Large Action Models: Applications and Benefits
Table of Contents
- TL;DR
- What Are Large Action Models?
- How Do Large Action Models Work?
- Applications of Large Action Models Across Industries
- Popular Large Action Models Examples
- LAMs vs. LLM Agents
- Key Benefits of LAMs for AI Workflows
- Challenges to Overcome
- Future Predictions for Large Action Models
- About Label Your Data
- FAQ
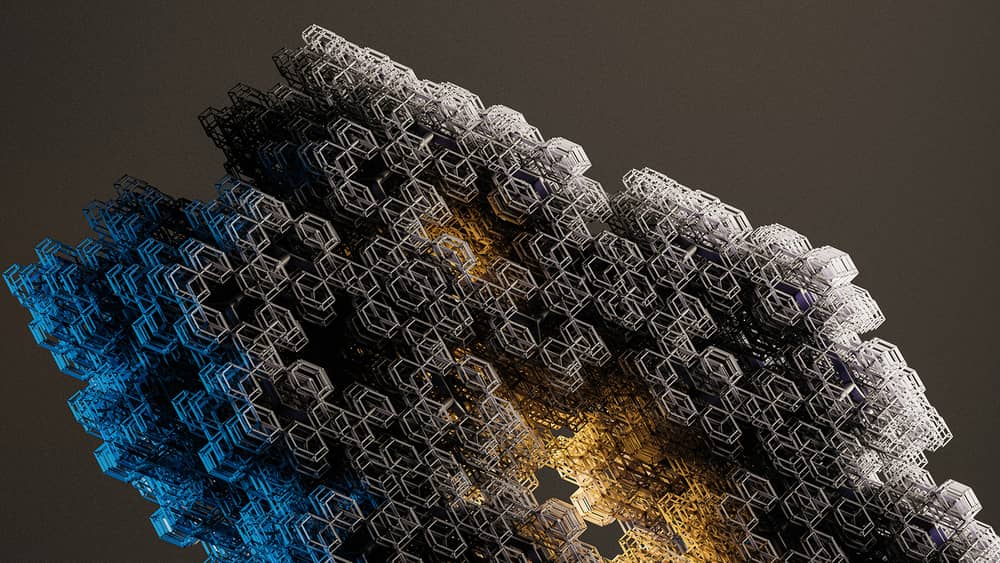
TL;DR
What Are Large Action Models?
Large action models, or simply LAMs, are AI systems that go beyond passive analysis and recommendations. We design them to understand user intent and execute real-tasks.
These models can not only complete complex actions, but they can make decisions based on the information available to them. They can:
Automate repetitive tasks
Improve efficiency
Adapt to new challenges on the spot
Say, for example, you’re comparing ad campaigns. A traditional AI system will analyze performance and see which ads performed best. It might then make recommendations based on the results.
LAMs would take things a step further. They might see that a customer fits particular criteria and serve a version of an ad that is most likely to appeal. Alternatively, they could take all the information and create new content that draws on the successes of previous campaigns.
How LAMs Change AI-Driven Workflows
Large action models AI can automate complex tasks and provide real-time adaptability, especially in data-intensive industries. They’re particularly useful in areas where you need to make split-second decisions.
LAMs can:
Perform complex workflows like managing data pipelines and scheduling tasks.
Interact with digital and physical systems, like with smart assistants and robotics.
Make decisions based on real-time data and learned experiences without human input.
They’re a lot more versatile than rule-based AI.
How Do Large Action Models Work?
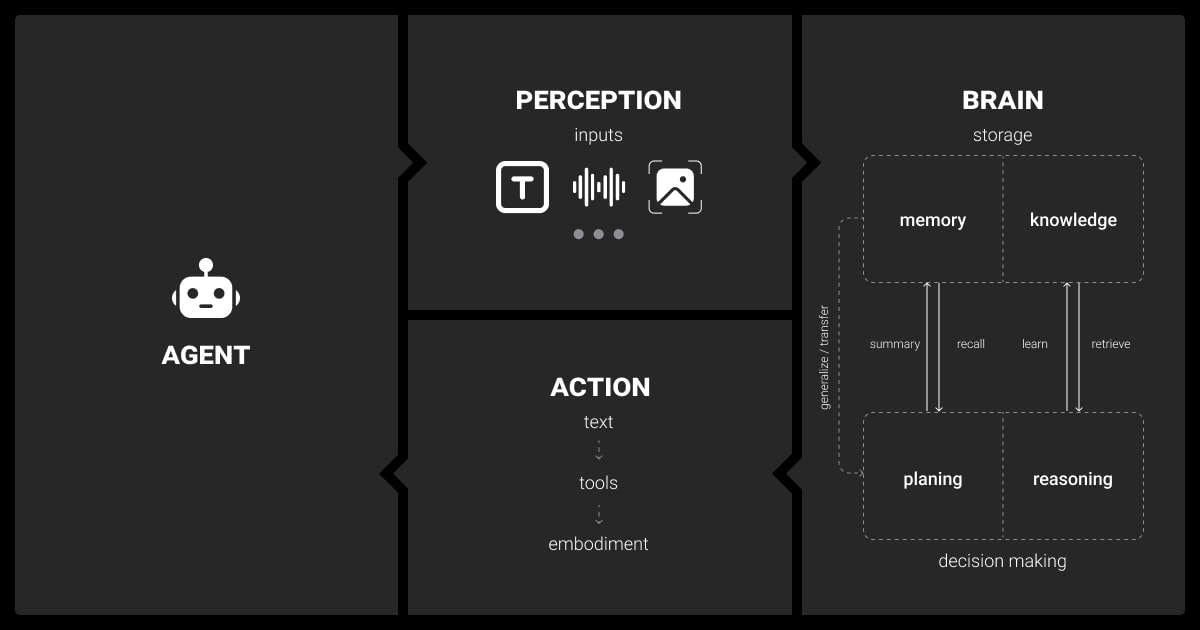
So, clearly LAMs are highly capable, but how do they actually work? Let’s dive right in.
Building on Language Models
LAMs help the more traditional large language models (LLMs) do more by integrating:
APIs
Application Programming Interfaces dictate how one application “speaks” to another. APIs ensure that different software can communicate and work together.
Databases
You might link up different data sources like purchase history, customer interactions, and knowledge bases.
External tools
You might want to incorporate other tools to improve usability. For example, you might work in a tool that can track packages.
A standard large action model only generates text. With an LAM in the mix, your LLM can interact with software platforms, automation tools, and even robotics.
Training and Fine-Tuning
How does an LAM get so clever? The secret sauce is the training you provide the model. You need to allow it to learn from real-world actions.
This can include annotated datasets and historical user actions that pertain to the industry you operate in. You’ll fine-tune the AI so that it performs the tasks you want it to.
Here, too, the training differs slightly from that of LLMs. You need to review the solutions the AI comes up with and reinforce the good decisions.
Applications of Large Action Models Across Industries
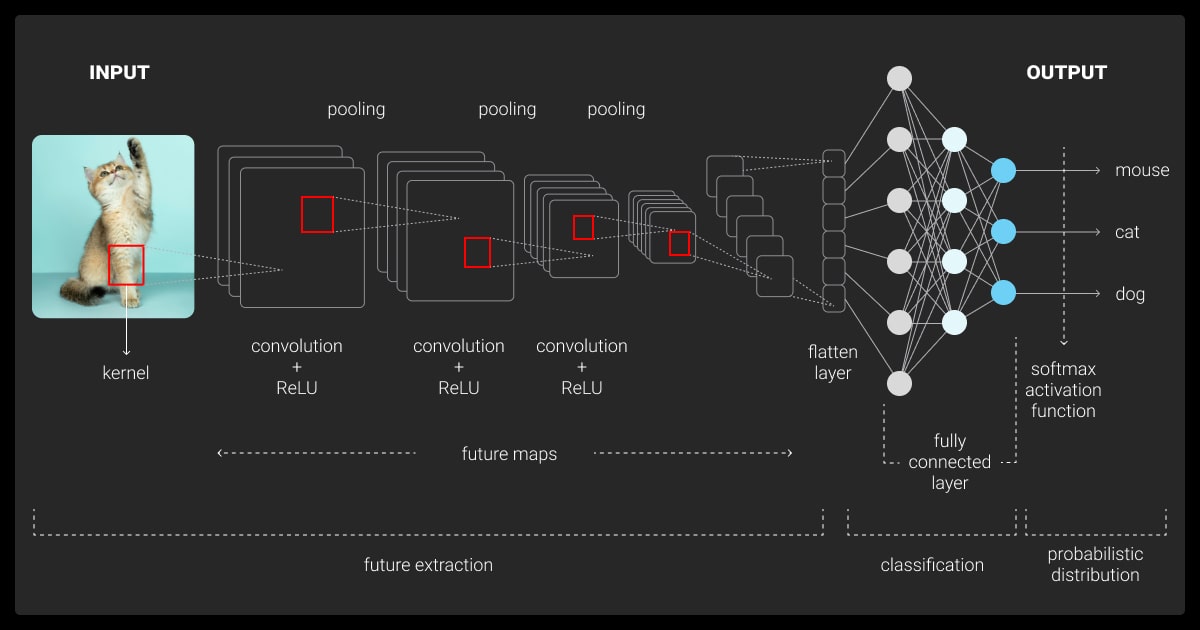
Where are LAMs most useful? We can use them across multiple industries, so let’s go over the main ones here.
Data Annotation Automation
LAMs make it easier to annotate data by automating the labeling process. It quickly categorizes unlabeled data, which means you can train AI models more quickly.
It also means that a data annotation company can upscale production easily. You can also drill down into basics like image classification vs. object detection.
You’ll require fewer annotators, meaning that data annotation pricing will drop. Using AI here means a greater consistency and accuracy with labels. It also means that data annotation services can become more competitive.
Better Accuracy
As an ancillary benefit, AI improves accuracy. Human annotators might have very different ideas of what an image or even text might mean. For example, one might call a puppy a dog and another a pup. The distinctions in data annotation are pretty much meaningless to the human mind, but they can be confusing for the AI you’re training.
ML Workflow Optimization
LAMs improve machine learning workflows by:
Managing large datasets
Refining annotations for AI training
Expediting iterative feedback cycles so you get useful insights quickly
Robotics and Automation
LAMs make it possible for robots and autonomous systems to execute precise real-world actions. We use them in transportation, manufacturing, and logistics. With LAMs, machines can make decisions on the floor, improving production. They allow us to automate complex physical tasks.
Smart Assistants and Virtual Agents
Step aside Siri and say hello to intelligent virtual assistants that can handle multistep processes like:
IoT device management
Customer interactions
Scheduling
LAMs also improve automatic speech recognition for voice-based assistants.
Healthcare and Biomedicine
LAMs are coming into their own in healthcare. They can:
Support surgical robotics
Automate patient data analysis
Accelerate drug discovery
Enhance image recognition
What this essentially means is that a doctor might feed the patient’s test results and X-rays into the system. AI will then suggest potential diagnoses and recommend treatment options. The doctor will choose the best one based on their experience.
This will improve clinical outcomes for patients because it ensures that no one misses anything. It will also speed up the diagnostic process.
Large action models have transformed emergency room operations, reducing patient wait times by 40% by automatically prioritizing cases based on symptoms and medical history. The system even flagged my neighbor’s unusual chest pain pattern, alerting specialists before things got worse.
Popular Large Action Models Examples
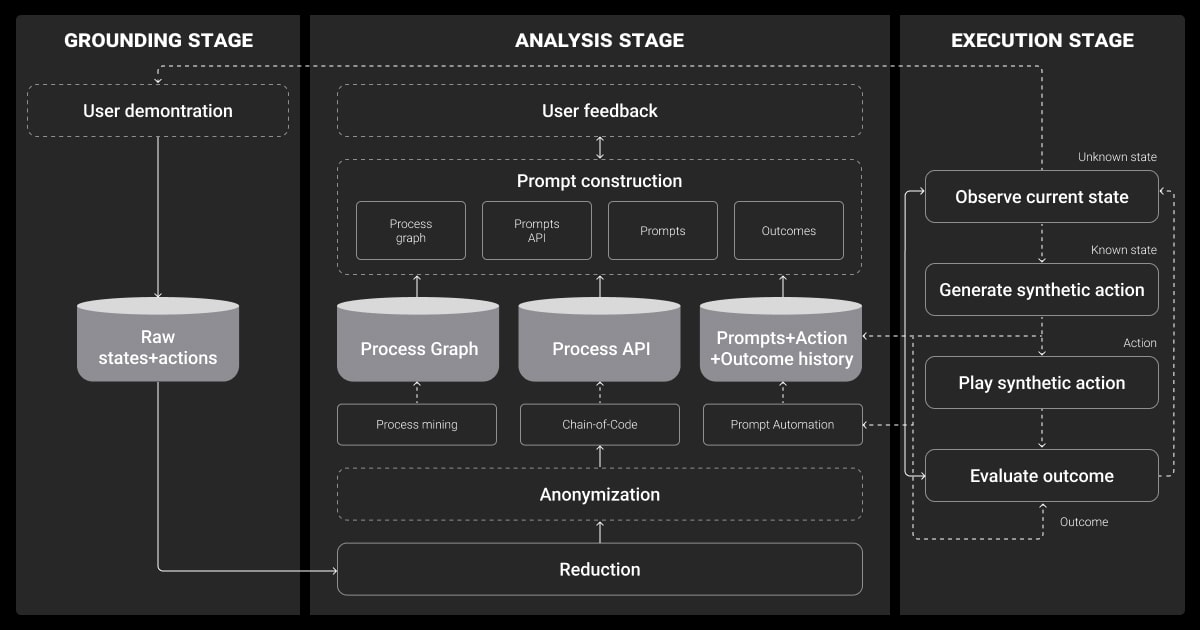
So, how widespread is this technology? Where can we see examples in action?
Claude’s Computer Use
Anthropic’s Claude automates tasks like navigating the digital environment. It improves workflows, speeding up the processes.
Rabbit AI’s R1
This smart tool makes it a snap to manage your smart device. It also acts somewhat like a personal assistant, handling tasks like scheduling.
Adept AI’s ACT-1
Adept AI’s Action Transformer model focuses on automating workflows and enhancing enterprise applications.
Salesforce xLAM
This model supports enterprise-scale automation and data-driven decision-making.
LAMs vs. LLM Agents
These look like two competing models, but they’re likely to work hand in hand. The most basic difference is in how far the system goes. You can train different types of LLMs to perform a basic task, like answer a customer query. If that customer wants you to process a refund, the LLM might hand that over to the LAM.
Core Differences
LAMs systems go beyond simple language tasks. They’re useful for detecting objects and image recognition as well. They can interact with other systems or environments, making them extremely useful.
LLM agents primarily focus on conversational and text-based interactions. They’re more specialized than LAMs. Since LLMs focus primarily on text processing, LLM data labeling tasks are simpler than LAM-based workflows, which must interact with multiple systems.
Use Cases
The system you choose depends on what you need the application to do. You would choose:
LAMs when you need an action-based workflow. For example, if you want the application to be able to schedule appointments autonomously.
LLMs for language processing tasks like translation, chatbots, or content creation.
Overlap and Collaboration
What if you want a chatbot that can also help customers schedule an appointment? You could set up the bot using an LLM and then expand its capabilities with an LAMs.
In the future, we’ll see LAMs and LLMs working in conjunction with one another.
Key Benefits of LAMs for AI Workflows
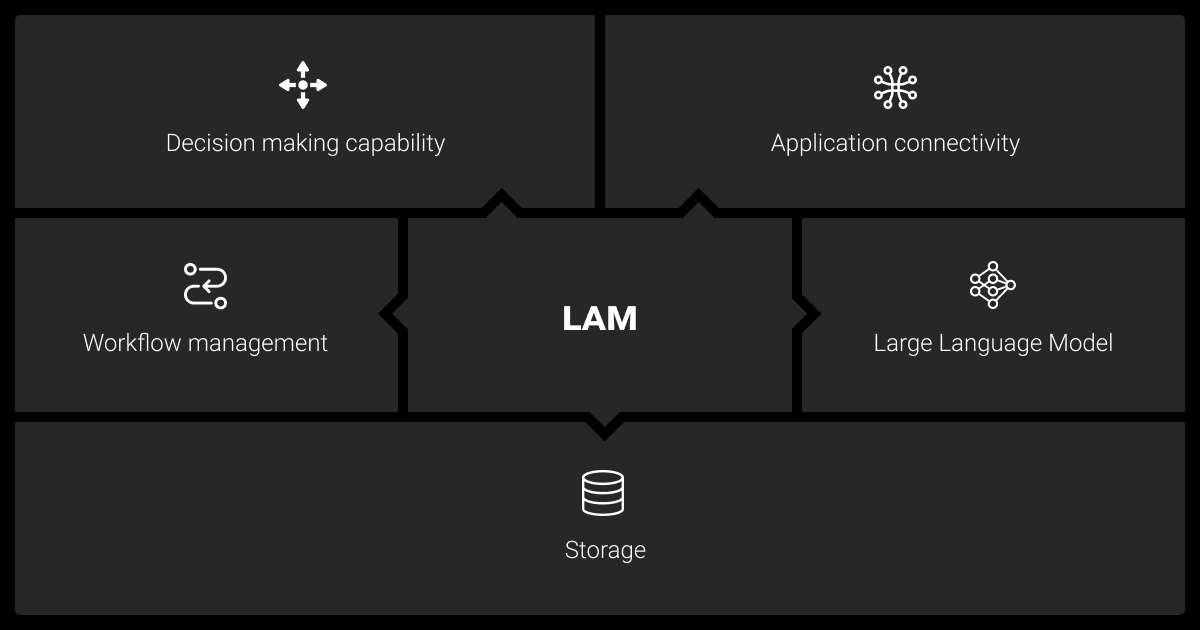
Now let’s look at why LAMs are so useful for AI workflows.
Efficiency and Scalability
LAMs reduce the manual workload by handling repetitive tasks. This makes it easier to scale for large projects.
Adaptability Across Tasks
LAMs adjust to changing scenarios in real time. They can also work with several types of data. This adaptability ensures that they perform consistently across various use cases.
Enhanced Decision-Making
LAMs can provide actionable insights using up-to-the-minute data, even for the most complex tasks. The system uses this information to optimize its operations. This is extremely useful in areas where time counts, like stock trading and risk management.
Multimodal Integration
LAMs work across text, images, video, and audio data in one cohesive system. This enhances OCR deep learning applications. It can also improve object detection and image classification for AI systems.
Cost Optimization
Human agents find repetitive tasks boring. They’re prone to lose focus and make errors. LAMs not only handle these tasks faster, but they don’t make the same mistakes. This can, for example, lower the cost of labeling your dataset.
By integrating large action models, platforms can automatically label incoming content, flagging items that might contain hate speech, harassment, or explicit imagery. This speeds up moderation, ensures a safer community environment, and reduces operational costs.
Challenges to Overcome
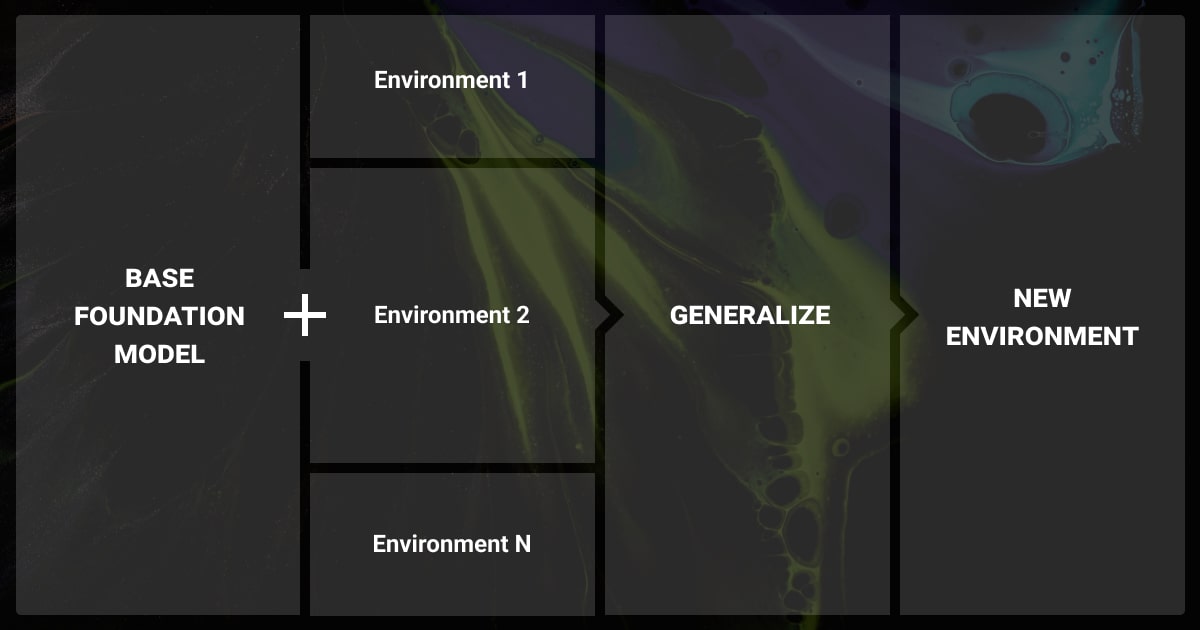
Here are the challenges you’ll need to overcome to make LAMs work for you.
Data and Infrastructure Requirements
You’ll need to train your AI to complete all the tasks. This means looking for datasets bigger than LLMs. You’ll need to dig deep to pay for computational power and will have to use an advanced machine learning algorithm.
Ethical and Security Considerations
You need to make sure your users know that the AI works on the information it has. It doesn’t have the same gut feelings a trader might have. Finally, you need to build in safeguards to prevent misuse.
Avoid Perpetuating Harmful Stereotypes
You also have to make sure you have a clean, unbiased dataset to train your model on. If you don’t, your model might perpetuate unhelpful stereotypes. Say, for example, you create an application to sort through resumes, conduct pre-interviews, and schedule formal interviews. If the training data is from older texts where only men were doctors, the AI might ignore female candidates.
Complexity of Fine-Tuning
Like with LLMs, you need to train your LAM to meet the needs for your specific domain or industry. This means feeding it examples that it can learn from. In some industries, this is challenging because of the technical nature of the information.
For example, if you’re creating a program to identify issues with crops, you might need expert geospatial annotation. You’ll not only need high-quality data, but also a team with specialist knowledge.
Finding Enough Data
You might also battle to find enough data to train your model on. Say, for example, you want to develop an application to diagnose rare diseases. There may not be enough cases to properly train your LAM.
We developed a large action model for a manufacturing plant that reduced production line downtime by predicting equipment failures before they happened. The system caught a failing motor bearing that would’ve caused a $50,000 shutdown.
Future Predictions for Large Action Models
Where is this industry going?
Multimodal Integration for AI Workflows
LAMs will soon process text, images, and audio simultaneously, making them more powerful and mainstream as the technology advances.
Human-LAM Collaboration
Rather than replacing humans, LAMs will assist in decision-making. For example, instead of automatically cutting shifts during slow periods, a LAM could alert HR with a recommendation, allowing managers to make informed, empathetic decisions.
About Label Your Data
If you choose to delegate data annotation, run a free data pilot with Label Your Data. Our outsourcing strategy has helped many companies scale their ML projects. Here’s why:
No Commitment
Check our performance based on a free trial
Flexible Pricing
Pay per labeled object or per annotation hour
Tool-Agnostic
Working with every annotation tool, even your custom tools
Data Compliance
Work with a data-certified vendor: PCI DSS Level 1, ISO:2700, GDPR, CCPA
FAQ
Are there any large action models?
Yes, there are large action models like Yes, LAMs like Claude, Rabbit AI, and Salesforce xLAM. There are even open source large action models like Gorilla. Looking for more examples of large action models open source? There aren’t many, but CogAgent is one more to look at.
What is a large action model in finance?
What is the difference between LLM and LAM?
LLMs focus on text-based AI, while LAMs execute actions and interact with different environments.
What are large agentic models?
Agentic models are AI systems that work on their own. They make decisions and execute tasks rather than making simple predictions.
Written by
Karyna is the CEO of Label Your Data, a company specializing in data labeling solutions for machine learning projects. With a strong background in machine learning, she frequently collaborates with editors to share her expertise through articles, whitepapers, and presentations.