Generative AI: The Creative Potential of Technology
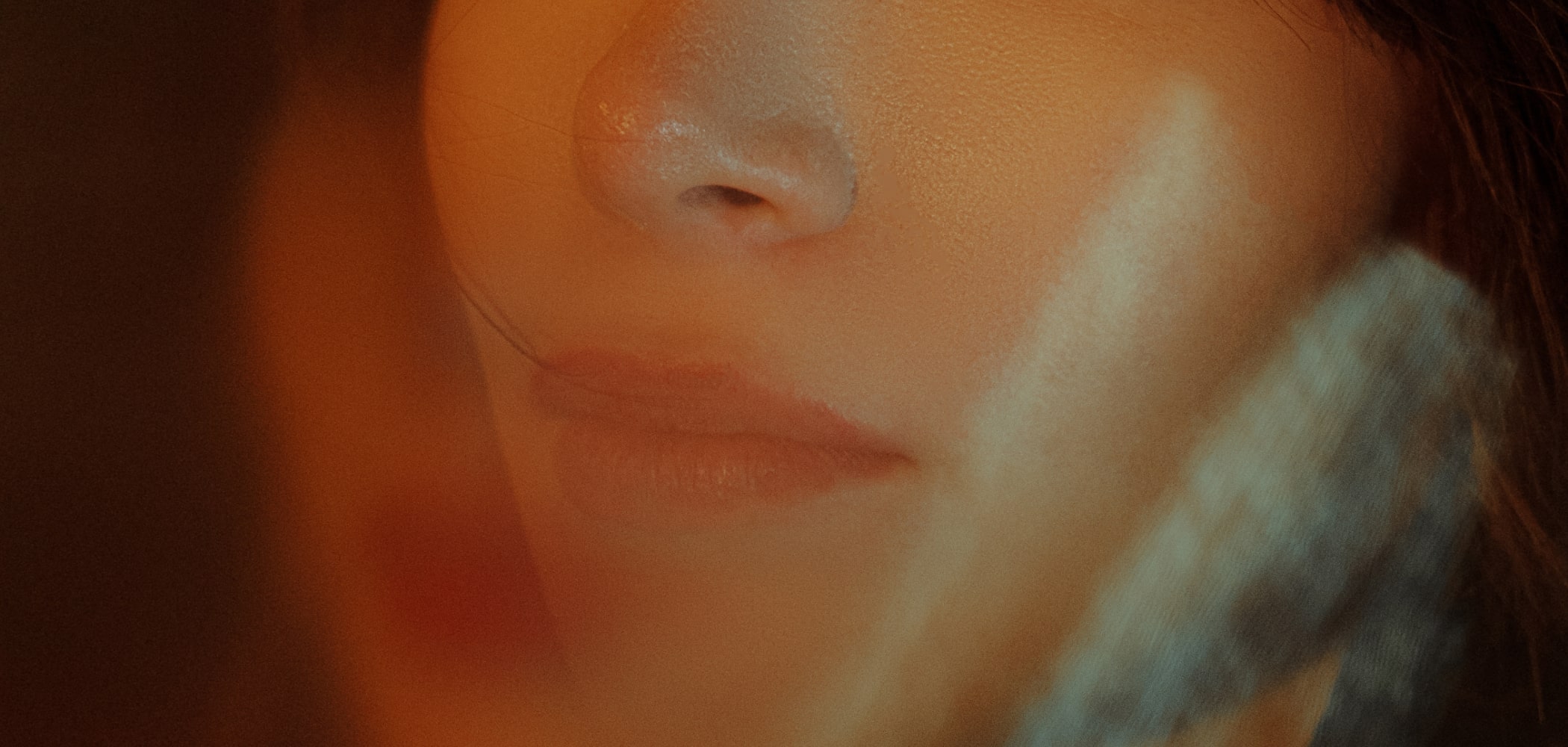
ChatGPT and Bard generate text. Midjourney and DALL-E generate images. MuseNet, among others, generates music. All that with no human involvement, just AI by itself making art and assisting humans with the majority of tasks we used to do on our own.
As the name suggests, generative artificial intelligence, or GenAI, means that something is being generated by this modern-day technology. Now, imagine a world where machines can create new and exciting content on their own. That’s the dream of generative AI, a branch of AI that specializes in producing brand-new data samples, from text to images, videos, and even 3D objects.
With advanced machine learning algorithms, generative AI is able to analyze existing data and learn its patterns and features, making it possible to generate all kinds of realistic and diverse outputs. For example, a generative AI model trained on datasets of famous paintings can create entirely new and unique artworks that capture the style and essence of the original pieces.
From content creation to design, entertainment and beyond, the potential applications of generative AI are truly limitless. Let’s explore them together.
How Does Generative AI Work?
Artists and musicians seem to be particularly excited about the possibilities that generative AI offers, according to the research. With the help of these advanced models, they are able to take their creativity to new heights and explore entirely new avenues of expression.
Generative AI models are being used across different fields today. This can encompass a wide range of sectors, including but not limited to content creation, design, gaming, and healthcare. They are built using machine learning techniques, such as deep learning and reinforcement learning, and they work by analyzing existing data and learning its patterns and features.
A diverse range of generative AI models exist, and these models can be classified into various types. They include generative adversarial networks (GANs), variational autoencoders (VAEs), and autoregressive (AR) models. Each model has its unique approach:
GANs
Generative adversarial networks (GANs) are a widely used generative AI models that involve two neural networks. More specifically, a generator and a discriminator. The role of the generator is to produce fresh data samples. The discriminator is responsible for distinguishing between real and fabricated samples. Both networks work together to improve the generator's ability to create synthetic data that looks similar to real data.
VAEs
The variational autoencoder (VAE) is a different category of generative AI model. It’s based on the principles of a neural network called an autoencoder. VAEs can be used to generate new data samples by learning the probability distribution of the input data and then sampling from that distribution to create new samples.
AR models
Autoregressive models belong to the category of generative AI models that leverage prior values in a sequence to predict the probability distribution of the upcoming value. These models can be used to generate sequences of data, such as natural language text or music.
The Business Benefits of Generative AI
McKinsey predicts that using generative design can slash engineering time by 23-38%, cut costs by 8-15%, and revolutionize the way we think about solving complex problems. Hence, innovative product development powered by generative AI will be able to revolutionize the industry, enabling businesses to save costs, reduce development time, and unlock creative and non-intuitive solutions.
Well, generative AI has a lot more to offer modern businesses:
- Creative empowerment: Generative AI technology adds to the massive creative potential of humans, providing novel solutions for content creators to discover new ways of creative expression.
- Personalized engagement: The generative technology can also personalize content for customers, resulting in a more personalized customer experience and improved engagement and sales.
- Better productivity: GenAI has the potential to boost productivity by automating mundane tasks such as video or image editing, allowing employees to allocate their time towards more strategic work.
- Cost reduction: The use of generative AI can lead to lower operational costs and increased productivity by automating tasks and streamlining workflows, resulting in reduced overhead expenses.
- Improved customer satisfaction: GenAI enables businesses to craft custom content and experiences that resonate with their audience, resulting in heightened loyalty and satisfaction.
- Scalability: By enabling enterprises to quickly and cost-effectively produce large volumes of high-quality content and meet demand, generative AI also boosts scalability.
- Competitive advantage: Generative AI offers a distinct advantage to enterprises by enabling them to produce exclusive and innovative content that differentiates them from their rivals
The Role of Data Annotation for Generative AI Models
Data annotation in AI and its various techniques that are used for generative AI encompass a range of methods, including image annotation, entity recognition, sentiment analysis, conversation categorization, and metadata annotation. Each technique involves labeling data with specific attributes or features that help generative AI models learn and generate new creative content.
Case in point, image annotation can involve tagging objects or people in images, adding descriptive labels, or indicating the location of specific elements within an image. Entity recognition, in contrast, entails labeling words or phrases with specific meanings or categories, such as identifying names or locations within a text.
Another data annotation technique, sentiment analysis, involves labeling text data with specific emotions or sentiments, such as positive, negative, or neutral. Conversation categorization, on the other hand, involves labeling text data into different categories, such as customer service conversations, sales conversations, or general inquiries.
Metadata annotation involves adding extra information to raw data to assist AI models in recognizing and interpreting patterns. This might include details like timestamps, location data, or other relevant metadata that can help AI models better understand the context of the data.
With well-annotated data, generative AI models can be trained more accurately and with greater precision, leading to improved performance and the ability to generate new content that meets specific criteria or objectives. You can also check our recent article where we’ve talked about data annotation for training ChatGPT.
Generative AI in Practice: Types, Applications, and Use Cases
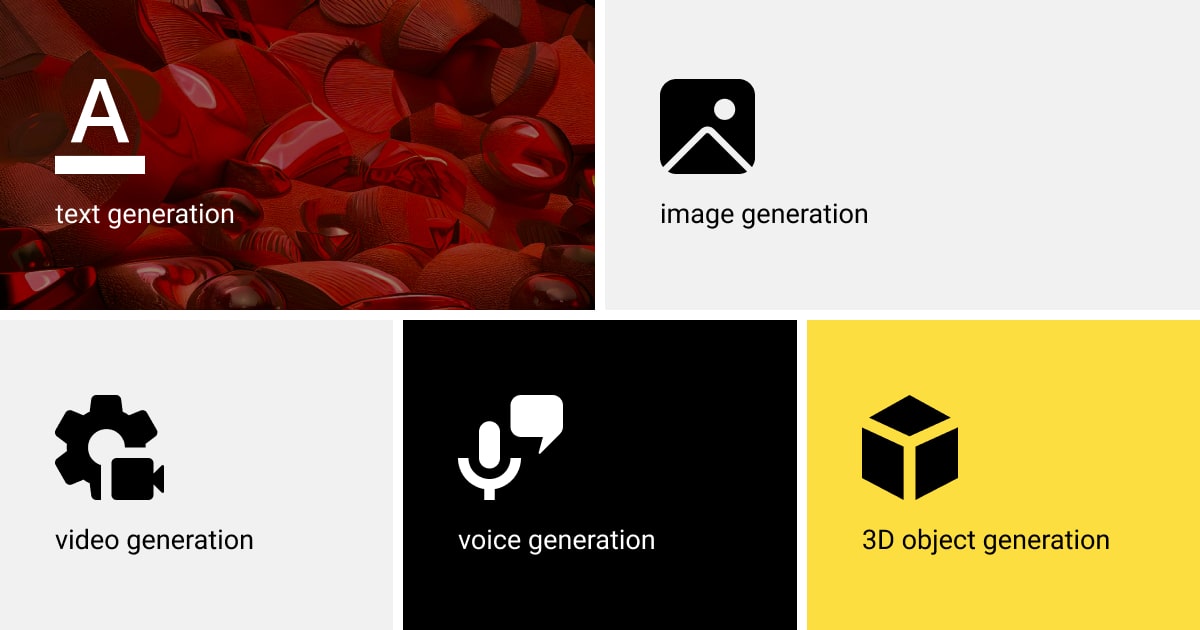
Generative AI is one of those mind-boggling technologies that have transformed the manner in which we operate and communicate with the world around us. For instance, ChatGPT with its 100 million users, or DALL-E that produces over 2 million images per day, are prime examples of how generative AI is quickly making its way into our lives.
From AI in healthcare to machine learning in finance, music, and art, generative AI has the power to revolutionize just about any industry. It’s still in its early stages, but we can expect it to have a massive impact on creative work and content generation.
Text generation
Text generation is when computers create human-like text by using advanced AI models that can understand and produce relevant content. These models leverage the Transformer architecture and learn from large datasets to create imaginative and coherent outputs. The most common applications of generative AI here are conversations with chatbots, content creation tools, translation, summarization of information, and knowledge management.
Image Generation
Image generation is the process of making artificial images through AI algorithms, which mostly use methods like Generative Adversarial Networks (GANs) and stable diffusion models. The generative AI applications that involve visual data, like images, include art creation, design generation, gaming art generation, data augmentation, text-to-image synthesis, and visual content creation for the advertising and media sector.
Video Generation
Video generation by AI involves using sophisticated algorithms. These are deep learning models that help produce fresh video content by drawing inspiration from extensive volumes of pre-existing video data, which has a broad range of applications in different industries. This type of generative AI is most widely used in the entertainment industry, VR and AR development, video games, education and training, as well as advertising.
Audio Generation
To generate human-like speech, voice generation uses advanced AI algorithms like WaveNet or Tacotron. These algorithms leverage deep learning techniques to learn human speech nuances, including pitch, rhythm, and intonation, to create natural-sounding audio from text inputs. Some common applications of audio generation AI are virtual assistants, audiobooks, text-to-speech tools, and even voice cloning.
3D Object Generation
Crafting 3D objects necessitates the use of software and computer graphics to fashion models with dimensions in height, width, and depth that can be visualized from various perspectives. Generative 3D models find applications in diverse fields such as animation, product design, gaming, architecture, and more. It’s also employed in the medical industry to generate 3D models of human anatomy, which are useful for research, education, and surgical planning purposes.
The Problems with Generative AI
Despite reaching surging popularity, GenAI technologies have raised several ethical concerns. One of the most pressing issues is the potential for job displacement and unemployment due to automation. Additionally, generative AI models require a significant amount of energy to train and operate, leading to environmental impact concerns. Another major issue is the inherent biases in training data that can perpetuate social inequalities and discrimination, leading to ethical dilemmas.
Additionally, there is an increasing concern about the potential misuse of AI-generated content to manipulate or deceive individuals or even society at large. The rise of deep fakes, which are AI-generated videos or images that appear to be real, has already been seen as a threat to public trust and security. Therefore, as generative AI technologies continue to advance, we must ensure that ethical considerations are addressed to mitigate potential negative consequences.
Final Words: Stepping Into a New Era of Creativity
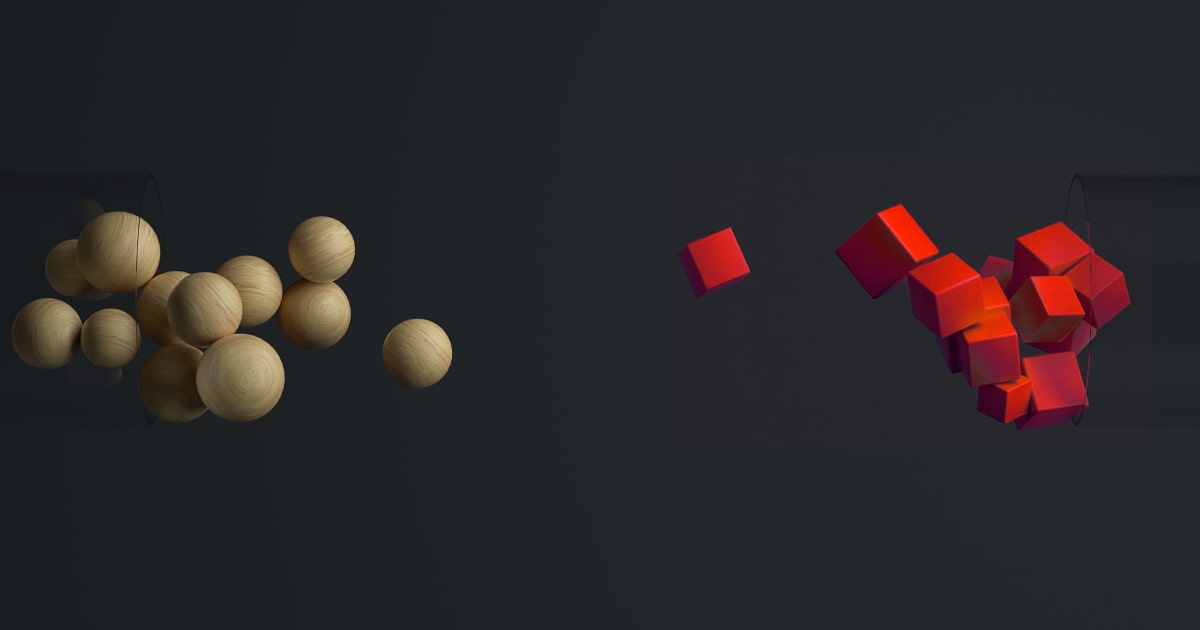
Generative AI provides numerous advantages to society. Notably, it can enhance our creativity levels and support us in tasks that were previously handled independently. This technology saves time, streamlines processes, and adds a personalized touch to various services, blurring the lines between man and machine.
While generative AI has enormous potential, it’s essential to use it with caution. It should complement human effort, not replace it, for optimal results. If you’re developing generative AI, our team of seasoned experts can provide you with meticulously annotated datasets to help you achieve your goals.
Send your request to Label Your Data and get the customized annotation solution for your AI project!
Written by
One of the technical writers at Label Your Data, Yuliia has been gradually delving into the intricate aspects of AI. With her strong passion for the written word and technical expertise, Yuliia has developed a keen interest in the evolving field of data annotation and the power of machine learning in today's tech-savvy world. Check out her articles to learn more about the complex world of technology and find the solutions that work best for your AI project!