Keypoint Annotation: Essential Tips for ML Teams
Table of Contents
- TL;DR
- How Keypoint Annotation Enhances ML Models
- Applications of Keypoint Annotation in Machine Learning
- The Keypoint Annotation Process: How to Do It Right
- Common Challenges and Solutions
- Best Practices for Efficient Keypoint Annotation
- Choosing the Right Keypoint Annotation Tool
- Optimizing Keypoint Annotation for Better ML Performance
- About Label Your Data
- FAQ
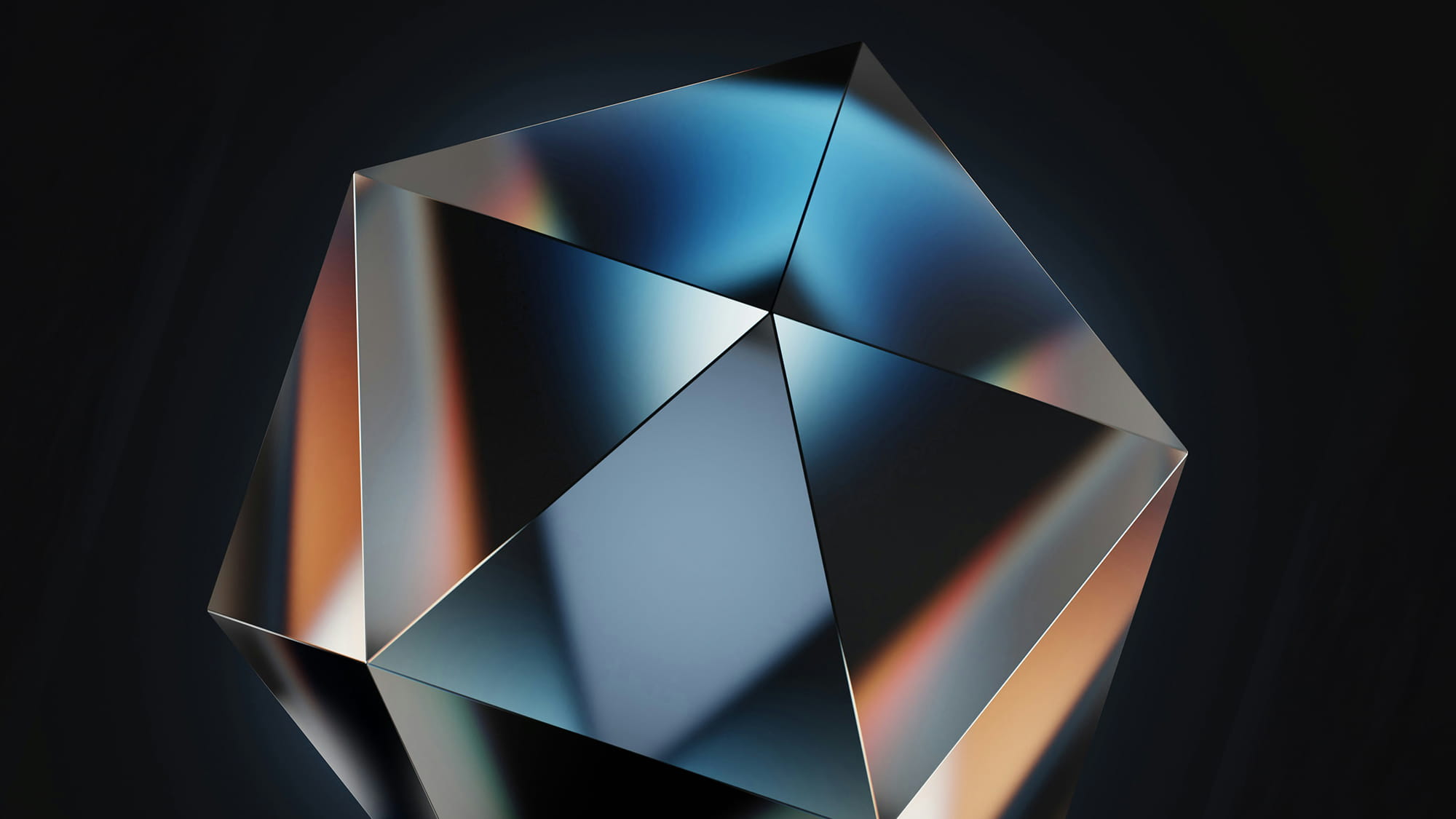
TL;DR
How Keypoint Annotation Enhances ML Models
Keypoint annotation helps train machine learning models by marking specific points in images or videos. These points, called “landmarks,” define features like eyes, joints, and object edges to capture structure and movement.
How it compares to other methods:
Keypoints: Mark precise points within an image. Best for tracking fine details.
Bounding box annotation: Outline an object’s general area, but include extra pixels. Less precise.
Polygons: Trace an object’s exact shape. Highly accurate but time-consuming and costly.
It’s as different as image classification vs. object detection. Keypoint annotation is ideal when precision matters, but full object segmentation isn’t necessary.
Keypoint annotation is a cost-effective way to get a good level of detail without breaking the bank. It’s ideal for tasks like tracking human movement and some image recognition tasks (e.g., facial recognition). It improves your machine learning algorithm by providing precise, structured data that enhances feature extraction and model performance.
Applications of Keypoint Annotation in Machine Learning
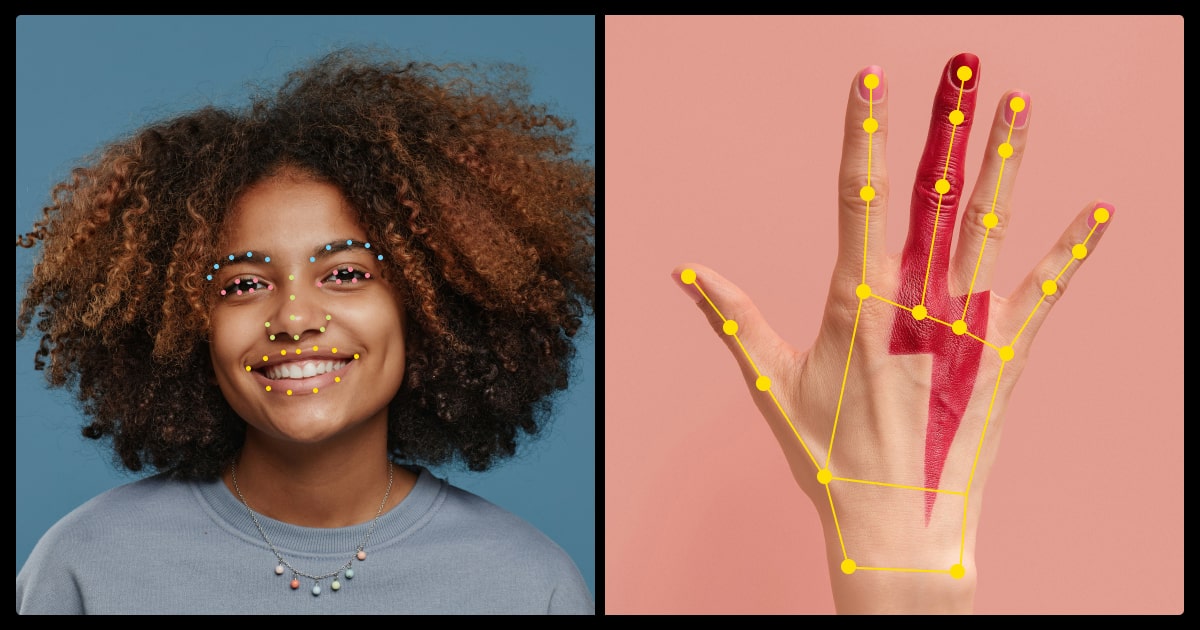
Key point annotation helps AI recognize patterns, track movement, and improve decision-making across various industries. It’s a good way to get through unlabeled data cost-effectively.
Medical Imaging
AI detects abnormalities like tumors and fractures by marking key anatomical points, providing detailed insights beyond full-image analysis.
Autonomous Driving
Keypoints track pedestrians and vehicles, improving motion detection and predicting actions like crossing the street.
Sports Analytics
AI analyzes player movement by marking body parts, helping coaches refine techniques like footwork and jump shots.
Robotics & Manufacturing
Keypoints enable gesture recognition, object tracking, and quality control, improving automation and machine interaction.
AR/VR & 3D Modeling
Defining spatial keypoints ensures realistic object interactions, enhancing immersion in virtual environments.
The Keypoint Annotation Process: How to Do It Right
So, considering how important this task is, how do you succeed?
Dataset Preparation
You get out what you put in. If you start with bad data, you can’t expect your model to perform well. Keep the dataset:
Diverse
Include variations in lighting, angles, occlusions, and backgrounds so your model is better able to generalize. Do include edge use cases that the model is likely to encounter in the real world.
Clean
Remove low-quality images. Make sure that all the images you label are clear, well-lit, and a good representation. If you can’t tell if it’s an elbow or a knee, AI won’t be able to either.
Balanced
You must guard against dataset biases. You can do this by including different object types, poses, and conditions.
Likewise, you should spend a little time pre-processing the images to improve their quality. While this extra step adds extra expense, the results are worthwhile. Look into resizing, augmenting, or normalizing images before labeling starts.
Ontology Definition
You need to clearly set out your labeling structure before you begin. That way, if you use a team of annotators, they’re all on the same page. This step ensures that your labels are consistent, which improves the accuracy of machine learning.
Define Keypoints
What landmarks do you need for your model? For example, if you’re creating facial recognition software, you’ll need 68 facial landmarks. If you’re training a model for human pose estimation, you may only need 17.
Establish Naming Convention
You can make the process more efficient by using standardized labels such as “Left Shoulder” or “Right Eye.”
Set Clear Annotation Rules
You need to set out guidelines for keypoint placement, handling occlusions, and ambiguous cases. That way, your annotators will all handle the project in the same way.
When you standardize data annotation, you give your model structured, high quality data it can learn from easily. You also reduce the risk of inconsistencies, which improves the real-world performance of your model.
Data Annotation
There are several ways to approach data labeling here. Your first choice is whether to use manual or automated annotation workflows. The option you select depends on the project’s scale, available resources, and accuracy requirements.
Manually labeling the data gives the best accuracy, but is time-consuming and labor-intensive. You can save time and resources by using tools like Roboflow keypoint annotation, but you’ll need to have humans check the results.
Quality Assurance
You’ll need to spend time reviewing the results. Here are some options:
Inter-Annotator Agreement
Check the consistency between different labels applied by different annotators to make sure everyone’s on the same page.
Spot-checking samples
Randomly review a subset of labels for accuracy and consistency.
Iterative refinement
Provide feedback loops, so your annotators can correct mistakes and improve accuracy as the project progresses.
You can also use automated validation tools to help flag missing or misplaced keypoints along the way.
Data Export
Once you finish the annotation process, you’ll need to format the data so it fits into your machine learning pipeline. You’ll need to think about:
Format: Use COCO keypoint annotation, CSV, or JSON.
Metadata: Maintain a clear structure for seamless training.
Storage: Organize data efficiently to speed up training.
Using active learning in conjunction with human-in-the-loop (HITL) validation increases keypoint annotation accuracy. This method involves predicting keypoints with machine learning models, which are then reviewed and refined by human annotators. It improves precision while saving time by focusing efforts where the model has low confidence.
Common Challenges and Solutions
Here’s what can go wrong in keypoint annotation workflows and practical solutions:
Best Practices for Efficient Keypoint Annotation
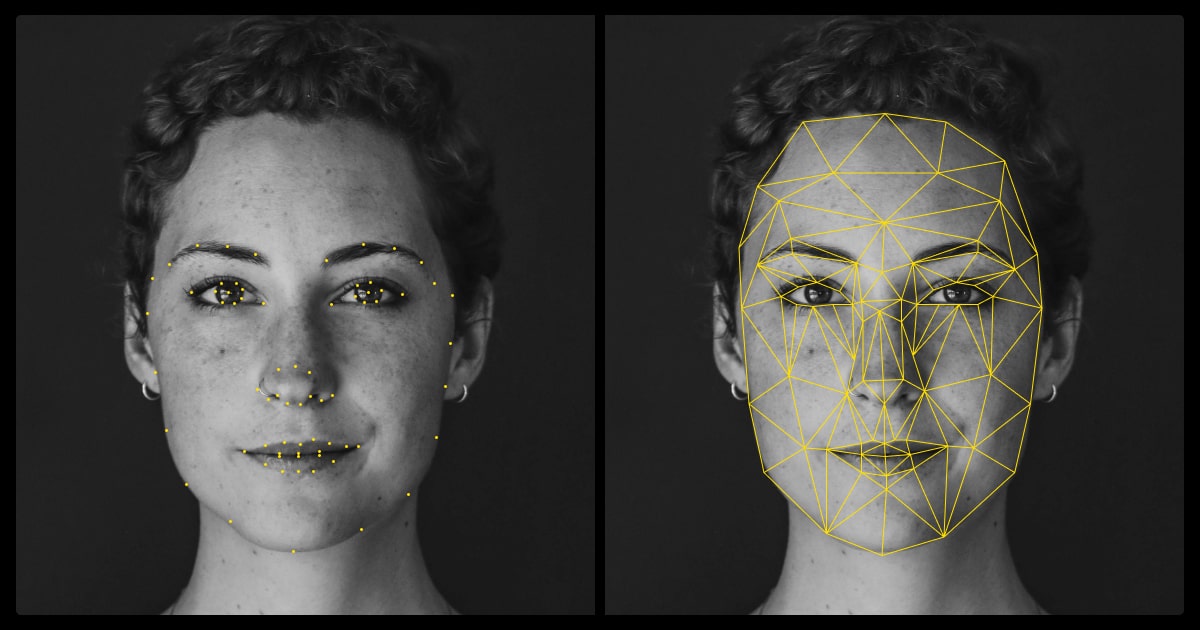
Planning is your best ally in the fight for efficiency here. Let’s go over how you can make sure your dataset is as good as it can possibly be:
Establish Detailed Guidelines
Set clear guidelines, so there’s no ambiguity in annotation.
Outsource to Trusted Vendors
Partnering with a professional data annotation company ensures accuracy and efficiency. To see how Label Your Data delivers quality results, check out the company review for insights.
Use Automation Where Appropriate
You should use pre-annotation tools to save time preparing the data.
Leverage Collaborative Tools
It makes sense to use these tools to make sure everyone’s on the same page. It also makes it easier to scale up the workload as necessary.
Ensure Ethical Data Practices
You need to fully anonymize the data you feed into your model and adhere fully to your company’s security protocols.
The adaptive consensus calibration method increased annotation accuracy from 78% to 97% in four months. By establishing a golden dataset and refining it with real-time calibration checks, errors dropped significantly. In medical imaging, for example, addressing lighting inconsistencies reduced keypoint placement errors by 40%. This method also cut rework time by 60%, making it highly effective for large-scale projects.
Choosing the Right Keypoint Annotation Tool
Some projects may require data annotation services to handle complex workflows, while others benefit from scalable, automated tools. Below, we’ll go over the key features to look for and the top keypoint annotation tools available.
Key Features to Prioritize
So, what features should you look for in a data annotation tool? You’ll need to be guided by what your project needs, but these are pretty good for a start:
Scalability
Automation
Collaboration features
Support for 3D data
Overview of Top Tools
Let’s look at the top tools on the market today.
Label Your Data
A data annotation platform that allows teams to upload datasets, choose annotation types, and download labeled data with no minimum commitment. With built-in instruction templates, team collaboration, and API access, it streamlines the annotation process for businesses of any size.
V7
V7 supports auto-labeling, collaborative workflows, and model-assisted annotation for images and videos. It’s ideal for OCR deep learning projects that require high accuracy, such as:
Healthcare
Autonomous driving
Robotics
Labelbox
Labelbox is cloud-based and supports image, video, and text labeling. It offers automation, AI-assisted labeling and project management. It’s the best option if you’re a large company working with big datasets.
CVAT (Computer Vision Annotation Tool)
Intel’s open-source tool supports image and video annotation. It has extra features like interpolation and object tracking, which make it useful for refining the quality of datasets. It also offers multiple annotation formats and is popular with many research institutions globally.
Roboflow
Roboflow is also cloud-based. It offers image annotation, dataset management and model training. It makes it easy to preprocess and augment your data where necessary. Therefore, if you have a limited dataset, it can help you stretch it out. It’s ideal for developers working on computer vision projects.
If you’re on a limited budget, free tools are an option, but data annotation pricing varies based on accuracy and project scale.
Optimizing Keypoint Annotation for Better ML Performance
High-quality annotations directly impact model accuracy. Poorly labeled data leads to incorrect keypoint placement and false positives.
How High-Quality Data Improves Accuracy
Precise localization: Helps models learn exact object or body part positions.
Better generalization: Enables adaptation to different lighting, poses, and occlusions.
Improved feature learning: Strengthens spatial understanding for accurate predictions.
Less noise and bias: Prevents models from learning incorrect patterns.
The best way to improve keypoint accuracy is using adaptive zoom levels. Manually adjusting zoom based on detail complexity ensures precise keypoint placement. In electrical schematics, this method reduced errors and helped teams follow annotated plans without misalignment, improving efficiency and reducing rework.
Evaluating Keypoint Detection
Two key metrics assess performance:
About Label Your Data
If you choose to delegate data annotation, run a free data pilot with Label Your Data. Our outsourcing strategy has helped many companies scale their ML projects. Here’s why:
No Commitment
Check our performance based on a free trial
Flexible Pricing
Pay per labeled object or per annotation hour
Tool-Agnostic
Working with every annotation tool, even your custom tools
Data Compliance
Work with a data-certified vendor: PCI DSS Level 1, ISO:2700, GDPR, CCPA
FAQ
What is key point annotation?
Keypoint annotation is a labeling technique where you highlight certain features instead of a whole object. This precludes the extra pixels that are problematic with bounding boxes. It’s also much less labor-intensive than using polygonal techniques.
What are key points in data annotation?
Key points in data annotation are specific coordinates marked on objects within images or videos. These points highlight important features like facial landmarks, joints, or object edges. They help AI models track movement and recognize patterns for tasks like pose estimation, facial recognition, and object detection and tracking.
What is an example of a keypoint?
An example of a keypoint is marking the corners of a person's eyes in facial recognition. In pose estimation, keypoints can track joints like elbows and knees to analyze movement.
How does keypoint annotation affect model training time?
Keypoint annotation can speed up training by reducing unnecessary data, but high-density keypoints may increase processing time. Efficient labeling and dataset management help balance speed and accuracy.
What are the challenges of scaling keypoint annotation?
Scaling introduces inconsistencies, making quality control harder. Clear guidelines, inter-annotator checks, and automation help maintain accuracy.
Written by
Karyna is the CEO of Label Your Data, a company specializing in data labeling solutions for machine learning projects. With a strong background in machine learning, she frequently collaborates with editors to share her expertise through articles, whitepapers, and presentations.