- What You Need to Know About Sora
- Training Sora: Data and Process Behind Text-to-Video Generation
- What Sora Can Do and What It Can’t (Yet) in Text-to-Video Generative AI
- Exploring Alternative AI Video Generation Tools
- How Label Your Data Can Help
- FAQ
Creating quality video content requires a significant investment in equipment, skilled pros, and a dash of creative vision. But OpenAI’s recent introduction of Sora, a groundbreaking text-to-video model, disrupts this traditional workflow.
Inspired by the Japanese word for “sky,” the model’s name reflects its potential for limitless creativity (the sky’s the limit, right?). Sora allows users to generate video scenes where real-life scenes are mixed with imaginative elements by simply providing a text prompt. In this blog post, we’ll explain what OpenAI’s Sora is, how it functions, and the potential applications it offers.
What You Need to Know About Sora
This new AI-powered video generator can create high-quality, minute-long videos that closely follow the user’s instructions provided in the written form. Sora can handle intricate scenes with many characters, specific movements, and detailed backgrounds. Remarkably, the text-to-video model grasps not just the user’s words, but also how these elements function in the real world.
While OpenAI intends to eventually release Sora to the public, no timeframe has been announced. Currently, access is limited. A select group of specialists (called “red team”) focused on identifying potential issues like misinformation and bias are conducting thorough testing. Additionally, filmmakers and artists are providing feedback on its potential in their fields.
To identify manipulated content, a popular and much-needed practice in AI content moderation, OpenAI is actively developing tools, including a classifier specifically designed to detect videos generated by Sora.
Looking for expert content moderation services? Contact us!
Training Sora: Data and Process Behind Text-to-Video Generation
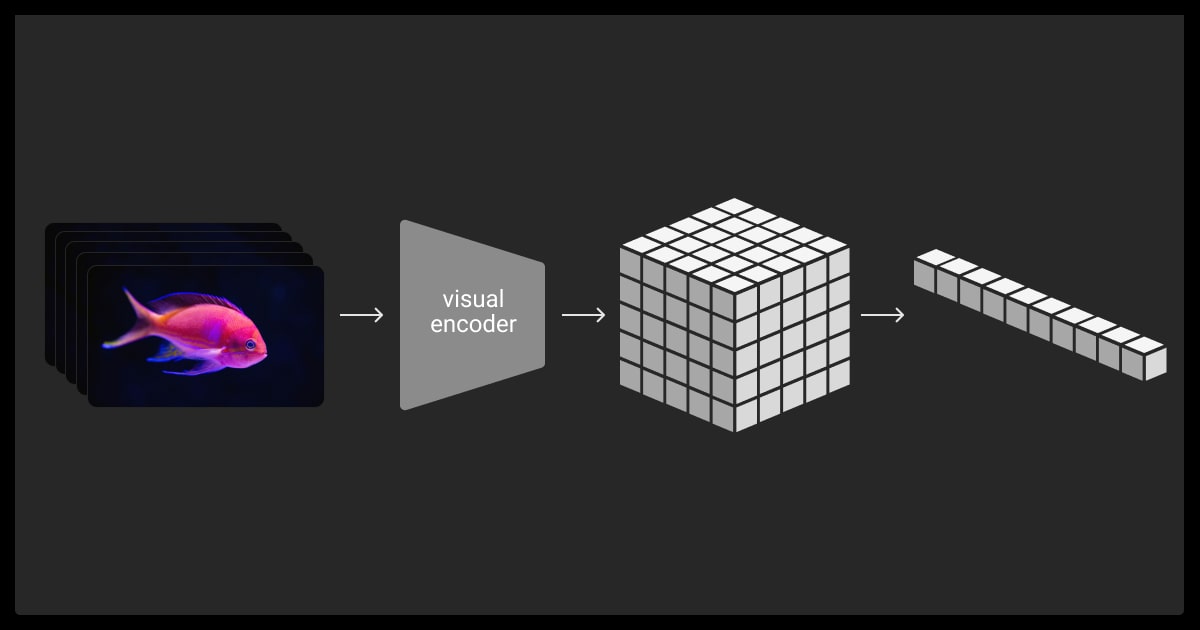
OpenAI has unveiled how they approach training of text-to-video models, specifically Sora, in their technical report. Here’s a simplified breakdown for our time-pressed readers who don’t want to miss out on any details.
Sora operates on the principle of diffusion models. It starts with a video resembling static noise and progressively refining it by eliminating the noise in a multistep process. This way, the model can create intricate details and smooth transitions within the generated video.
Compared to other text-to-video models, Sora can not only independently generate entire video sequences from scratch but also seamlessly extend existing footage, ensuring object coherence through its multi-frame foresight.
Inspired by GPT models’ architecture, Sora leverages a transformer architecture (operating on spacetime patches of video and image latent codes), enabling it to excel in terms of scalability. It leverages DALL-E 3’s recaptioning technique, where detailed descriptions enrich training data. This enables Sora to meticulously follow user instructions within the generated video.
Beyond text-to-video generation, Sora breathes life into static images with detailed animations. It can even manipulate existing videos by extending or completing missing frames. This way, Sora serves as a foundation for the development of future models capable of comprehending and simulating the real world — a crucial milestone towards achieving Artificial General Intelligence (AGI).
How Data Is Fueling Text-to-Video Generative AI
Training data received from professional video annotation services plays a vital role in the success of video generation models. Traditionally, text-to-video AI models were trained on limited datasets with shorter videos and specific goals.
However, OpenAI doesn’t reveal the source of training data used for Sora. They mention needing vast amounts of captioned videos, hinting at the internet’s role in training Sora. However, the exact source of visual data remains unknown. All we know is that Sora utilizes a diverse and extensive dataset encompassing videos and images with varying lengths, resolutions, and aspect ratios.
The model’s ability to recreate virtual environments suggests its training data likely includes gameplay footage and simulations from game engines. This comprehensive approach makes Sora a “generalist” model in the artificial intelligence in media domain, similar to how GPT-4 operates in the NLP realm.
Similar to how large language models (LLMs) are trained on vast text datasets, the same principle was applied to Sora using video and image annotation services, as well as text annotation services. Moreover, Sora leverages “spacetime patches” for text-to-video tasks. This method enables effective learning from the vast dataset, enhancing the model’s ability to produce high-fidelity videos while requiring less computational power compared to existing architectures.
Get high-quality training data for your video generation projects!
The Process of Training of Text-to-Video Models: A Look at Sora’s Case
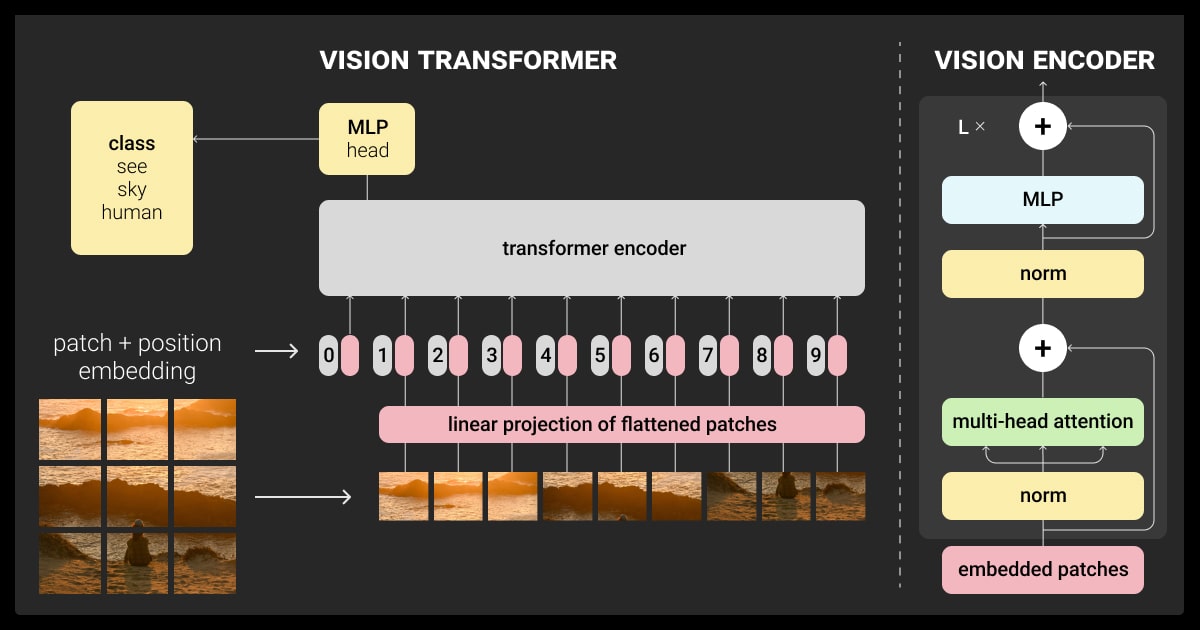
Here we explore the training process behind text-to-video models, using OpenAI’s Sora as a prime example.
-
Transforming visual data into patches
Inspired by successful LLMs that learn from vast amounts of text data, Sora uses a similar approach, but with “visual patches” instead of text tokens. These patches are efficient for training generative AI models on diverse video and image formats. Videos are first compressed into a lower-dimensional form, then broken down into space-time segments to create these patches.
-
Video compression network
A specialized network condenses the video data. This compressed version, capturing both temporal and spatial information, serves as the training ground for Sora. The network also includes a decoder that converts the generated compressed form back into a full video.
-
Spacetime latent patches
From the compressed video, smaller segments combining spatial and temporal data are extracted, acting like individual tokens in language models. This approach works for both videos and images (seen as single-frame videos).
Patch-based representation allows Sora to handle videos and images with varying resolutions, lengths, and aspect ratios. During video generation, the model can control the final size by arranging randomly generated patches in a specific grid.
-
Scaling transformers for video generation
Sora is a type of diffusion model. Given noisy visual patches and additional information like text instructions, it learns to reconstruct the original clear image. Notably, Sora uses a transformer architecture, known for its ability to handle various tasks like language processing, image generation, and now, video creation.
*As training progresses with more computational power, the quality of the generated videos demonstrably improves.
-
Embracing diverse formats
Traditional video generation methods often involve resizing or cropping videos to a standard format. Sora bypasses this step by training on data in its original size, offering several advantages.
-
Flexible sampling
Sora can directly generate content in various aspect ratios, from widescreen 1920×1080p videos to vertical 1080×1920p phone formats. This allows for creating content tailored to different devices without additional resizing steps.
-
Enhanced framing and composition
Training on videos in their original aspect ratios demonstrably improves the final video’s framing and composition. Compared to a model trained on cropped squares, Sora (trained on various aspect ratios) produces videos where subjects are fully visible and positioned well within the frame.
-
Language understanding
Training a text-to-video generative AI system requires a vast amount of video data paired with corresponding textual descriptions. Similar to DALL-E 3, Sora leverages a technique called “re-captioning” to create detailed captions for the videos in the training set.
Additionally, GPT is used to expand short user prompts into comprehensive descriptions that guide the video generation process. This combined approach allows Sora to create high-quality videos that closely match the user’s intent.
What Sora Can Do and What It Can’t (Yet) in Text-to-Video Generative AI
To start with, while the basic task the model performs is text-to-video generation, Sora’s abilities extend further. It can use various inputs beyond text prompts, including existing images and videos. This way, you can use Sora to perform diverse editing tasks:
Seamless video loops: Create videos that seamlessly repeat without any noticeable interruptions.
Static image animation: Image-to-video generation by transforming still images into video sequences.
Video extension: Extend existing videos forward or backward in time, maintaining a cohesive narrative.
Animating images
Sora can generate videos based on a combination of an image and a textual prompt. For instance, it generated videos derived from images created by DALL-E 2 and DALL-E 3.
Extending video length
Sora can lengthen videos, either by adding footage before the original content or extending it after the ending. This technique can be used to create infinite loops by extending a video both forward and backward.
Blending videos
Sora can create smooth transitions between entirely different video clips. This is achieved by progressively interpolating between the two input sources, resulting in a seamless blend despite contrasting subjects and settings. Check out OpenAI’s report to see how Sora can seamlessly connect two different videos.
Image generation
Beyond video generation, Sora can also produce images. This is accomplished by strategically arranging patches of random noise in a grid-like structure with a single frame duration. The model can generate images in various sizes, with a maximum resolution of 2048×2048.
Simulation skills
Training video models at large scales unlocks a range of unexpected capabilities. They allow Sora to simulate certain aspects of the real world, including individuals, animals, and their environments. Notably, these properties emerge without explicitly incorporating biases towards 3D objects or environments; they solely arise from the model’s extensive training.
Maintaining 3D consistency: Videos generated by Sora exhibit consistent movement of people and objects within a 3D space, even with dynamic camera movements.
Long-range coherence and object persistence: A longstanding challenge in video generation has been ensuring temporal consistency for longer videos. Sora is able to model both short-term and long-term dependencies, effectively maintaining the presence of individuals, animals, and objects throughout the video, even during occlusions or when they exit the frame. Additionally, the model can generate numerous clips featuring the same character while preserving their appearance.
Limited environmental interaction: In some instances, Sora can simulate actions that subtly alter the environment.
Simulating digital environments: Sora can even simulate artificial processes, like video games. The model can concurrently control a player within Minecraft using a basic strategy, while simultaneously rendering the game world and its dynamics in high detail. These functionalities can be triggered without any additional instructions by simply providing prompts mentioning “Minecraft.”
Continually scaling text-to-video models holds immense potential for the development of powerful simulators capable of replicating the physical and digital worlds.
However, like any AI model, Sora has certain shortcomings:
It might struggle to depict complex physics, such as how objects interact or break. Additionally, the model might not always fully grasp the cause-and-effect relationship within a scene. Spatial awareness can also be an issue, with Sora potentially confusing directions like left and right. Furthermore, describing events that unfold over time, like specific camera movements, can be difficult for the model.
Adding to these limitations, Sora itself acknowledges difficulties in simulating the real world. These include:
Inability to accurately portray basic physical phenomena, like how glass shatters.
Interactions with objects, such as eating, might not always result in the expected changes to their state.
Videos generated for longer durations can become illogical or contain objects appearing out of nowhere.
Exploring Alternative AI Video Generation Tools
While Sora offers a compelling solution, there are several other noteworthy options in the text-to-video generative AI domain, including both open source AI video generators and proprietary solutions:
-
Established players:
Runway Gen-2: This leading competitor mirrors Sora’s capabilities, functioning as a text-to-video AI accessible through web and mobile platforms.
Google’s Lumiere: A recent offering from Google, Lumiere integrates as an extension within the PyTorch deep learning framework.
Meta’s Make-a-Video: Unveiled in 2022, this tool by Meta also leverages the PyTorch framework.
-
Specialized solutions:
Pictory: Caters to content creators and educators by simplifying text-to-video synthesis.
Kapwing: Provides a user-friendly online platform for crafting videos from text, ideal for social media content and casual use.
Synthesia: Geared towards presentations, Synthesia offers AI-powered video creation with customizable avatars for businesses and educational institutions.
HeyGen: Streamlines video production for various purposes including product marketing, sales outreach, and educational content.
Steve AI: This platform facilitates video and animation generation from various inputs like text prompts, scripts, and even audio.
Elai: Focuses on the e-learning and corporate training sector, enabling instructors to effortlessly convert their materials into engaging video lessons.
How Label Your Data Can Help
Generative AI text-to-video models require high-quality training data in order to translate users’ text prompts into visual content. The ML datasets for video generation models need massive volumes of labeled video clips and corresponding descriptive text sentences. Our skilled data annotators can enrich your dataset with details like object presence, actions, scene flow, and even storyboards to facilitate your text-to-video model development.
We can solve the most common text-to-video dataset challenges:
Limited labeled data: Training requires a massive amount of labeled videos.
Biased models: Imbalanced data leads to skewed model results.
Costly video annotation: Manually labeling videos is expensive and time-consuming.
Copyright issues: Using web videos for training might raise legal concerns.
FAQ
What text-video data was used to train Sora?
While OpenAI keeps the specific source of text-video data used to train Sora under wraps, they acknowledge the need for a massive collection of captioned videos. This suggests the internet likely plays a role in Sora’s training. However, the exact details of the visual data source remain unknown.
How do text-to-video AI models, like Sora, function?
Generative AI text-to-video models like Sora are trained on massive datasets of text and video pairs to analyze the meaning and context of a text prompt, then transform text descriptions into videos. This process often involves deep learning techniques, specifically:
Recurrent Neural Networks (RNNs) that decipher the text
Convolutional Neural Networks (CNNs) that analyze the corresponding visuals
Generative Adversarial Networks (GANs) that iteratively refine the generated video based on the prompt and the learned video data.
They allow the model to translate the text description into a sequence of images forming a cohesive video.
How can I access Sora?
As of March 2024, only specialists who test and pinpoint issues in Sora, called “red team”, can use the model. This helps developers at OpenAI fix all the problems before making Sora available to the public. While there’s no official release date yet, it’s expected to launch sometime in 2024.
Table of Contents
Get Notified ⤵
Receive weekly email each time we publish something new: