Advanced Applications of Computer Vision in Manufacturing Processes
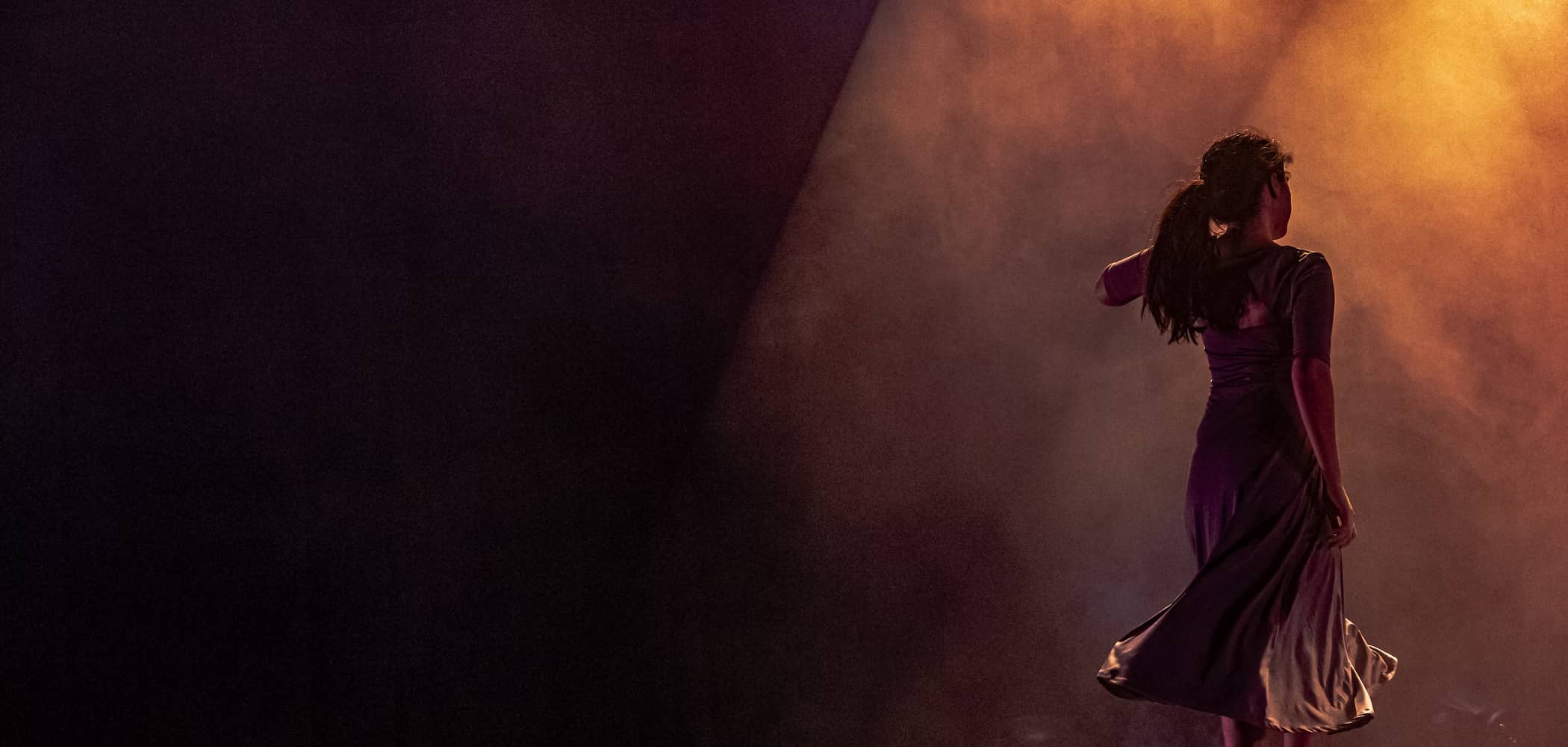
As we keep exploring the limitless possibilities of artificial intelligence solutions for major industries, AI never ceases to amaze. Here’s a fresh case to spark your curiosity:
3B-Fibreglass, a leading glass-fiber product developer, found itself grappling with a persistent issue: fiber breakages during production, causing costly delays. The solution? They implemented a camera-based monitoring system coupled with deep-learning computer vision to detect and predict fiber breaks, enabling swift operator response. By employing computer vision technology and DL algorithms, the system excelled in identifying and forecasting breaks 75 seconds in advance.
Looking at this case, as well as at a number of research studies, we can safely say that computer vision services have dominated the manufacturing field. The 3B-Fibreglass case is just one captivating example of how computer vision is revolutionizing manufacturing. In this article, we’ll explore key applications and examples of computer vision in manufacturing, shedding light on its transformative potential across the industry.
Several Important Techniques of Computer Vision in Manufacturing Industry
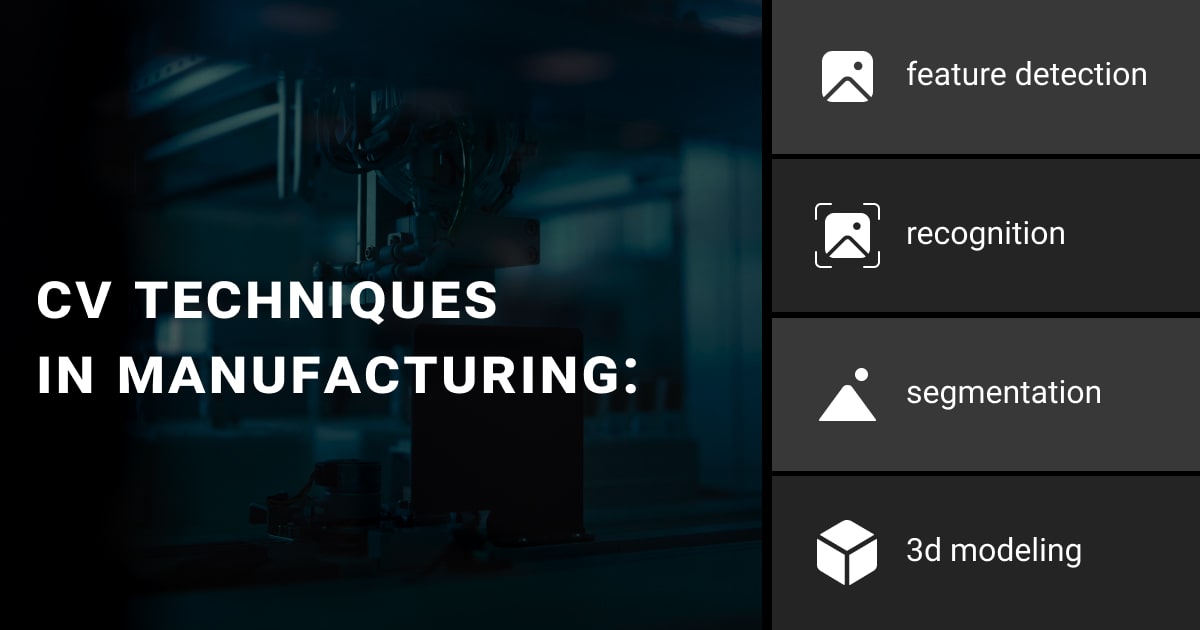
Earlier, we delved into the applications of computer vision in agriculture, illustrating how this technology elevates automation within the field. In manufacturing, too, efficiency and precision are paramount. To achieve these critical goals of AI-powered manufacturing, the industry has also embraced some key techniques of computer vision in manufacturing:
Feature Detection
Feature detection identifies visual elements like points, edges, and lines in images, crucial for various tasks of computer vision in the manufacturing industry. Two main types of computer vision features exist: keypoints (specific regions of interest like human eyes or license plates) and edge features (for detecting object boundaries). Keypoints are found using local or global search, suitable for quick or dynamic scenes. Edge detection locates abrupt color/brightness changes, often indicating object edges, using gradient-based methods with noise reduction filters.
Recognition
Recognition encompasses three main tasks: instance recognition (identifying specific known objects through feature matching and machine learning); class recognition (recognizing objects belonging to specific categories, resembling image classification); and general category recognition (the most complex task, requiring the identification of object locations and categories, often leveraging deep learning methods). Action recognition in videos remains a challenging task in CV, with applications in safety assurance in manufacturing, where the accurate identification of human actions is essential.
Segmentation
In image segmentation, the goal is to categorize pixels into different groups based on the objects they belong to. Think of it as sorting pixels into clusters. Modern segmentation techniques rely on algorithms that consider factors like how consistent pixels are within a region and how different they are between regions. Some methods included mean shift, graph-based merging, graph cut-based Markov models, and level sets. Yet, the effectiveness of these algorithms depends not only on their design, but also on the quality of the training data.
3D Modeling
3D modeling deals with two main challenges: stereo correspondence and 3D reconstruction. The first challenge is about creating a 3D model of an object/scene through the use of two or more images of it. In contrast, 3D reconstruction aims to build a 3D model from just one image. Evaluating the accuracy of predicted 3D point clouds compared to the actual object shape can be tricky, and one way to do it is by checking how well the projections of the predicted 3D points match the silhouette of the real object.
7 Notable Examples of Computer Vision in Manufacturing & Key Applications
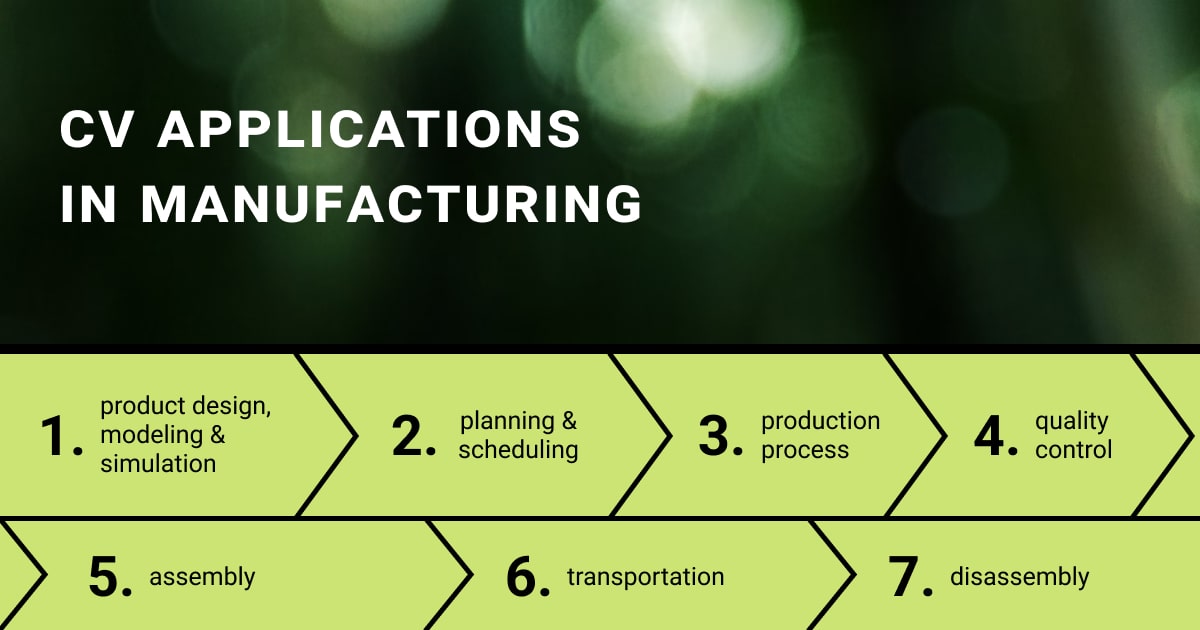
The entire product lifecycle involves several stages in manufacturing, which often overlap. Despite the challenge, CV has found its applications in each of these stages.
1. Product Design, Modeling, & Simulation
In the product lifecycle’s initial stage, design aims to innovate or enhance products. Computer-aided design (CAD) and computer-aided manufacturing (CAM) have long been integral to manufacturing. CAD harnesses computers to create valuable 3D models for prototyping.
Computer vision technology in manufacturing contributes by transforming 2D images into 3D models and validating designs. It also aids in reconstructing 3D models from 2D product images, generating solid models from scans, and estimating 3D poses using 2D images and CAD data. As CAD evolves, interdisciplinary modeling gains traction, reducing 3D model reconstruction needs. The current challenge is to explore fresh applications for CV technology in product design and modeling.
2. Planning & Scheduling
Once product design is confirmed, the next step involves crafting a production plan, specifying subsequent actions and schedules for execution. In lumber production, for instance, computer vision plays a pivotal role by aiding in lumber sawing plan creation. It analyzes CT images to identify internal defects, enhancing sawing strategies.
Computer vision also extends its utility to additive manufacturing and CNC production planning. This encompasses tasks like optimizing 3D printing component placement and generating welding path plans for golf club heads. Nonetheless, real manufacturing settings introduce uncertainties like machinery breakdowns, demanding dynamic plan and schedule adjustments based on real-time visual data.
3. Production Process
The production phase is pivotal in a product’s life cycle, where raw materials transform into finished goods through diverse operations. Manufacturing-oriented computer vision systems are instrumental, encompassing several crucial tasks. These include production process control, where CV manages processes like mineral wool trajectory control, fiber measurement, and iron industry bubble analysis. It also guides robots with methods like stereo vision, ensuring safe human collaboration.
Part classification relies on CV to categorize products based on attributes like color and texture, utilizing both traditional and modern approaches like CNNs. Furthermore, computer vision accurately measures 3D positions of parts, products, and tools using recognition and triangulation techniques. Lastly, CV enhances production safety by identifying helmets, preventing tool-component collisions, and providing control instructions in uncertain environments.
4. Quality control
Computer vision in manufacturing is widely employed for quality control, encompassing measurement, examination, and testing to assess specific object features and ensure quality standards. Effectively managing the substantial data generated by vision-based inspection systems remains a challenge.
CV applications for inspection cover mechanics, where it inspects surfaces, identifies damaged parts, and aids in defect detection using techniques like blob analysis and CNN segmentation. In the automotive sector, CV measures surface characteristics and detects defects, while in 3D printing, it ensures quality through printhead calibration and defect identification. Other industries benefit from CV-based inspections, covering areas such as fiber defect detection, electronics defect identification, and anti-counterfeiting product identification through vision-based matching algorithms.
5. Assembly
Assembly, a critical process in discrete manufacturing like automotive and aviation, involves combining parts into semi-finished products and then into final products. Achieving safe and intelligent human-robot collaboration in assembly remains a challenge. Computer vision plays a pivotal role in automatic assembly, aiding in motor stator assembly, cabin product assembly, packaging with assembly robotics, and automatic part picking and placing. For instance, it facilitates the assembly of flexible printed circuit cables onto hard disk drives in printed circuit board assembly.
Computer vision in the manufacturing industry is also essential for assembly quality control, detecting errors through statistical pattern recognition and identifying human errors in bolt securing during automotive assembly. Additionally, augmented reality (AR) and CV are combined to guide assembly operations, including recognizing human motions in mechanical assembly and challenging single-image-based 3D part assembly.
6. Transportation
Transportation in manufacturing involves material movement and logistics management, but CV systems face challenges from complex lighting conditions causing image variation. AGVs, equipped with navigation, power, and control systems, handle material, part, and product delivery. Computer vision aids AGV navigation, including path planning and obstacle detection, through local and remote guidance methods.
In logistics, CV extends to larger autonomous transportation systems like forklift trucks, employing covariance matrix algorithms for object detection and tracking, as well as autonomous driving vehicles. Improved denoising and normalization techniques are essential to enhance the robustness of transportation CV systems in varying lighting conditions.
7. Disassembly
Disassembly, crucial for responsible product disposal, is now more feasible with robotic advancements and CAM techniques. Historically, manual disassembly was labor-intensive, but automation reduces costs. Computer vision systems, essential in semi-automatic and fully automatic disassembly, generate sequences and control robots.
Complex products benefit from computer vision techniques, which create assembly structures from images, aiding sequence generation. CV also guides disassembly robots in automotive, electronics, and display product disassembly, with features like gray scale and depth aiding screw detection. Yet, human involvement remains necessary in current systems, highlighting the importance of safe human-robot interactions during disassembly.
Examples of Computer Vision in Manufacturing: Top Companies Leading the Way
In the realm of manufacturing, several computer vision companies are making significant strides:
- Mech-Mind Robotics, China’s leading 3D vision company, offers integrated hardware and computer-aided software solutions that streamline manufacturing tasks, including bin picking, machine tending, and assembly, with quick robot calibration.
- Instrumental, based in Palo Alto, CA, focuses on electronics manufacturing, using computer vision and predictive analytics for real-time monitoring and defect detection, catering to both low and high-volume production.
- Protex AI, an Irish startup, enhances safety in manufacturing facilities using computer vision via existing CCTV infrastructure. Their platform allows users to create custom safety rules and employs object detection and tracking for rule enforcement.
- Cognex, a global leader, supplies manufacturing-oriented computer vision systems and barcode readers. Their products improve product quality, reduce costs, and enhance efficiency in assembly, inspection, and sorting processes.
- RIOS Intelligent Machines aims to automate factories using AI-powered robotic workcells. They offer advanced hardware, such as tactile sensors and grippers, and Factory Automation-as-a-Service for flexible automation solutions.
- Omron Automation delivers integrated solutions, combining automation products like industrial robots and vision sensors for industries including automotive and food and beverage.
- ISRA VISION provides 3D imaging and inspection solutions for various industries, including manufacturing, ensuring quality control, defect detection, and process automation.
These companies are at the forefront of computer vision-powered devices and solutions, enabling manufacturers to streamline operations, improve product quality, and enhance safety. But if you have a specific request for your AI project in manufacturing, here’s a handy spreadsheet with top computer vision tooling providers.
Exploring the Benefits of Computer Vision in Manufacturing
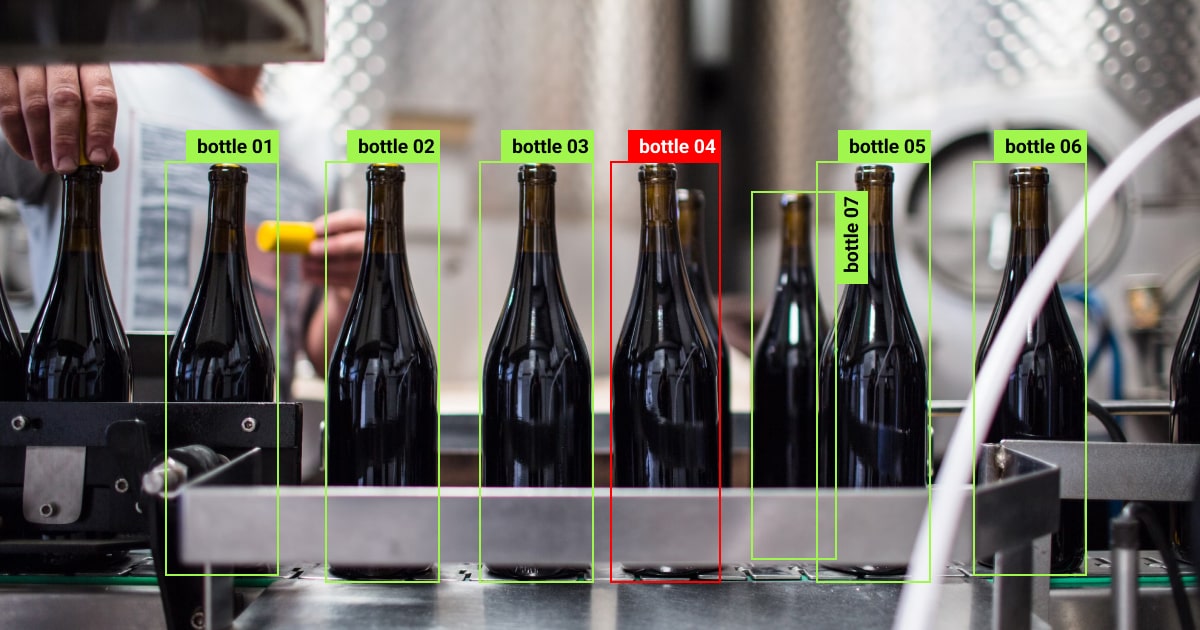
CV technology holds numerous advantages in the manufacturing industry:
Enhanced productivity
Computer vision technology in manufacturing accelerates production cycles, resulting in increased labor productivity by 12% and total production output by 10%, as reported by Deloitte. This is achieved through real-time data analysis and process optimization.
Cost optimization
Cost efficiency is a key benefit, as automation and computer vision-based maintenance can reduce operating costs by up to 50%. This occurs due to higher productivity and less machine downtime.
Improved product quality
In terms of product quality, robots guided by computer vision operate with precision, leading to a 10-20% reduction in quality assurance costs, according to McKinsey, while also helping to avoid human errors.
Workforce safety
One of the most important benefits of computer vision in manufacturing is its ability to enhance employee safety by identifying malfunctions, monitoring worker conditions, and detecting signs of fatigue or discomfort. Another study shows how computer vision technology in manufacturing was also suggested for monitoring workers via CCTV to ensure safety, including social distancing and face mask detection.
Understanding the Challenges of Adopting Computer Vision in Manufacturing
Implementing computer vision (CV) in manufacturing is no easy task, as it comes with various hurdles and complexities:
Adoption hurdles
The rapid advancements in CV technology, like convolutional neural networks (CNNs) and deep learning models, often remain disconnected from practical manufacturing applications. Established computer vision systems for manufacturing still rely on conventional algorithms, such as SVM and k-nearest neighbors (KNNs). Large-scale companies may have the resources but face complexity integrating new CV research into multifaceted manufacturing processes, distinct from ideal problem models.
Data collection
Gathering high-quality data, especially in complex manufacturing environments with lighting issues and reflective surfaces, remains a persistent challenge. Poor lighting and reflective surfaces introduce biases in image capture, even with the growing prevalence of Internet of Things (IoT) and sensor technologies.
Data preprocessing
As data collection devices proliferate, the volume of structured and unstructured data escalates. However, effective data preprocessing mechanisms are lacking, leading some companies to store data temporarily, incurring higher costs and reduced processing efficiency. In that case, they need expert additional services to deal with data preprocessing for computer vision in manufacturing.
Data labeling
Though more visual data is available, it often lacks essential labels for supervised learning. So it’s important to find a reliable partner offering high-quality data annotation services for the retail industry and manufacturing in general. Otherwise, adopting an ML-based computer vision solution in manufacturing necessitates better algorithms for handling unlabeled data and automatic labeling methods.
Benchmarking challenges
Existing benchmarks, like COCO and UA-DETRAC, cater to specific tasks like vehicle detection and aren’t readily applicable to manufacturing scenarios. More industry-specific benchmarks are needed to continuously advance computer vision applications in manufacturing.
Final Takeaways
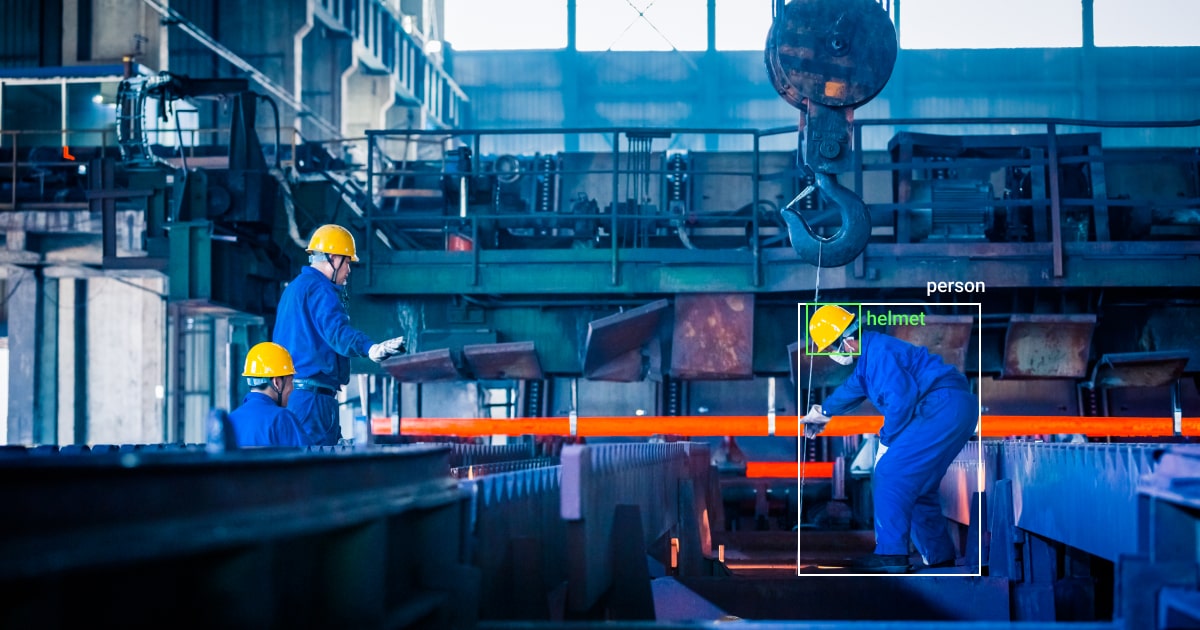
Summing up, computer vision’s impact on manufacturing is undeniable. It streamlines processes, enhances quality, and predicts maintenance needs. Yet, it’s vital to remember that humans still bring creativity and adaptability to the table.
Hence, balancing automation and human expertise will be the key to a bright future in manufacturing. As we embrace this technological revolution, let’s not forget that the most successful outcomes often arise from the collaboration between human ingenuity and the precision of machines.
FAQ
How reliable are computer vision systems in manufacturing?
The reliability of computer vision systems in manufacturing depends on the quality and accuracy of data annotation, as well as the robustness of the underlying algorithms and hardware.
What is computer vision for manufacturing quality control?
Computer vision for manufacturing quality control is a CV-based automated system that uses cameras and image analysis algorithms to inspect and assess the quality of products on production lines, ensuring they meet specified standards and detecting defective objects or discrepancies.
What are the emerging computer vision applications in manufacturing that are revolutionizing production processes?
Some prominent CV applications in manufacturing encompass quality inspection, object tracking, robotic automation, defect classification, predictive maintenance, inventory management, AR in assembly, process optimization, safety monitoring, and gesture control.
Written by
One of the technical writers at Label Your Data, Yuliia has been gradually delving into the intricate aspects of AI. With her strong passion for the written word and technical expertise, Yuliia has developed a keen interest in the evolving field of data annotation and the power of machine learning in today's tech-savvy world. Check out her articles to learn more about the complex world of technology and find the solutions that work best for your AI project!