- Automated Content Moderation: How Can AI Help?
- AI Content Moderation Tools & Methods
- Real-World Applications of AI Content Moderation: Insights from Industry Leaders
- Final Thoughts on AI in Content Moderation: Friend or Foe?
- FAQ
With the proliferation of social media, the issue of cyberbullying has emerged as a major stumbling block for platforms trying to maintain a safe online environment. Nearly 4 in 10 people (38%) bear witness to this negative behavior daily, underlining the pressing need for innovative content moderation strategies. Artificial intelligence is increasingly being employed to tackle this persistent issue head-on.
Users are at great risk of being exposed to inappropriate content with widespread internet access and the abundance of digital media. This usually includes flagged content and is categorized as violence, sexually explicit material, and potentially illegal content. Also, unlawful and offensive content may seriously affect the mental health of both users and moderators, which is why the content moderation process has become a major headache.
To take a breather, moderation teams are employing adaptable AI solutions to monitor the continuous stream of data generated by brands, customers, and individuals, guaranteeing a secure and non-offensive digital space. With that said, let’s dive deeper into novel approaches to content moderation and see if technology is a better way to process digital content compared to humans.
Automated Content Moderation: How Can AI Help?
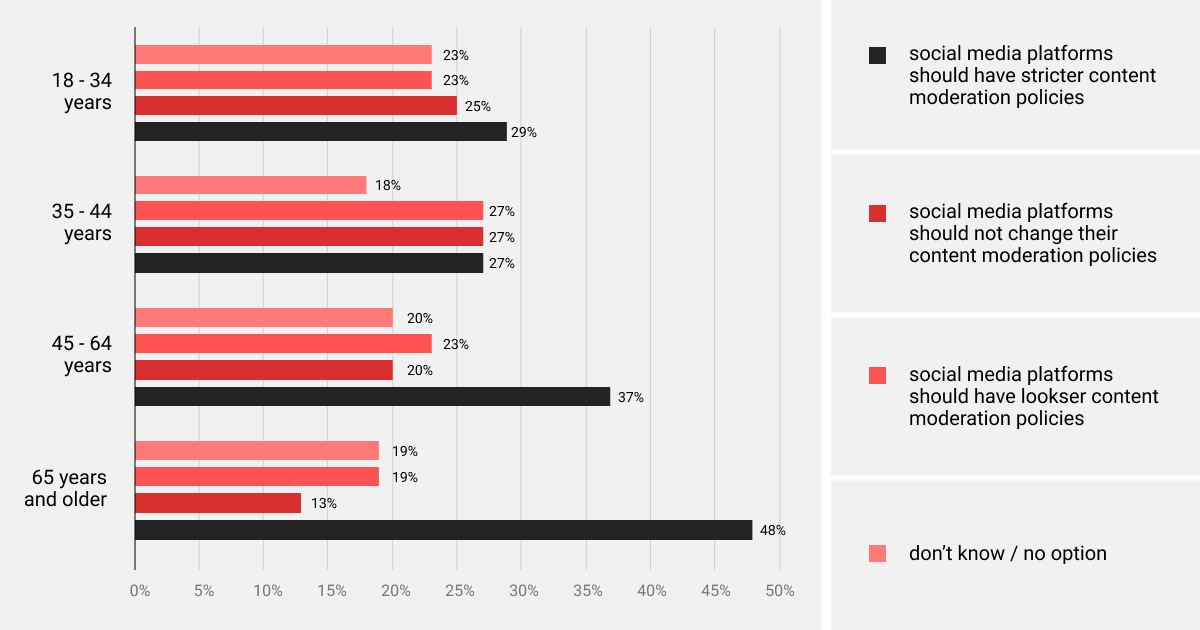
According to Gartner, 30% of big companies will recognize content moderation services for user-generated content as a significant matter for their executive leadership teams by 2024. With only a year to spare, can companies expand their moderation capabilities and policies? If organizations invest in content moderation tools to automate and scale up the process, it is highly likely to be achieved.
For instance, Facebook and YouTube use AI-powered content moderation to block graphic violence and sexually explicit/pornographic content. As a result, they managed to improve their reputation and expand their audience. However, as the problematic content grows both in volume and severity, international organizations and states are concerned about the impact of such content on users and moderators.
Among the main concerns are the lack of standardization, subjective decisions, poor working conditions of human moderators, and the psychological effects of constant exposure to harmful content. In response to these critical issues that traditional content moderation has raised, automated practices are in active use to make social media safe and responsible. It can be something simple, like keyword filters, or a more complex process that relies on AI-based algorithms and tools.
Organizations often follow one of the main content moderation methods:
- Pre-moderation. Content moderation is performed before it is published on social media.
- Post-moderation. Content is screened and reviewed after being published.
- Reactive moderation. This method relies on users in detecting inappropriate content.
- Distributed moderation. The decision to remove content is distributed among online community members.
- Automated (algorithmic) moderation. This method relies on artificial intelligence.
However, most platforms today use automated content moderation. An essential feature that satisfies the requirements for transparency and efficacy of content moderation is the ability of AI-powered systems to offer specific analytics on content that has been “actioned.” Simply said, AI offers a much more desirable solution to many issues that emerged as a result of poor content moderation and inefficient human labor.
Some of the most common practical applications of algorithmic content moderation include copyright, terrorism, toxic speech, and political issues (transparency, justice, depoliticization). As such, the role of AI in content moderation encompasses the ability to swiftly remove a wide range of harmful and noxious content, thereby safeguarding the mental well-being of users and human moderators alike.
AI Content Moderation Tools & Methods
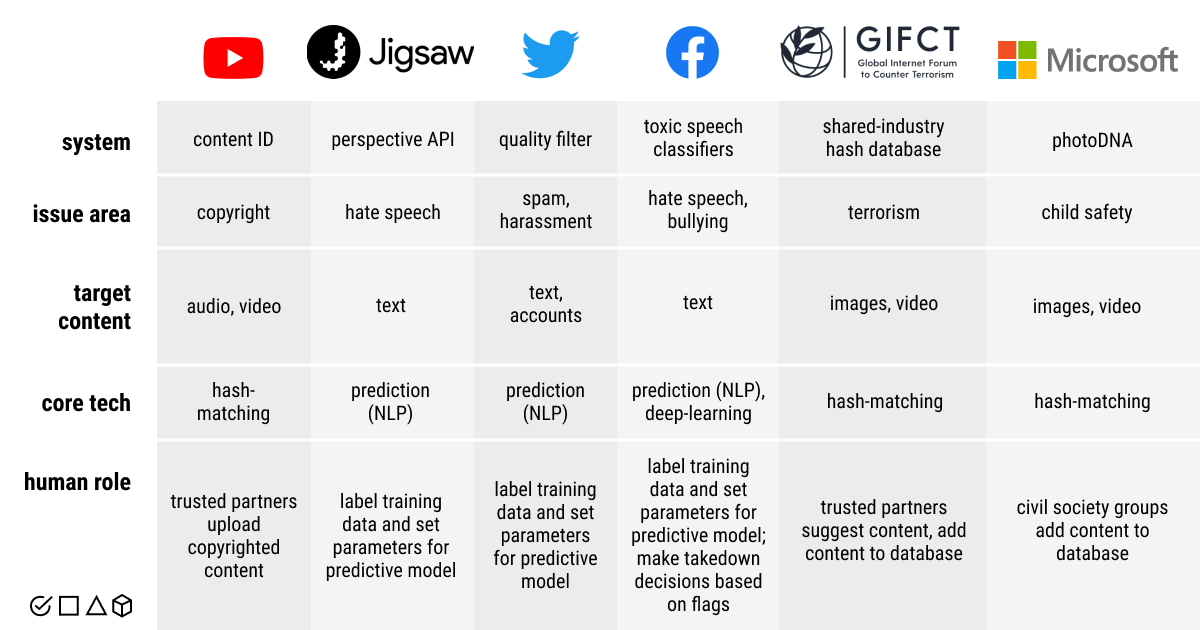
As AI in media continues to evolve and become more sophisticated, it has the potential to improve content moderation efforts and enhance user experience. At its core, AI for content moderation is when machine learning algorithms are used to detect inappropriate content and take over the tedious human work of scrolling through hundreds and thousands of posts every day. However, machines can miss some important nuances, like misinformation, bias, or hate speech.
When machine learning is in play, they require large-scale processing of user data to create new tools. However, the implementation of a content moderation tool by an online platform must be transparent to its users in terms of speech, privacy, and access to information. These tools are often trained on labeled data, such as web pages, social media postings, examples of speech in different languages and from different communities, etc.
Annotated data helps these tools to decipher the communication of various groups and detect abusive content. However, like any other technology, automated tools used for content moderation must be designed and used in accordance with international human rights law. This also largely depends on the type of data being moderated.
Text Moderation
Online content is usually associated with text and human language, since the volume of text information exceeds that of images or videos. Hence, Natural Language Processing (NLP) methods are used for moderating textual content in a way similar to humans. For instance, tools trained in AI emotion recognition can predict the emotional tone of the text message (i.e., sentiment analysis) or classify it (e.g., a hate speech classifier). The training data is usually devoid of features like usernames or URLs, but it was not until recently that emojis have been included in sentiment analysis.
An excellent example of using AI for content moderation is Google/Jigsaw’s Perspective API. Other NLP models, like OpenAI’s ChatGPT, can be used for text moderation by analyzing language patterns to identify and flag inappropriate content. Yet, the use of AI in online content moderation requires careful attention to ethical considerations and limitations associated with such novel approaches.
Image Moderation
The increased use of AI image recognition for automated image detection and identification requires a bit more sophisticated approach. There are so-called “hash values,” distinct numerical values that are produced as a result of running an ML algorithm in a file. The hash function creates a unique digital fingerprint for a file by computing the numerical value depending on its attributes.
The same hash function may be used to check if freshly uploaded content matches the hash value of previously recognized content. You can check Microsoft’s PhotoDNA tool to get the full picture. Among other tools for image moderation, computer vision in machine learning is used to identify certain objects or characteristics in an image, such as nudity, weaponry, or logos. Besides, OCR (optical character recognition) tools can be useful for detecting and analyzing text in the images and make it machine-readable.
Video Moderation
Image generation methods are also gaining traction in AI for content moderation. For instance, Generative Adversarial Networks (GANs) are used to identify a manipulated image or video. Most importantly, these ML models help detect deepfakes, videos that represent fictional characters, actions, and claims.
Deepfake technology is quite a controversial issue prevalent in the online space and television, too. It might provoke disinformation and threaten privacy and dignity rights. So, having AI handle the problem is an important step towards responsible social media and automated content moderation.
Real-World Applications of AI Content Moderation: Insights from Industry Leaders
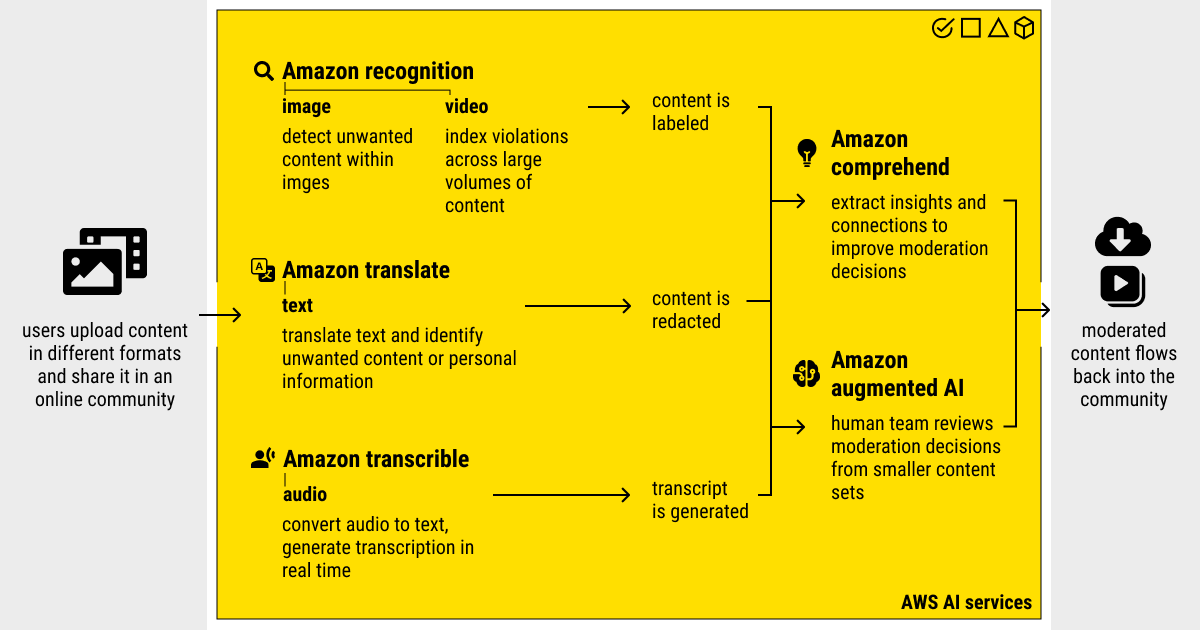
Alternative social media sites and their larger competitors moderate user-generated content, despite their emphasis on freedom of speech, with almost all sites removing offensive, violent, racist or misinformation posts or accounts that share them.
Many of these platforms rely on AI to help ensure safe content is available to users:
Case 1: Amazon
Human-led content moderation is insufficient for the expanding content scope and user protection. Online platforms require novel strategies to tackle the task, otherwise, they risk damaging their reputation, harming online community, and ultimately disengaging their users. AI-based content moderation with AWS (Amazon Web Services) provides a scalable solution to current moderation issues, using both machine learning and deep learning techniques.
Amazon Rekognition is the tool designed to maintain users’ safety and engagement, cut operational costs, and increase accuracy. It can identify inappropriate or offensive content (e.g., explicit nudity, suggestiveness, violence, etc.) at an 80% accuracy rate and remove it from the platform. It’s a great way to automate the moderation of millions of images and videos and speed up the process using content moderation APIs, with no need for ML expertise.
Case 2: Facebook
Facebook, one of the most popular social media networks in use today, has been under scrutiny for its content moderation issues, such as the Christchurch Attacks and the Cambridge Analytica lawsuit. The first case affected thousands of Facebook users, allowing a terrorist to livestream the massacre. Facebook handled the incident both manually and computationally, and now each video would be hashed, compared to the database, and finally blocked if a match was found.
But the lesson was learned, and soon Facebook started using AI for proactive content moderation. Zuckerberg claims that its AI system discovers 90% of flagged content and that the remaining 10% is uncovered by human moderators. AI-based content moderation at Facebook detects and flags potentially problematic content using their in-house systems, such as Deep Text, FastTex, XLM-R (RoBERTa), and RIO. Today, however, Facebook relies on Accenture in moderating its content by building a scalable infrastructure to prevent harmful content from appearing on its site.
Case 3: YouTube
In just one quarter of 2022, a whopping 5.6 million videos were taken down from YouTube, including ones flagged by the platform’s automated tools for breaking community guidelines. The use of AI in online content moderation led to higher rates of upheld removal appeals (nearly 50%) compared to human moderation (less than 25%), but this also resulted in lower accuracy of removals.
More specifically, almost 98% of the videos on YouTube that were removed for violent extremism were flagged by ML algorithms. One of its noteworthy algorithmic moderating systems is known as Content ID, which is used in the copyright domain (hash-matching audio and video content). The platform has also managed to regulate toxic speech in the uploaded content by training ML classifiers. They are trained to predict hate, harassment, as well as swearing, and inappropriate language in a video.
Case 4: Twitter
Twitter has been long criticized for not being able to respond efficiently to harassment online. Therefore, this platform has been working on its internal AI-powered tool for content moderation, and eventually came up with Quality Filter. This filter can predict low-quality, spammy, or automated content on the platform using NLP, labeled training data, and established parameters for predictive ML models.
However, Quality Filter was designed to not remove potentially inappropriate content, but to make it less visible to users, given the First Amendment stance on freedom of expression. So, the paradigm shift towards automation in content moderation practices made us rely more on AI in deciding what content we should trust or expose ourselves to.
Final Thoughts on AI in Content Moderation: Friend or Foe?
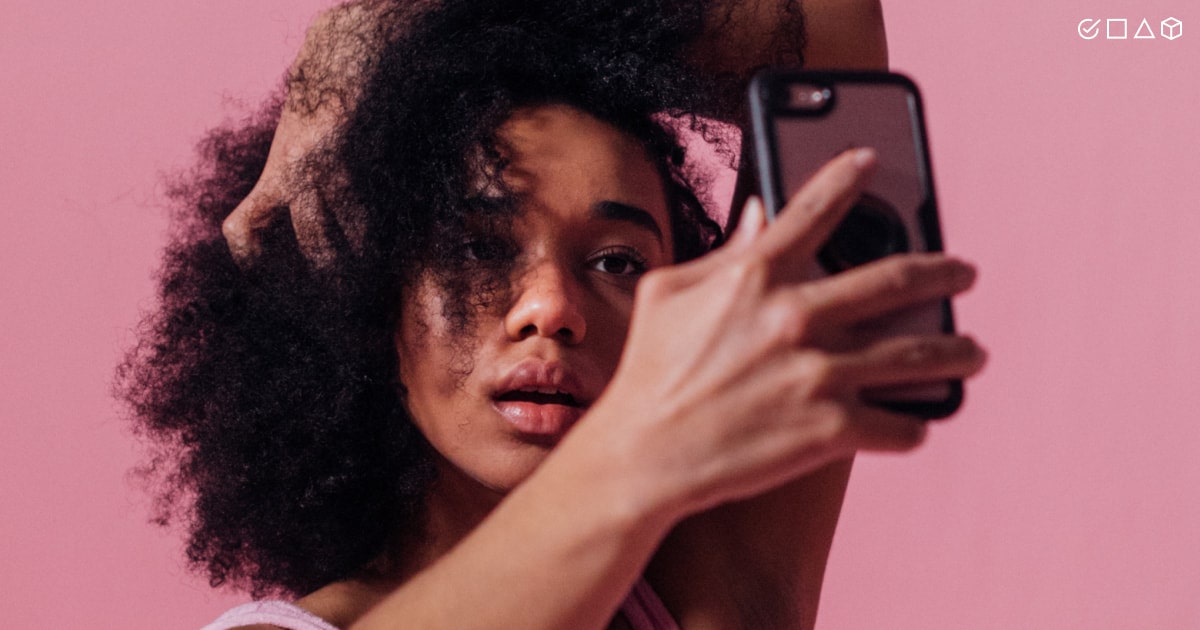
Smart content moderation using AI matters to everyone. On the one hand, AI is a powerful tool for filtering and removing harmful content from the internet, but on the other hand, it is not without its drawbacks. ML algorithms can be biased, imperfect, and prone to making mistakes. However, with the right approach and fine-tuning, AI can be an invaluable tool for moderating content online.
The success of AI content moderation will ultimately depend on how well it is implemented and how effectively it balances the competing interests of free speech and harmful content. Yet, it’s best to have human oversight in making the final decision on banning users or deleting content. The future of AI content moderation is bright, but we must approach it with caution and ensure that we do not sacrifice our fundamental values in the pursuit of a quick fix.
FAQ
-
How does AI-based content moderation work?
Content moderation practices based on artificial intelligence use machine learning algorithms to monitor and regulate user-generated content on online platforms.
-
How AI is useful in content moderation?
Automated moderation stage powered by AI can improve the efficiency of content moderation by detecting harmfulness or uncertainty and prioritising content for review by human moderators.
-
What are the key benefits of using AI in content marketing?
Overall, using AI in content marketing can enhance audience engagement, optimize campaigns, and improve ROI.
Table of Contents
Get Notified ⤵
Receive weekly email each time we publish something new: