AI Sound Recognition: Can a Machine Listen and Hear?
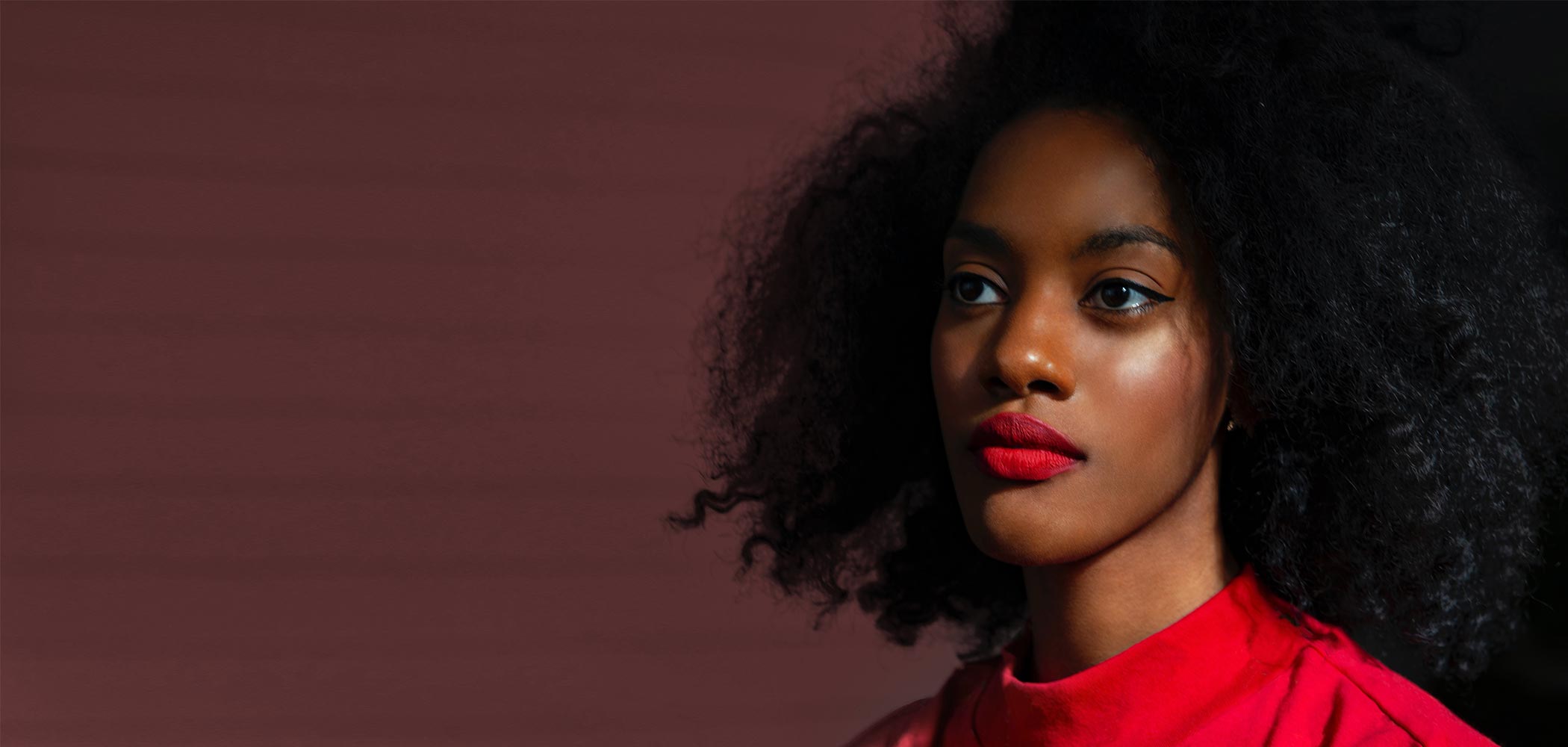
During the last couple of years, artificial intelligence has become a household name for businesses in any industry on the market. If there’s data to be collected and utilized, an organization can benefit from adopting AI as part of its workflow.
The type of data specifies the tasks and, in turn, the type of algorithms to be used. Recognition is arguably among the loudest and flashiest of these tasks. Yet, ironically, among the wide variety of types of recognition algorithms, there are none as quiet and taciturn as sound recognition AI.
When we think about recognition AI, we think of image recognition (whether it’s detecting faces or emotions), OCR that helps to decipher symbols (words and numbers) and turn them into editable, printable text. However, if we dig deeper, sound recognition is everywhere nowadays.
- It’s in your home assistant, listening to your requests to turn on the specific track and telling you jokes when you’re feeling down.
- It’s in automation models that allow the transcription of tedious, unending meetings into succinct business reports.
- It’s in CRM systems that use a specific set of algorithms to gather the most vital information about a customer and use it to their (and company’s) benefit.
We really don’t think about sound recognition AI since it’s something so essential to human beings: to listen and be heard. So let’s take a look at how we can help a computer hear as we do and if it’s a way for better communication between people and the machines or even for improving inter-human communication.
Sounds in AI Recognition: Why Is It Done?
While we might not think of it as anything groundbreaking, sound recognition actually plays a tremendous role in both the comfort of our modern lives and in the further development of humankind. It offers a surprising number of opportunities for practical implementation.
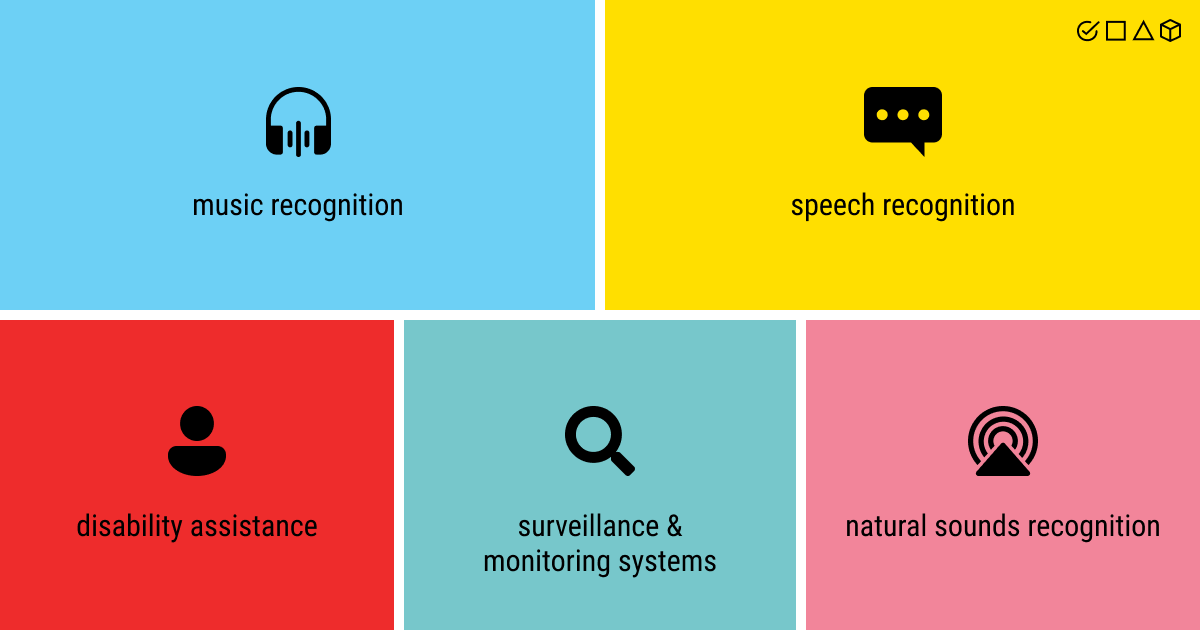
Here are a few groups of different sound detection algorithms that could give you an idea of the flexibility and versatility of this type of recognition model:
- Music recognition. Probably the one that each of us is most familiar with, music recognition refers to the algorithms that are able to detect and classify music. These models can be quite models, like something that puts a label of a specific genre on a composition. Or it could be an intricate and world-renowned algorithm like the one in the basis of Shazam, our beloved music-recognition software.
- Speech recognition. Another wildly popular class of sound recognition algorithms, it can be found in a variety of contexts. In business, it may serve to record the speech during a meeting. In private lives, it’s used for voice recognition in virtual assistants (chatbots) and home assistants like Alexa or Siri.
- Disability assistance. Hearing loss may be a great tragedy for a person living in an acoustic world. That’s why hearing aids or activity recognition for aging users created with the help of sound recognition models can be profoundly impactful if not essential for people with such disabilities. Manufacturers of household objects and gadgets have long started to implement such features into their products. For example, only recently Apple has introduced a new Sound Recognition Accessibility Feature for their iOS 14.
- Surveillance systems. This class of sound recognition models can be divided into two: sound monitoring and sound verification. As the attention to security grows, sound systems that are able to detect the variety of automated monitoring signals (such as alarms, sounds of break and entering or violence, and even unusual sounds for industrial settings) are all in high demand. On the other hand, the development of the technology of acoustic fingerprints is underway to become another important component of both private and business security. In addition, sound recognition has a lot of potential as a part of robust AI projects such as video analytics and event detection.
- Natural sounds recognition. These sound detection models, although not as common as the previous ones, offer the opportunity to study the surrounding world and learn more about the different species that live beside us. Natural sound recognition technology is commonly used in oceanography, weather forecasting, thermometry, etc.
While this list is not exhaustive, it gives a general understanding of how wide the array of possibilities for sound recognition is. Yet there’s another question to answer: how does this AI magic work?
AI Sound Recognition: How Is It Done?
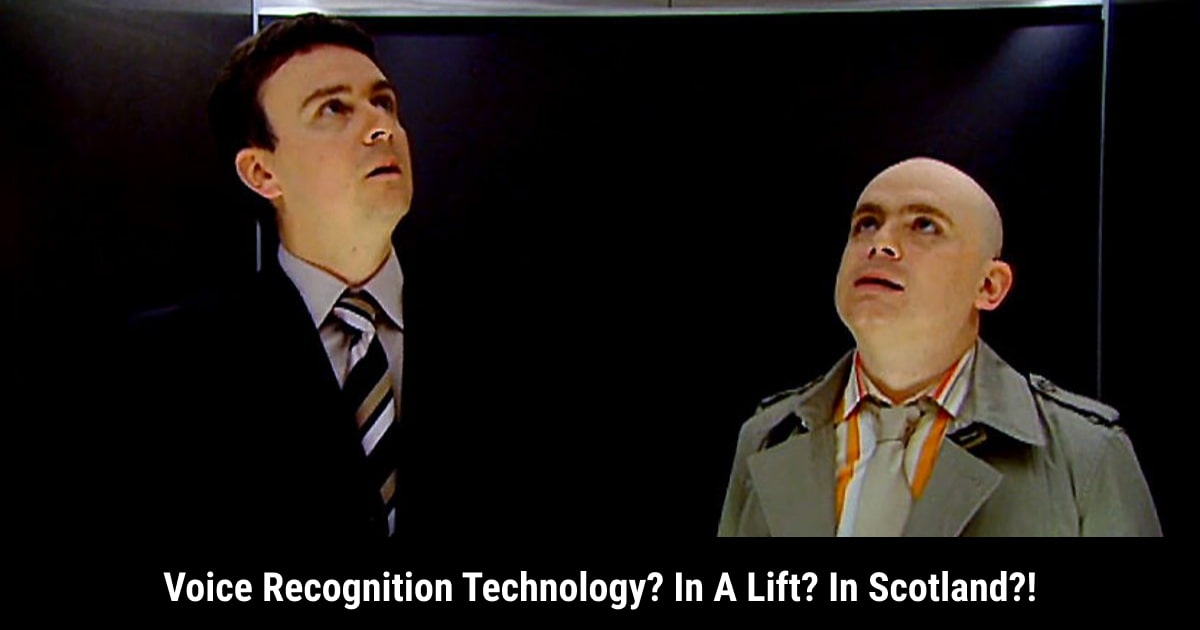
The multiple sound recognition tasks all have a slightly different approach but the core is all the same. Here’s a list of steps that every sound recognition algorithm goes through:
- First of all, a sound recognition model records the sounds that it hears. They can be in different forms, live or already recorded. This step is essential as it allows the model to create a foundation for further processing of sounds.
- Next, the model analyzes the recordings. This means that the recorded sounds are compared to the data the model was trained on. The better the training process was and the higher quality the data, the faster and more accurate the prediction will be.
- Finally, the model provides meaningful output. This can be anything from finding an answer to your request to transcribing speech into editable text to looking through the database of similar sounds using the classification method to pinpoint the source of the sound.
Audio-to-Text Transcription
A similarly simple task like audio-to-text transcription that we take for granted in our home assistants and chatbots takes at least three major steps to convert a human input (a question or a request) into machine output (an answer).
ASR (automatic speech recognition) is used to break down the speech (either live or recorded) into segments. Then, the algorithm analyzes the sounds using NLP (natural language processing) and predicts what words in a given language it may correspond to. This allows the machine to find the appropriate answer to the user’s request. Finally, TTS (text-to-speech) converts the answer into a human-like speech.
So, when you ask Siri to tell you a joke next time, don’t be annoyed at the substance of the joke. Keep in mind that the algorithm under the hood of your smartphone undergoes a complex sound recognition process just for your entertainment.
Data Annotation for Sound Recognition AI
Building a sound recognition algorithm is quite a complex task as it requires a few essential steps to take place. On one hand, you need a team of developers and data engineers to design a machine learning model. On the other hand, most of your time will be spent on collecting, analyzing, processing, and annotating the data.
The latter part is often overlooked by data engineers since it’s a less creative, more tedious, and much more laborious process than developing an ML model. However, in order to proceed with algorithm training, you need to make sure you have a good, high-quality and voluminous, dataset.
Data annotation specifically is an essential process of adding labels to the data pieces. This is important because machines do not analyze sounds the same way humans do. The labels are required to teach the machines to understand what we want them to hear and what those sounds mean.
Since this is a very time and effort-consuming task, a lot of companies tend to look for annotation partners. This saves the businesses the need to tear their focus away from the core product and helps to prioritize the creative processes. If you need help with data labeling for sound recognition, contact us at Label Your Data, and we’ll lead you through all the pitfalls smoothly.
Conclusion: Sound Recognition as a Universal Tool
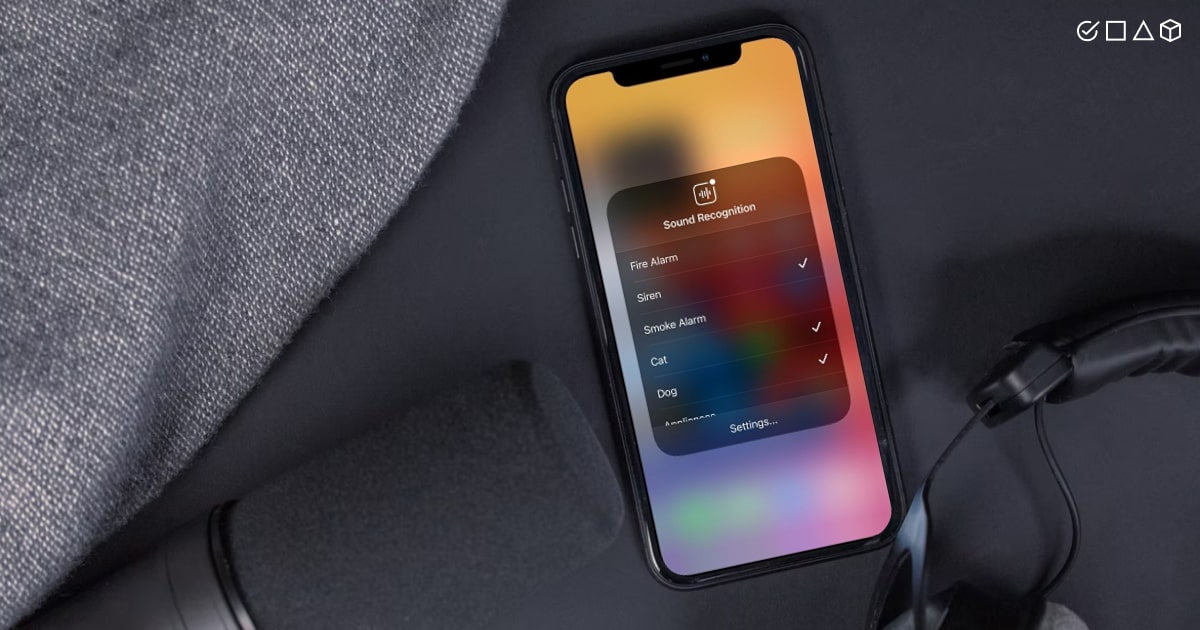
We may not think about sound recognition as a particularly interesting technology. However, its uses today are very wide. In your phone and home assistant, in CRM systems, in business chatbots, and in scientific equipment, you can find sound recognition algorithms nearly anywhere.
It’s a tool that helps people better communicate with machines. With the advent of personal virtual assistants, it became possible to use the power of a computer to facilitate everyday lives and put our minds at ease when it comes to routine tasks.
Sound recognition technology also helps us stay connected with other people. The application of such algorithms as personal translators and disability assistants indicates that sound recognition AI can bring us a step closer to better understand each other.
Written by
Karyna is the CEO of Label Your Data, a company specializing in data labeling solutions for machine learning projects. With a strong background in machine learning, she frequently collaborates with editors to share her expertise through articles, whitepapers, and presentations.