In-House vs. Outsourcing Data Annotation: What Fits Your ML Project Best
Table of Contents
- What ML Challenges Can In-House Data Labeling and Outsourcing Data Labeling Solve?
- Comparing Outsourcing vs. In-House Data Annotation: Scale Up or Partner Out?
- When Outsourcing Is the Smart Move to Make?
- Why Choose Label Your Data?
-
FAQ
- In what ways can outsourcing data annotation benefit my ML project?
- What are the trade-offs between building an in-house data labeling team and outsourcing the process?
- When deciding between in-house data labeling and outsourcing data labeling, what factors should I prioritize to ensure quality training data for my ML model?
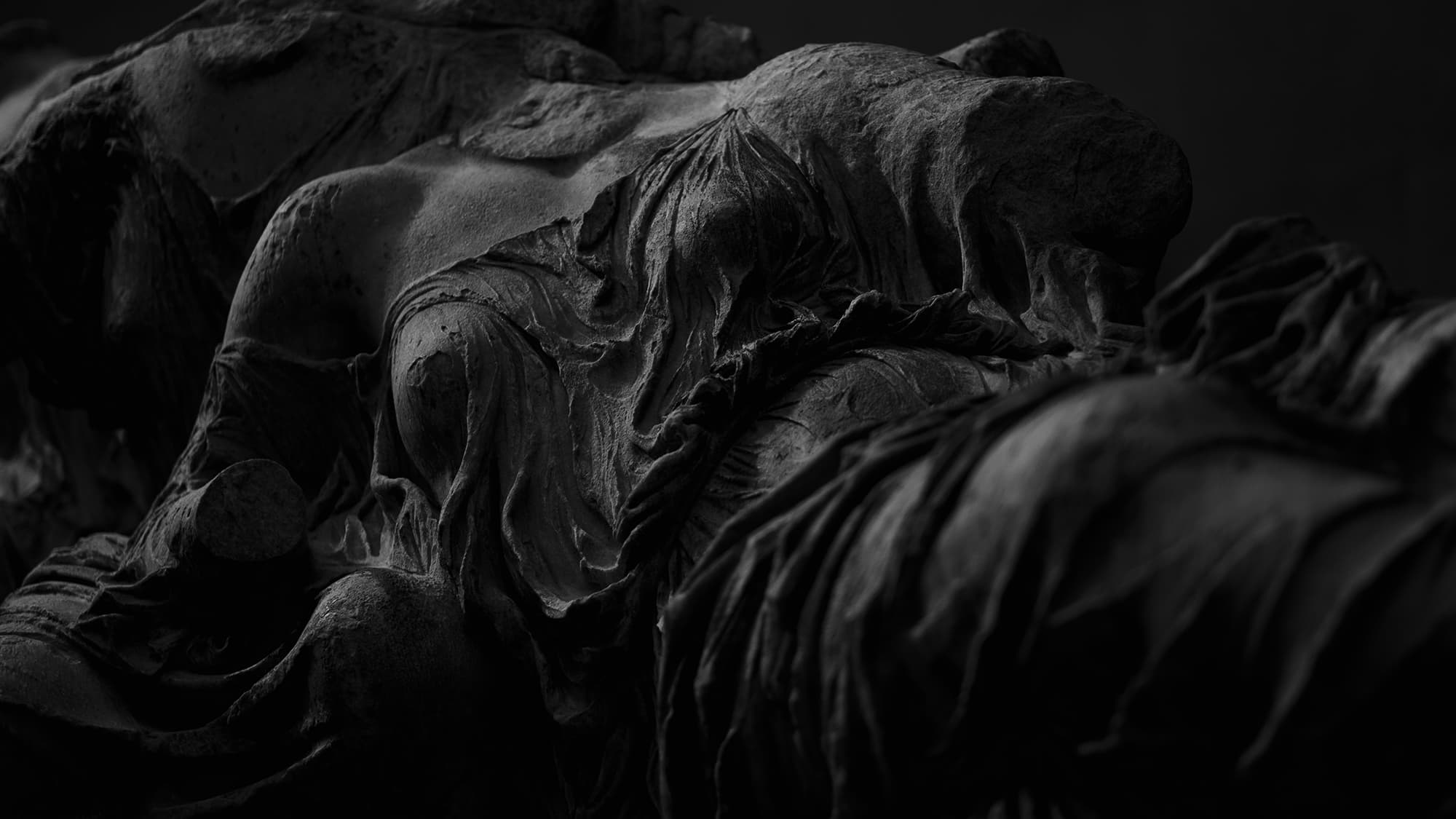
AI progress empowers businesses but creates choice overload. Picking the right data annotation strategy can be surprisingly complex, even for experienced data scientists and other ML project stakeholders.
Machine learning requires skilled specialists to handle growing data volumes and complex goals. Besides, because machine learning relies on iterative data labeling, companies need an adaptable data annotation strategy with efficient methods and feedback loops. This can be achieved through:
Building an internal team: Hiring data annotators in-house.
Outsourcing: Partnering with a data annotation vendor.
We’ll delve into both in-house data labeling and outsourcing data labeling to help you choose the right strategy for your ML project.
What ML Challenges Can In-House Data Labeling and Outsourcing Data Labeling Solve?
What matters in data labeling for machine learning is accuracy, security, and precision. ML projects grow fast when carried out efficiently, and here, the choice arises: in-house vs. outsourcing data annotation.
The majority of data scientists’ work involves working with training data. It has to be properly annotated so that it can be effectively used for a specific use case in machine learning. However, it takes a skilled manpower to perform high-quality data annotation and tackle the following annotation challenges:
Accuracy and consistency: Companies often lack in-house expertise in machine learning and dedicated annotation teams. Assigning annotation tasks to existing personnel, like business executives or industry specialists. However, if they aren’t properly trained, they might not follow the exact instructions, leading to mistakes.
Scalability: Labeling a small batch of data for a new project is feasible. But as projects grow and require more training data, data annotation can quickly become overwhelming. This can overwhelm small and medium-sized businesses, who may struggle to scale their annotation efforts efficiently with limited resources.
Cost and time pressures: As the demand for AI applications intensifies, companies face mounting pressure to deliver solutions swiftly and cost-effectively. Inefficient annotation pipelines lead to project delays, resulting in lost opportunities and additional expenses.
There are two options to overcome these annotation challenges: building a team in-house or outsource data annotation. Let’s delve deeper into each approach.
With a strong applicant pool, a full-time team of experts, and a QA team, we can take on various annotation projects with robust strategic management from our side. Contact us!
In-House Annotation Team
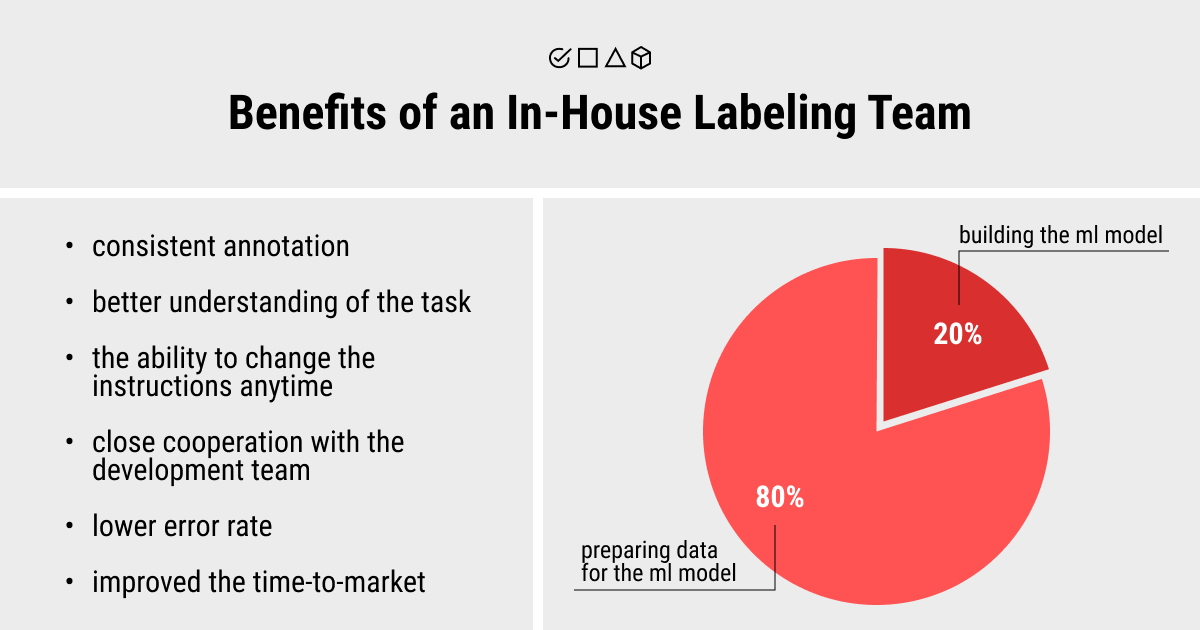
In both in-house data labeling and outsourcing data labeling, never disregard data privacy and security underpinning most processes in ML. This means deploying data labeling security strategies to handle massive volumes of sensitive data. Also, some labeled data cannot be transmitted online. And in such cases, having an in-house team makes sense in terms of security and control over your data.
Having your own team of data annotation specialists is great, but it goes hand in hand with tons of human resources to onboard, finances to spend, and time and efforts to devote. Data annotation is deceptively easy, but managing a labeling expert is a commendable effort for the company.
Hiring and training an in-house annotation team is the right thing to do when your ML project is long-term and includes large datasets. This also ensures that the project is carried out safely, following the highest data security standards.
To build an in-house labeling team, you need to:
Allocate HR and financial resources
Develop a labeling tool or use ready-made solutions
Build a QA team for error risk reduction
Supervise the annotation team
To lead an internal labeling team effectively, the company must strike the right balance between strategic development and high-quality performance of labeling tasks. Still, it’s not a scalable solution due to operational issues and insufficient training data expertise, unless it’s managed by a tech giant. If not, a third-party support from data specialists, like we provide at Label Your Data, might be a smarter choice to make.
Pros of in-house annotation | Cons of in-house annotation |
Consistent, reliable process for long-term success | Not practical for all data/company sizes |
Continuous improvement through feedback loop | Expensive and time-consuming to set up |
Strong quality control and lower error rate | Requires investment in finding the right tools |
Choice of existing tools or in-house development | May require a large team for complex or large data |
Data Annotation Outsourcing
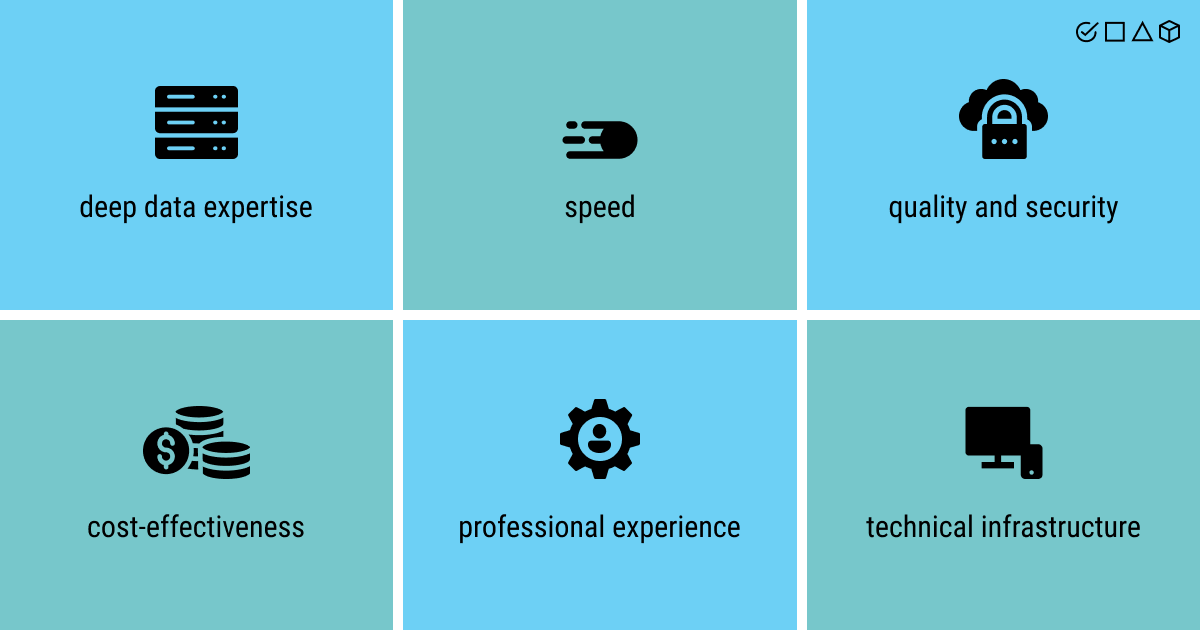
When comparing quality between in-house and outsourcing alternatives, the latter is often considered the most time- and cost-effective way to handle complex ML projects. If a company decides to outsource the labeling tasks to a third party, all the burden associated with an in-house option immediately casts off.
Most companies specializing in data annotation have state-of-the-art tools and software that allows clients to review their tasks and monitor the progress. Professional outsourcing partners also provide customized solutions to satisfy different ML projects’ needs.
Outsourcing data entry services, data labeling, or any other data-related service works well when there’s a clear vision of the rules and standards for the training data used to train the algorithm for a specific use case. Yet, the lowest price can undermine the quality and confidentiality of the annotated dataset, which directly affects the ML model. So pick your outsourcing partner wisely.
Besides, outsourcing inevitably entails the issue of finding a reliable and trustworthy service provider for labeling datasets whose service is built around the highest data security and privacy standards.
Pros of data annotation outsourcing | Cons of data annotation outsourcing |
High-quality work through hand-selected workforce | More expensive than crowdsourcing |
Cost-effective compared to in-house teams | Knowledge transfer limited due to external workforce |
Tailored solutions through consultation | Setup time can be lengthy depending on data complexity |
Efficient handling of large, diverse data volumes | Professional approach might be overkill for simple projects |
Strong security protocols |
Trust data annotation to the seasoned pros and secure your ML workflow. Send your quote!
Comparing Outsourcing vs. In-House Data Annotation: Scale Up or Partner Out?
Both options have their own benefits, but your final decision when choosing between in-house and outsourced data annotation would depend on factors like flexibility, pricing, management, training, security, and time:
Factor | In-House | Outsourced |
Flexibility | Suitable for simple ML projects where internal control and easy communication are crucial. Yet, scaling up for complex projects can be challenging. | Ideal for complex ML projects with specific labeling needs, offering access to a wider range of expertise and diverse datasets. Flexibility might be limited by the vendor’s capabilities. |
Pricing | Expensive due to infrastructure and training costs, including hiring dedicated staff, procuring software, and maintaining hardware. While cost-effective in the long run for high-volume projects, upfront costs can be significant. | Generally more affordable with various pricing plans based on data volume, complexity, and turnaround time. Finding the right balance between cost and quality requires careful evaluation of vendors. |
Management | Requires significant investment in time, money, and resources to manage an in-house team, including recruitment, training, performance evaluation, and QA. This can divert resources away from core development activities. | Frees up internal resources to focus on core development activities like ML model development. However, managing a vendor relationship also requires effort, including establishing clear communication channels and monitoring performance. |
Training | Requires significant time and money for training annotators on specialized tools, project-specific guidelines, and QA processes. This can delay project timelines and impact initial costs. | No training costs as data labeling service providers have experienced teams that can adapt quickly to project requirements and tools. Yet, ensuring consistency in annotation quality might require additional oversight. |
Security | Offers higher data security as project details and sensitive data remain within the organization. This is crucial for projects involving confidential information or regulated industries. | Lower inherent security risk as data is shared with a third party. Choosing providers with robust security protocols, data encryption, and compliance certifications is essential to mitigate risks. |
Time | Generally slower due to the time required for team training, infrastructure setup, and initial project setup. This can be a downside for projects requiring fast turnaround times. | Faster due to established provider infrastructure and readily available skilled team. This can be great for projects with tight deadlines or ongoing data annotation needs. |
When Outsourcing Is the Smart Move to Make?
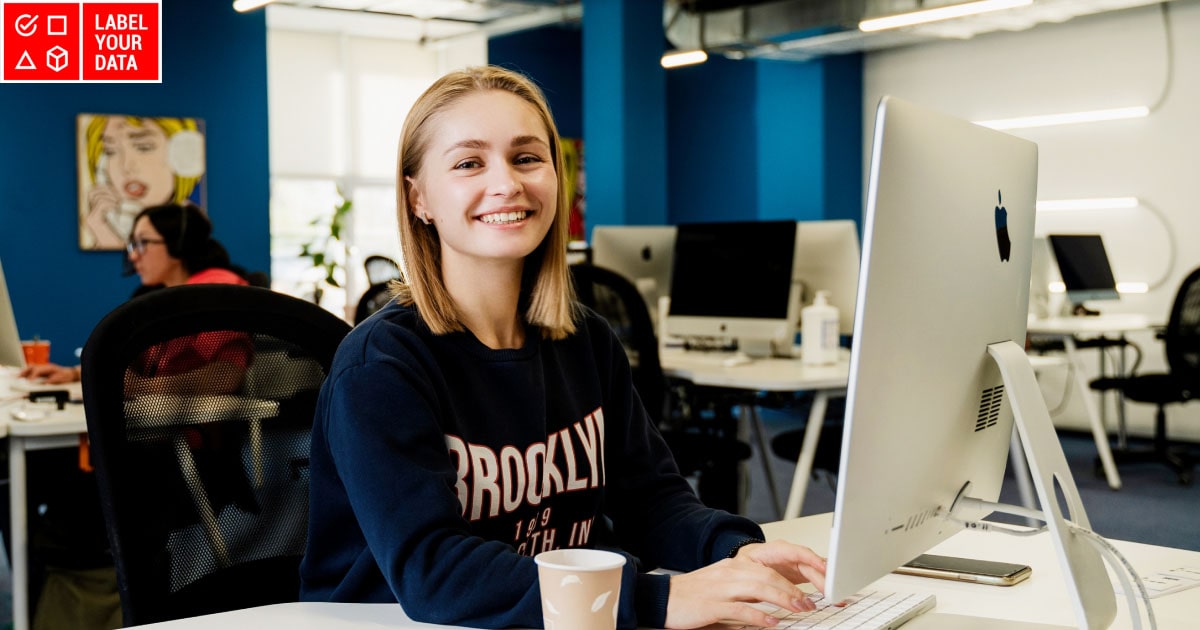
Performing data annotation in-house vs. outsourcing data annotation becomes more complicated when the project scales up. The most common issues the internal team encounter are:
A lack of vision and understanding of data annotation and its methods
Insufficient time, finances, and HR capabilities
Managing a large team of data annotators
Providing consistent, high-quality annotations
Implementing the right tools and technologies
Complying with data security and privacy standards
At this point, outsourcing data annotation can tackle these challenges:
Outsourcing allows you to focus on what matters
Training ML models requires properly annotated data, a crucial but time-consuming task. Assigning skilled data scientists to this repetitive work hinders their ability to tackle more complex problems. You can streamline the development process and free up your experts by outsourcing your data annotation project, so that they can focus on building powerful ML models.
Outsourcing guarantees quality and efficiency
One of the main advantages of outsourcing data annotation is that it ensures timely completion and high standards. Dedicated teams with extensive experience handling diverse datasets handle your project, applying their expertise to deliver accurate and efficient results.
Outsourcing allows scaling effortlessly
Annotating vast amounts of data can be overwhelming. In-house teams may struggle with fluctuating workloads, leading to delays and requiring additional resources from other departments, impacting overall productivity. Outsourcing allows you to scale your data labeling efforts seamlessly, regardless of the ML project size.
Whichever way you go, in-house or outsourced data annotation, always ensure that your instructions are clear and detailed, and that annotation experts are fully committed to your ML project and meet data security standards.
Why Choose Label Your Data?
The decision between outsource vs. in-house data annotation rests with you, yet for optimal results, entrust the task to the pros. Our outsourcing strategy at Label Your Data has helped many companies to scale their ML projects. And here’s why:
Security
A reliable annotation service provider is the one that follows a unique approach to each ML project and puts data security above all. Our data labeling and data processing services are ISO/IEC 27001:2013 certified and GDPR and CCPA compliant.
No commitment
We offer our clients a free pilot to estimate the project’s requirements and deadlines, test the quality, and demonstrate our labeling capabilities before moving on to a full collaboration.
Tool-agnostic
Better and faster data labeling at scale requires novel solutions, which is why we focus on integrating semi-automated annotation.
If you choose to delegate the data labeling operations to our specialists, you’ll avoid a lot of stress and adverse business outcomes you might face on your way up in AI.
FAQ
In what ways can outsourcing data annotation benefit my ML project?
Outsourcing data annotation can greatly enhance your ML project by helping you to:
Gain access to a diverse array of specialists
Expedite project timelines with faster completion times
Lower expenses compared to in-house annotation
Tap into a larger, potentially global talent pool
Elevate the quality and consistency of annotations
Yet, keep in mind that these benefits apply only to trusted data annotation vendors.
What are the trade-offs between building an in-house data labeling team and outsourcing the process?
Choosing between an in-house labeling team and outsourcing involves trade-offs between control, cost, and scalability. Building your team offers greater control over the process but comes with higher costs and potential scaling challenges. Outsourcing is generally more cost-effective and scalable, but may involve less control and potential security risks. Ultimately, the best option depends on your specific project requirements and priorities.
When deciding between in-house data labeling and outsourcing data labeling, what factors should I prioritize to ensure quality training data for my ML model?
To ensure high-quality labeled data for your AI project, consider both cost-effectiveness and data security when deciding between in-house data labeling and outsourcing. Weigh the expertise and scalability of each option against potential control and privacy concerns for your specific project needs.
Written by
Karyna is the CEO of Label Your Data, a company specializing in data labeling solutions for machine learning projects. With a strong background in machine learning, she frequently collaborates with editors to share her expertise through articles, whitepapers, and presentations.