCR data extraction aids in retrieving valuable information from scanned documents and images.
Data Enrichment Examples and Use Cases
Data enrichment commonly involves enhancing customer data by incorporating demographic information from various sources, both internal and external. This practice finds application in numerous scenarios, including the following data enrichment examples:
Retail: Adding data enhances customer profiling to understand needs for effective recommendations, upselling, and cross-selling.
Marketing: Incorporating data from diverse sources improves the precision of marketing campaigns and processes, enabling enhanced targeting and personalized offers.
Insurance: Maximizing the inclusion of data, whether internal or from third-party sources, enhances it for customer categorization, segmentation, and targeted marketing efforts.
Lending: Employing third-party databases aids in creating comprehensive customer profiles, assisting with credit scores and underwriting processes.
Businesses across various sectors are implementing data enrichment to enhance their understanding of customers, improve personalization efforts, and make data-driven decisions based on more comprehensive and accurate insights. They use enriched data to refine customer segments and improve conversion rates through more precise lead scoring.
Summary
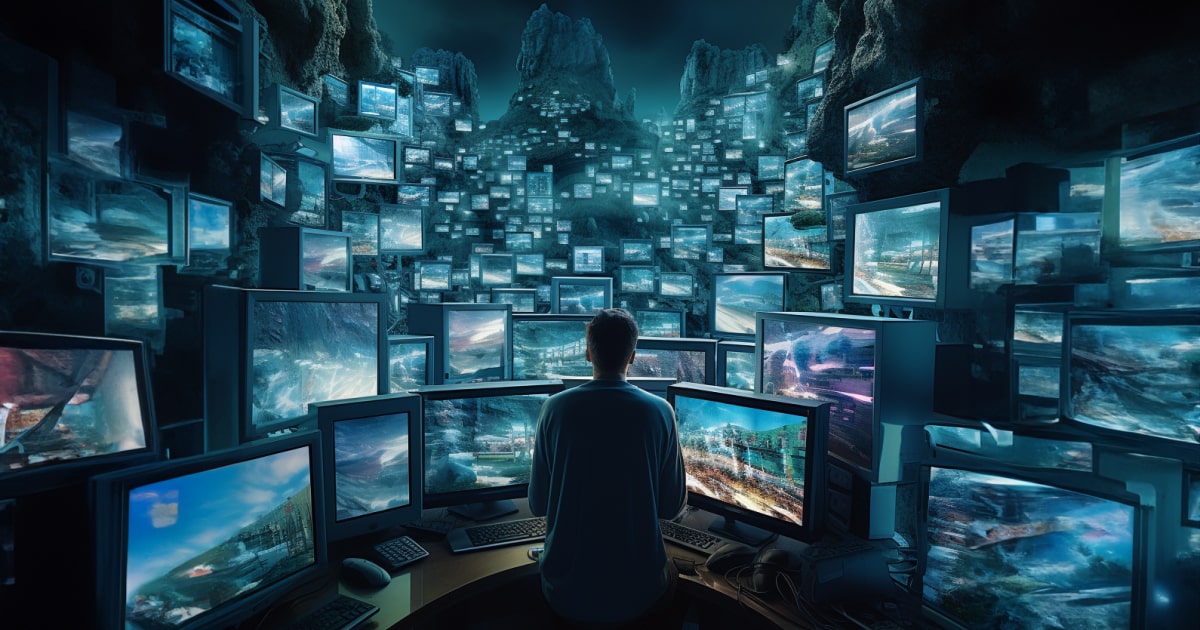
Customer data enrichment is an ongoing and privacy-conscious process that requires constant attention and updating the data regularly as it evolves. Neglecting continuous data enrichment means missing out on opportunities to deliver value through personalized offers and experiences.
However, you must prioritize privacy and compliance while ensuring that the enriched data remains actionable, valuable, and easily interpretable. That’s where our Label Your Data team excels.
FAQ
What is the difference between data enrichment and data cleansing?
Data enrichment involves enhancing existing data with additional information and making your customer records as complete as possible, while data cleansing removes duplicate or inaccurate records and leaves accurate data alone to improve its quality.
What are the goals of data enrichment?
The objectives of data enrichment are to make a dataset more valuable and facilitate informed decision-making. Customer data enrichment, for instance, elevates customer experiences by providing unique insights on customers.
How does data enrichment optimize marketing efforts?
Data enrichment services play a crucial role in marketing by enhancing customer understanding, enabling personalized targeting, and optimizing campaign effectiveness through the enrichment of data with additional insights and attributes.
Written by
Karyna is the CEO of Label Your Data, a company specializing in data labeling solutions for machine learning projects. With a strong background in machine learning, she frequently collaborates with editors to share her expertise through articles, whitepapers, and presentations.