Data Annotation and Health Crisis – A Dynamic Duo?
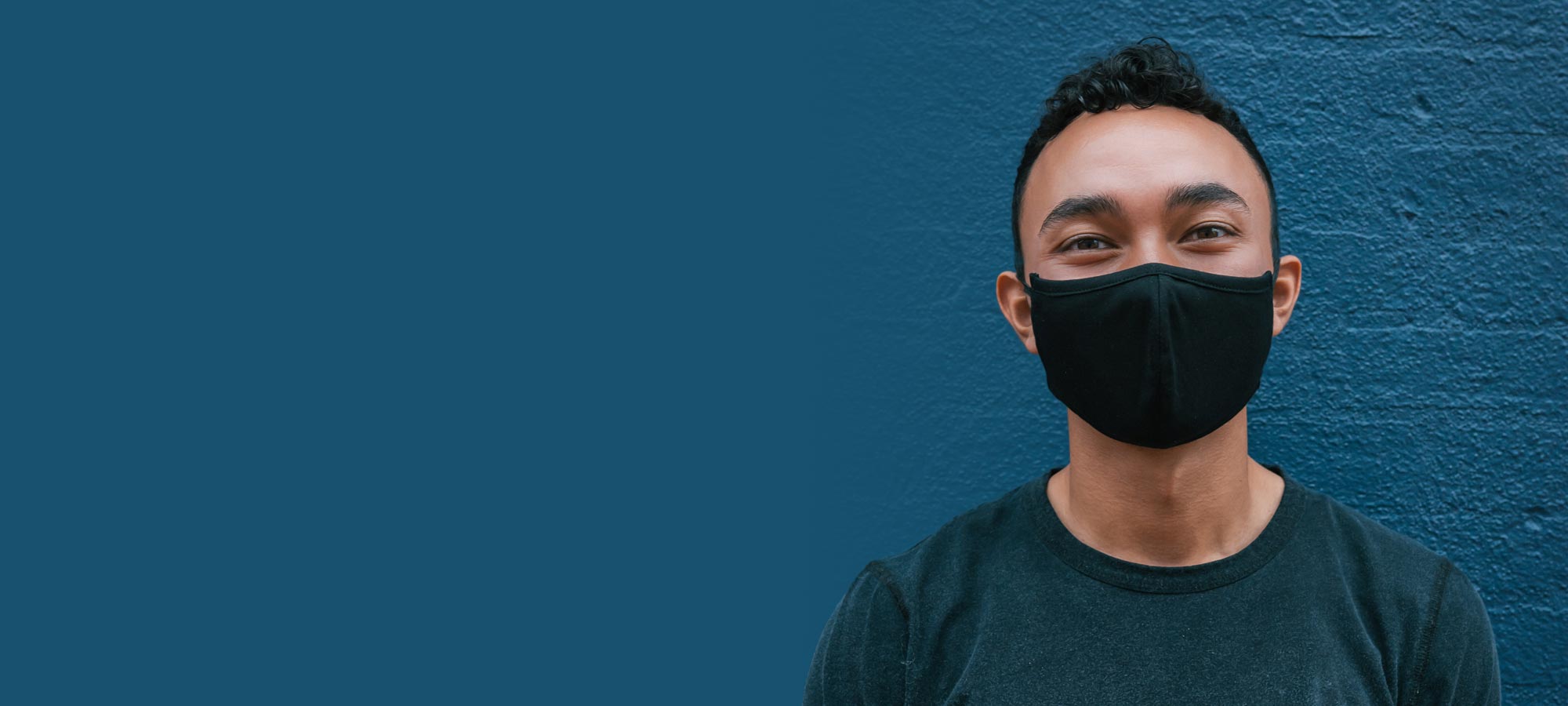
At the outset of the new digital paradigm, artificial intelligence was half a gamble of creating the thinking machine and half a problem-solving invention for humanity. In a decades-long journey, advanced technology managed to fulfill most of our needs and even augment the reality that we live in today.
Such changes are quite pronounced in the medical sector. The last two years, however, have made AI reclaim one of its initial purposes – helping humans face the unforeseen public health crisis caused by the COVID-19 pandemic. The role of data, i.e., health data, has resounded more loudly than ever.
While data annotation hardly ever appeared in the news about AI and COVID-19, it has, too, figured prominently in the process of combating the virus. Despite the scarcity and heterogeneity of medical records, data annotation has contributed to the global mission of fighting the pandemic of coronavirus disease. Let’s have a look at how things went!
What Challenges Came AI’s Way During the Pandemic?
We see the COVID-related statistics all over the media, which is the public health data of confirmed cases, hospitalization, tests, and deaths collected almost second by second. Here, data is used to demonstrate the life-threatening situation on a global scale for the last two years with daily updates.
For the record, the daily reporting on the COVID-19 crisis provided by health authorities is being collected from 181 countries. Given such international and quantitative coverage, this process would be almost impossible without expert help from data annotators.
How has data annotation helped countries and businesses in faring with COVID-19? We should first go through AI solutions during the pandemic, and then discuss the data annotation component, without which this whole AI system wouldn't be viable in the first place.
AI holds many promising capabilities to mitigate the disease and control its accelerated spread. Still, finding the novel approaches to AI throughout the pandemic comes with a handful of obstacles and limitations involved with AI implementation into the COVID-19 research:
- The scarcity of standard datasets. Standard data is required to devise an effective solution against the virus.
- Cross-validation of the trained models. This eliminates the biases that affect government officials’ and healthcare organizations’ decisions and actions.
- Data privacy and security. Patient data such as X-ray pictures, CT scans, MRI, travel history, anamnesis, GPS position, and regular activities must be rigorously protected in light of the pandemic.
- Inconsistent pandemic data patterns. Variability in the dataset occurs as a result of different approaches of healthcare institutions, undermining the credibility of predictive modeling for COVID-19.
- Resemblance of symptoms. While the disease keeps spreading, it remains complicated to detect the best ML models to analyze the COVID-19 cases because of the similar patterns in symptomatology.
- Anomalies. Given the contradictory nature of the coronavirus pandemic, anomalies have been detected in many naturally occurring datasets.
Data Annotation Strategies to Tackle the Pandemic-Related Challenges
Limited time and data availability made it harder to build AI-based algorithms that can be effective in controlling the spread of coronavirus disease. As the virus failed to respect our lives and safety, it was mandatory to deploy new approaches to AI to protect society.
In healthcare, data annotators mostly deal with medical images that, unfortunately, come in limited collections. As a result, data annotation becomes cost-intensive. And while many data annotation techniques have been proposed, this issue remains unresolved both for experts in the field and healthcare providers.
Nonetheless, data annotation played a crucial role in the course of the pandemic. The reason for that is the nature of the data itself. Without analyzing its context through labeling, data cannot provide any useful information and valuable knowledge that are especially important for medical settings.
The human input of data annotators gives meaning to the medical data so that the algorithm can generate trustworthy predictions. The COVID-19 pandemic has served as a major catalyst for the rapid progress of data annotation and has strengthened the collaboration between AI and the healthcare industry as a whole.
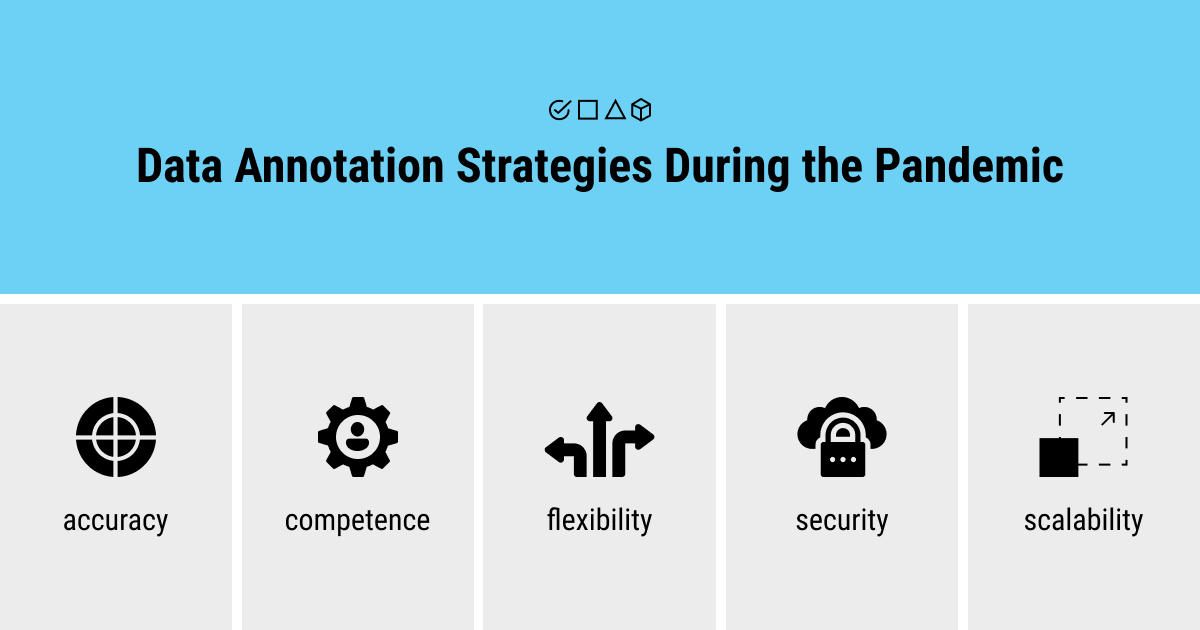
- Accuracy. The accuracy of data labeling ensures the effectiveness of the AI-based models.
- Competence. An expert data annotator knows the fine line between maintaining data quality and achieving better performance at lower costs.
- Flexibility. The main goal is to make labeled data agile to different tools and platforms, so it can fit any AI project at hand.
- Security. As data labeling experts ourselves, we can’t stress enough the importance of security compliance for any data-related work.
- Scalability. The team of annotators must be able to promptly and cost-effectively respond to changes in data volumes, given the scope of the virus spread.
However, there’s one important thing to explain: medical data annotation is a viable practice only when we can engage healthcare professionals or medical students in it. Let’s say you need to label tumors on a CT scan. Annotating medical data requires specialized knowledge from a healthcare professional. However, medical workers have limited time to dedicate themselves to data annotation, which, in turn, causes projects’ delays and higher costs.
That said, a highly specialized focus of the medical discipline urges the need for healthcare staff to be involved in data labeling. But this brings about an additional challenge to hire and keep the medical personnel at full disposal throughout the project.
COVID-19: How Data Annotation Came to the Rescue
With the coronavirus outbreak, the value of well-annotated and accessible data has never been more clear. Poorly labeled or unlabeled pandemic data results in compromised AI and questions AI-based models that are built to fight the pandemic, respectively.
In the data-driven environment we have, people are massively looking for and relying on COVID-related information from multiple online sources. They keep people updated, informed, and help them avoid panic. Such a soaring demand for pandemic data left data annotators no choice but to organize this information properly to provide useful insights for the society.
The manual type of data annotation was the most popular approach of AI in the context of the pandemic. Label Your Data team has carefully studied all the subtleties of this laborious process. We have expanded our domain expertise by helping our clients with:
- Data anonymization of medical dermatological data
- Text classification for COVID-related fake news detection
- OCR of medical records and diagnoses
- Medical image categorization and annotation of the blood cells
Let us know if you’re just getting started with a new AI project. Our expert team of data annotators at Label Your Data will find the most suitable option for you!
In 2020, the manual annotation has reached the highest revenue share, and that says a lot. It’s also worth mentioning the key attributes behind this process, including data accuracy, ability to grasp extreme cases, and intelligent manpower. Altogether, these attributes guarantee high quality, secure data annotation across large volumes of pandemic data.
Besides, manual annotation remains the most relevant tool used to train ML algorithms for computer vision applications because of the prevalence of medical imaging annotation. Now let’s get back to business and discuss data annotators’ contribution to mitigating COVID-19.
Data Annotators in the Spotlight
Why is it necessary to annotate data? Machine learning models always rely on trained annotated datasets. Trusting unlabeled data in the healthcare industry is a vain attempt at building effective, unbiased AI-powered innovative systems.
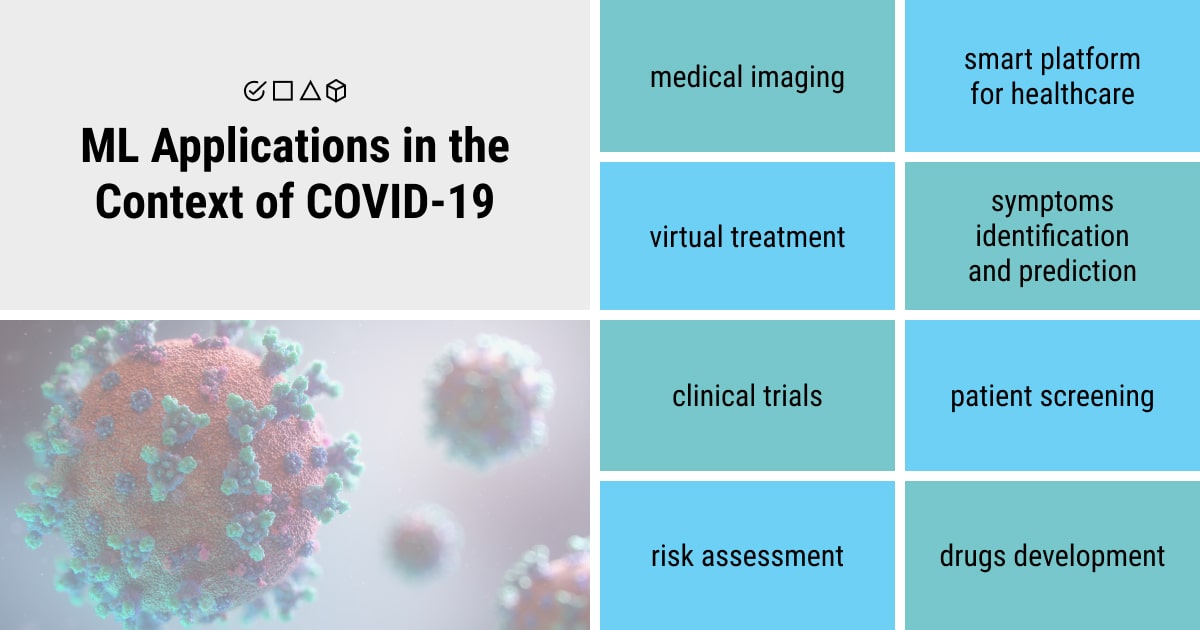
Based on the above-mentioned ML application during the pandemic, we can now understand the role of data annotators who were to improve the performance of the ML models and do their part in the fight against the virus. Besides helping society understand and respond to COVID-19, data annotation turned out to be an indispensable screw in the virus-fighting mechanism.
Here’s a list of data annotation solutions during the COVID-19 pandemic:
- Providing resources for the public sector to monitor the crisis
- Supporting COVID-19 academic and medical research
- Visualizing the global pandemic data for daily reporting
- Examining the global effect of COVID-19
- Analyzing the global news narrative about the coronavirus
- Assisting businesses in managing operations throughout the pandemic
- Lung and infection segmentation based on COVID-19 cases
- Ontology-based annotation and analysis of COVID-19 phenotypes
- Diagnosing, containing, tracking, and finding a long-term virus treatment
- Automatic analysis of CT images to detect COVID-19 pneumonia features
- Face mask and social distance detection and tracking with YOLOv4
- Tracking COVID-19 misinformation on social media
- Social media sentiment analysis towards COVID-19 vaccines
- Stigma annotation schemes for pandemic-related discussion on social media
- COVID-19 vaccine safety and effectiveness analysis
While data annotation contribution to the COVID-19 research has been quite vivid, this is not an exhaustive list of its capabilities used to counteract the virus. There are some interesting cases and data annotation tools applied during the crisis that are worth your attention!
Pandemic Data Annotation: Tools, Strategies, and Use Cases
Labeling data is a pivotal step for any AI project. Data has to be properly labeled before it’s fed into an ML algorithm. In healthcare, this strategy defines data quality and credibility that are of the utmost importance in this field. Annotated data is prepared for building complex predictive models for COVID-19 medical research.
Given the pandemic’s scope, AI researchers had to use every tool and technique available to track down the virus and develop new strategies to counter the disease. Here, machine learning methods proved to be fundamental. But, as with any novel disease, there is very little data to rely on, which makes data annotation far more difficult. Correctly annotated pandemic data to train ML models or diagnostic tools became tremendously important.
At Label Your Data, our goal is to forge ahead with effective developments in AI, not less significant than the global effort to address the coronavirus. Who knows what the future holds for us, right?
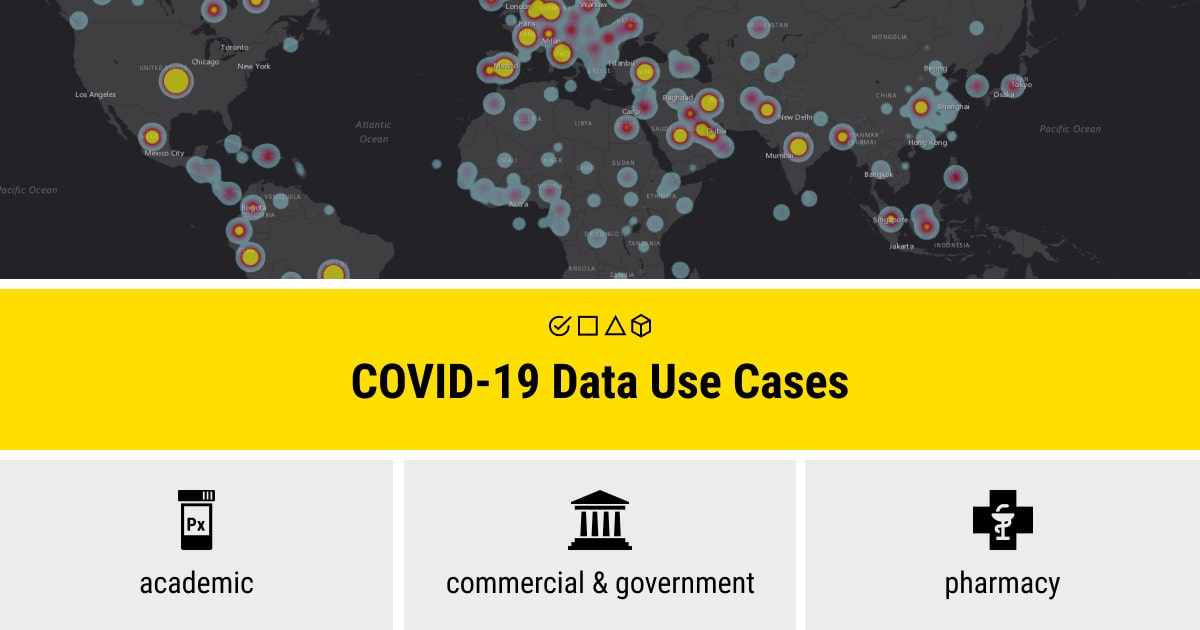
BlueDot
The first warning of the rapid virus spread in Wuhan, China, at its nascent stage, was sent by the AI-powered platform BlueDot. The software used data integration to prepare the pandemic data for the AI system based on NLP and ML. Data annotation with appropriate medical terminology is a crucial step in the data integration framework. By processing large volumes of data, the system even managed to detect the top twenty cities under a great risk of being impacted by the virus.
COVID-19 Global Tracking Map
A Johns Hopkins University project aimed at expanding the global awareness and understanding of the coronavirus pandemic. Data collection, annotation, and visualization have been conducted for the past two years. As a result, we now have access to 10.000 data points for 3500 point locations on an hourly basis, 24/7 time horizon. This is the prime example of how annotated data becomes a key component of a good data visualization.
COVID-19 Open Research Dataset (CORD-19)
The CORD-19 dataset has been annotated by the recognition tool called TERMite using COVID-19 focused vocabulary. The richly labeled dataset consisted of more than 45 million annotations with 62,746 unique ontology concepts.
Google Cloud
COVID-19 research from Google Cloud has also supported additional data annotation on the coronavirus pandemic and large-scale disease outbreaks. The study is comparing COVID-19 radio coverage on ten major U.S. stations using Cloud Speech-to-Text. The acquired dataset clarifies the television and radio coverage of the pandemic compared to its online exposure.
The COVID Tracking Project
Data labeling has been actively used for state reporting practices on the pandemic. In this project, annotations were organized by state and metric to improve decision-making and COVID-19 research on the governmental level. The ultimate goal was creating an extensive archive of COVID-related metadata to shed light on the state-level pandemic.
Open-Access Data Sources
There are several open-access data and computerized resources presented by federal authorities, including NIH, public consortia, and private entities. The sources showcase all types of data, including case studies, epidemiology, genomics, bioactivity, and visualization tools, to name a few. Annotated sources are provided as well to address the virus.
LitCovid
LitCovid is a first-ever, open-source, COVID-19-specific library. It’s a hand-selected scientific hub of up-to-date information about the pandemic, where data and their associated annotations played a major role in analyzing the pandemic research landscape and building a semantic network about COVID-19.
Predicting Data Annotation Trends for the Future
As we have explored the role of data annotation in the context of the COVID-19 pandemic, it’s time to assess the future chain of events in this industry. This will help devise new strategies and approaches to AI that will improve medical data annotation and prepare humanity for possible global health crises in the future.
Here are some critical takeaways from the data annotation market research from 2020 to 2030:
- Manual data annotation will hold its leadership position in the market
- Automatic options for data labeling are predicted to grow as well
- The text annotation segment is expected to skyrocket due to e-commerce and clinical research applications
- Audio annotation tools will face moderate development
- Video and image annotation will continue to lead the healthcare, retail, and automotive sectors
- Data annotation ecosystem will branch out, providing more options for AI
- The healthcare sector is anticipated to steadily grow by adopting AI training datasets
With that being said, data labeling is predicted to face an expanding reach in the global economy of the AI industry. This entails an increase in intelligent algorithms that will, in turn, reduce the reliance on manual techniques and lower operational expenses for users.
However, data annotators should beware of incomplete ML and AI data analytics infrastructure and accuracy concerns in this modern-day craft.
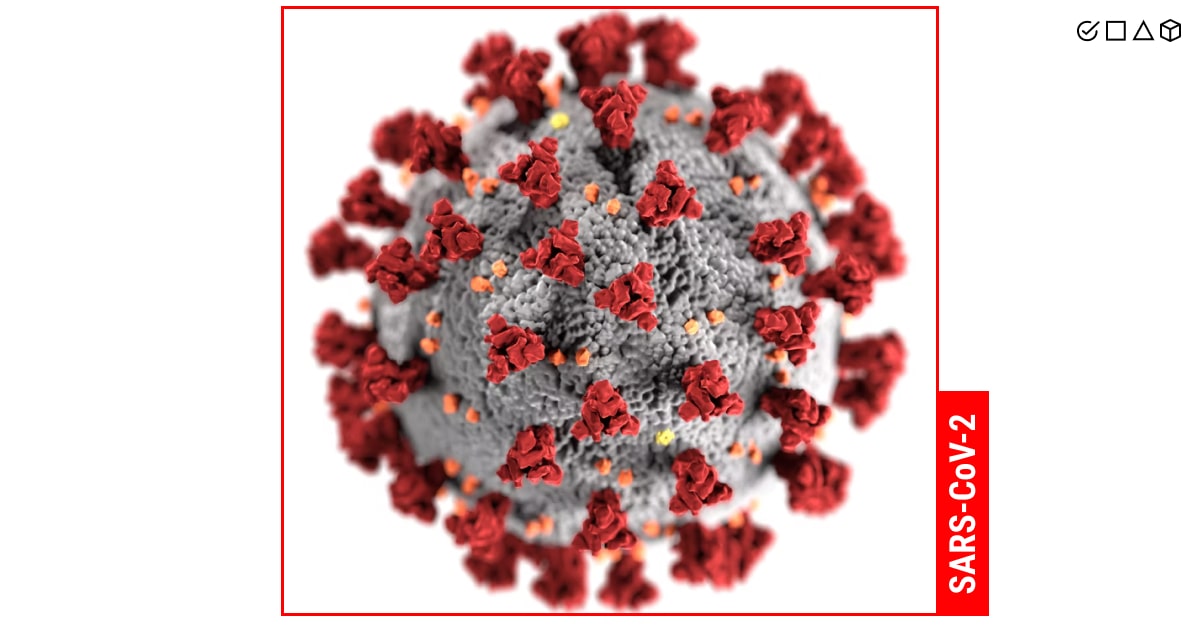
Final Thoughts: How a Nasty Surprise Turned Into a Global Fight for Life
Data is only as valuable as it is used. The COVID-19 pandemic has caught off guard every single industry worldwide. For data annotators, it was a significant challenge to handle enormous volumes of pandemic data and contribute to development of radically new approaches to AI in healthcare.
As the role of data has been accelerating, so has the need for smarter annotation tools and AI solutions to ensure accurate and reliable predictions. The coronavirus pandemic has not only pushed data experts to hone their skills, but also made healthcare actors raise their investments in AI-led medical research. So it’s a win-win game for both data annotators and healthcare providers working together to protect human lives.
Even at Label Your Data, we learned the hard way because of the pandemic. Our team has grasped the opportunity for devising new data annotation strategies and approaches in the overly complex data-intensive environment. Contact us to get more information about data annotation services we provide to assist you in your next AI project!
Written by
One of the technical writers at Label Your Data, Yuliia has been gradually delving into the intricate aspects of AI. With her strong passion for the written word and technical expertise, Yuliia has developed a keen interest in the evolving field of data annotation and the power of machine learning in today's tech-savvy world. Check out her articles to learn more about the complex world of technology and find the solutions that work best for your AI project!