What Is Edge AI?
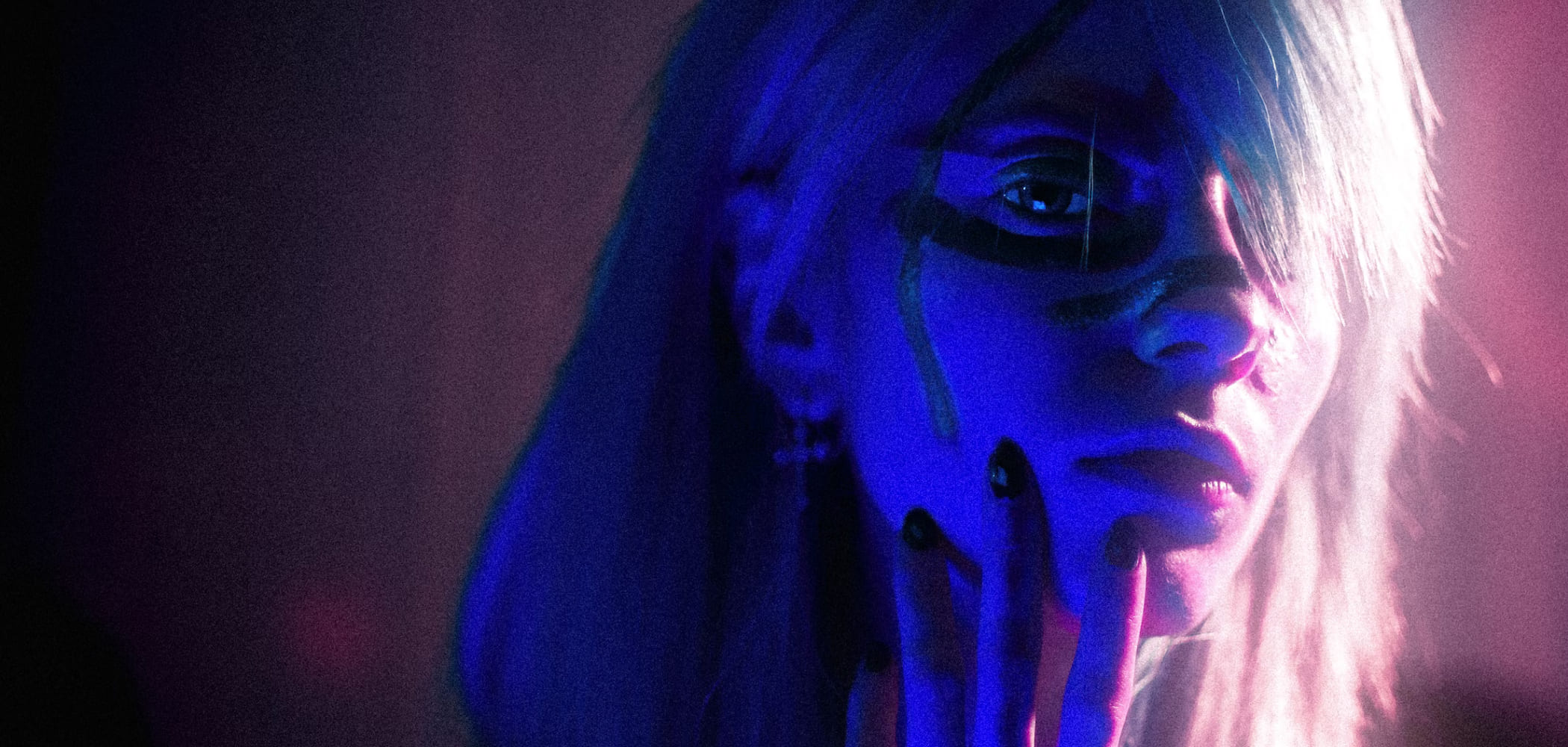
Artificial intelligence was formerly thought of as a futuristic technology. Today, AI has helped us with a lot of issues and is now ingrained in our daily lives. Moreover, AI keeps evolving, as much as humans do. And so the idea of Edge AI was formed. If you’ve never heard of it before, make yourself comfortable and grab a great read from us!
Let’s start with artificial intelligence first. AI is a broad term, but it all boils down to one goal: teaching machines how to mimic human intelligence and behavior, yet as safely as possible. Modern machines are already skilled enough to analyze mass volumes of data, find patterns in this data, provide accurate predictions and recommendations, understand languages, and solve vision issues, as well as other problems that humans are dealing with. All this became possible with the power of automation, which is how artificial intelligence has transformed the entire business landscape as we know it.
What about edge computing? A network architecture known as edge computing brings processing and storage closer to the [edge] sources of data. The typical sources of data usually come in the form of IoT sensors mounted on the machinery. Yet, with the dynamic growth of IoT (Internet of Things) devices, tremendous amounts of data are being gathered. In this case, an edge network can enhance bandwidth, speed up reaction time, and deliver real-time insights by bringing computing capacity closer to key data sources.
What is Edge AI, then? Edge AI is simply a combination of edge computing and artificial intelligence to perform machine learning tasks on connected edge devices. Low latency, ultra-low energy sensors with on-board computing are essential as the IoT develops. Without this architecture, systems would be forced to process data on remote clouds and data centers. There would be no way to unleash the full potential of artificial intelligence.
Edge AI aims squarely at these issues. Pushing AI computation away from the data center and toward the edge is necessary to fully and pervasively integrate AI into the processes that make up our life. By eliminating the requirement for a distant data center — and occasionally circumventing it in a convoluted manner — this method significantly lowers latency (aka the time it takes to process one unit of data).
Thus, Edge AI proves to be a useful solution for businesses with a wide range of applications, including:
- Energy sector: An energy company can employ Edge AI to have network sensors predict power outages;
- Agriculture: Using edge models to teach operational sensors, a farming operation may employ Edge AI to bundle data before transmitting it to the cloud;
- Autonomous industry: Autonomous cars must rely on Edge AI to enable sensors to respond to shifting road conditions;
and many other major industries.
With that said, by bringing intelligence to IoT devices, Edge AI allows monitoring and analyzing data in real-time and with little to no latency. So far, this may only give you a rough idea of what Edge AI is, so we suggest you continue reading this article and learn about all the intricacies of edge computing and artificial intelligence mixed up together. We’ll cover the benefits, core technologies, and interesting use cases of Edge AI across the industry as well!
Edge Computing & Artificial Intelligence in Tune: An Unrivaled Combo
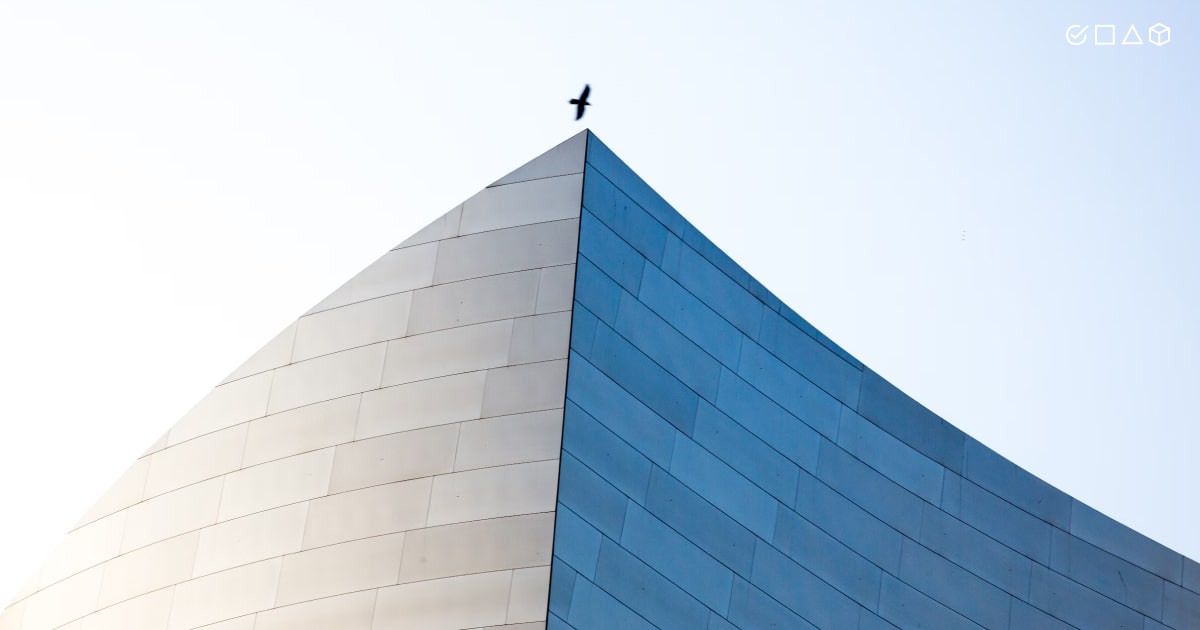
As the name suggests, the workloads in edge computing are positioned as near as possible to the edge, where data is being produced and actions are being made. Therefore, the edge is closer to users than the cloud, which is why it can solve quite a few issues in AI. It makes sense to gradually combine AI and edge computing, mutually enhancing the development of edge intelligence and intelligent edges (yes, these are two different concepts we’ll discuss shortly). Case in point, hardware and software solutions that provide edge functionality have been launched by Google and Amazon Web Services, respectively, as Edge TPU and Wavelength.
The real value behind the concept of Edge AI lies in its capacity to add new processing layers between the cloud and user devices. What’s more, it can divide up application estimates between these layers. A major change in data privacy might result from pushing intelligence to the edge. The information supplied from smart devices, such as TVs and digital speakers, might be managed by specialized chips and cloudlets (aka small-scale data centers) that operate in a house, place of work, or a vehicle.
A straightforward but significant difficulty lies at the core of Edge AI: how to have computing systems make decisions at the same rate as the human mind and in real-time? Any system containing AI must run without experiencing a major decrease in speed and accuracy in order for it to completely develop. Typically, latency under 10 milliseconds is required for this. However, the response time of modern clouds is around 70 milliseconds or more, and wireless connections are significantly slower.
The existing method of driving data streams via a handful of sizable datacenters limits the potential of developing digital technologies. Edge AI, in turn, offers a whole new approach. Instead of implementing algorithms in distant clouds and remote data centers, it does it locally on chips and specialized hardware. What does this mean for smart IoT devices? A device may function without maintaining a constant connection to a specific network or the Internet, and it can access external connections and transmit data as needed.
In Simple Words on a Complex Matter: More of Edge AI Explained
The data received from a hardware device is usually processed by Edge AI systems using machine learning algorithms. Data processing is performed to make further decisions in real-time, however, this also requires a computer to be connected to the Internet.
This substantially lowers the connection expenses of the cloud model. So, we can assume that Edge AI brings data and the processing stage much closer to the user, or rather the devices they use, such as smartphones, IoT systems, or an edge server. This is Edge AI in layman’s terms. As data experts ourselves, we’d also like to talk about Edge AI from a deeply technical perspective.
How to make sense of Edge AI systems? First, it’s important to learn about the first essential component of Edge AI. Edge computing can best be understood by comparing it with cloud computing. The latter refers to the process of delivering computing services over the Internet. Edge computing systems, in contrast, are not linked to the cloud, they run on local devices instead (think dedicated edge computing server, a local computer, or an IoT device). As such, edge computing bears a lot of benefits because it’s unconstrained, while cloud-based systems are limited by latency and bandwidth.
Now, we can move on to deciphering the idea of Edge AI, where algorithms run on computers with edge computing capabilities. This way, we can have our data processes in real-time with no need for cloud connectivity. Cloud is a viable asset when it comes to sophisticated data-related processes in AI that require a considerable amount of computing power. As a result, these AI systems are robust against outages.
The necessary data processes may be completed locally before being transferred over the Internet, saving time, because Edge AI systems operate on an edge computing system. The data starts on the computer, where deep learning algorithms will operate. This way, Edge AI grows in value as more and more devices require artificial intelligence when they can’t access the cloud.
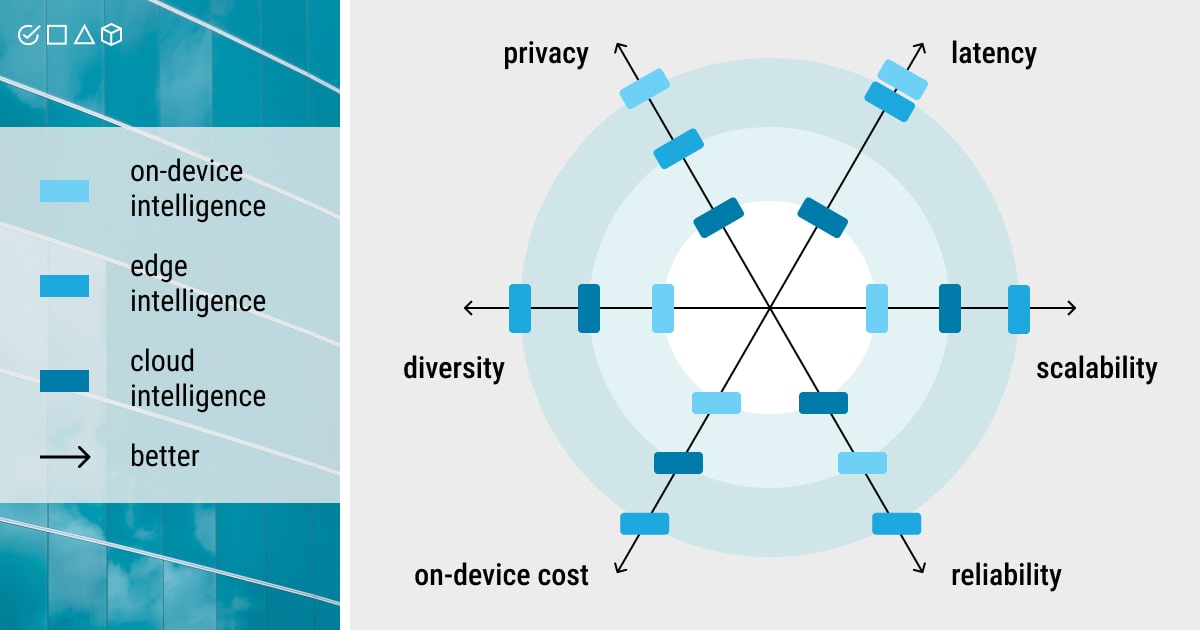
Top 5 Benefits of Edge AI
Edge AI simplifies decision-making, protects data processing, improves user experiences, lowers costs, and increases energy efficiency in smart devices. But this only accounts for a small portion of why Edge AI is so well-liked right now, namely:
- Decreased latency
- Scalability
- Real-time analytics
- Lower expenses
- Data privacy and security
How do these benefits play out in Edge AI? Let’s see!
The network undergoes significant modifications as decision-making and other tasks are pushed to the edge. An autonomous car, for instance, may utilize its onboard machine learning to dynamically adjust to various driving situations and conditions on the road. A system of sensors in a home or medical facility might monitor patients, particularly the elderly, more effectively and identify possible issues, such as a patient’s incapacity to get out of bed or neglecting to take their prescriptions.
Additionally, Edge AI might keep tabs on the condition of subterranean pipelines for decades without the need to replace a battery in a difficult-to-reach sensor. Yet, even though many of these tasks are feasible without Edge AI, cutting off the round trip to the cloud will drastically change their functionality.
Wake-on-command features are yet another intriguing aspect of edge AI. When a gadget is not in use, these methods may reduce power usage almost to zero. Some devices can now function for years or even decades without a recharge or a new battery thanks to this technology. This functionality would be useful for remote video cameras, medical implants and other devices, and embedded sensors.
Wearable tech that is centered on user experience, such as bracelets that track your sleep and workout routines in real-time, is easier to integrate thanks to Edge AI. This is due to latency times, lowered costs, and enhanced user experience that we’ve discussed above.
The agreed rates for Internet service may technically drop when the required bandwidth is reduced. Also, technologies that rely on Edge AI systems don’t require any data scientist or AI developer to be involved in their maintenance because it’s an autonomous technology.
Intelligent Edge & Edge Intelligence: What Is the Difference?
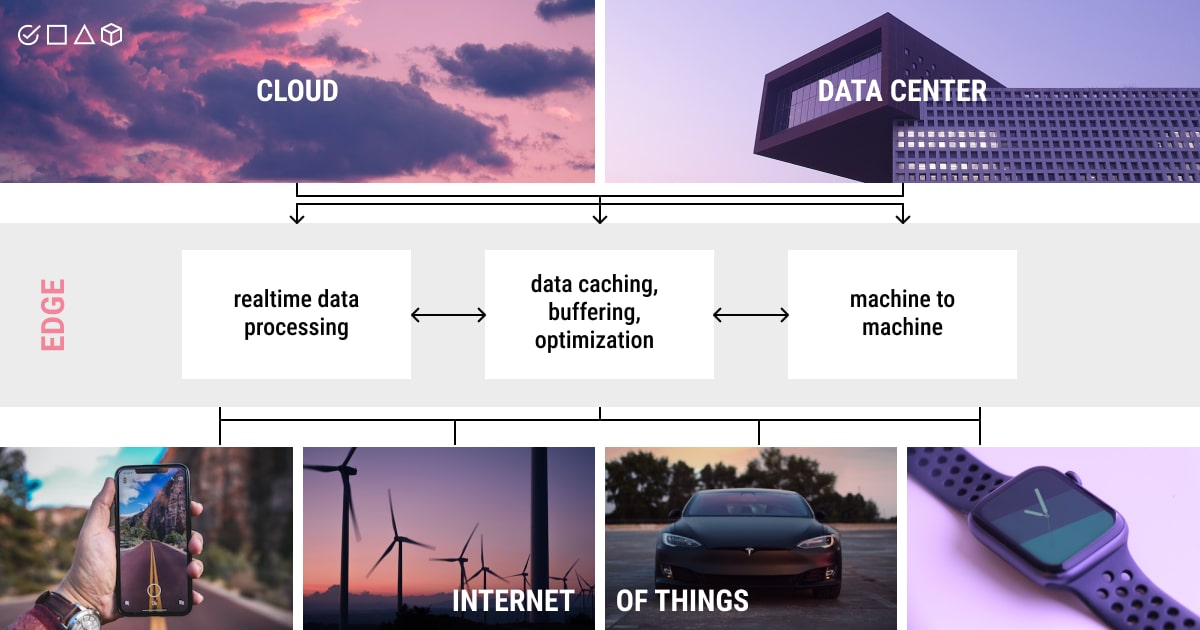
The terms intelligent edge and edge intelligence are not mutually exclusive. Edge intelligence is essentially the goal, while AI services in intelligent edge are part of the objective. Edge intelligence can then use intelligent edges to increase service throughput and resource consumption.
More specifically, edge intelligence enables dispersed, low-latency, and dependable intelligent services by pushing AI calculations from the cloud to the edge as much as feasible. The consequences are fairly positive:
- AI services are set up locally to the users who are making requests. Plus, the cloud comes into play only when further processing is necessary. This greatly reduces the latency and expense of transferring data to the cloud for processing;
- The protection of user privacy is improved since the raw data needed for AI services is saved locally on the edge or user devices themselves rather than in the cloud;
- AI computation becomes more accurate when it is done using a hierarchical computing architecture;
- Edge computing facilitates the widespread use of AI, making it accessible for everyone and everywhere with richer data and applications scenarios at hand;
- Due to the diversity and benefits that AI services deliver, edge computing’s economic value may be expanded, speeding up the adoption and expansion of Edge AI.
The intelligent edge, in contrast, intends to integrate AI into the edge for dynamic, adaptive edge maintenance and management. The variety of network access techniques is growing along with communication technologies. Additionally, the edge computing infrastructure serves as a middleman, enhancing the dependability and persistence of the connection between ubiquitous end devices and the cloud. As a result, a community of shared resources is being gradually formed from the end devices, edge, and cloud.
Yet, it’s quite a challenge to maintain and administer such a sizable and intricate overall architecture (community), which includes wireless communication, networking, computation, storage, and so on.
Edge AI Technologies: What Problems Do They Solve?
The following five technologies are commonly identified as being crucial for Edge AI given that edge intelligence and intelligent edge, or Edge AI, share some of the same problems and practical concerns in many aspects:
- AI applications on Edge — technical frameworks for methodically combining AI and edge computing to offer intelligent services.
- AI inference in Edge — concentrating on the accurate and timely deployment and inference of AI inside the edge computing architecture to meet various requirements.
- Edge computing for AI — enhances the network architecture, hardware, and software of the edge computing platform to enable AI computation.
AI training at Edge — training AI models for edge intelligence at distributed edge devices, considering resource and privacy limitations. - AI for optimizing Edge — AI-driven solution for controlling and maintaining various edge computing networks (systems), such as edge caching and compute offloading.
As you may observe, Edge AI becomes more and more ubiquitous due to the growing number of devices that require artificial intelligence when they don’t have access to the cloud. Some good examples are manufacturing robots or self-driving vehicles that rely on computer vision (CV) algorithms.
In these particular cases, Edge AI might prevent hazardous outcomes of the delay in data transmission and save human lives (because there’s no room for latency when an unmanned vehicle detects objects on the road). Therefore, an Edge AI system allows the devices to respond fast as possible by analyzing data with no need for a cloud link.
Closing Remarks: Let’s Discover New Powers of AI, Shall We?
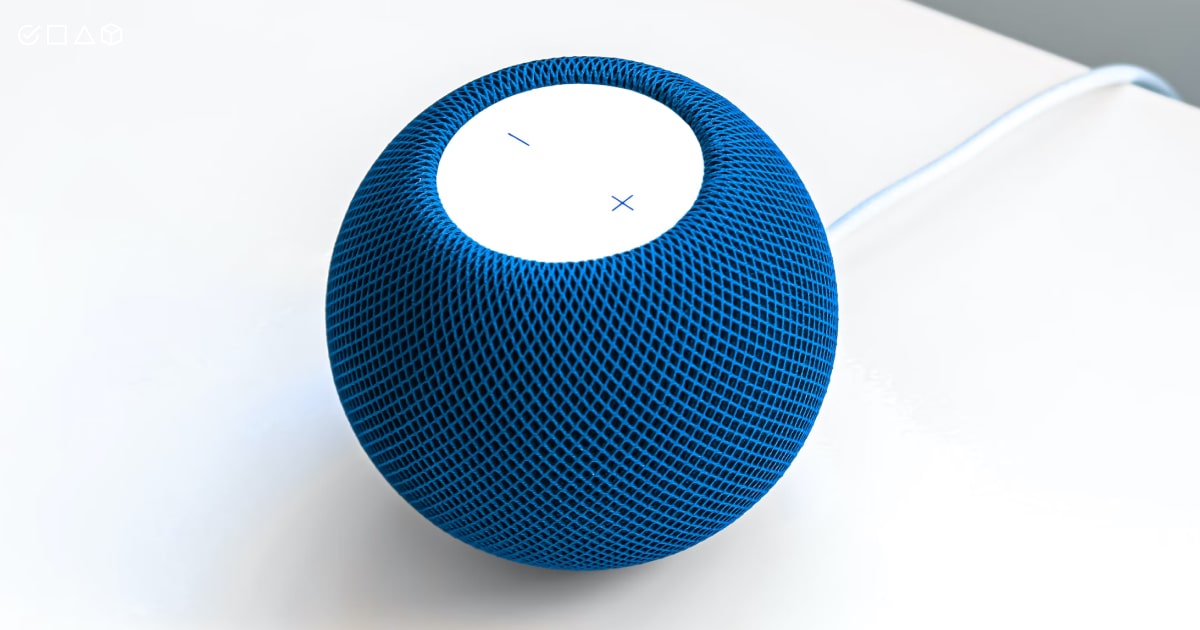
Although Edge AI technology has concerns, such as how to best address physical security and cybersecurity, the paradigm is gaining popularity and momentum. Cloudlets that are widely used would significantly alter how processes work in AI, how data flows, and how machines make decisions. This way, Edge AI seems a promising technology that may give smart gadgets a brand-new, cutting-edge functionality.
Indeed, artificial intelligence and edge computing complement each other quite well, but the challenge remains. That is, bringing AI to the edge of the network through designing and building edge computing architecture under the multiple constraints of networking, communication, computing power, and energy consumption to achieve the best performance of AI training and inference.
Nevertheless, Edge artificial intelligence will become more prevalent as the computational capacity of the edge rises. Intelligent edge, in turn, will serve a crucial supporting function in helping to enhance the performance of Edge AI.
What about boosting the performance of your own AI project? Send your data to our team of annotation experts and scale your project to new heights with machine-readable data from Label Your Data!
Written by
Karyna is the CEO of Label Your Data, a company specializing in data labeling solutions for machine learning projects. With a strong background in machine learning, she frequently collaborates with editors to share her expertise through articles, whitepapers, and presentations.