LLM Use Cases: For Data Science and ML Workflows
Table of Contents
- TL;DR
- How LLMs Are Transforming Activities Across Industries
- LLM Use Cases in Finance: Reducing Risk and Ensuring Compliance
- Improving Patient Outcomes with LLM Use Cases in Healthcare
- LLM Use Cases in Retail: Optimizing Inventory
- Increasing Efficiency with LLM Use Cases in Manufacturing
- LLM Use Cases in Banking: Revolutionizing Service and Credit Scoring
- E-commerce: Enhancing Product Search and Descriptions
- About Label Your Data
- FAQ
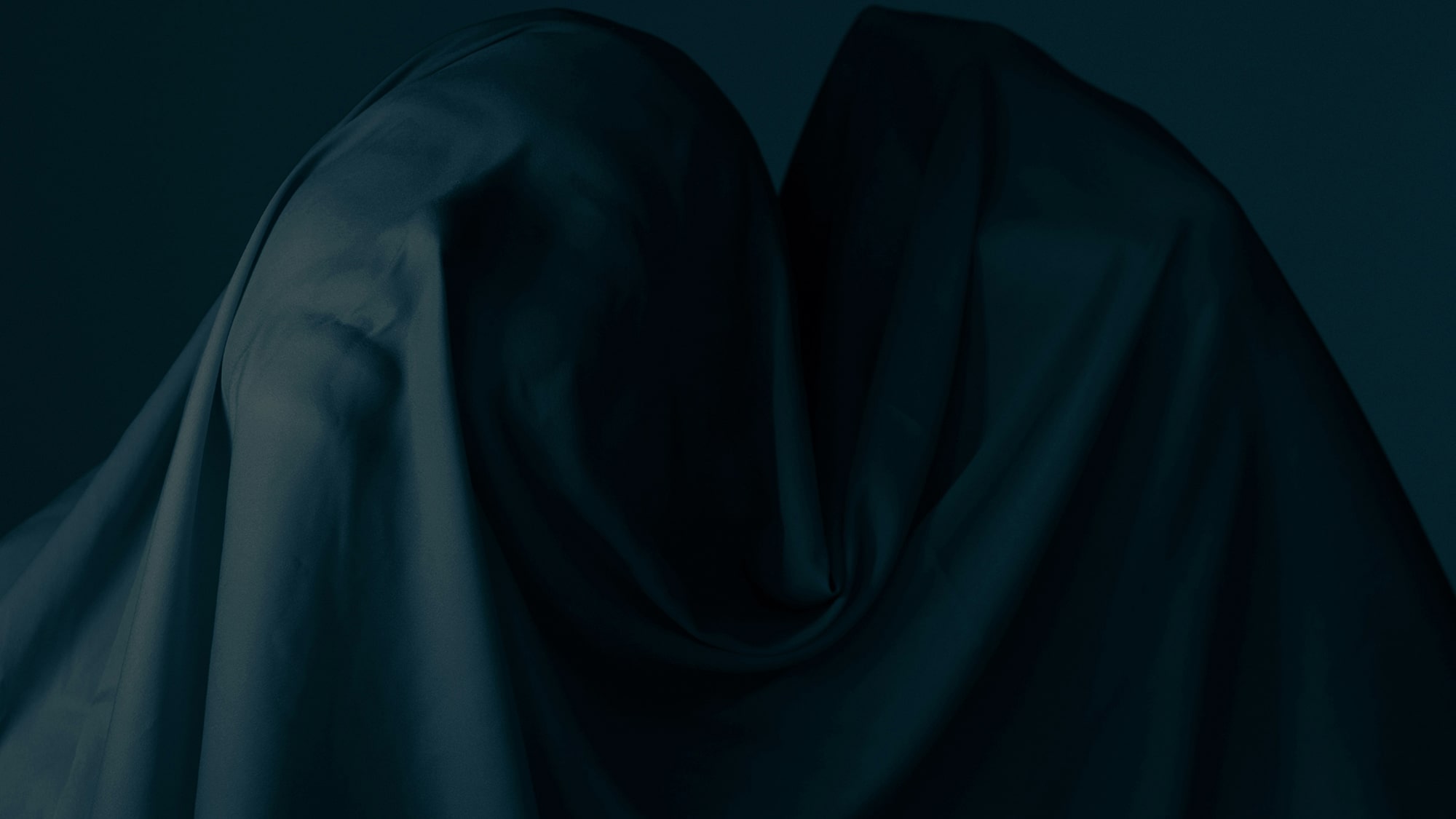
TL;DR
How LLMs Are Transforming Activities Across Industries
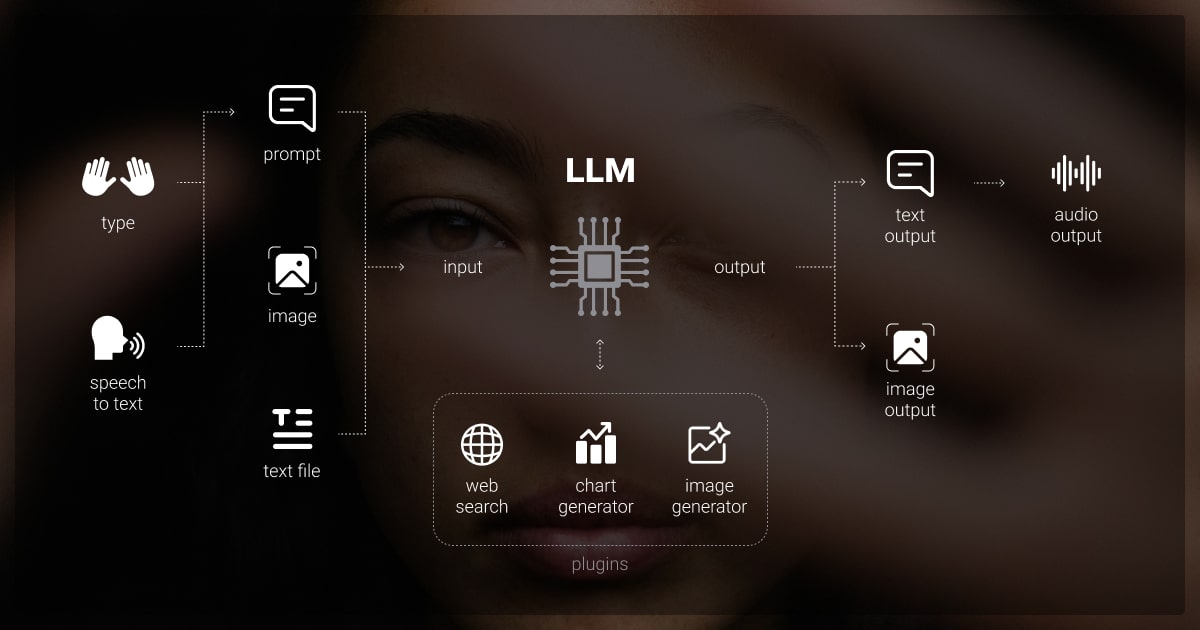
Large language models (LLMs) are transforming data science and machine learning (ML) workflows across industries. This is due to different LLM architectures that allow automating and enhancing complex ML processes.
As LLMs are implemented across various sectors, they serve as a link between unstructured and structured data. They drive insights, automate ML workflows, and improve user experiences.
Integrating LLMs into traditional ML workflows can significantly enhance data science operations by streamlining processes like data preprocessing. LLMs excel in transforming unstructured text data into structured formats, making it easier for ML models to work with. Additionally, they automatically generate relevant features from textual data, improving downstream model performance.
With the ability to analyze and interpret texts at such an extensive scale, LLMs bring efficiency to the next level, while opening new possibilities. Here’s how they are reshaping essential ML activities that cut across industries:
Data Analysis and Insights Generation
In sectors from healthcare to finance, LLM use cases process enormous amounts of raw data. They assist with uncovering trends, correlations, and elaborative insights. The example in healthcare can be the analysis of a patient's record or data received from clinical trials. By working with research papers, they can generate summaries and identify patterns that support doctors in diagnostic and treatment decisions.
LLMs bring a deeper understanding of language, enabling applications that integrate text and numerical data. By working with ML models, LLMs reduce errors and ensure results are grounded in actual data, making the system more reliable and cost-effective.
Customer Interaction and Support
Customer service has become more efficient. It provides faster responses with LLM-driven chatbots and virtual assistants. Use cases of LLM engage with customers, executing various tasks. Some examples include sentiment analysis, intent recognition, and question answering.
According to McKinsey, with the application of generative AI, the issue resolution can increase by 14% an hour, while the time spent on handling the case reduces by 9%.
Content Creation and Personalization
LLMs allow industries like media, retail, and education to generate content dynamically. With LLMs, you can create unique product descriptions, knowledge-based materials, and carve personalized marketing campaigns. LLMs add efficiency to content-heavy workflows. By tailoring a machine learning algorithm, brands can engage users on a personal level, building loyalty and improving engagement rates.
Operational Automation
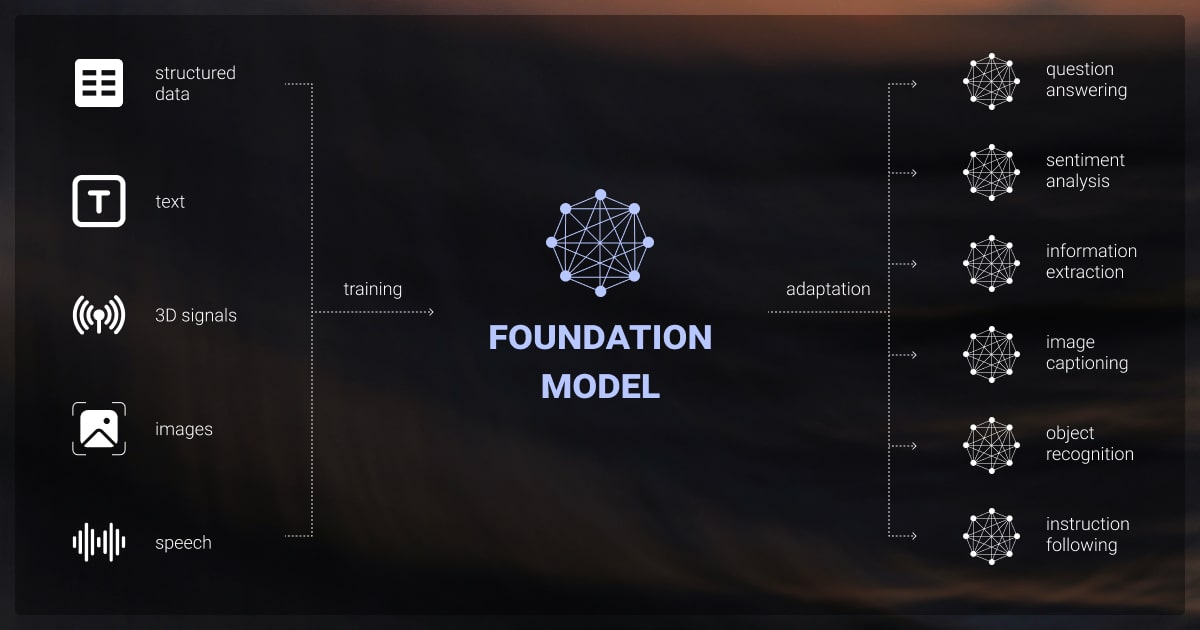
Repetitive tasks, especially document handling and data entry, have historically required extensive manual effort. Now, LLMs complete these tasks swiftly and accurately, freeing human teams to focus on higher-level functions. For example, legal firms are using LLMs to review and summarize contracts, flag critical clauses, and identify discrepancies. Similarly, in finance, LLMs help streamline processes like transaction reconciliation and account management.
LLMs automate data preparation, boost feature engineering, and strengthen interpretability through natural-language explanations. Their ability to handle unstructured text enhances decision-making and productivity in data science projects.
Predictive Modeling and Support in Decision-Making
LLMs accomplish predictive and forecasting tasks in various industries, including finance and healthcare. Analyses based on LLM predictability significantly improve overall planning and strategy. With elaborated LLM fine-tuning, large language models create forecasts and guide decision-making.
With their transformative potential across these core activities, LLMs add value reshaping entire industries. Let’s dive deeper into specific applications across key sectors.
LLM Use Cases in Finance: Reducing Risk and Ensuring Compliance
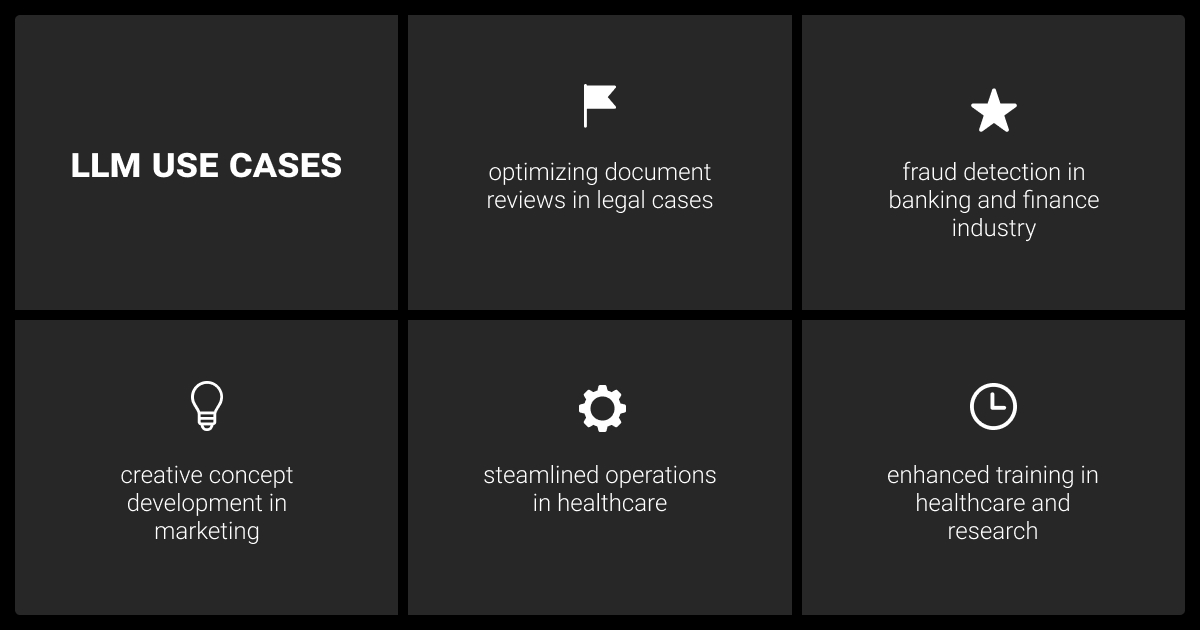
The financial sector processes financial data and clients' sensitive data. This is the area where compliance and regulatory measures are top priorities. That's why, before implementing any LLMs for specific tasks, financial datasets pass through the steps of data annotation in fintech and further ML training. With augmented accuracy, LLMs can offer a technological advantage, streamlining complex workflows.
Fraud Detection
Financial fraud is often hidden within vast data networks, and LLMs excel at identifying irregular patterns. By analyzing massive volumes of transactional data in real-time, LLM use cases in banking flag potentially fraudulent activities. For example, a model can detect that an account uses unusual location for sign in. It can also detect signs of anomalous spending, alerting fraud teams immediately. This helps mitigate risks and prevent substantial financial losses.
Compliance Monitoring
Financial regulations vary globally and constantly evolve, requiring institutions to stay vigilant. With LLMs, you receive significant help scanning compliance data. They monitor any violations or generate final reports for audit purposes. This level of automation minimizes human error, ensures that companies adhere to legal standards, and reduces the risk of regulatory fines.
Customer Sentiment Analysis
Banks benefit from analyzing customer sentiment to refine their service offerings. LLMs comb through social media, reviews, and customer feedback. They gauge sentiment, providing banks with important information on customer's preferences or needs. By understanding client needs more clearly, banks can adjust their products. With right communication strategies they can build stronger relationships.
Improving Patient Outcomes with LLM Use Cases in Healthcare
Healthcare uses insights from data to improve patient care. LLMs perfectly take this task on them, providing quicker and more accurate insights. From clinical data analysis to supporting drug discovery, LLM models drive value in multiple ways.
Clinical Data Analysis
In healthcare, doctors process vast volumes of patient data. These include electronic health records (EHRs), lab results, and diagnostic notes, among others. With LLMs, this activity becomes straightforward and quicker. It helps identify trends and correlations that may go unnoticed in a traditional analysis. For example, LLMs can scan through EHRs to highlight patients with similar symptoms. This can offer doctors a basis for diagnosing rare diseases and personalizing treatment plans.
Medical Imaging Interpretation
One of the vital areas in radiology is interpreting images like MRIs and CTs. The process takes much time before doctors can come to conclusions or diagnoses. LLMs assist by analyzing these images, producing reports that outline potential abnormalities and recommendations for further action. The models don't do prescriptions, but help to notice potential areas of concern. With accurate analyses, diagnostic accuracy increases as well.
Drug Discovery and Research Summarization
With AI in drug discovery, LLMs play a significant role in accelerating drug discovery. They analyze a vast body of medical research to identify promising compounds or treatment options. With LLMs, the process of drug discovery becomes faster. Models help to summarize findings in research papers and clinical trials in less time. This capability is crucial, especially when rapid development is needed, such as in response to a health crisis.
LLM Use Cases in Retail: Optimizing Inventory
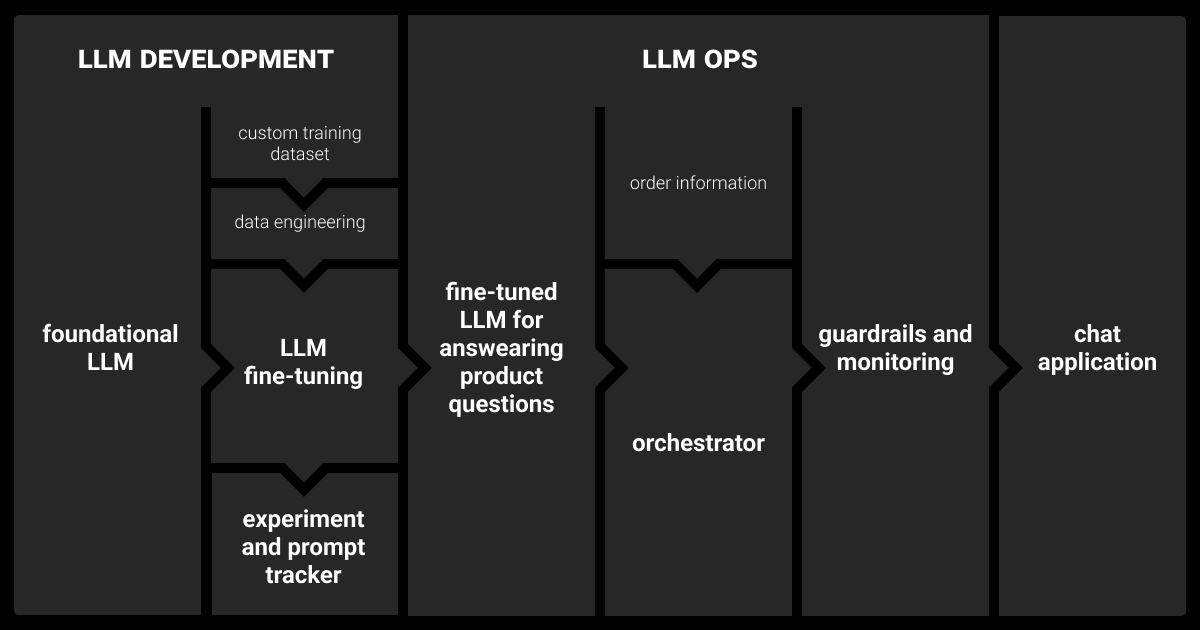
LLM business use cases in retail are not only about timely service. They transform customer experience and provide insights into customer behavior.
Customer Sentiment Analysis
LLMs come into play by analyzing customer feedback, product surveys, and decoding consumer reviews. With more accurate customer sentiment analysis, we can adjust product offerings, marketing strategies, and the overall shopping experience. This capability allows brands to engage more deeply with their customer base, building loyalty and driving long-term growth.
Product Recommendations
Personalized product recommendations wouldn't be as widespread as they are today without LLMs. Machine learning algorithms analyze browsing pattern. They provide valuable insights into demographics, purchase history, or product preferences. This increases conversion rates and customer satisfaction, as each interaction feels relevant and thoughtfully curated.
Demand Forecasting
Effective inventory management is vital for success in the retail industry. By examining historical sales data and factoring in external influences like seasonal demand, LLMs enable precise demand forecasting. With these insights, retailers can adjust stock levels proactively. As a result, this reduces the expenses tied to surplus inventory or stock shortages. This approach helps ensure that high-demand products are readily available when customers are most likely to purchase them.
Increasing Efficiency with LLM Use Cases in Manufacturing
Operational efficiency is essential in manufacturing. LLMs support this by enabling manufacturers to foresee potential issues. They streamline supply chain processes and uphold quality standards. These models empower manufacturers to enhance productivity while proactively addressing challenges across operations.
Predictive Maintenance
LLMs minimize costly downtime by analyzing equipment sensor data. This helps to detect early malfunction signs. Models alert maintenance teams ahead of breakdowns, preventing disruptions or repairs. Predictive maintenance through LLMs cuts costs and enhances safety on the factory floor.
Supply Chain Optimization
With a resilient supply chain, LLMs provide visibility into every chain link. They analyze logistics, supplier performance, and market demand. With LLMs' insights, manufacturers respond to disruptions ahead of time. If a supply delay occurs, the model might recommend alternative suppliers or transport routes. This keeps production on schedule and meets customer demands efficiently.
Quality Control and Defect Detection
LLMs also support quality control by identifying defect patterns in production data. They reduce the risk of faulty products reaching customers. In sectors like automotive or electronics, these insights improve product reliability. As quality is a must, they reduce warranty costs and protect brand reputation.
LLM Use Cases in Banking: Revolutionizing Service and Credit Scoring
For banks, delivering personalized service and managing risk are top priorities. LLMs enhance both areas, making customer interactions more efficient and credit assessments more precise.
Automated Customer Service
Banks deal with high volumes of customer inquiries, often repetitive in nature. LLM-powered chatbots handle routine questions. They manage balance checks to transaction histories. They also provide customers with 24/7 support. This saves time for bank representatives, allowing them to focus on complex issues. At the same time, customers enjoy quick and accessible service.
Credit Scoring and Loan Assessment
Traditional credit scoring methods often exclude individuals lacking a long credit history. LLMs change this by analyzing diverse data points like transaction history and spending habits, creating an accurate risk profile for each applicant. This allows banks to assess creditworthiness more inclusively and streamline loan approvals.
E-commerce: Enhancing Product Search and Descriptions
E-commerce platforms rely on LLMs to personalize shopping experiences. They help improve how customers navigate extensive product catalogs.
Product Descriptions
Manually writing unique descriptions for thousands of products is labor-intensive. LLMs generate descriptions from product attributes. They ensure consistency and optimize for search engine rankings. This automation boosts visibility and brings more potential customers to the site. And the teams benefit from less workload.
Search Relevance
When customers use complex search queries, LLMs help by refining search algorithms. For example, if a user searches for “comfortable office chairs,” LLMs understand the context and present options that meet the criteria.
As LLM technology advances, its applications will undoubtedly expand. It will offer even more tools to industries that embrace its potential. In a data-centric world, LLMs are indispensable assets. They redefine what’s possible in the business landscape.
About Label Your Data
If you choose to delegate data annotation, run a free data pilot with Label Your Data. Our outsourcing strategy has helped many companies scale their ML projects. Here’s why:
No Commitment
Check our performance based on a free trial
Flexible Pricing
Pay per labeled object or per annotation hour
Tool-Agnostic
Working with every annotation tool, even your custom tools
Data Compliance
Work with a data-certified vendor: PCI DSS Level 1, ISO:2700, GDPR, CCPA
FAQ
What are the use cases of LLMs?
LLMs are used in diverse fields like customer support, content generation, and data analysis. Companies like Label Your Data enhance these models by fine-tuning them for industry-specific tasks, improving accuracy in tasks like sentiment analysis, predictive maintenance, and automated reporting.
What are the practical use of LLM?
Practically, LLMs automate and improve workflows in sectors such as finance, healthcare, and retail by powering chatbots, summarizing complex information, and generating insights from large datasets.
What is the use case of LLM agent?
An LLM agent autonomously handles tasks by understanding context and interacting with users. It’s practical in customer service, data querying, and personal assistance, where you can fine-tune these agents to perform reliably in specific industries.
How can LLM help in daily life?
LLMs assist daily by powering virtual assistants, enhancing content recommendations, and automating customer service responses, making technology interactions faster and more intuitive.
Written by
Karyna is the CEO of Label Your Data, a company specializing in data labeling solutions for machine learning projects. With a strong background in machine learning, she frequently collaborates with editors to share her expertise through articles, whitepapers, and presentations.