Human in the Loop Machine Learning: The Key to Better Models
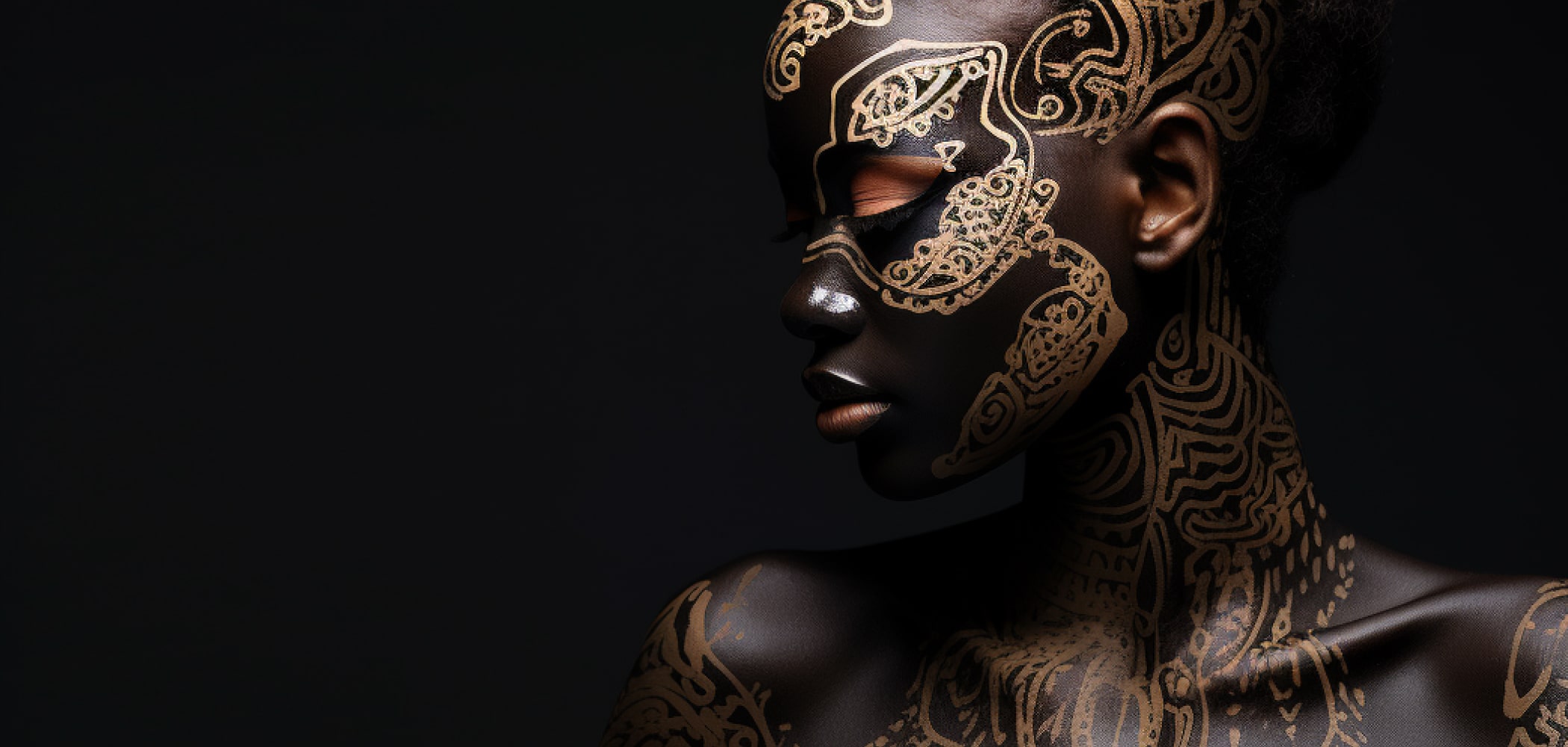
TL;DR
What is Human in the Loop Machine Learning and Why Does It Matter?
Machine learning is becoming an increasingly important concept for modern businesses of all kinds, from fintech to health care and from heavy machinery to retail. And the reason for such popularity is simple: ML allows us to cut corners by making accurate predictions and introducing automation.
However, that doesn’t mean that people are out of work now that the machines have been giving the spark of artificial intelligence. On the contrary: human experts are as valuable as ever, especially in areas like data annotation, where precise labeling is critical for model accuracy. That’s why human in the loop machine learning has been gaining tremendous popularity in recent years.
But there’s more to human-in-the-loop than meets the eye. Aside from why it’s beneficial, it’s also worth acknowledging that there may be different types of “humans” in the machine learning loop.
Defining Human in the Loop Machine Learning
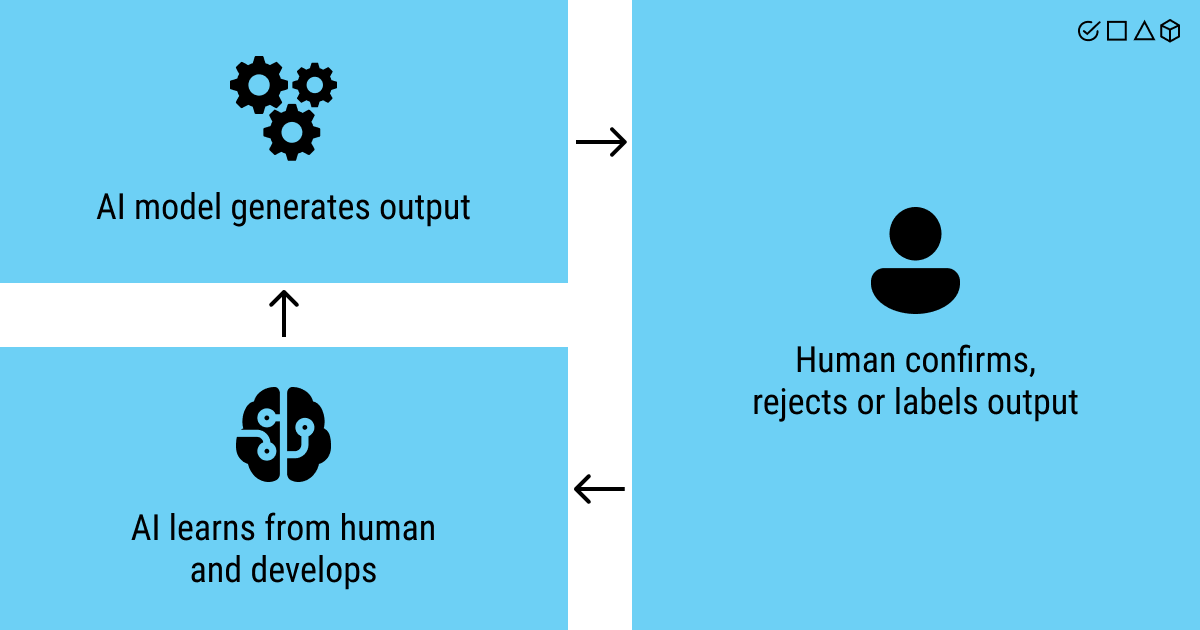
Human in the loop machine learning refers to human involvement in machine learning workflows to reduce errors and improve model performance. In its narrow sense, it involves specialists like data annotators, QA experts, data scientists, and ML engineers, who refine models and ensure data quality.
In a broader sense, human-in-the-loop approach includes product managers, analysts, and domain experts who guide planning, assess model feasibility, and align AI with market needs.
HITL accelerates learning by integrating human feedback, which automation alone cannot fully replace for complex tasks. For example, while tools can pre-label data, human annotators are essential for assigning contextually accurate labels (e.g., distinguishing “name” from “product”). Even with unsupervised learning, some human annotation is often necessary for sophisticated tasks.
Humans remain vital to achieving reliable and effective AI systems. Their role can vary depending on the level of involvement, leading to two primary approaches: Human in the Loop (HITL) and Human on the Loop (HOTL).
Human in the Loop vs Human on the Loop
When it comes to integrating human expertise into ML workflows, there are two primary approaches: Human in the Loop (HITL) and Human on the Loop (HOTL). Both involve human interaction, but their roles and levels of engagement differ significantly.
Human in the Loop (HITL): Humans are directly involved in training, validating, and refining AI models, such as during LLM fine-tuning, where human input ensures the language model aligns with specific use cases. They provide feedback, correct errors, and improve data quality during development stages. This method is crucial for tasks requiring high accuracy or nuanced judgment.
Human on the Loop (HOTL): Humans oversee the AI systems from a supervisory perspective. They intervene only when necessary, such as when the AI encounters edge cases, anomalies, or critical decision points.
Here’s a quick comparison:
Understanding the differences between these two approaches helps teams decide when and where to involve human expertise for optimal AI performance.
Human-out-of-the-Loop
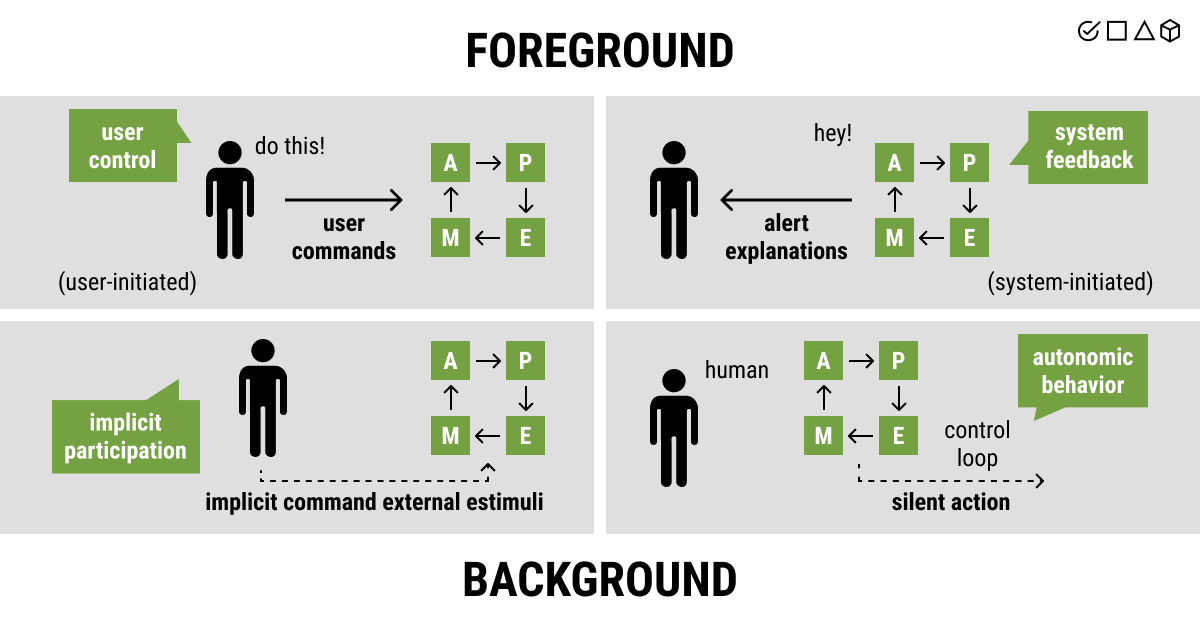
The concept of Human-Out-of-the-Loop (HOTL) focuses on removing human involvement from learning and decision-making processes, allowing machines to operate independently. While this may sound like the opposite of Human-in-the-Loop, its relevance depends on specific applications.
For example, in autonomous driving, taking humans out of the loop can address problems like distracted driving, slow reaction times, and human error—key contributors to road accidents. Fully autonomous systems can potentially reduce risks and improve safety.
Similarly, reinforcement learning enables machines to learn through reward-based systems without human supervision. Although its applications are currently limited to fields like gaming (e.g., chess and Go), it offers potential for future machine learning tasks where independent training is feasible.
In certain high-risk areas, like weapons development and deployment, removing humans from the loop is not just practical but essential to uphold ethical standards and minimize harm. By automating dangerous tasks, HOTL aligns with humanitarian values and reduces potential risks
Human-in-the-Loop Examples: Real-World Use Cases and Benefits
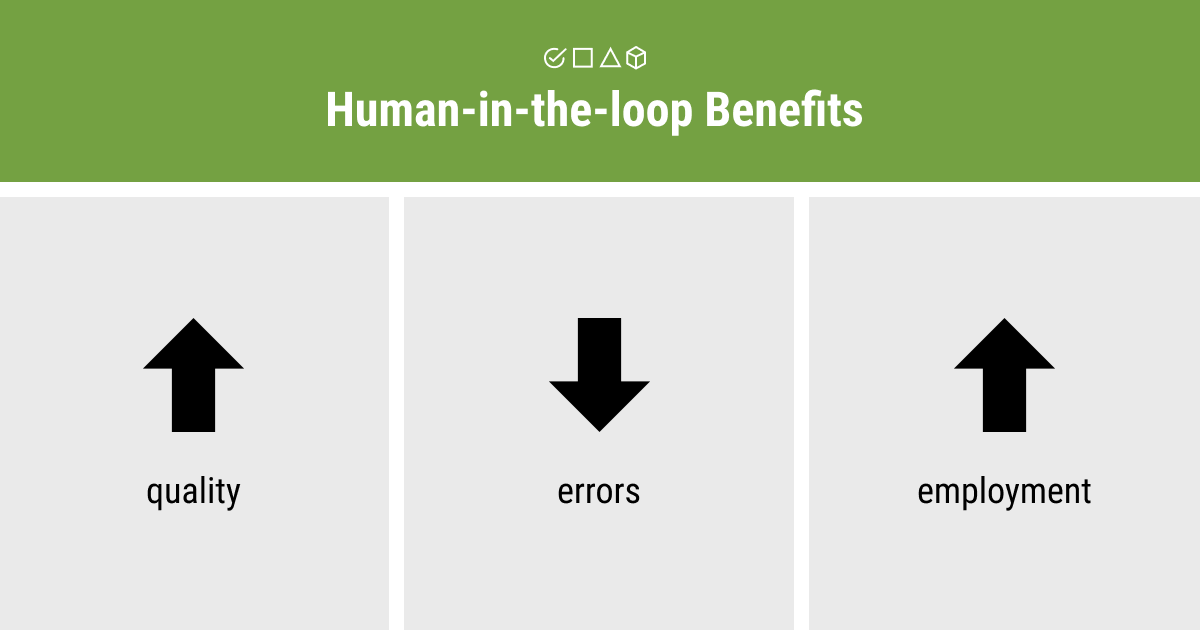
So if human-out-of-the-loop may both be effective and decrease the risks for people, why do we still need the human-in-the-loop concept? The simple answer is that, for now, human-out-of-the-loop still only can be applied within very narrow areas. For a majority of cases, human-in-the-loop is what works best.
The fact is that human-in-the-loop systems bring a number of significant benefits to the process of algorithm development. Still doubtful? Let’s see why this concept is essential for developing a machine learning model. Seek out human in the loop examples to uncover the true power of HITL.
Increasing Quality and Accuracy
Whatever you may give priority to when building a model (from costs to speed to ingenuity), high quality is the only thing that you should never sacrifice. That’s why precision and accuracy become the primary goal when training any machine learning algorithm or model. In situations where safety is paramount, such as aircraft engineering, you cannot go without a human in the loop.
Naturally, automation is a major benefit of introducing machine learning into a workflow. And the combination of ML and human effort seems to give the best results through human in the loop automation: one of the studies proved that such a combined system outperforms both human doctors and ML-only systems in identifying breast cancer based on X-rays. This means that, while the machines are capable of facilitating the work of human experts and people bring in the quality, it’s a good idea to use both to strengthen the overall effect.
Domain expertise is yet another thing you cannot forget about when building a machine learning algorithm. To design a model that could give you a competitive advantage in the market, it’s important to incorporate sophisticated and unique knowledge only available from human domain experts. Such is the case with the Nasdaq discovery tool that aimed to improve the management of trading activities and was built with the help of human analysts.
This human-in-the-loop benefit also considers covering the corner cases, for example, for the models that require rare or insufficient data to properly work. The absence of good data may also serve as a barrier to training ML models without human supervision. Facebook, for example, monitors social activity with the help of human experts, as it’s still hard to teach all the nuances and intricacies of communication.
Lowering the Number of Errors
A great example of human-in-the-loop and its advantages is data quality assurance, or QA. While certain companies such as UiPath champion the idea of “automation first”, where every person gets a robot, it’s important to keep in mind the essential need for quality control. After all, there’s a good reason why large audit companies invest in the human-in-the-loop concept to automate their document workflow. There will obviously be mistakes. Since the training data that the models used is not perfect, there will be errors and blank spots that a machine cannot uncover on its own.
A human in the loop, on the other hand, is capable of spotting the problematic spots before any damage is done and to re-train the ML model. Curiously, a few global manufacturers have started to use deep learning as a QA tool; however, there’s still a need for a human expert to tell a machine what color and curvature a banana should be to stay classified as ripe and/or edible.
Quality and decreasing errors can also be achieved by detecting and avoiding bias in machine learning. As ML models train, it’s sometimes very hard to ensure that the data used (especially if it’s big data) is not biased. Adding a human into the loop to utilize both machine and human intelligence, as well as human ingenuity, can help to see and prevent the spread of bias as soon as it occurs, not in the final testing stages. Remember the case of an autonomous Uber car that hit a woman on the streets of Tempe, Arizona? While many were pointing at people who didn’t know how to use the new technology properly, the responsibility should properly be shifted to the lack of human supervision that could help uncover the biases in training before any fatality occurred.
Employment Opportunities
Last but not least, human-in-the-loop allows negating the popular discourse point about AI taking away jobs from people. On the contrary, using this concept means introducing new, better, more interesting, and ambitious options for people. Human-in-the-loop embraces the highly technological era we’re living in and allows people to strive during this time. An Indian market is the primary example of this, as the data annotators have been bringing significant growth to the national economy during recent years.
Besides, sometimes a human-in-the-loop means that a human expert is placed outside the loop and can serve as an arbitrator who can be addressed in case a machine makes a judgment error. While the Canadian visa officers were in part replaced by robots, there’s always a human officer you can address to re-negotiate a machine-made decision.
How the Human-in-the-Loop Approach Enhances Machine Learning Outcomes
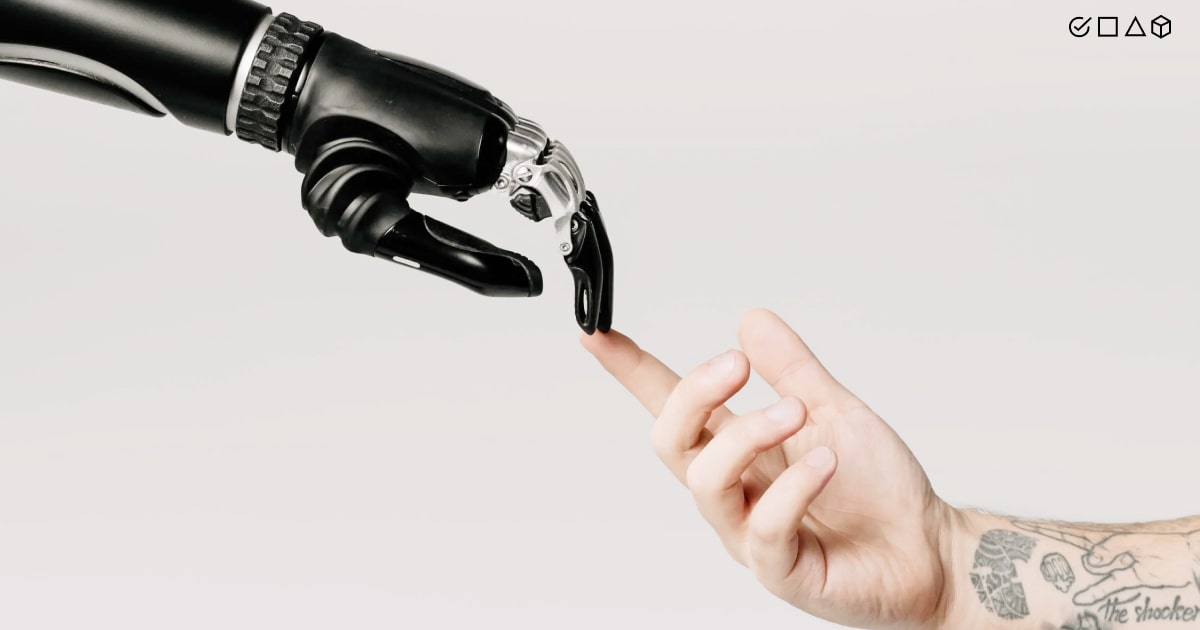
The Human-in-the-Loop approach is essential for ensuring accuracy, addressing edge cases, and improving the overall quality of machine learning models. By combining automation with human expertise, HITL systems deliver adaptable, reliable, and high-performing AI solutions.
Industries are leveraging human in the loop machine learning in diverse ways:
Healthcare: AI-assisted diagnostics benefit from radiologists' insights, leading to more accurate medical imaging results.
Retail: Recommendation engines are fine-tuned with human input to personalize customer experiences.
Autonomous Vehicles: HITL is critical for training models to identify safety-critical scenarios and reduce errors.
To integrate HITL effectively, teams should implement intuitive feedback systems, encourage collaboration between AI and humans, and rely on expert data annotation services like those offered by Label Your Data. This ensures ML models meet real-world demands while retaining ethical and practical oversight.
As AI evolves, HITL remains crucial for delivering smarter, safer, and more trustworthy solutions. Whether it's labeling datasets or guiding complex decisions, human expertise ensures machine learning continues to align with real-world needs.
About Label Your Data
If you choose to delegate data annotation, run a free pilot with Label Your Data. Our outsourcing strategy has helped many companies scale their ML projects. Here’s why:
No Commitment
Check our performance based on a free trial
Flexible Pricing
Pay per labeled object or per annotation hour
Tool-Agnostic
Working with every annotation tool, even your custom tools
Data Compliance
Work with a data-certified vendor: PCI DSS Level 1, ISO:2700, GDPR, CCPA
FAQ
What is human-in-the-loop in machine learning?
Human-in-the-Loop (HITL) in machine learning refers to a process where humans actively participate in training, validating, and improving AI models. They provide feedback, correct errors, and enhance data quality to ensure better model performance, especially for complex tasks where automation alone may fall short.
What is the human-in-the-loop theory?
The human-in-the-loop theory emphasizes the importance of integrating human expertise into AI workflows. It highlights how human involvement can enhance machine learning models by providing context, reducing errors, and enabling better decision-making. The theory underlines the balance between automation and human input to achieve reliable AI systems.
What do you mean by humans in the loop?
Humans in the loop refers to the active involvement of people in machine learning processes. They guide AI systems through tasks like data annotation, validation, and error correction, ensuring accuracy and context that machines can't achieve independently.
What is the difference between human-in-the-loop and out of the loop?
What is an example of the human-in-the-loop concept?
A good example of the human-in-the-loop concept is a content moderation system, where human reviewers oversee and make decisions on flagged or potentially objectionable content alongside automated algorithms.
Written by
Karyna is the CEO of Label Your Data, a company specializing in data labeling solutions for machine learning projects. With a strong background in machine learning, she frequently collaborates with editors to share her expertise through articles, whitepapers, and presentations.