Machine Learning in the Finance Industry: Is It Worth Taking the Risk?
Table of Contents
- Finance Is a Different Niche for Machine Learning
- The Status Quo of Machine Learning in the Financial Sector
- How Can Machine Learning Be Used in Finance?
- What Are the Most Common Machine Learning Applications in Finance?
- Machine Learning Projects in Finance: Label Your Data Shares Its Experience
- Summary: Can Machine Learning Algorithms Build a Neo-Finance Era?
- FAQ
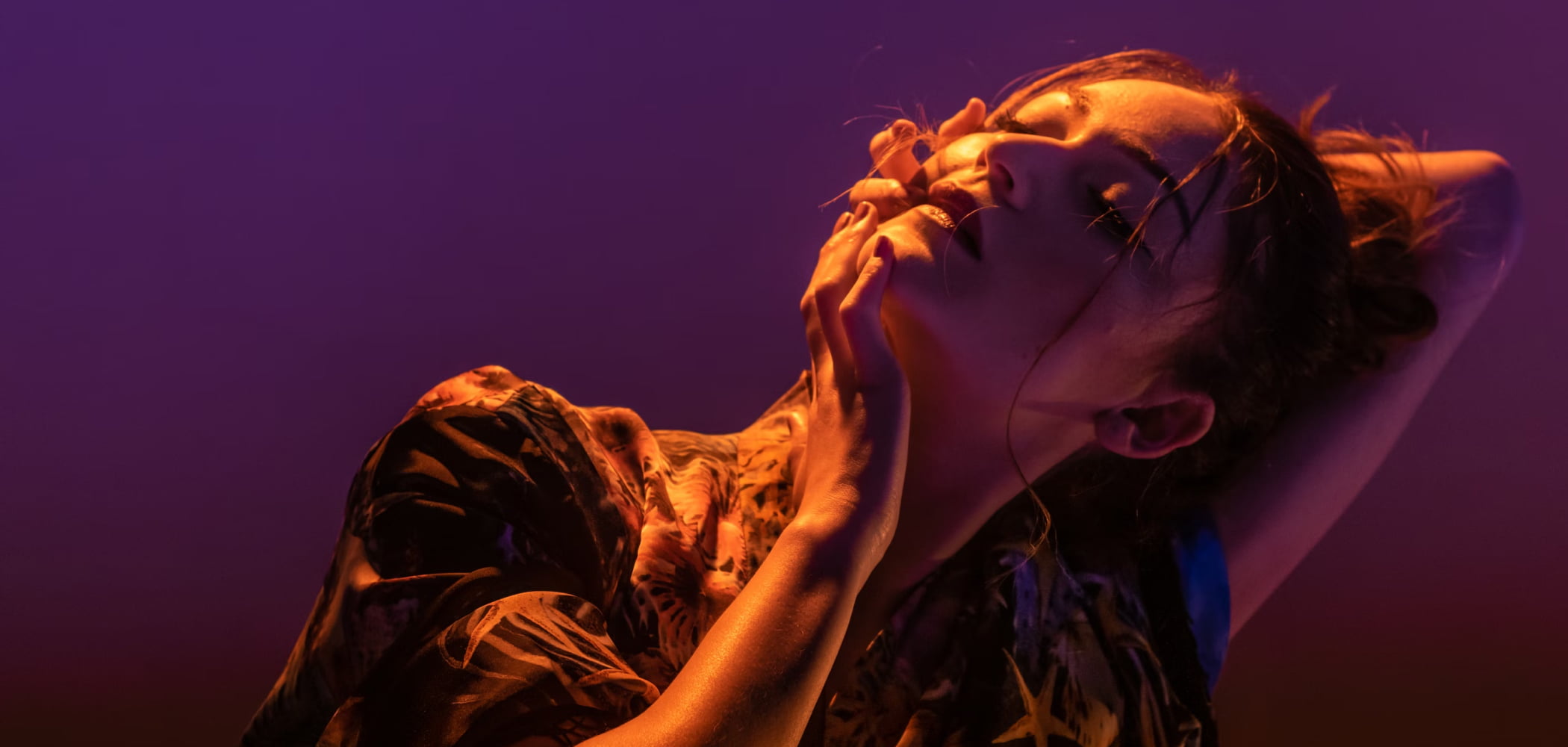
As they say, time is money, especially for a modern-day entrepreneur. But how to manage both in finance? We at Label Your Data believe that automation is the answer.
According to market research, the global AI in fintech market saw a growth from $9.15 billion in 2022 to $11.59 billion in 2023, with a yearly growth rate of 26.8%. These numbers are backed up with the latest stats in finance: 70% of financial services companies use machine learning to enhance their statistical and predictive capabilities. Such tendencies force banks and other financial institutions to rethink their traditional approaches to handling financial activities across the market.
Machine learning in the financial industry is a promising method as it has gradually found its way into this sector. Many crises have influenced the rising demand for ML solutionsin finance, notwithstanding many barriers to their adoption. However, it’s still unclear to financial circles how to deploy ML properly so that it becomes an asset, not a liability for them.
This isn’t the first time we’ve touched on the topic of finances and how sophisticated techniques like machine learning can help manage them. Most recently, we’ve talked through the ins and outs of financial datasets, the foundation of any manipulation in financial ML. We’ve also discussed how ML can be used for stock market predictions, a long shot for machine learning right now.
Today, we’ll try to analyze the entire financial industry to gain a comprehensive picture of ML capabilities in this sector and put together a puzzle of prior financial conversations.
Finance Is a Different Niche for Machine Learning
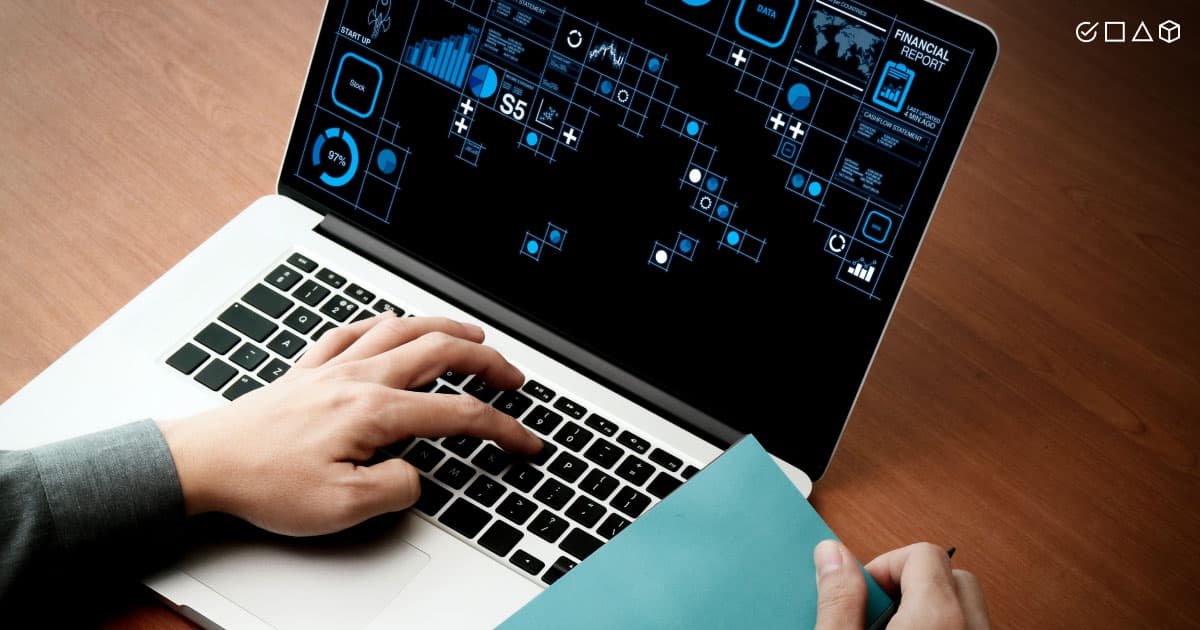
The rise of business intelligence data and machine learning solutions has spawned the sector of fintech, short for financial technology. In essence, it’s a skilled combination of digital innovations and technology-enabled business models in the financial sector.
What innovations have influenced the current state of fintech? They include cryptocurrencies, blockchain technology, new digital advisory, trading systems, equity crowdfunding, peer-to-peer lending, and of course, mobile payment systems. Many of them are on everyone’s lips, and we bet you’ve read about some of them in today’s financial headlines.
So, why is machine learning for finance a unique target for this industry compared to others?
- Small data vs. big data. TFinancial research mainly focuses on big data, but small data is the real issue in asset management. The core task in asset management, return prediction, is a small data problem. Big data in finance refers to many variables to predict returns. Yet, the richness of an ML model is defined by the number of observations, which is usually small.
- Unstructured data. This is typically text data or alternative (alt) data, as it’s called in financial circles. Most of the alt data have short data history, which undermines the accuracy of backtesting.
- Low SNR (signal-to-noise ratio). Financial data is noisy. This is mainly caused by unstable economic growth and financial market behavior that are difficult to predict. Also, the predictable signal in financial markets is small.
- Financial market dynamics. The data generating process is directly related to the actions performed by trading agents. Thus, technological advancements can also impact how we approach the financial market.
- Interpretability. Finance requires interpretable ML models to avoid algorithm biases and adverse outcomes.
The Status Quo of Machine Learning in the Financial Sector
There is no universally accepted approach to ML for various business cases in finance. There are many of them, actually, which is why machine learning can be considered a multifaceted strategy for regulating financial services. Moreover, ML can provide fresh new insights into the business performance, risk, and opportunities in this industry.
Is Finance Ready for Machine Learning?
While the banking sector’s need for advanced technological solutions is constantly rising, the industry is still not prepared for a significant shift in its traditional business practices. As in any other industry dealing with ML, there are numerous impediments to its smooth and fast adoption that apply to both people and technology.
First, financial organizations usually have unrealistic expectations towards machine learning and its capabilities. It’s also a cost-intensive venture to conduct research and development (R&D) in ML. While the first two issues can be solved over time, the third one becomes the main obstacle to effective ML adoption in finance. It’s an evident lack of ML experts and engineers to help companies find the right strategy for their business goals.
Last but not least, success with any ML project requires financial workers to be agile enough to follow the changes and update data infrastructure, as it should be. Are you contemplating an AI project and need high-quality financial datasets? Send a quote request to our team at Label Your Data and see what we have to offer!
Why Should Finance Embrace Machine Learning?
Today, the finance industry is becoming increasingly dependent on advanced computational practices. Meanwhile, financial systems are undergoing the rise of machine-readable data. This is quite a fast-moving trend that, in turn, requires an equally fast reaction from the industry wishing to follow and benefit from ML.
Machine learning can significantly reduce the operating costs of banking firms by providing automation opportunities where necessary. This way, the tandem of machine learning and finance can concurrently increase revenues by enhancing productivity and user experience. While the financial companies are benefiting from the automation capabilities of machine learning, they can also strengthen their security compliance.
How Can Machine Learning Be Used in Finance?
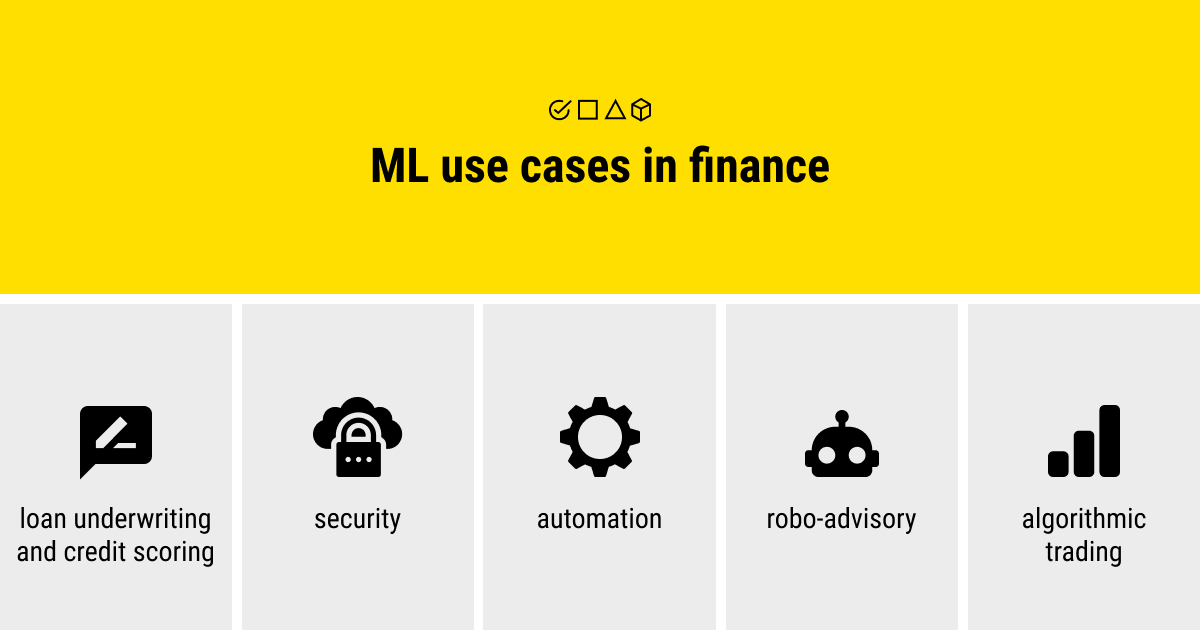
Financial experts need a technically sound business plan to join the accelerating pace of machine learning in the industry. However, one should bear in mind that financial ML methods don’t curtail the role of theory. ML goes hand-in-hand with econometrics so that complex ML algorithms can understand patterns in high-dimensional banking and financial space.
With a broad range of advanced algorithms and tools available today, financial organizations start to fully exploit the benefits of financial data by using the opportunities of AI in finance. And there’s a wider scope of uses of machine learning in financial services that already help the industry to get ahead of the digital curve.
What are the main machine learning use cases in finance?
— Automation
Automation gives an edge to major industries today. In finance, it’s the most crucial application of ML. Replacing manual tasks, automating the repetitive ones, and boosting productivity have never been easier in the financial sector. The right ML strategies made it possible. But let’s dig deeper.
Some of the automation use cases of ML in finance include:
- Chatbot technology. AI-powered chatbots are widely used in the banking sector to automate repetitive tasks, such as sending reminders or regular alerts, balance inquiries, and payment information.
- Call-center automation. Automated solutions for call centers can augment agents’ work and deliver a fast and personalized experience for financial service customers.
- Automated document management. Paperwork automation in finance implies dealing with digitized financial information: an automated collection of required data from different documents and value extraction from this data.
- Gamified employee training solutions. Corporate training in finance is getting more and more digitized to make the process easier, more efficient, and improve job satisfaction among financial workers.
JPMorgan Chase, BNY Mello, and Wells Fargo heavily rely on process automation in their financial services.
— Security
While ML proves to be an effective solution for many industries today, the digital transformation makes them more vulnerable to criminal activity via computer networks. Finance, sadly, is the most popular target for cybercriminals.
Cybersecurity threats in finance might result in the loss of all financial resources and even entire businesses. How can ML algorithms help address this issue?
- Fraud detection. ML algorithms can recognize unique features of fraudulent transactions (suspicious account behavior) and remember them for good by being trained on many cases of fraudulent behavior.
- Financial monitoring. A crucial security measure in finance, where the ML system is taught to identify a variety of micropayments and tag such money laundering methods as smurfing.
- Network security. ML algorithms can be trained to detect and isolate cyber threats because they can examine thousands of parameters in real-time.
Payoneer, PayPal, Stripe, Skrill, and Adyen are some of the financial companies that have integrated ML for security control into their systems.
— Loan Underwriting and Credit Scoring
Loan underwriting and credit scoring are quite popular tasks in finance and insurance. ML-based scoring engines in finance, particularly in lending, can significantly lower the cost of credit underwriting and help employees work faster and with better precision. Therefore, ML leads to financial inclusion in this area.
ML models can also improve the assessment of the creditworthiness of prospective debtors, strengthen the underwriting decision-making process, and enhance lending portfolio management for a banking credit analyst.
— Algorithmic Trading
Machine learning for finance can help make better trading decisions, financial planning, and assist with risk management strategies. ML algorithms can be used to analyze numerous data sources at once, which is impossible for human traders. ML can provide trading strategy suggestions and activate automated trading systems. These systems can make trading forecasts, decide on the course of action, and carry out trades.
In fact, AI-powered trading systems in finance can operate with no need for human intervention. It’s the power of advanced computation, machine learning, and probabilistic logic that makes it possible.
— Robo-Advisory
The rise of robo-advisors in fintech is caused by the need for sophisticated solutions in this sector. More specifically, it’s the need to analyze investing strategies and possible risks to see how they may affect personal finances in the future. Robo-advisory is primarily used for the following financial tasks:
- Portfolio management: algorithms and statistics
- Financial product recommendation: personalized and accurate recommendations
What Are the Most Common Machine Learning Applications in Finance?
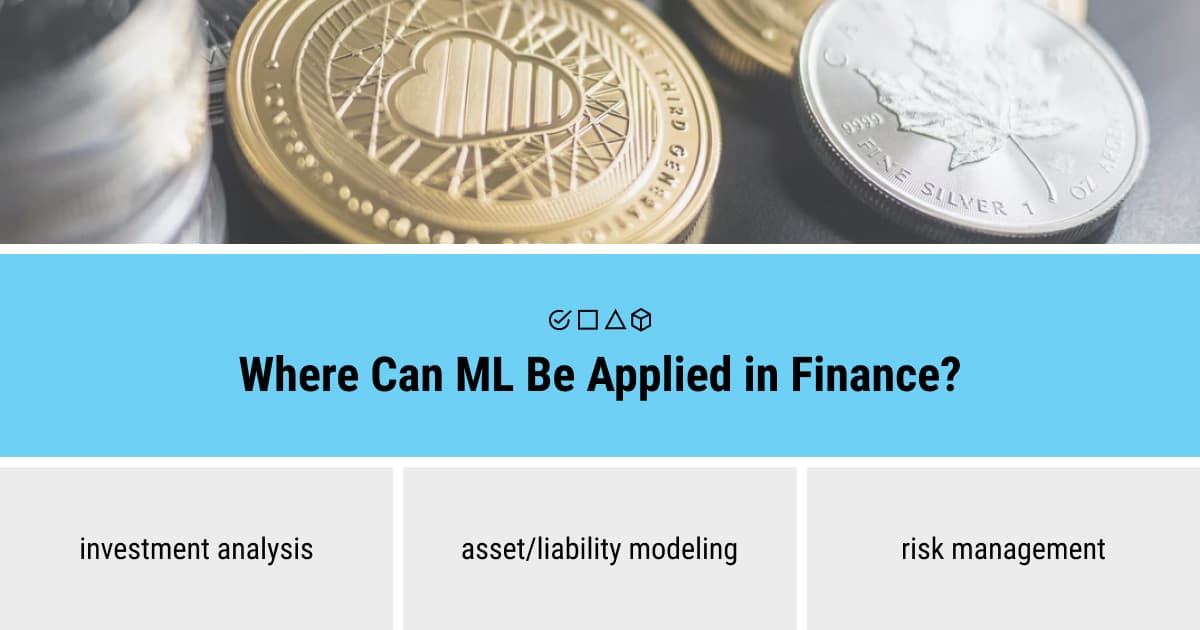
The modern finance and banking market is complex. It covers a broad spectrum of banks, insurance companies, accounting firms, investment organizations, and real estate firms. Thus, applications of ML in this sector are versatile. For instance, computer vision services are often applied in the analysis of graphical data, including charts and graphs, for predicting market trends and stock price fluctuations.
The efficient integration of machine learning and finance provides decision-makers with radically new methods of modeling and prediction that are efficiently applied for investment decisions, asset pricing, and risk management. Alongside its primary functions, machine learning offers the potential for additional services, broadening its impact on the finance industry.
Let’s look at these financial subfields in more detail and see what ML methods can be used for each:
Investment Analysis
- Investor sentiment analysis: Support Vector Machine (SVM)
- Technical analysis: Quantitative Analysis (QA)
- Investor behavior analysis: Behavioral Finance theory
- Investor decision support analysis: Calculation + iteration capabilities of ML
Asset/Liability Modeling
- Investment portfolio optimization: genetic algorithms and artificial neural networks (ANNs)
- Volatility modeling: Random Forest and neural networks
- Financial forecasting technology: recurrent and cyclic neural networks
Asset/liability modeling is based on the powerful forecasting capabilities of machine learning.Risk Management
- Risk assessment: Decision Tree, neural networks, Game Theory models
- Risk prediction: Decision Tree, neural networks
- Operational risk management: learning from known risk cases to build appropriate ML models
- Credit risk modeling: ANNs and traditional credit modeling method (linear regression, logistic regression, and unit probability regression)
Machine Learning Projects in Finance: Label Your Data Shares Its Experience
How to succeed with an ML project in the world of complex financial affairs? Well, certain things are required from the financial incumbents involved in this process, as well as from data scientists and ML engineers.
Any ML project, especially in finance, relies on a clear vision and solid technical expertise. It is quite hard to develop an efficient ML model without these two elements. It also takes a strong determination from banking employees to be willing to learn this discipline and quickly adapt to changes. As a result, one can succeed with an ML project in finance by building effective data infrastructure, collecting necessary financial datasets, and applying the right ML algorithms.
But after all, what is success without challenges? We at Label Your Data know all the pitfalls of annotating financial data. Some of the machine learning use cases in finance we’ve been working on include:
- Text classification of bank transactions for Named Entity Recognition
- OCR of financial documents and invoices for improved user experience
- Data collection for building the cryptocurrency chatbot with blockchain technology
Labeling invoices is, by far, among the most popular requests that we’ve received from our clients in the context of high-quality and secure NLP services.
Summary: Can Machine Learning Algorithms Build a Neo-Finance Era?
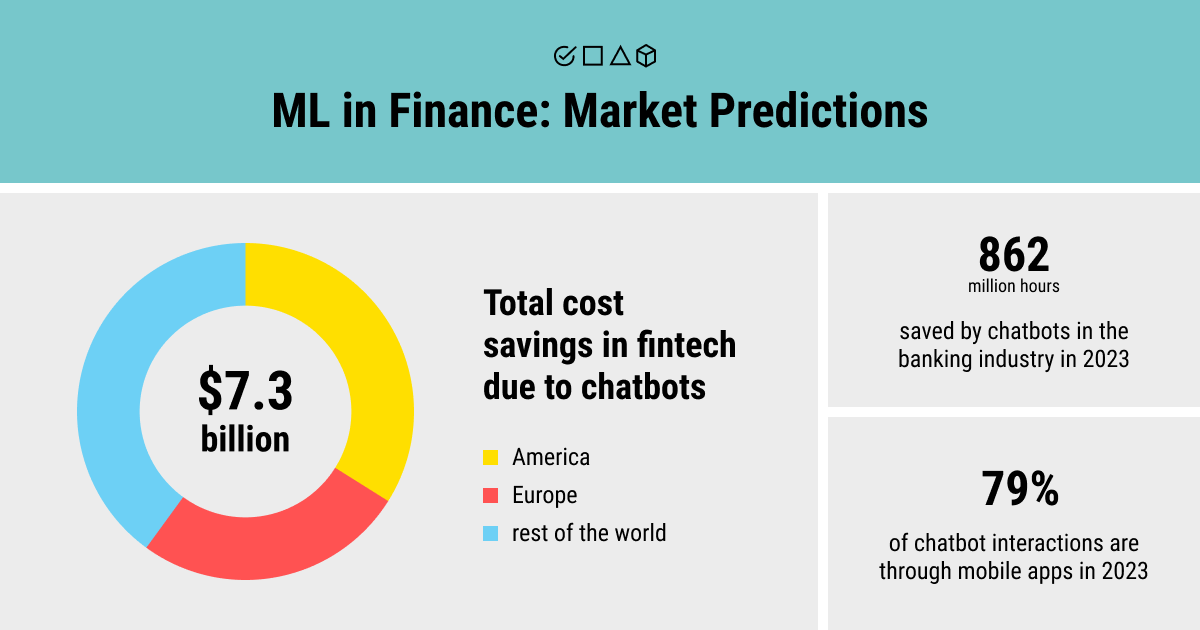
Finance has always been under the clear guidance of great minds who were in charge of important financial decisions. They shaped the finance and banking industry that we see today. However, human intelligence proved insufficient for the modern industry that seeks to follow the burgeoning pace of AI and digitization.
It’s impossible to succeed in this sector today without understanding advanced strategies across dynamic capital markets, like machine learning in financial services. ML methods play a pivotal role in various financial processes, so companies must stay agile enough to learn the trend and know how to use ML correctly to yield positive results in the market.
We at Label Your Data believe that it’s high time to start using capabilities of machine learning for financial services. When it comes to annotating your data, we ensure security, quality, and transparency, which is something that finance places a premium on.
FAQ
Do investment banks use machine learning?
Investment banks frequently leverage machine learning techniques to facilitate diverse functions such as refining trading strategies, evaluating risks, detecting fraudulent activities, and conducting customer analytics.
Is machine learning used in FinTech?
Machine learning is a cornerstone of FinTech, powering advancements in fraud detection, personalized financial recommendations, efficient trading algorithms, and risk assessment, thereby reshaping the landscape of modern financial services.
To what extent does ML impact and shape various aspects of the finance industry?
In finance, ML models can spot potential threats and help reduce foreseeable risks in capital markets. More specifically, banks and financial establishments harness this technology to enhance customer service through personalized solutions, streamlining operations, and saving customers valuable time and effort through process automation.
Written by
One of the technical writers at Label Your Data, Yuliia has been gradually delving into the intricate aspects of AI. With her strong passion for the written word and technical expertise, Yuliia has developed a keen interest in the evolving field of data annotation and the power of machine learning in today's tech-savvy world. Check out her articles to learn more about the complex world of technology and find the solutions that work best for your AI project!